最强铁基超导磁体诞生!科学家基于机器学习设计新研究体系,磁场强度超过先前记录 2.7 倍
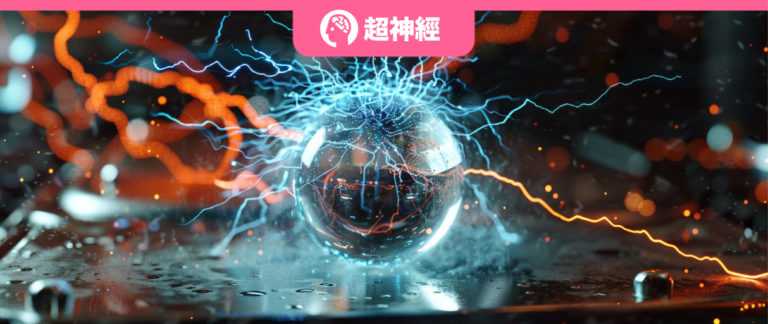
超导现象,自 1911 年被发现以来,始终保持着前沿性与高价值,吸引了大批学者投身其研究中。超导现象是指某些材料在低于特定温度时电阻突然降为零,这不仅是材料学的革命性突破,也为电力传输、磁悬浮交通和医疗成像等领域带来了应用创新的巨大动力。然而,传统的超导材料往往需要在极低的温度下才能实现超导状态,这限制了它们的实际应用。直到铁基高温超导体 (IBSs) 的出现,这一局面才得到了根本性的改变。
IBSs 能够在相对较高的温度下实现超导,超导临界温度 (Tc) 可达约 60K,比传统超导材料的转变温度高出许多。这一特性不仅降低了超导应用的制冷成本,也为超导材料的广泛使用铺平了道路。此外,IBSs 的高上临界场 (Hc2) 特性,使其在高磁场环境下也能保持超导状态,这为粒子加速器、医学成像等技术的发展提供了新的可能。
近日,来自英国和日本的科学家 Akiyasu Yamamoto 等人,利用机器学习技术,设计了一种将 researcher-driven 与 data-driven 方法相结合的研究体系,成功制造出世界上已知最强的铁基超导磁体。最新研究有望促进新一代磁共振成像 (MRI) 技术和未来电气化运输技术的发展。
相关论文以「Superstrength permanent magnets with iron-based superconductors by data- and researcher-driven process design」为题,已发表于 Nature 子刊 NPG Asia Materials 上。
研究亮点:
* 该研究成功研制出一种实用的铁基超导永磁体,磁场强度明显超过了先前的记录 2.7 倍
* 通过将研究人员的专业知识与机器学习的能力相结合,设计了一套成功的研究流程
* 数值模拟结果与实验结果吻合良好,表明材料内部存在均匀的超电流分布 Jc
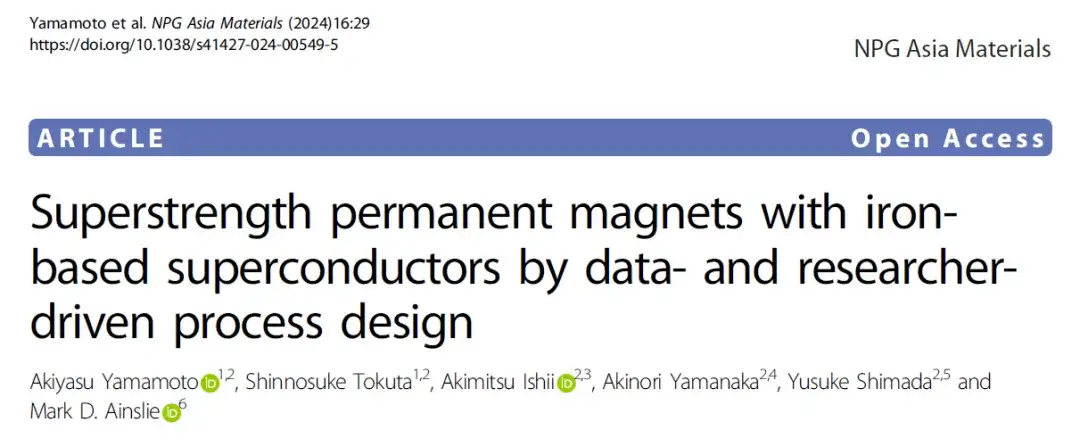
论文地址:
https://doi.org/10.1038/s41427-024-00549-5
开源项目「awesome-ai4s」汇集了百余篇 AI4S 论文解读,还提供海量数据集与工具:
https://github.com/hyperai/awesome-ai4s
全新研究体系:researcher-driven 与 data-driven 相结合
该研究使用 BOXVIA 机器学习系统,将 researcher-driven 与 data-driven 方法相结合,设计了全新的研究体系。
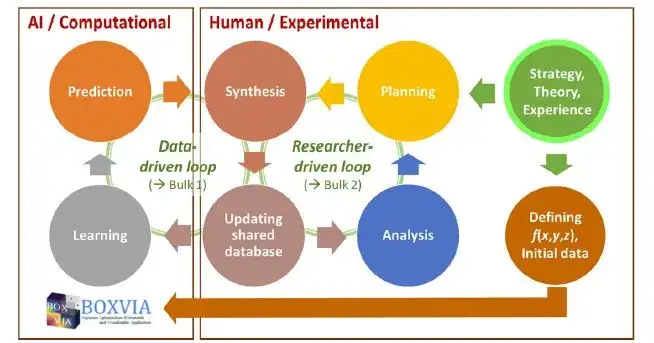
首先,研究人员基于过往研究经验,提供了各类工艺参数和初始数据,并将这些数据输入到机器学习算法中,用来预测产生性能优越的合成条件。
随后,研究人员可以根据提出的条件合成样品,并更新数据库。在机器学习算法中,研究人员为机器学习和设计过程提供了一个总体框架,从数据驱动循环 (data-driven loop) 中获得的数据,在下一步工艺中合成样品。这种「数据驱动循环」被重复使用,有助于扩展机器学习数据,并提高流程设计的效率。
在第一阶段,研究人员系统地梳理了可量化的工艺参数,以确定对最终性能有重大影响的因素。 进而,研究人员选择专注于 3 个关键的工艺参数,分别表示为 x(升温和速率)、 y(最大温度)和 z(保持时间)。 这些参数控制电火花放电烧结 (spark plasma sintering) 过程,可以应用于通过高能研磨法 (high-energy milling),获得的机械合金化 (Ba0.6K0.4) Fe2As2 前驱体粉末。
通过上述工艺,该研究首先合成了两个较大的钾掺杂 Ba122 (Ba0.6K0.4Fe2As2) 永磁体原型 Bulk1 和 Bulk2,分别对应 data-driven 和 researcher-driven 驱动,每个样品直径为 30mm,厚度为 6mm 。其中,Bulk1 的参数 (x, y, z) 设置为 (+49.8°C/min, 556°C 和 32.47min),Bulk2 的参数设置为 (+50°C/min, 600°C 和 5min) 。
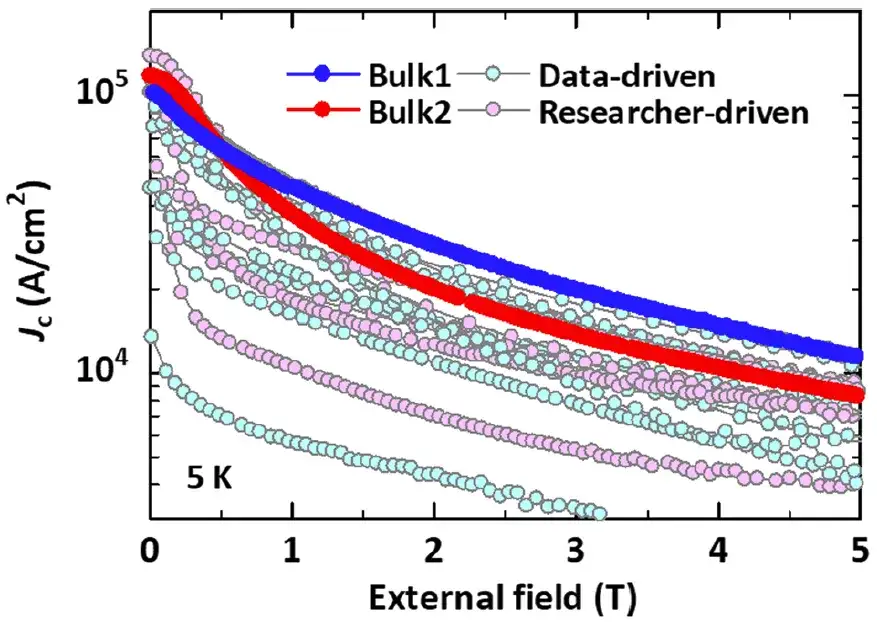
在合成样品之后,该研究进一步研究了 5K 时确定了临界电流密度 (Jc) 对磁场的依赖关系,确定了 Jc,从而识别出最佳参数。研究表明,两种方法的优化工作都导致了 Jc 的提高,但趋势存在一定差异。在 researcher-driven 的方法下,临界电流密度与磁场强度的关系表现出急剧增加的趋势,如上图红色线条所示,在 0T 时 Jc 值达到最大。而 data-driven 的方法显示出磁场强度的渐进性影响,如上图蓝色线条所示,在 3T 时实现了最高的 Jc 值。
为了优化临界电流密度,该研究为机器学习算法开发了专门用于贝叶斯优化的 BOXVIA 软件包,建立了与实验参数之间的相关性,即 Jc =f(x,y,z),其中 f 为超参数黑盒函数 (black-box function),在假设 f(x,y,z) 及其变量 x 、 y 和 z 连续的前提下,这一过程无需定义一个具体的方程来描述 f(x,y,z) 。 在贝叶斯优化算法中,利用初步数据集对函数 f 进行了建模,并使用高斯过程回归。因此,临界电流密度 Jc 被描述为高斯分布。
在局部优化方面,researcher-driven 的方法 (Bulk2) 通过以 50°C 为增量来优化最大烧结温度 x,从而得到优化后的 x=600°C 。相比之下,贝叶斯优化是以 1°C 为增量进行的,将结果精确到了 556℃。
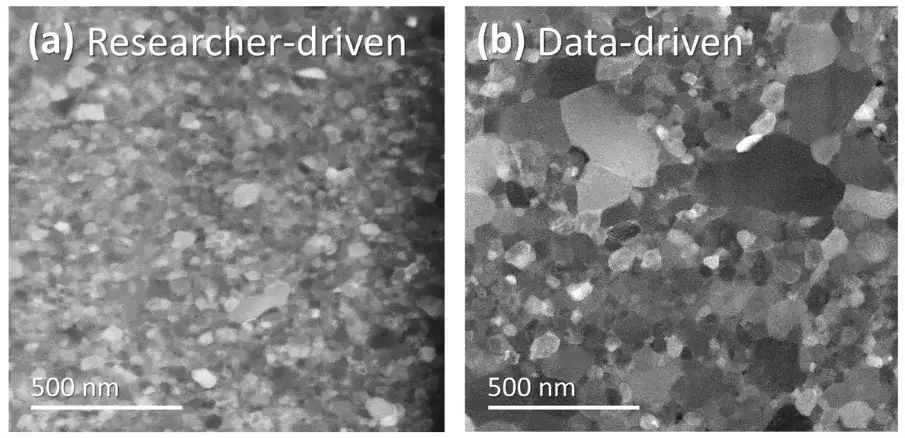
研究人员对 Bulk1 和 Bulk2 进行了纳米结构和成分分析,结果显示,Bulk2 的显微结构(上图 a)表现出由数十纳米大小的非晶相组成的致密网络结构。这种特征是由研究人员在 600°C 下采用短烧结时间获得的。相比之下, Bulk1(上图 b)通过贝叶斯优化程序制备(涉及低温下的长时间烧结),显示出分离成几个十纳米的细小颗粒的趋势。
Ba122 永磁体:磁场强度是先前报道的 2.7 倍
为了进一步分析 Ba122 永磁体对磁场和温度的依赖性,该研究在温度约为 5K 时,通过制冷机进行了快速的磁场冷却,并且外加 7T 磁场。
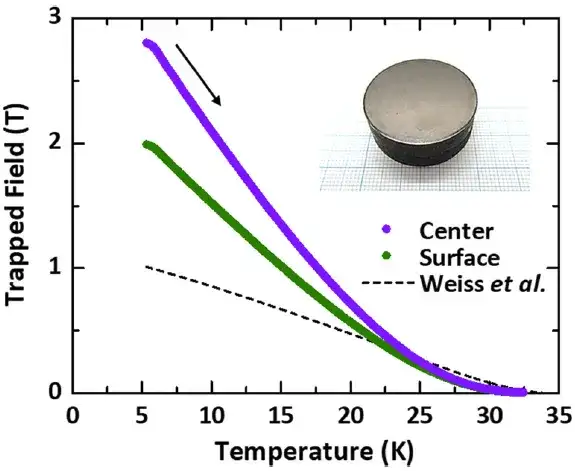
在磁场冷却过程之后,可记录的最大磁场为 2.83T,位于这一对样品的中心。这个测量值约为之前铁基超导磁体实现的最大磁场记录的 2.7 倍。
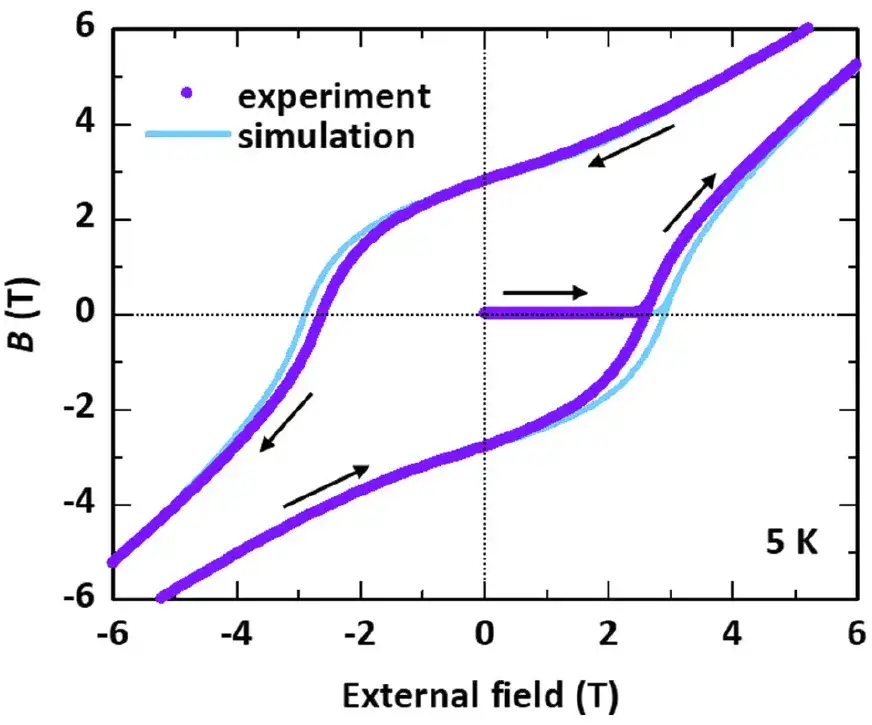
在零场冷却 (zero-field cooling) 至 5k 后,该研究通过 0T→7T→- 7T→7T 的扫描顺序进行扫描。在 7T 时,由于强磁钉和高度不可逆的磁场,磁滞回线表现出明显的增加。这与数值模型的结果非常吻合。同时,研究表明,磁化及磁流变实验结果与模型结果之间存在显著的一致性。
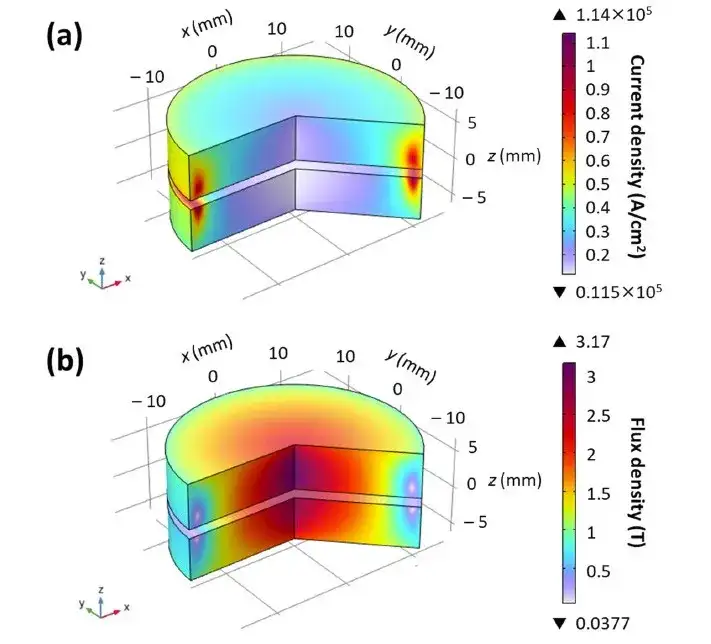
在场冷磁化 (FCM) 模型中,样品的中心区域观察到了高磁通量密度,并伴随有相关电流密度的降低。相反,在向样品边缘移动的过程中,由于临界电流密度 Jc(B) 的固有特性,这一趋势发生了反转。此外,在 Bulk1 和 Bulk2 之间观察到了轻微的不对称性。 Bulk1 中心处的电流密度大于 Bulk2,此处局部(被动)磁场最高。然而,在边缘处,Bulk2 的电流密度比 Bulk1 高,此处局部(被动)磁场最低。
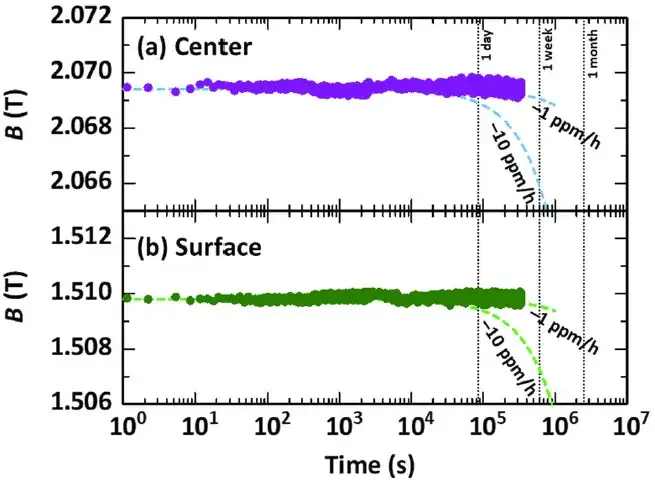
令人惊讶的是,被捕获的磁场在中心处为 2.0T 、表面处为 1.5T 的磁通量密度下,表现出近似恒定的时间行为,即使在三天后也几乎没有衰减,样品材料表现出非常高的磁场稳定性,其观察到的行为超过了 -0.1 ppm/h 的衰减率基准。这个值被认为是医疗磁共振扫描仪的关键,并且对于获得极其精确的横截面图像至关重要。
AI「炼丹」,效率大幅提升
在过去几年中,常温超导一直是全球科研领域的热门领域之一。随着市场开始从 AI 应用创新的角度审视每个行业,其对核聚变、磁悬浮、量子计算机、电力传输等技术突破的探索逐渐加深,而这些技术的大规模应用均与超导技术和超导材料大规模制备直接相关。
寻找超导材料的难度堪比大海捞针,因此有人调侃道寻找室温超导体就像「炼丹」。曾有研究人员提出,「在寻找各种各样新的超导材料过程中,确实有些像炒菜,过去只能是结合科学家的经验,把各种元素混在一起,然后在各种情况下测试其是否超导,所以效率非常低。」
近年来,AI 的成熟发展为该领域的科研探索带来全新的解题思路。 2023 年 11 月,谷歌 DeepMind 开发了全新的 AI 工具 GNoME,成功预测了 220 万种晶体结构,其中有 38 万种具有最稳定性的特性。要知道,在使用 AI 辅助材料发现之前,人类发现的稳定晶体数量也不过才 48,000 个。
具体来看,GNoME 是一种最先进的图神经网络模型,所采用的是两条工作管道来发现稳定材料。其中,「结构管道」创建具有与已知晶体结构相似的候选物,而「成分管道」则采用遵循基于化学式的更随机的方法。随后,GNoME 使用密度泛函理论计算来评估两条工作流的输出,并将这些结果添加到 GNoME 数据库中,为下一轮主动学习提供信息。基于此,GNoME 将材料稳定性预测的发现率成功从 50% 左右提高到 80% 。
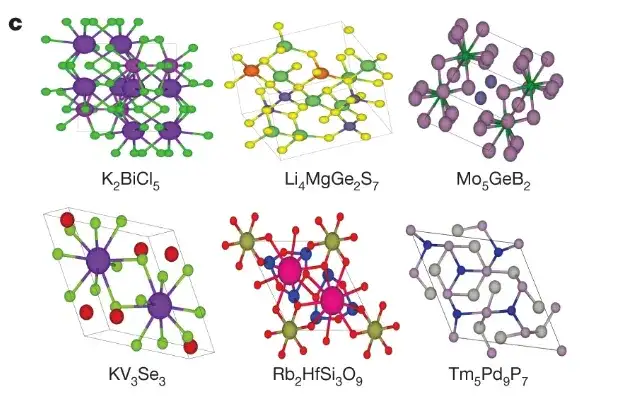
在 GNoME 预测到的新的稳定结构中,有 736 种是和其他科学家独立发现的稳定材料是一致的,其中便包括了潜在的超导体(上图中 Mo5GeB2)。这些新材料快速涌现必然推动行业创新的变革,并在超导体、电动汽车电池研发以及超级计算机供电等领域发挥作用。
同年 12 月,微软团队也顺势推出下一代生成式 AI 工具——MatterGen,大大提升了设计所需特性材料的速度,释放了 AI 在材料设计和筛选方面表现出的巨大潜力。
诚然,人类开始进行超导研究已有 112 年之久,但迄今还没有完全理解各类超导体的微观机理,尽管 AI 在探索超导体方面已经愈发成熟,但超导体的真正应用并非一朝一夕所能解决的。伴随着 AI 技术对于超导体材料的广泛挖掘,或许不久的未来我们终能得到答案。
最后推荐一个活动!
扫码即可报名参与「Meet AI Compiler」技术沙龙第 5 期线下聚会↓
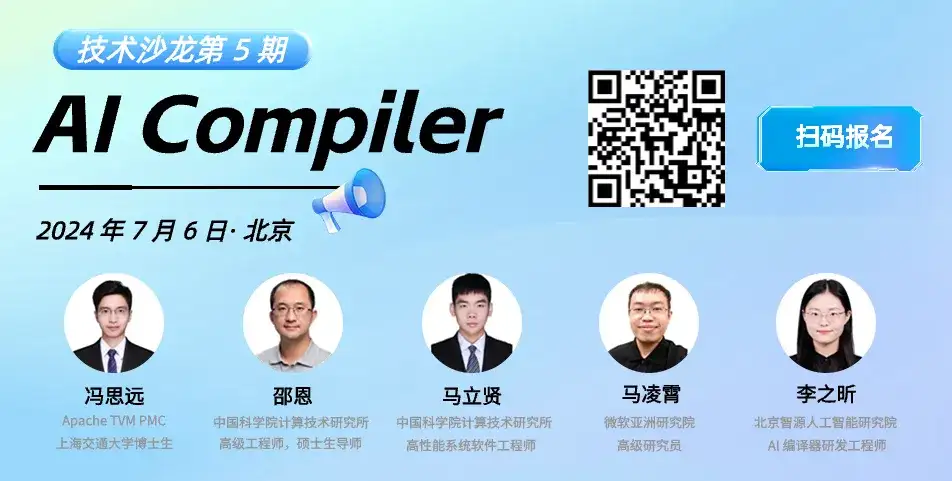