Taking the 1.7K Shenzhen Residential Housing Price As an Example, the Zhejiang University GIS Laboratory Uses the Attention Mechanism to Mine Geographic Context Features and Improve the Accuracy of Spatial Non-stationary Regression.
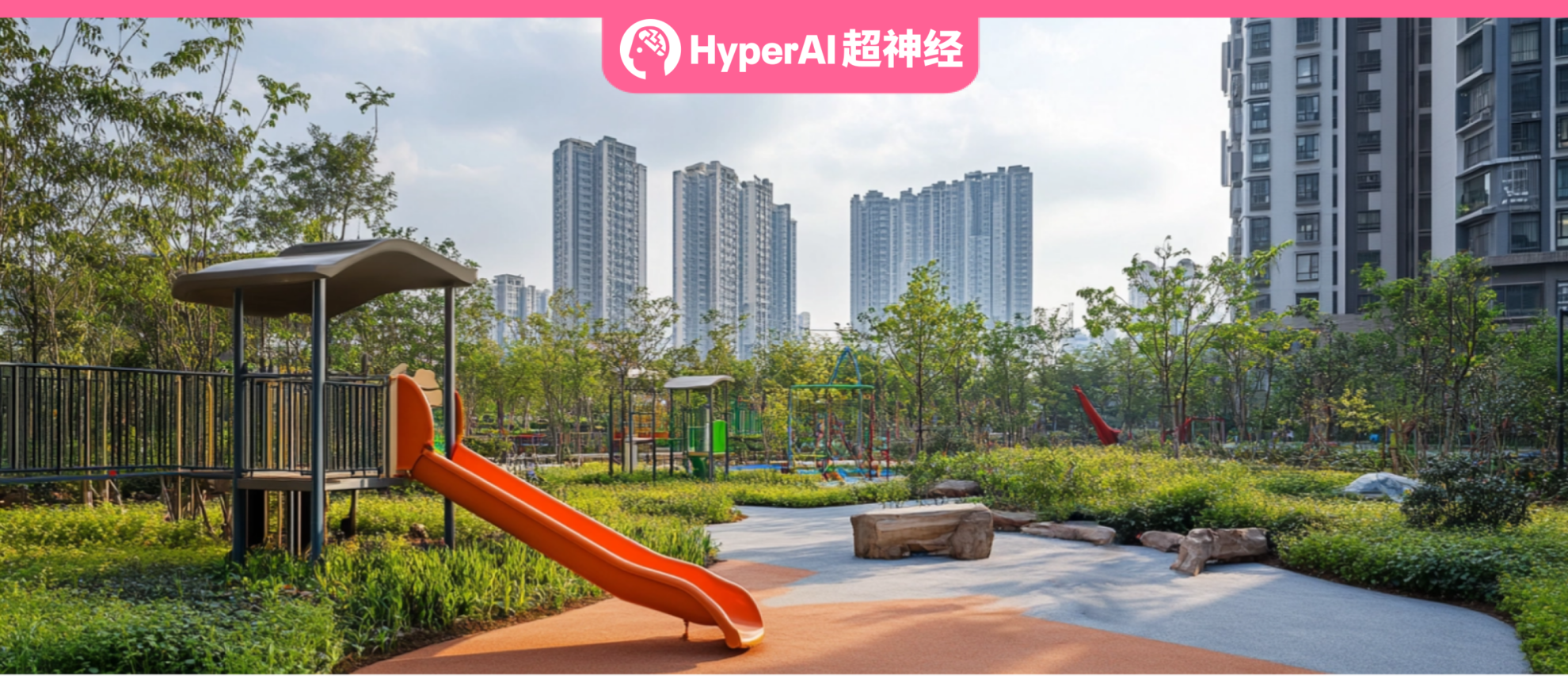
Geographically Weighted Regression (GWR) is a statistical method widely used in geospatial analysis to capture the spatial non-stationarity (i.e., spatial heterogeneity) of geographical phenomena. Traditional GWR assigns weights to each observation point to reflect its influence on the regression parameters. These weights are usually calculated based on spatial distance (such as Euclidean distance), following the principle of "the closer the distance, the greater the influence". However,This distance-based approach ignores the complex contextual similarity of geographical phenomena.For example, similarities in socioeconomic factors or environmental characteristics may have important effects on regression models. For example, in an urban environment, two distant areas may show similar housing price characteristics due to similar socioeconomic or environmental factors such as transportation convenience and population structure.
To solve this problem,Researchers from Zhejiang Provincial GIS Key Laboratory proposed a deep learning model based on attention mechanism, Context-Attention Geographically Weighted Regression (CatGWR).The model introduces an attention mechanism to combine the spatial distance and contextual similarity between samples, thereby more accurately estimating spatial non-stationarity. This innovation provides a new perspective for geospatial modeling, especially when dealing with complex geographical phenomena, and can better capture spatial heterogeneity and contextual influences.
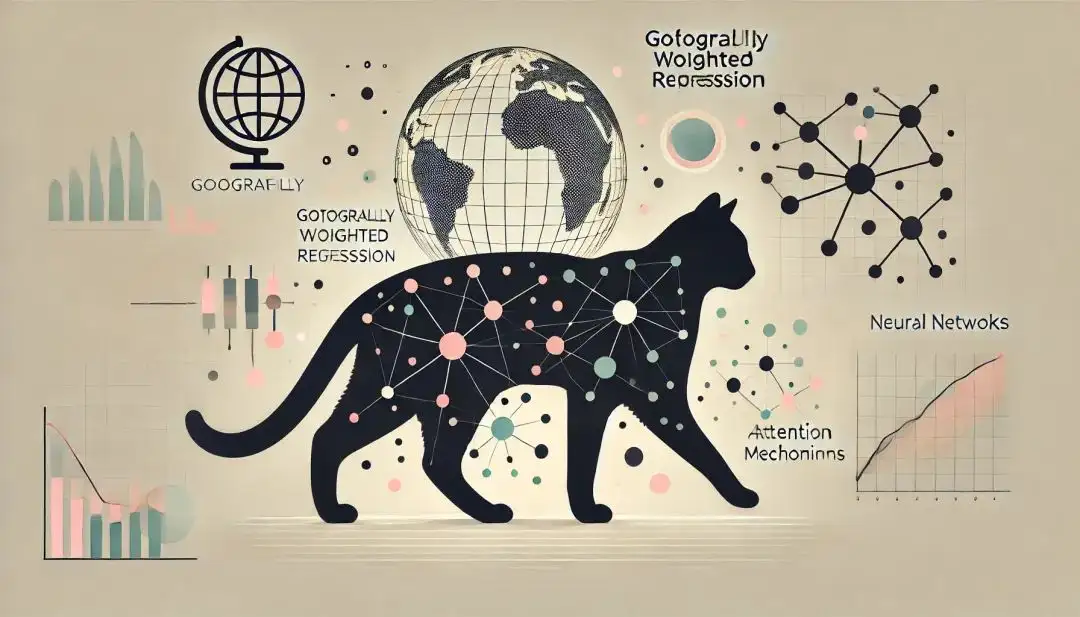
The related results were published in the International Journal of Geographical Information Science under the title "Using an attention-based architecture to incorporate context similarity into spatial non-stationarity estimation".
Research highlights:
* The CatGWR model introduces an attention mechanism to calculate the contextual similarity between samples, which can effectively avoid noise interference in contextual features and obtain a more accurate similarity expression.
* The CatGWR model has significant accuracy improvements on both simulated and empirical datasets and provides more detailed interpretation directions.
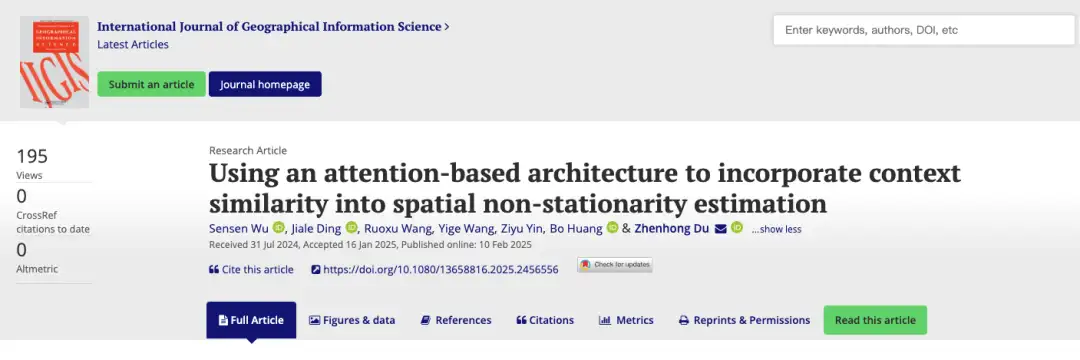
Paper address:
https://doi.org/10.1080/13658816.2025.2456556
Project open source address:
https://github.com/yorktownting/CatGWR
Dataset: Combination of simulation experiments and actual cases
This paper verifies the effectiveness of the CatGWR model through simulation experiments and actual case studies.The simulation experiments used two 64×64 synthetic datasets (S1 and S2), designing scenarios with contextual heterogeneity and only spatial heterogeneity, respectively.These datasets construct regression relationships through simulated situational properties such as spatial heterogeneity and random distribution characteristics, thus providing a controllable experimental environment for model performance evaluation.
The actual case study takes the housing price data of Shenzhen, China as an example.Shenzhen is a typical example of China's rapid urbanization, and housing prices show significant spatial heterogeneity. The research data includes housing price samples of 1,776 residential communities, as well as 7 independent variables related to housing prices (such as building age, management fees, greening rate, etc.). In addition, the study also introduced 6-dimensional taxi passenger data as contextual features. These data can reflect urban dynamics and human activity patterns, providing rich spatial and contextual information for the model.
Model Architecture: Geographically Weighted Regression Driven by Contextual Attention
The CatGWR model uses an additive attention mechanism to calculate geographic context similarity and combines it with spatial distance weights.The model is divided into three modules: Preprocessor Module, Amplifier Module and Regression Module.As shown in the following figure:
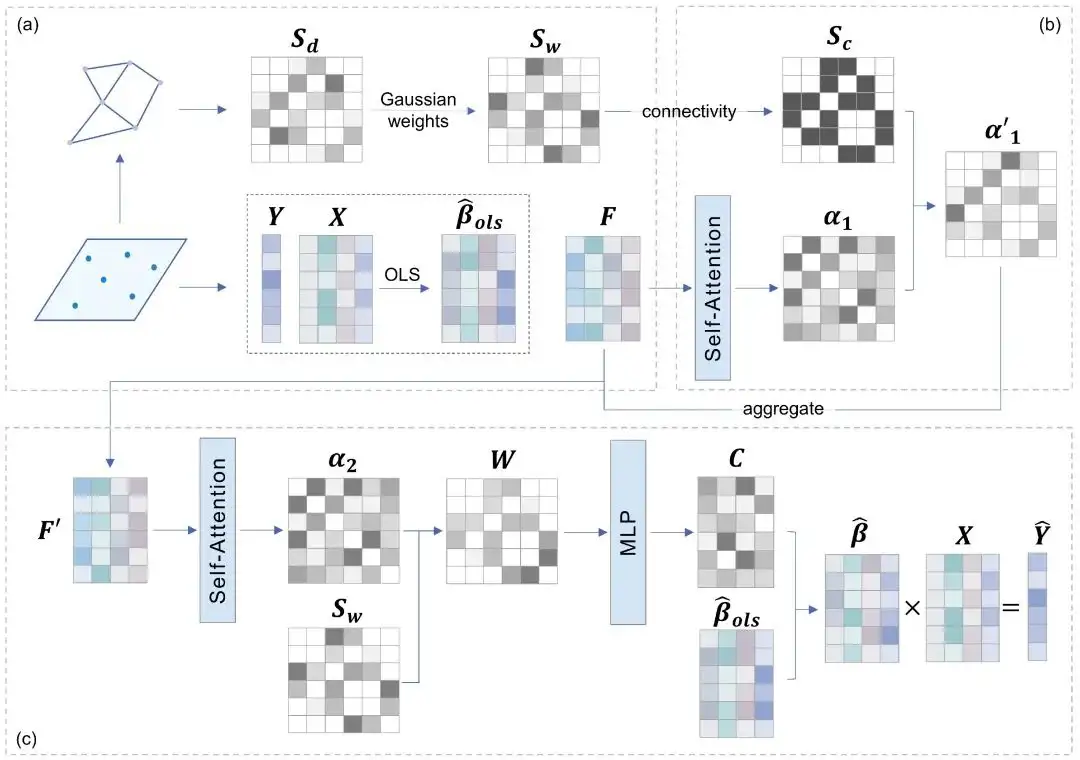
(a) Preprocessing module:Responsible for extracting dependent variables, independent variables and contextual features from the input data, and calculating the spatial weight matrix and spatial connectivity matrix between each sample and its neighborhood.
(b) Amplification module:Expand the receptive field of the model and enhance the model's utilization of neighborhood information.
(c) Regression module:The contextual similarity between samples is calculated through the attention mechanism, and it is combined with the spatial weight matrix to obtain the contextualized spatial weights; the multi-layer perceptron (MLP) is used to convert the contextualized spatial weights into regression coefficients, thereby realizing the estimation of spatial non-stationarity.
Experimental conclusion: revealing the spatial non-stationarity of the determinants of Shenzhen housing prices
This paper verifies the effectiveness of the CatGWR model through simulation experiments and empirical research on Shenzhen housing prices.In the simulation experiment, we first simulated and generated the contextual variables of four geographic scenarios, and then used the generated contextual variables to further construct two sets of simulated data sets: S1 (the contextual variables participated in the data set generation as part of the coefficients) and S2 (the contextual variables were irrelevant to the regression relationship and became the noise of the input CatGWR). The experimental results show that:
* In the scenario scenario (S1),CatGWR can more accurately resolve scenario similarity and effectively couple it with spatial proximity, significantly outperforming existing models such as GWR, MGWR, CGWR and GNNWR.
* In the non-contextualized scenario (S2),Even if “context variables” that are irrelevant to the dataset are introduced as noise, due to the robustness of the attention mechanism used by CatGWR, its performance is still not inferior to the traditional GWR model.
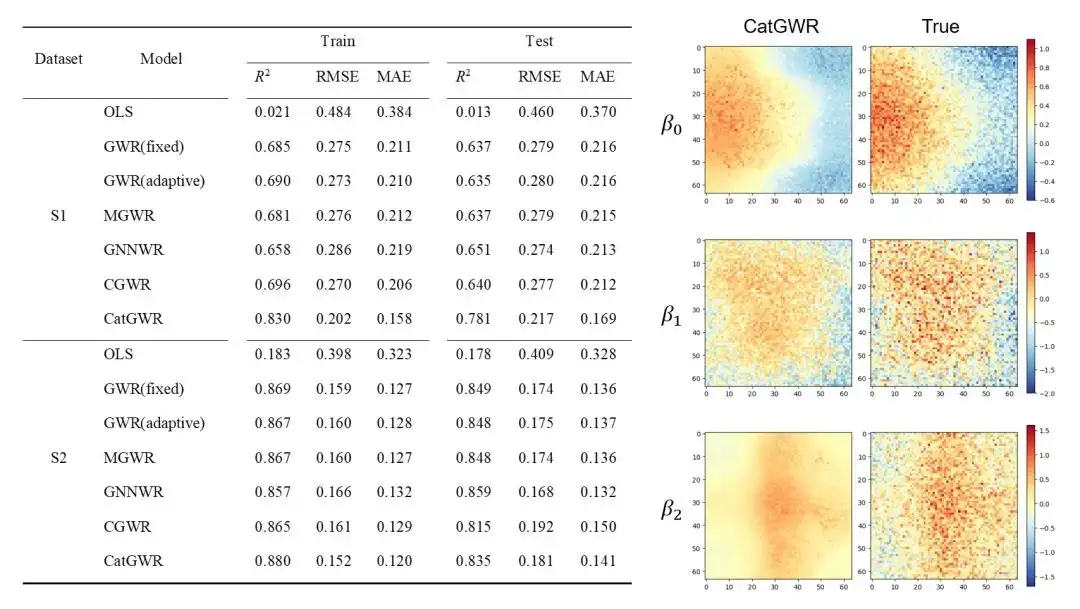
On the Shenzhen housing price dataset, the CatGWR model further demonstrates its superiority.Compared with the existing models, the R² value of CatGWR on the training set increased from 0.853 to 0.920, and the R² value on the prediction set increased from 0.717 to 0.764, and the RMS E and MAE decreased by 28% and 26% respectively.
also,The CatGWR model also reveals the spatial non-stationarity of the determinants of Shenzhen housing prices.For example, near Shenzhen Bay, due to the influence of Shenzhen-Hong Kong commuting residents brought by the Shenzhen-Hong Kong Western Corridor, the impact of the number of supporting parking spaces on housing prices is more significant than in other areas. At the same time, the "similar distance but different weights" characteristics of the situational spatial weights between samples also reflect the characteristics of urban construction and zoning in Shenzhen. This shows that CatGWR can effectively capture the impact of spatial heterogeneity and situational similarity on housing prices.
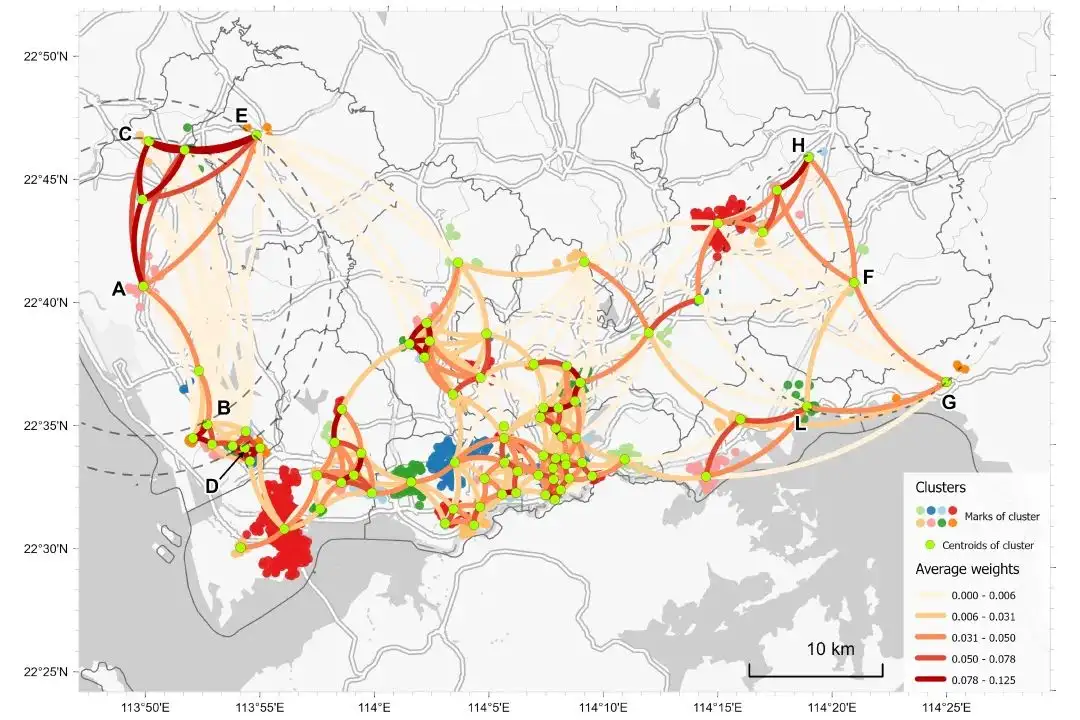
* Urban-rural differences caused by the construction of special economic zones (weights AE > AD, AC > AB at similar physical distances)
* Differences in land use types (satellite town-scenic area) (FH > FL, FG > FL)
The CatGWR model successfully combines scene similarity with spatial proximity by introducing the attention mechanism, significantly improving the accuracy and robustness of spatial non-stationarity modeling.This model not only performs well in simulating data, but also demonstrates strong fitting capabilities in practical applications, providing new ideas and methods for geographic process modeling.
Using housing price forecasts to scientifically explain geographical processes
April 2024The research team of Zhejiang Provincial GIS Laboratory also published a paper on the same research field in the International Journal of Geographical Information Science.The spatial proximity metric optimized by neural network is further combined with the Geographically Neural Network Weighted Regression (GNNWR) method to construct the osp-GNNWR model, which realizes the training of neural network by solving the spatial non-stationary regression relationship between dependent variables and independent variables.
Paper link:
https://www.tandfonline.com/doi/full/10.1080/13658816.2024.2343771
Coincidentally, this study used Wuhan real estate data as an example for research and verification. The experimental results showed that the osp-GNNWR model has potential advantages in depicting the spatial heterogeneity of real-world geographical processes.
The study's author is Ding Jiale, a doctoral student in remote sensing and geographic information systems at Zhejiang University.He once introduced in an online academic sharing session, "As an explorer of geographical science, if the model we come up with can only simply predict housing prices, then such results are boring in my opinion. What we pursue is to use the series of regression coefficients output by these models that vary with spatial location to make reasonable scientific explanations of geographical processes or patterns. Such research is more practical."
It is true that research related to earth science may be hidden in the high-rise buildings of the city or sailing on the top of mountains, rivers, lakes and seas, but it will eventually land on this land to help people better understand geographical processes and explore the meaning behind geographical phenomena. In recent years, with the continuous advancement of observation technology, spatiotemporal data in the field of earth science has shown explosive growth, which has further promoted the implementation of emerging technologies such as AI in the field of earth science.
Zhejiang Provincial GIS Key Laboratory is a pioneer in interdisciplinary research between AI and Earth Sciences.Combining the concept of traditional geographical weighted regression with neural network technology, a series of innovative models are proposed, including geographic neural network weighted regression (GNNWR) and geographic spatiotemporal neural network weighted regression (GTNNWR).
Since the publication of the first paper, GNNWR, GTNNWR and other series of methods have attracted much attention and have been widely used in oceanography, geography, atmospheric science and geology. The team has published more than 30 related papers. At the same time, the relevant results have also inspired other teams in the industry. Many external teams use similar modeling ideas or technical architectures to carry out research, which is precisely the charm of open source research.
GNNWR open source address: