Academic Sharing | Tsinghua University Postdoctoral Fellow Li Yuzhe Explains the Cell/Nature Sub-journal Paper in Detail, Exploring the Application of AI in Genomics
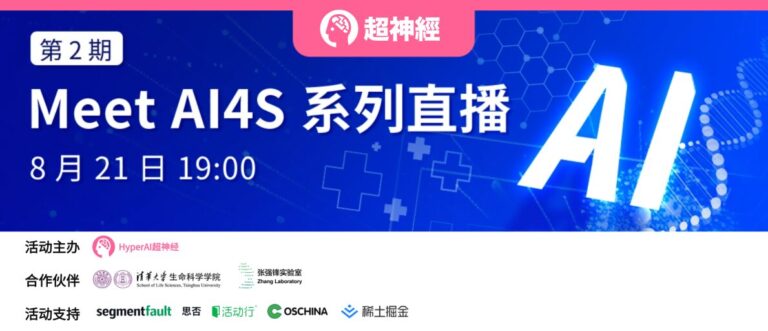
The second episode of the "Meet AI4S" series of live broadcasts invited Li Yuzhe, a postdoctoral fellow in Zhang Qiangfeng's laboratory at Tsinghua University. On August 21, Dr. Li Yuzhe will further share with everyone the AI methods in spatial transcriptomics and single-cell omics research in the form of an online live broadcast.
Spatial transcriptome technology is one of the major breakthroughs in the field of bioinformatics in recent years.It was named Technology of the Year by Nature Method in 2020.
Based on spatial transcriptome technology, we can not only obtain high-resolution transcriptome data, but also correspond it with position information to determine the spatial distribution and positional relationship of different cell subtypes or transcriptional states.
With the continuous development and iteration of spatial transcriptomics technology, researchers can obtain the gene expression profile of cells at single-cell resolution while retaining the spatial location information of cells within the tissue.How to effectively use this spatial information to identify spatial cell subtypes and discover tissue modules has become a core task in spatial transcriptome data analysis.
In recent years, the AI wave has surged into the scientific research field, and has also provided innovative ideas for spatial transcriptomics and single-cell omics research.
For example,The research group of Associate Professor Qiangfeng Zhang from the School of Life Sciences at Tsinghua University has developed an artificial intelligence algorithm called SPACE based on the deep learning framework of graph autoencoders.The ability to identify spatial cell types and discover tissue modules from spatial transcriptome data at single-cell resolution can be used for large-scale spatial transcriptome studies.
In the second episode of the "Meet AI4S" live broadcast series, HyperAI was fortunate to invite Li Yuzhe, the first author of the research paper and a postdoctoral fellow in Zhang Qiangfeng's laboratory at Tsinghua University. On August 21, Dr. Li Yuzhe will further share with everyone the AI methods in spatial transcriptomics and single-cell omics research in the form of an online live broadcast.
Scan the QR code to schedule a live broadcast:
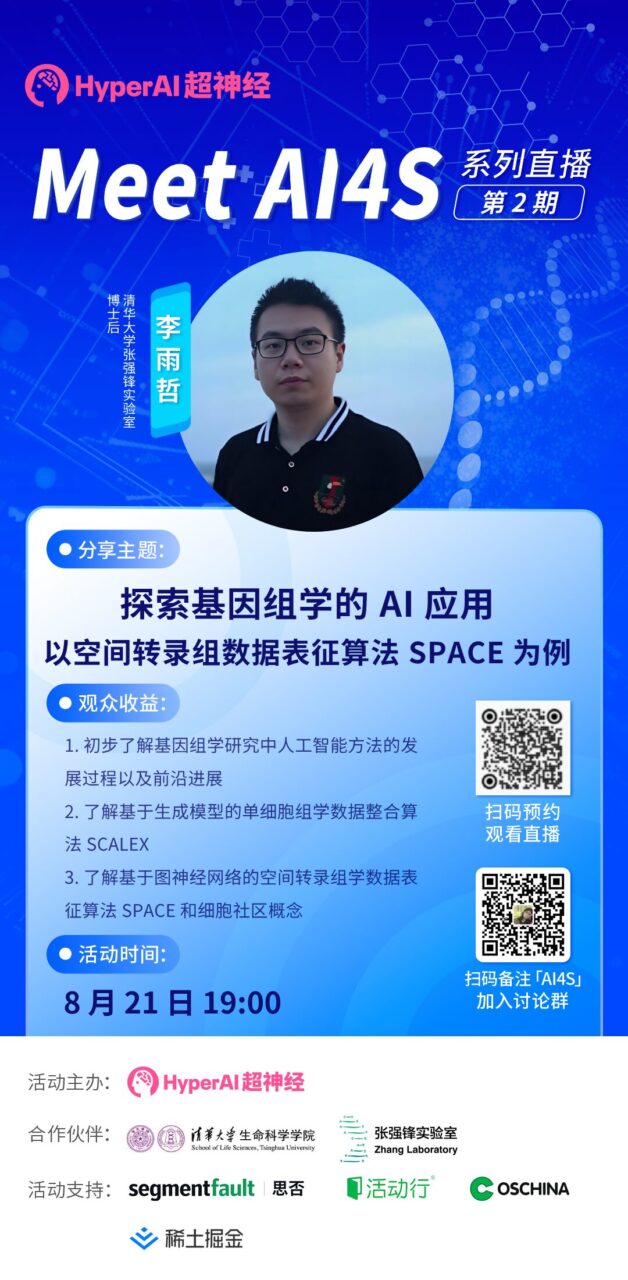
Paper Review
HyperAI has previously interpreted and shared a research paper titled "Tissue module discovery in single-cell resolution spatial transcriptomics data via cell-cell interaction-aware cell embedding" with Dr. Li Yuzhe as the first author.
Research highlights
* Developed SPACE, an artificial intelligence analysis tool for spatial transcriptome data, which can identify spatial cell types and discover tissue modules from spatial transcriptome data with single-cell resolution.
* SPACE significantly outperforms other tools in cell type identification and tissue module discovery, especially in complex tissues containing multiple cell types.
* SPACE defines and discovers cell communities, i.e., tissue modules composed of spatially homogeneous cell types with recognizable boundaries.
* Cell communities are defined by similar interaction networks among the cells they comprise, which can be used to refine ligand-receptor based inferences about cell communication.
* SPACE can be used for large-scale spatial transcriptome studies to understand how interactions between spatially neighboring cells affect the biological functions of cell types and tissue modules.
Dataset acquisition
In order to verify the capabilities of SPACE, multiple datasets were used in the study. Download address:
https://hyper.ai/datasets/32698
Model architecture: A cell-cell interaction-aware cell-embedded model
SPACE uses a graph autoencoder framework to learn low-dimensional cell embeddings, which describe the gene expression information of each cell in the spatial transcriptome data and its interaction information with spatial neighboring cells (so the cell embedding is called cell-cell interaction-aware cell embedding). Based on this cell embedding, SPACE uses a clustering algorithm to identify spatial cell subtypes and discover tissue modules.
From the architecture point of view, the SPACE model consists of three parts: encoder (three-layer graph attention network), neighbor graph decoder and gene expression decoder. The following figure shows the overall framework of the model:

Performance Evaluation
* SPACE can identify biologically distinct cell types based on the spatial information in the ST dataset.
* SPACE outperforms currently available tools for distinguishing spatially informative cell types from ST data.
* SPACE outperforms state-of-the-art tools in tissue module discovery.
Tsinghua University Zhang Qiangfeng Laboratory
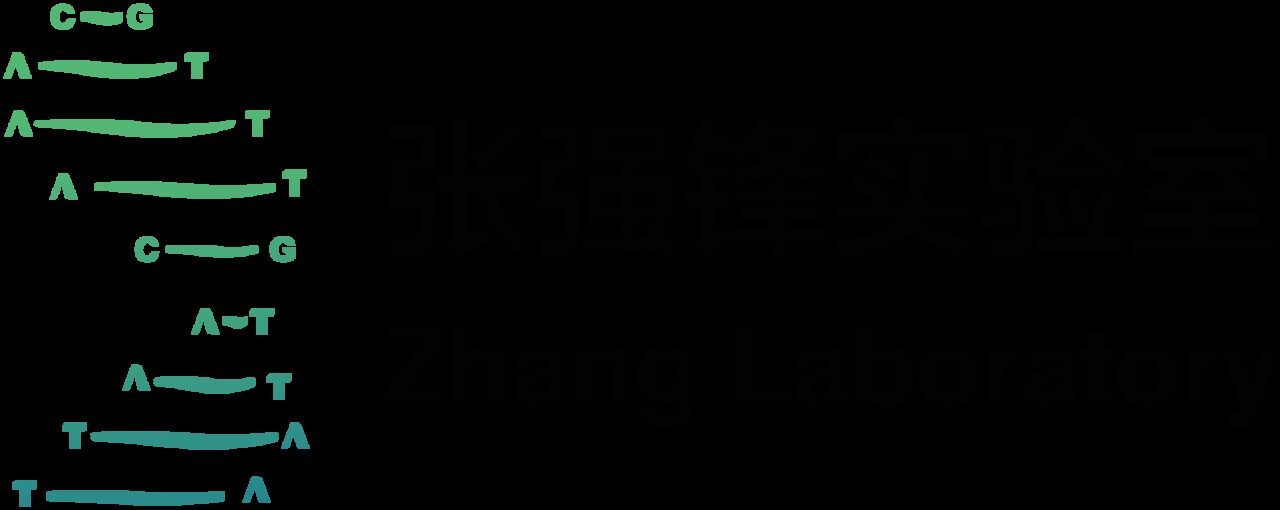
Zhang Qiangfeng's laboratory is affiliated with the School of Life Sciences of Tsinghua University. It is also an important part of the Tsinghua-Peking University Joint Center for Life Sciences and the Beijing Advanced Innovation Center for Structural Biology.
The laboratory's research focuses on interdisciplinary fields such as structural biology, genomics, machine learning and big data analysis. The main research direction is to combine structural biology and systems biology, develop and use a combination of computational and experimental methods to interpret the structure and function relationship of biological macromolecules (such as proteins, RNA, DNA), reconstruct their interaction networks, and discover the pathogenesis and possible treatments of complex diseases (including cancer and infectious diseases) related to changes in protein and RNA structure and abnormal macromolecular interactions.
The laboratory has unique protein and RNA structure modeling, RNA structure measurement based on next-generation sequencing, high-throughput RNA-protein interaction detection technology, and powerful computational and experimental platforms to advance researchers' cutting-edge research.
Meet AI4S Live Series
HyperAI (hyper.ai) is China's largest search engine in the field of data science. It focuses on the latest scientific research results of AI for Science and tracks academic papers in top journals such as Nature and Science in real time. So far, it has completed the interpretation of more than 100 AI for Science papers.
In addition, we also operate the only AI for Science open source project in China, awesome-ai4s.
* Project address:
https://github.com/hyperai/awesome-ai4s
In order to further promote the popularization of AI4S, further reduce the dissemination barriers of scientific research results of academic institutions, and share them with a wider range of industry scholars, technology enthusiasts and industrial units, HyperAI has planned the "Meet AI4S" video column, inviting researchers or related units who are deeply engaged in the field of AI for Science to share their research results and methods in the form of videos, and jointly discuss the opportunities and challenges faced by AI for Science in the process of scientific research progress and promotion and implementation, so as to promote the popularization and dissemination of AI for Science.
We welcome efficient research groups and research institutions to participate in our live broadcast activities! Scan the QR code to add "Neural Star" WeChat for details↓
