ScribblePrompt Medical Image Segmentation Tool
ScribblePrompt

HyperAI Super Neural Paper Interpretation:Selected for ECCV 2024! Covering 54,000+ images, MIT proposed a general model for medical image segmentation, ScribblePrompt, which performs better than SAM"
Related dataset downloads:MedScribble multi-image segmentation biomedical task dataset
Project Introduction
ScribblePrompt
The main goal of the tool is to simplify the segmentation process of medical images, which is crucial in applications such as tumor detection and organ contouring. Instead of relying on a large amount of manually annotated data, the tool allows users to guide the model to optimize the segmentation results with a small amount of input (such as simple scribbles or points). This approach reduces the time and effort of medical experts in image annotation while ensuring the accuracy of segmentation.
ScribblePrompt
Features include:
- Efficient labeling: Through simple scribble operations, the workload of manual labeling is greatly reduced, which is particularly suitable for medical scenarios that require precise structural depiction.
- Human-machine collaborative system: Introduces real-time human-machine interaction to ensure that the segmentation results are not only automated but also consistent with expert knowledge, allowing immediate correction and optimization.
- Scalability and flexibility:
ScribblePrompt
It supports multiple medical imaging modalities, can process 2D and 3D images, and is suitable for different medical fields. - Improved accuracy with minimal input: The system optimizes segmentation results with minimal user input, ensuring high accuracy while reducing the burden on medical experts.
- Open source and scalability: As an open source project,
ScribblePrompt
Designed to be highly scalable, researchers and developers can customize the tool to meet specific needs or integrate it into a larger medical image processing pipeline. ScribblePrompt
A balance of accuracy, flexibility, and efficiency is achieved in interactive biomedical imaging tools, providing great convenience for medical experts and researchers.
Effect examples
![]() | ![]() | ![]() |
Run steps
1. 在该项目右上角点击「克隆」,随后依次点击「下一步」即可完成:基本信息> 选择算力> 审核等步骤。最后点击「继续执行」即可在个人容器内开启本项目。
2. 等待容器资源分配完成后,可直接使用平台提供的 API 地址进行操作页面的访问(需要提前完成实名认证,此步无需打开工作空间)
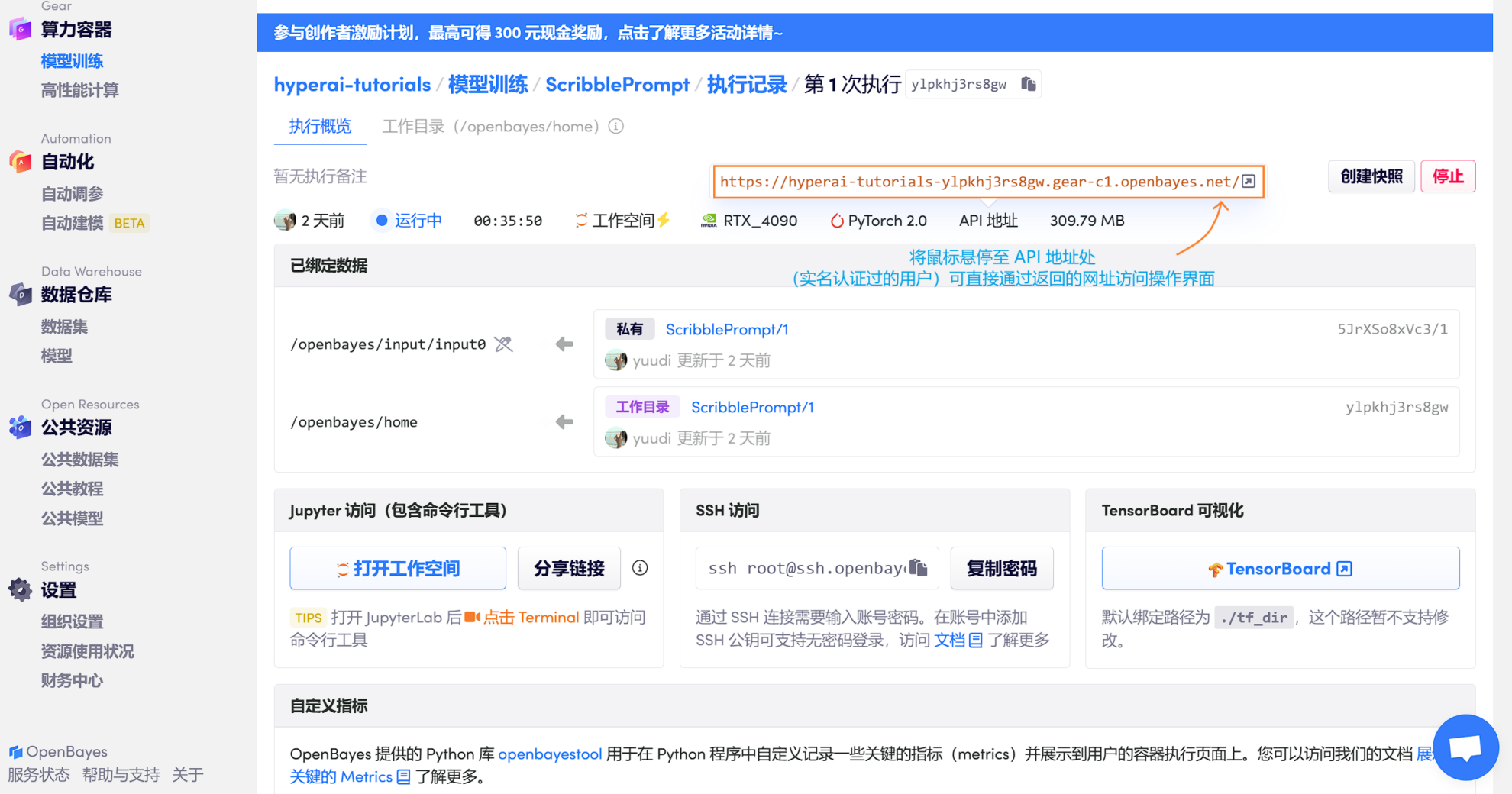
3. 上传目标图片

4. 目标内容识别与切割
第一种方式:Clicks/Boxes 。具体操作方式参考图片

第二种方式:Scribbles 。具体操作方式参考图片
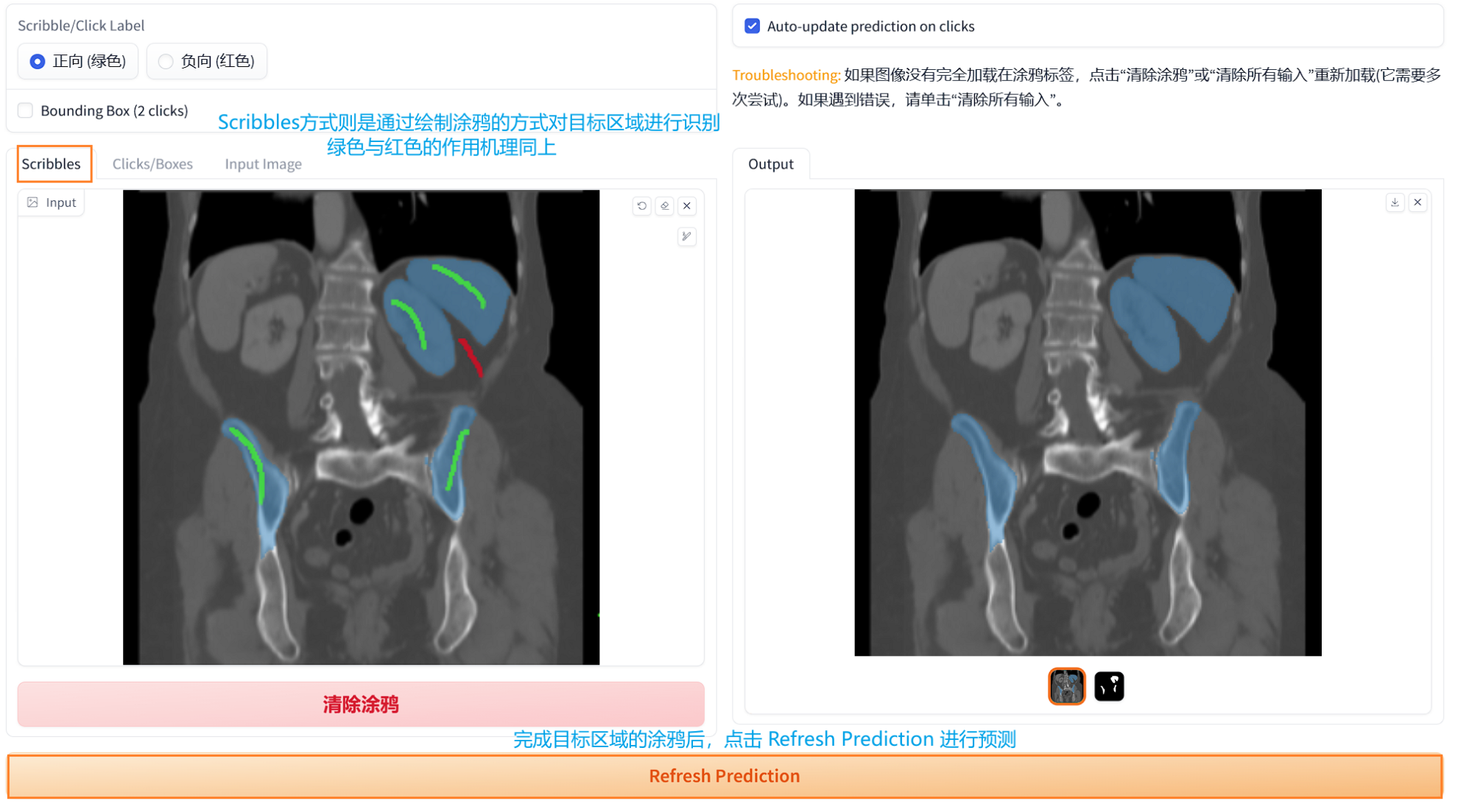
第三种方式:锚框内容检测。操作步骤如下
① Enable Bounding Box mode and uncheck the Auto-update prediction on clicks option.
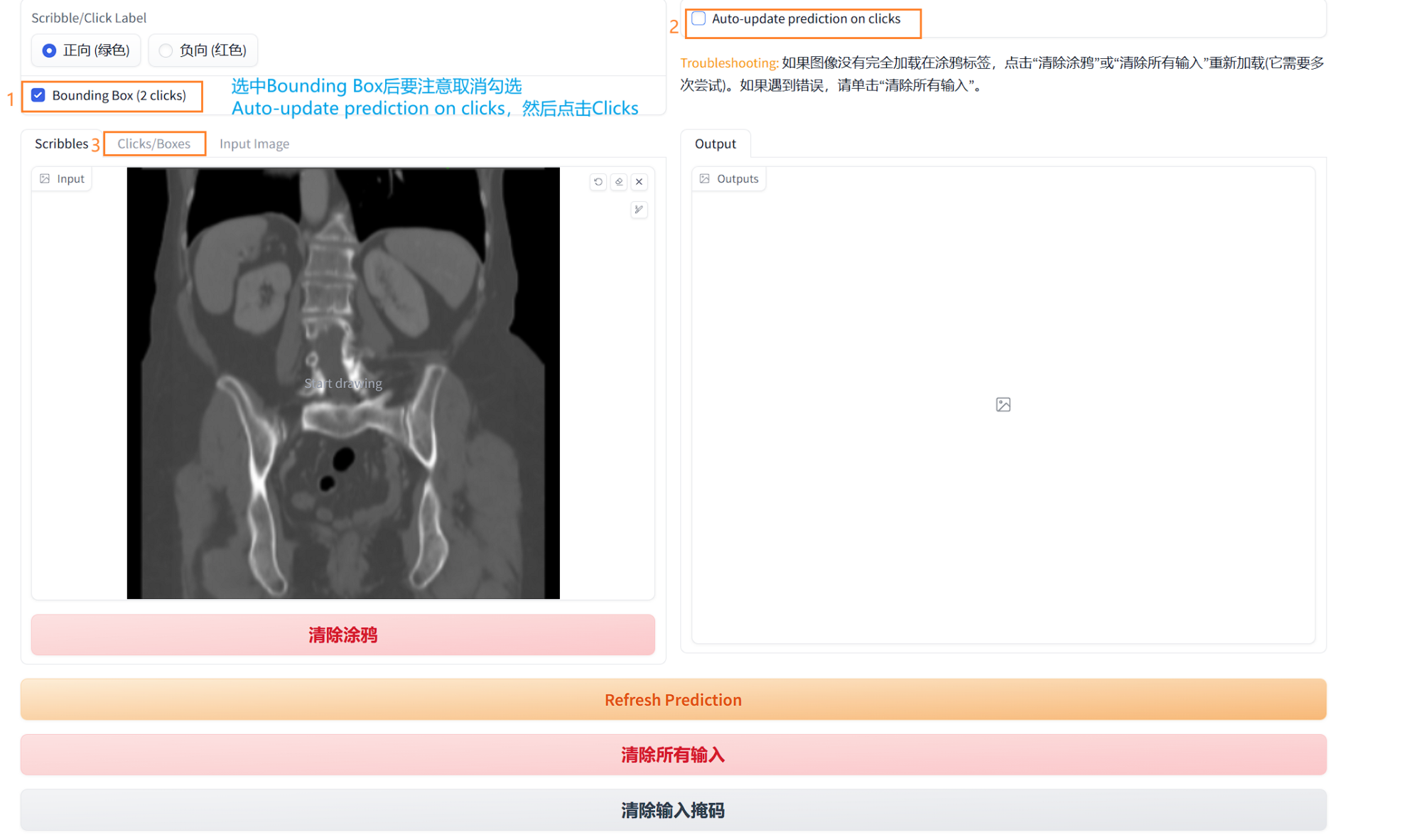
② Use anchor boxes to select target content. (Note that a single image only supports the detection of a single anchor box. If the model needs to recognize and cut multiple contents, the first two methods will achieve better results.)
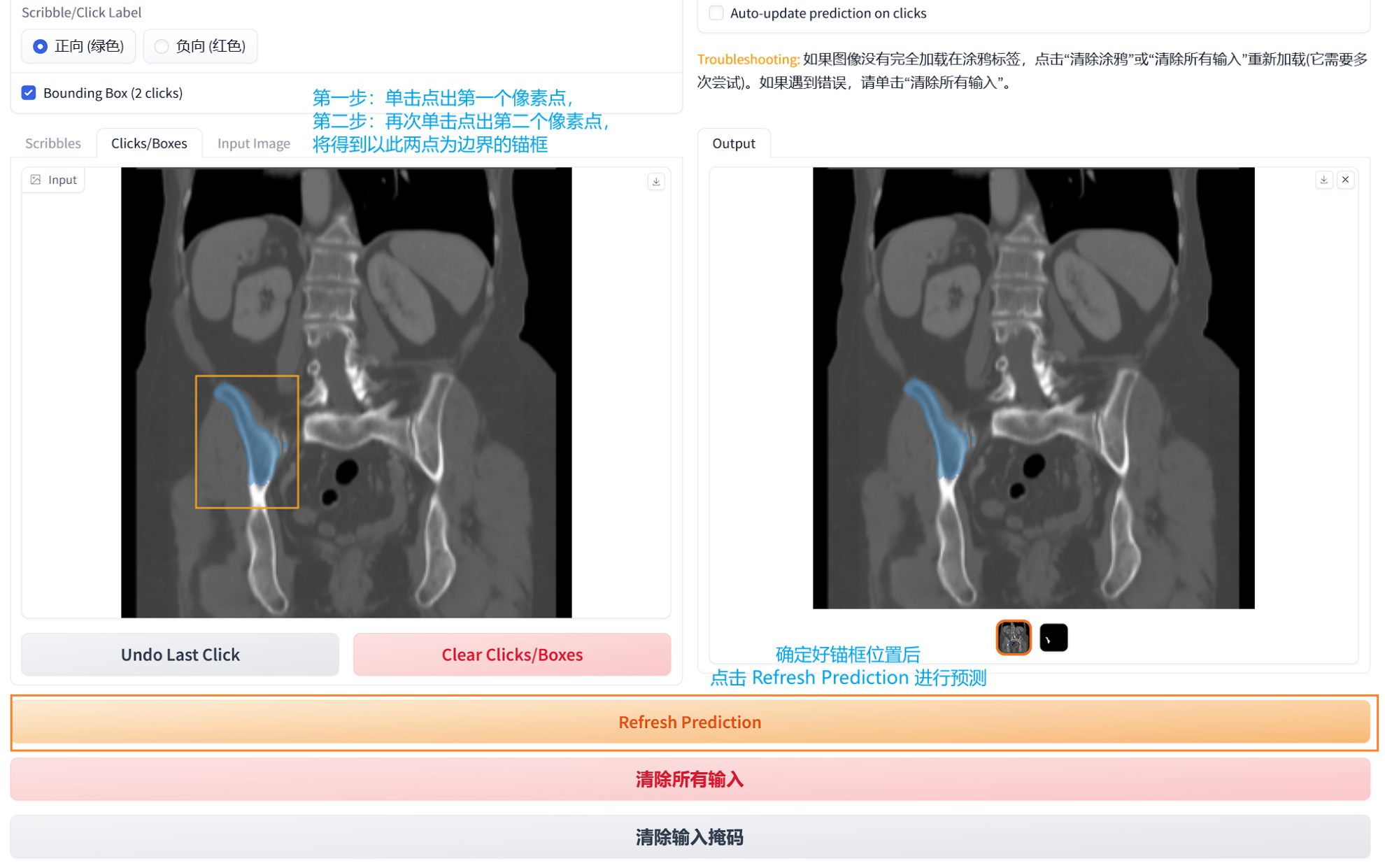