Chatting With the Co-author of the Nature Cover Article About the Research Story of the Movement
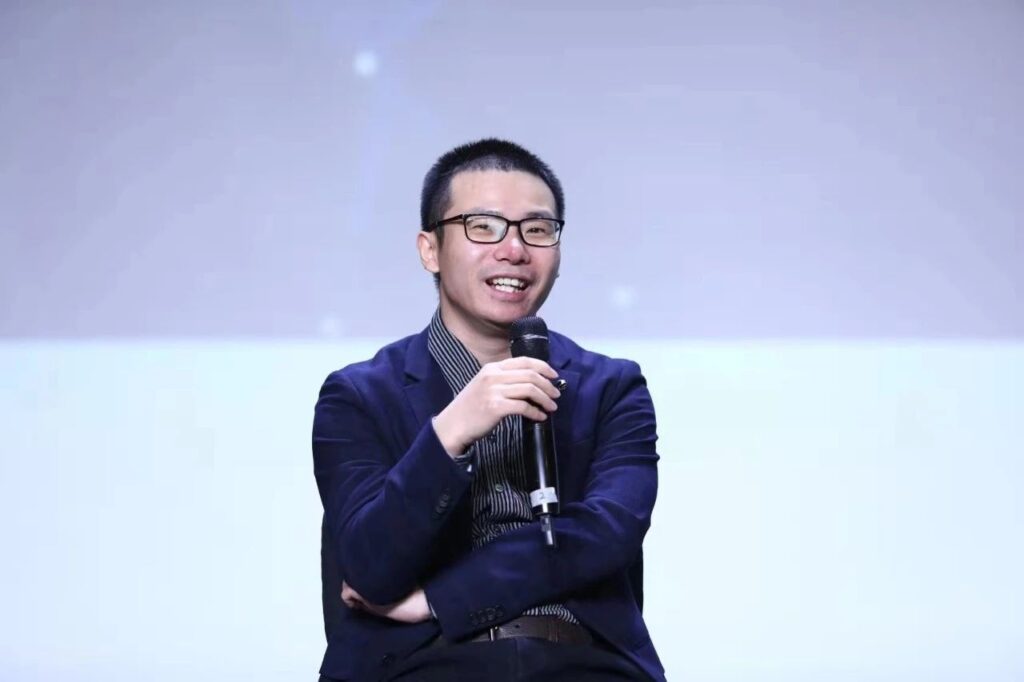
On October 17, the "From AlphaGo to Brain-like Tianji Chips, Where is Artificial Intelligence Heading?" organized by Tsinghua University Science and Technology Association Spark Forum, Tsinghua University Brain-like Computing Research Center and HyperAI was successfully held in the Meng Minwei Building of Tsinghua University. In the opening roundtable forum, Deng Lei, the first brain-like doctor at Tsinghua University, recounted his journey with brain-like computing, interpreted brain-like computing from multiple perspectives, answered questions for the students, and gave us a new understanding of the development of brain-like computing and artificial intelligence.
Deng Lei, the first doctoral student in brain-like computing at Tsinghua University and a postdoctoral fellow at the University of California, Santa Barbara. The cover of Nature magazine on August 1 showed the articleHeterogeneous Tianjic Chip Architecture for Artificial General Intelligence, as the first author, was responsible for the chip design and algorithm details.
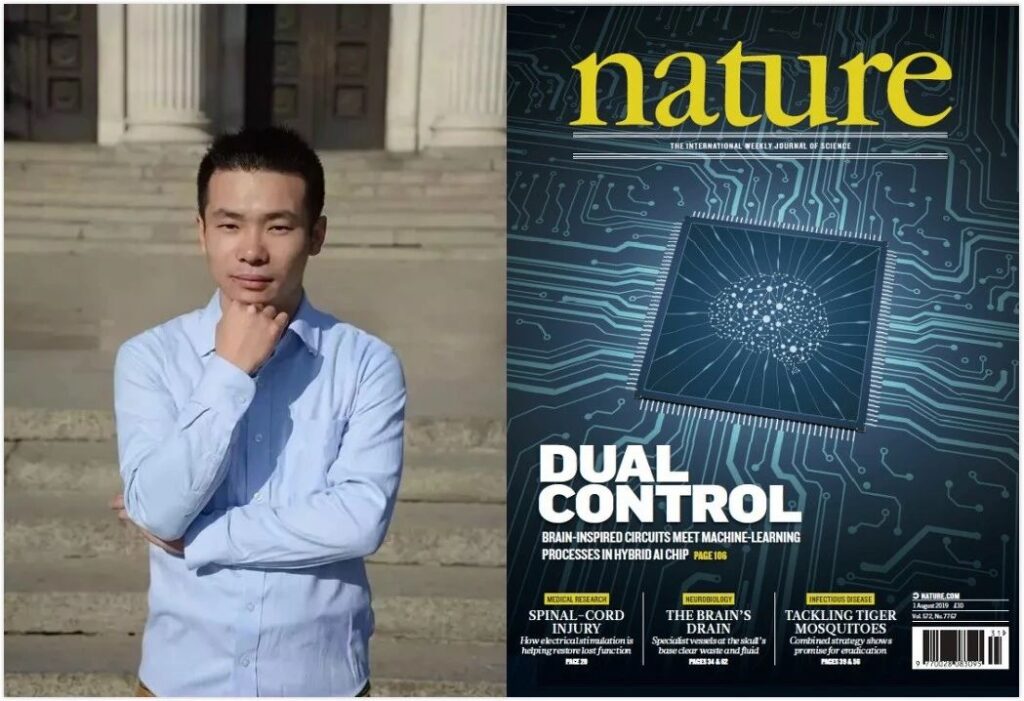
Last Thursday, Tsinghua University Science and Technology Association Spark Forum, Tsinghua University Brain-like Computing Research Center, and HyperAI held a forum on "From AlphaGo to Brain-like Tianji Chips, Where is Artificial Intelligence Heading?" Deng Lei was invited as a special guest to share some of his views in the form of a roundtable forum. This article will follow the questions in the forum and review some of his insights in the field of AI and brain-like computing.
Learning and exploring: The first PhD student at the Center for Brain-Inspired Computing
Question: How did you get into the research field of brain-inspired computing? What does this subject specifically involve?When I was studying for my doctorate in brain-like computing, it was not yet popular. I searched for it at the time, but did not find much useful information. Later, I asked my supervisor specifically...
As the first PhD student at the Brain-Inspired Computing Research Center, I have witnessed the center's development from scratch to where it is today.Including starting a company and doing research. After 2017, I graduated and went to the United States, and then switched to computer science. Now I have 50% for theory and 50% for chips.
I majored in mechanics in college, but later I found that I didn't have much talent for mechanics, so I gradually switched to making instruments. Later, I also made robots and studied some materials and microelectronics. After that, I started to work on some algorithms and theories of AI, and finally moved on to chips, and slowly entered into brain-like computing.The process is probably like this: keep moving forward and keep learning along the way.
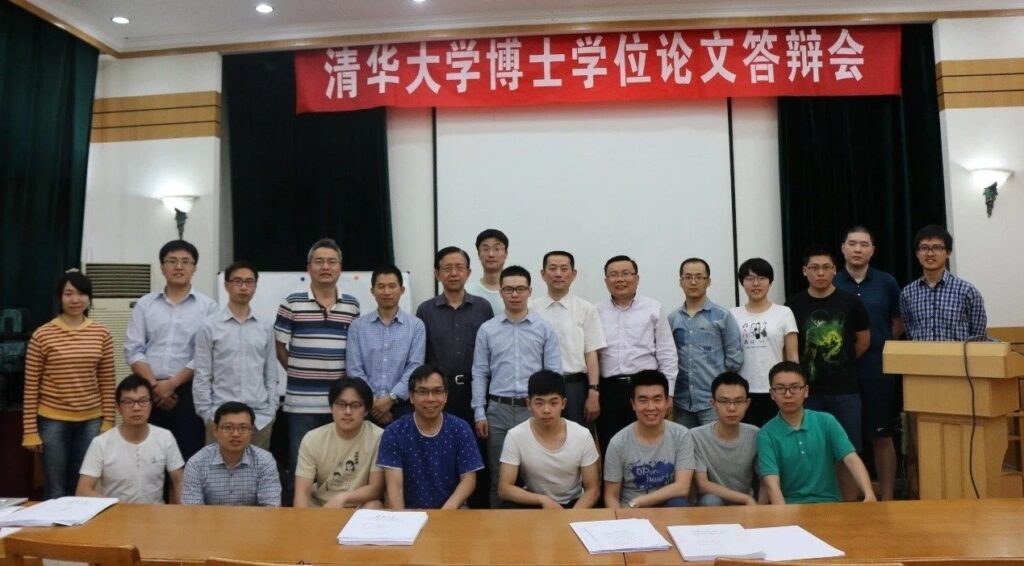
Note: Tsinghua University Brain-Inspired Computing Research Center was founded in September 2014, involving multiple aspects such as basic theory, brain-inspired chips, software, systems and applications. This center is jointly established by 7 schools and departments in Tsinghua University, integrating disciplines such as brain science, electronics, microelectronics, computers, automation, materials and precision instruments.
The research on brain-like computing involves the cross-integration of multiple disciplines.The source is definitelyMedicine (Brain Science), today’s artificial intelligence was originally derived from psychology and medicine, which provided some basis for the model.
Next isMachine LearningThey will definitely come together in the future, but they are discussed separately now because machine learning has more experience in product development and is usually thought of from an application perspective.
In additioncomputer ScienceNow there are problems that GPU cannot solve, so Alibaba and Huawei have begun to make their own dedicated chips. Students majoring in computing architecture can also consider developing in this direction.
Further down isChips and other hardwareThis involves microelectronics and even materials, because we need to provide some new devices. Now we still use some very basic storage units, but there will definitely be some new devices in the future, such as carbon nanotubes, graphene and other materials that can be applied.
In additionAutomation directionMany people who do machine learning are usually from computer science and automation departments, because automation is about control and optimization, which is similar to machine learning. In brain-like computing, these disciplines are well integrated.
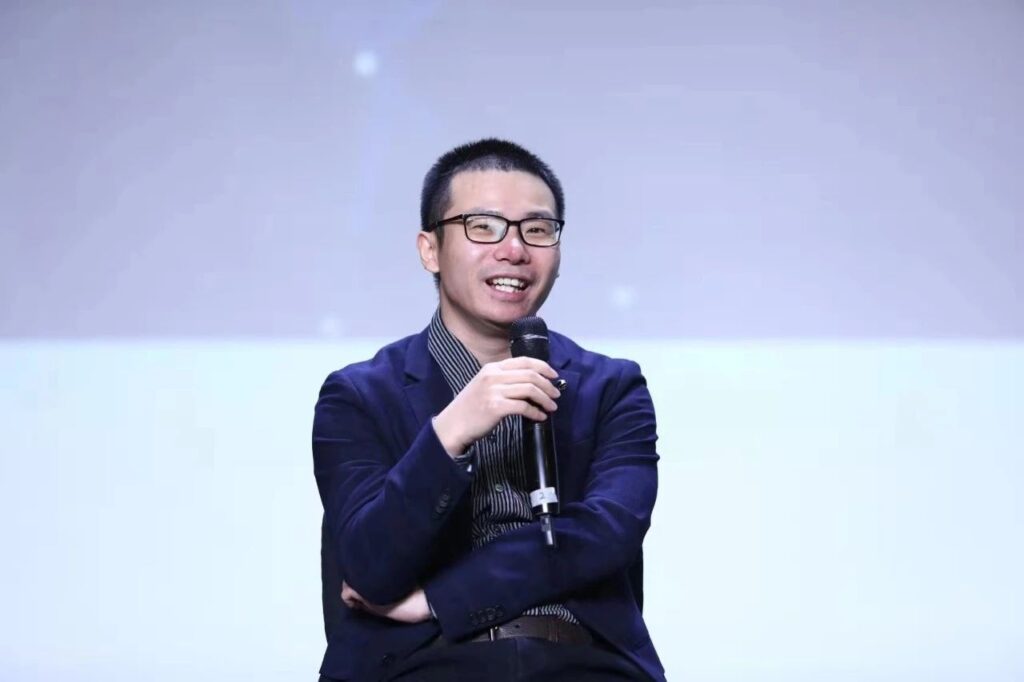
Question: What was the driving force or opportunity that led you to choose this direction?
In a word,The biggest charm of this direction is that it is never finished.
I once thought of a philosophical paradox: the study of brain-like computing is inseparable from the human brain, but we don’t know how far we can go if we use the human brain to think about the human brain, and the study of it will never end. Because human beings’ thinking about themselves will always exist, and will always experience a climax, then enter a flat period, and suddenly there will be a breakthrough, and it will never stop. Looking at it from this perspective is very worthwhile to study.
Question: What are the differences in your research during your current postdoctoral period?
When I was working on chips at Tsinghua University, I was more concerned with practical aspects, thinking that I could make a device or an instrument. But after I went to the United States, I considered this issue more from a disciplinary perspective, such as computing architecture in the computing field and many ACM Turing Awards. Although we were doing the same thing, our perspectives were different.
From the perspective of computing architecture,Any chip consists of three parts: computing unit, storage unit and communication.No matter what you do, it falls within the scope of these three things.
Tianji Chip and Brain-like Computing: Bicycles Are Not the Focus
Question: This article in Nature is a milestone event. What milestone events do you think have occurred in the past few decades? In the field of brain-like computing, what events have promoted the development of the industry?
The field of brain-like computing is relatively complex, and it will be more obvious if I sort it out from the context of artificial intelligence. Artificial intelligence is not a single discipline, and can basically be divided into four directions.
The first is the algorithm, the second is data, the third is computing power, and the last is programming tools.Milestone events can be viewed from these four perspectives.
In terms of algorithms, of course, it is deep neural networks, there is no doubt about that; from the perspective of data, ImagNet is a milestone. Without the support of big data, deep neural networks were almost buried. From the perspective of computing power, GPU is a great birth. Programming tools, such as popular applications such as Google's TensorFlow, are an important factor in promoting development.
These things have promoted the progress of AI, and they are an iterative development process. Without any of them, there would be no current situation. But AI also has its own limitations. For example, AlphaGo can only perform a single task and can't do anything else except playing chess. This is different from the brain.
The second is interpretability. We use deep neural networks for fitting, including adding reinforcement learning, but it is still unclear what happens inside them. Some people are trying to visualize this process or figure out its principles.
The third is robustness. AI is not as stable as humans. For example, in autonomous driving, AI is currently only used for assisted driving because it cannot guarantee absolute safety. Because of these shortcomings, we must pay attention to the development of brain science and introduce more brain science mechanisms.In my opinion, the most urgent thing is to make intelligence more universal.
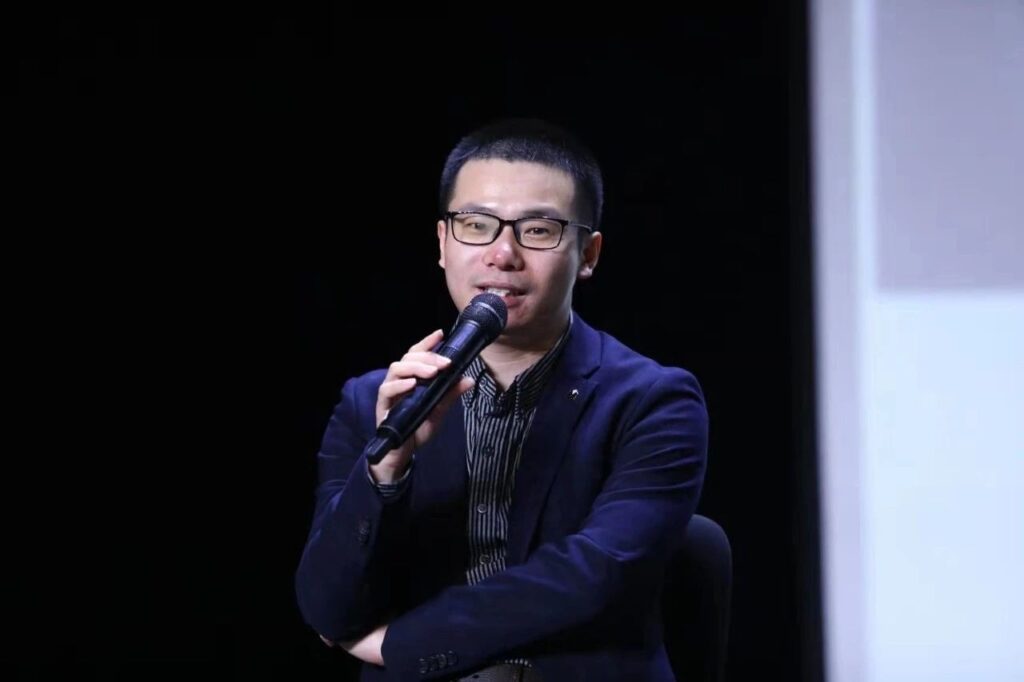
As for milestone events, AlphaGo can be considered one, because it brought AI to the public's attention, and reinforcement learning became popular only afterwards. From the perspective of chips, there are two types of chips: those that focus on algorithms and those inspired by biological brains. In the development of these two types of chips, there are two milestones respectively.
The first category is machine learning. Deep neural networks are now calculated on GPUs, but GPUs are not the most efficient. A group of companies like Cambrian are looking for solutions to replace GPUs. This is an important event.
The other category is not limited to machine learning. It is to find models from the perspective of the brain and make dedicated chips. IBM or Intel do better in this regard.
The reason why the Tianji chip has attracted so much attention is that it integrates the respective advantages of these two categories into one architecture.
Question: Your team has released the test of Tianji chip on bicycles. Can you give us more details about this?
Everyone on the Internet was attracted by bicycles, but our team knew that bicycles were not our focus. They were just a demo platform, because at that time we were thinking about finding a good platform to show everyone.

The bicycle demonstration included vision, hearing and motion control. It was an ideal platform to use a single chip to complete these functions. We considered it from this perspective at the time. Bicycle control is not difficult. We just wanted to demonstrate a new model.
The future of brain-like computing: breaking the von Neumann architecture
Question: What is the connection between future artificial intelligence or brain-like computing and the existing von Neumann architecture? Will they evolve towards the form of the human brain?
This is a very important question. There is a basic trend in the current semiconductor industry, including the 2018 Turing Award, which was also awarded to researchers who did research on computing architecture. There are two directions to try to improve the performance of GPUs. The first is to make transistors smaller, that is, physical miniaturization, following Moore's Law. But in the past two years, everyone has realized that Moore's Law has begun to fail, and related developments are getting slower and slower. One day, it will no longer be possible to make transistors smaller.

Another direction is to develop computing architecture, and try to design a framework to make the computing unit, storage unit, and communication units all work very efficiently. The human brain is amazing. Through the accumulation of learning, the knowledge of each generation is increasing. We should learn from the evolution of this knowledge.
In the last century, the development of general-purpose processors basically followed Moore's Law. Because transistors could be made smaller and smaller, the development of computing architecture was buried to a certain extent. Now that Moore's Law is blocked and applications such as AI require high processing efficiency, the research on computing architecture has regained attention. The next decade will also be the golden age of dedicated processors.
When it comes to brain-like research, the question people ask most often is: what can brain-like computing do?
This is a fatal problem. Many people working on artificial intelligence or brain science are not clear about the mechanism behind it. Take brain science for example, there are three levels that are still relatively disconnected.
The first is how nerve cells actually work. Many medical scientists and biologists are still conducting difficult explorations on this issue.
The second is how nerve cells are connected. There are 10 to the 11th power of nerve cells in the brain. How they are connected is also difficult to understand, and it requires the power of optics and physics.
Finally, we need to know how they learn, which is the most difficult but most important question.
There is a gap in every aspect, but difficulties cannot be a reason to give up exploration.If you do nothing, you have no chance. Do something at each level, and eventually something new will be born, and then continue to iterate.
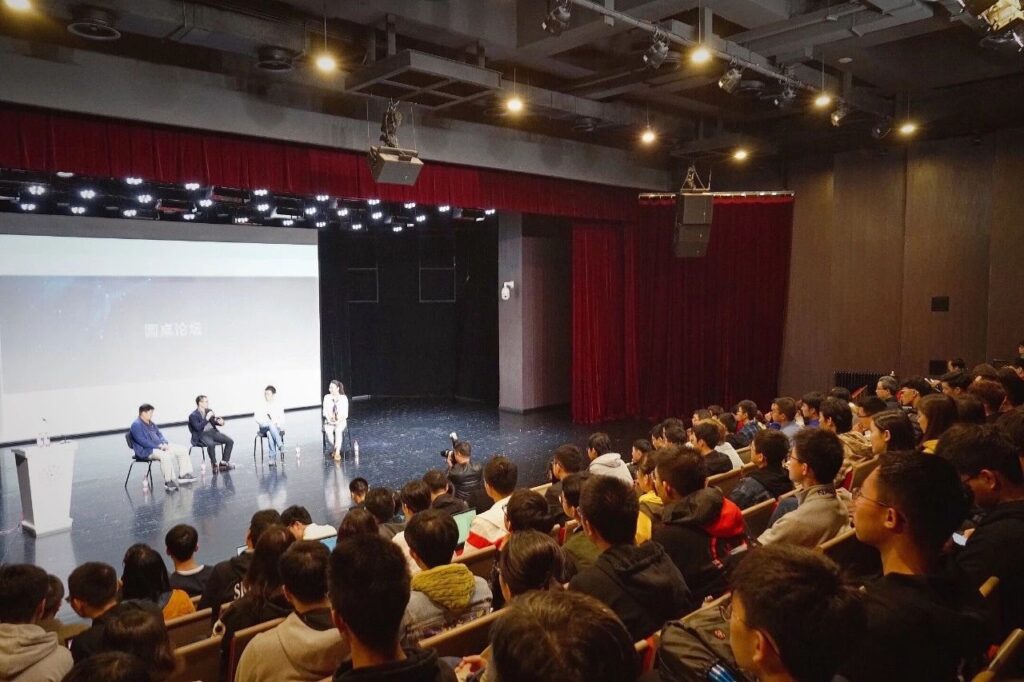
If we wait until brain science is fully understood before we proceed, it will be too late and others will surely have gotten ahead of us.
For example, making CPU is not as simple as everyone thinks. It’s not that Chinese people are not smart. The same goes for engines. Everyone understands the principles, but it is not easy to do it well. The engineering difficulty and technical accumulation cannot be achieved overnight.
One of the reasons is that many things have a large industrial chain. If you don’t do it at the beginning, you will lose many opportunities for trial and error. There will be no rapid breakthroughs in this field, and we can only proceed in a down-to-earth manner. As for the future, I think the current artificial intelligence, strong artificial intelligence, artificial intelligence 2.0 and brain-like computing will all end up in the same place, because they all originate from the brain, but they just have different directions.
Question: There was another article in Nature some time ago in which researchers drew a complete map of all the neurons in nematodes and all 7,000 connections between all neurons.
Question: Is this work related to brain-like research? Can we use existing technology or von Neumann's CPU to simulate the work of nematodes? In addition, what can we expect to happen in the next 3 to 5 years?
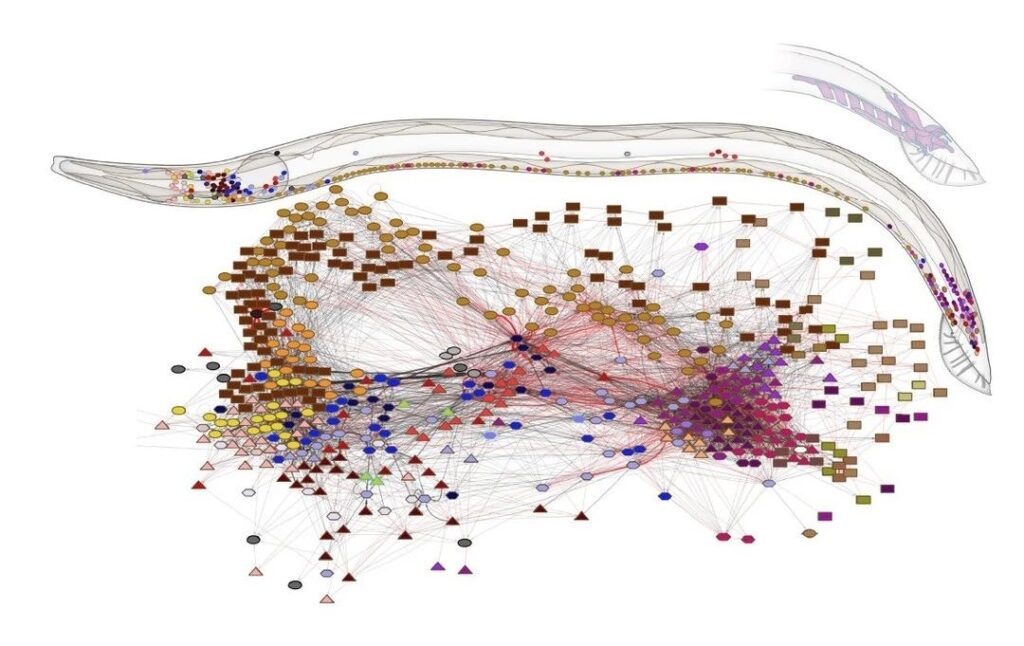
I have read the research on the structure of nematodes, which has a great impact on brain-like research. In fact, the connection structure of current AI models, whether it is brain-like computing or artificial intelligence, is mostly derived from the current hierarchical deep neural network, which is actually very superficial.
Our brain is not a simple layered network, but more like a graph. And the connections between brain regions are very complex. The significance of this study is that it allows us to think about whether we can learn from this way of connection.
There was a view before that in the structure of neural network,The role of the connection structure is actually greater than the specific weight of each connection, that is, the meaning of the connection is greater than the meaning of each parameter.
The reason why convolutional neural networks are more powerful than previous neural networks is that their connection structure is different, so their ability to extract features is stronger. This also shows that the connection structure will lead to changes in the results.
It is actually a bit difficult to achieve this result on a traditional processor. The most typical feature of the von Neumann architecture is that it requires a very clear storage unit and a very clear computing unit.

But there are no such clear boundaries in our brains. Although we have a hippocampus that is specifically responsible for long-term memory, from the perspective of neural networks, you are not clear which group of cells in the brain are responsible for storage and which are just for calculation.
The brain is more like a chaotic network, where computing and storage are difficult to distinguish. So from this perspective, it is difficult to use traditional chip or processor technologies.
Therefore, we must develop some new non-von Neumann methods and use new architectural support to conduct brain-like research.
For example, the 2018 Turing Award announced that specialized chips will become more and more popular. Nvidia is now promoting heterogeneous architecture, with a variety of small chip IP cores in one platform, which may be similar to the human brain.
Therefore, it is no longer like before that one CPU can solve all problems, and there is no one chip that can solve all problems efficiently. In the future, there will be a gradual trend towards the development of various efficient and dedicated technologies, which is the current trend.
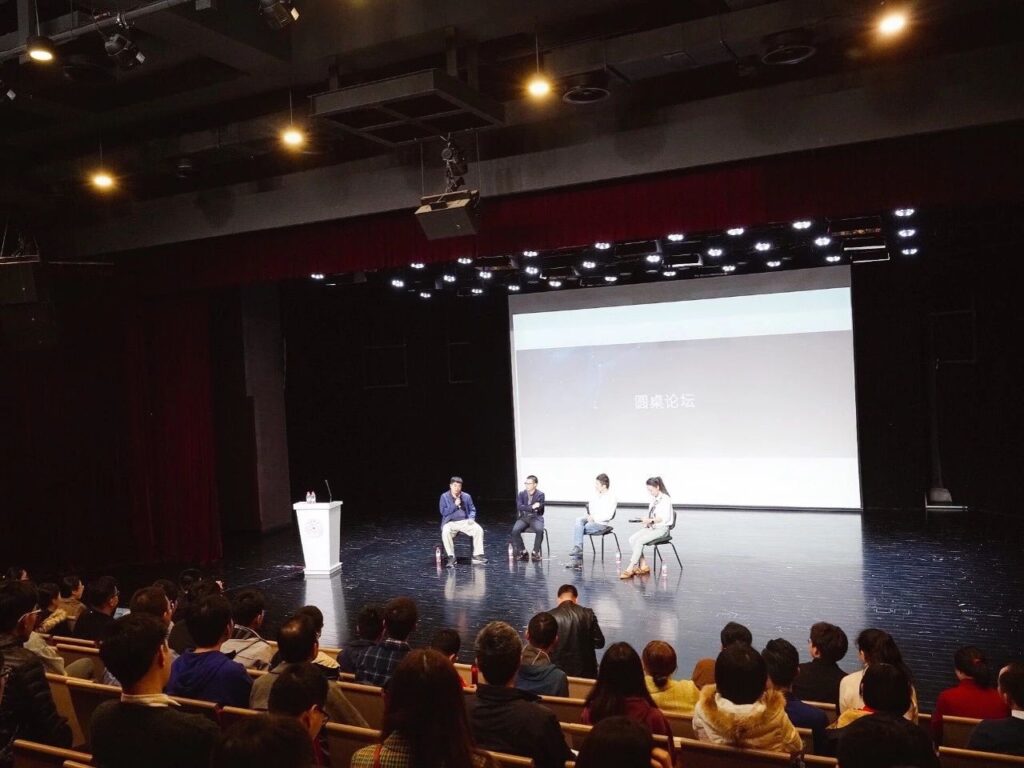
At present, people's understanding of brain science or brain-like computing is not as thorough as that of artificial intelligence. One very important reason is that investors and the industry have not yet been involved. Therefore, it is difficult to develop data computing power or tools. Brain-like computing is at such an early stage. I believe that when more and more universities and companies get involved, it will become much clearer.
Question: What are the differences between the architecture of brain-like chips and the traditional von Neumann architecture?
Brain-like chips can be divided into brain-like and computing. From the perspective of brain-like, it is not just the deep neural network in AI, but also combines some brain science computing.
From the perspective of architecture, there is a bottleneck in the von Neumann system. The architecture of the entire semiconductor industry is actually facing this problem: as the storage capacity increases, its speed becomes slower and slower. If you want to expand the scale and increase the speed, it is impossible to achieve it. Basically, people who design architectures are mostly studying how to optimize the storage hierarchy and make it faster.
Tianji is different from other architectures and does not use memory that requires expansion.The Tianji chip is more like a brain, which is equivalent to cells connected into many small circuits, which in turn expand into many networks, and finally form functional areas and systems.It is an easily scalable structure, not like a GPU.
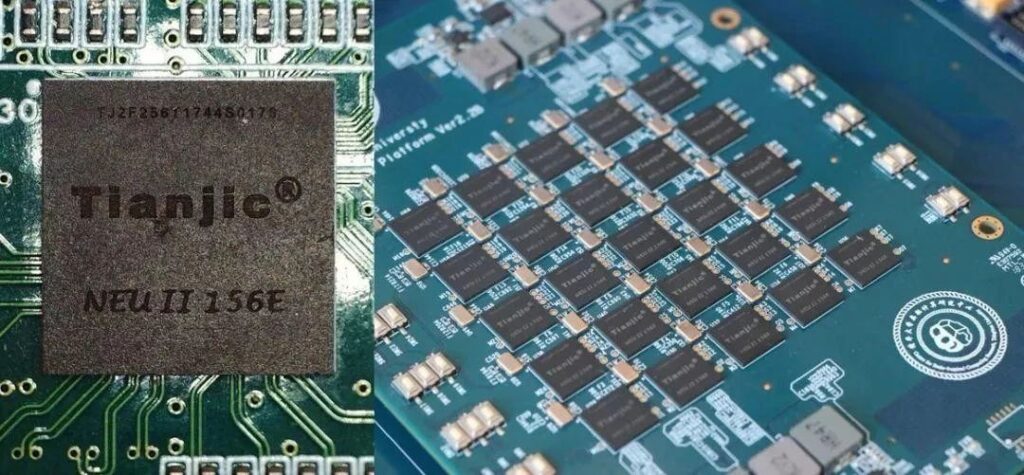
The decentralized architecture of Tianji's multi-core processor determines that it can be easily expanded into a large system without the constraints of storage walls. In fact, it is a non-von Neumann architecture that integrates storage and computing. This is the biggest difference between the architecture and existing processors. The previous one is the difference at the model level. Basically, there are two major differences.