Gradient Accumulation
Gradient Accumulation is a mechanism for dividing a batch of samples used to train a neural network into several small batches of samples that are run sequentially.
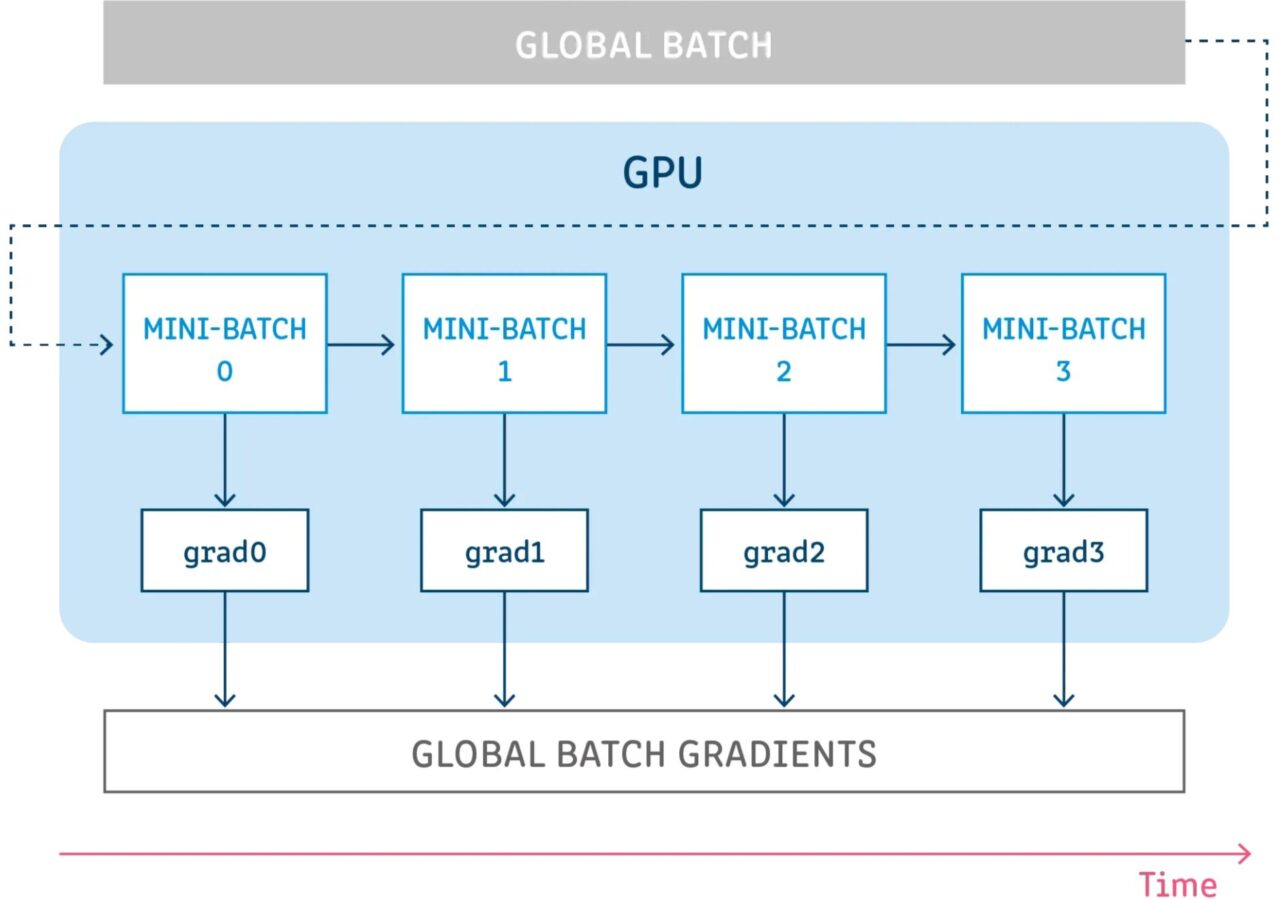
Before discussing gradient accumulation further, it is best to review the backpropagation process of a neural network.
Backpropagation of Neural Networks
Deep learning models consist of many interconnected layers where samples are propagated through forward propagation at each step. After propagating through all layers, the network generates predictions for the samples and then calculates a loss value for each sample, which specifies "how wrong the network is for this sample." The neural network then calculates the gradients of these loss values with respect to the model parameters. These gradients are used to calculate updates for individual variables.
When building a model, you choose an optimizer, which is responsible for the algorithm used to minimize the loss. The optimizer can be one of the common optimizers already implemented in the framework (SGD, Adam, etc.) or a custom optimizer that implements the algorithm you want. In addition to the gradient, the optimizer may also manage and use more parameters to calculate the update, such as the learning rate, the current step index (for adaptive learning rate), momentum, etc...
Gradient accumulation of technology
Gradient accumulation means running a configuration for a certain number of steps without updating the model variables while accumulating the gradients at those steps, and then using the accumulated gradients to compute the variable updates.
Running a number of steps without updating any model variables is a way to logically split the batch of samples into several small batches. The batch of samples used in each step is actually a small batch, and the samples from all these steps combined are actually the global batch.
By not updating the variables in all these steps, all mini-batches use the same model variables to compute gradients. This is mandatory behavior to ensure that the same gradients and updates are computed as if the global batch size was used.
Accumulating the gradients at all these steps yields the same sum of gradients.
References
【1】https://towardsdatascience.com/what-is-gradient-accumulation-in-deep-learning-ec034122cfa