This Decision Helped Fei-Fei Li Establish Her Status As the Queen of AI.
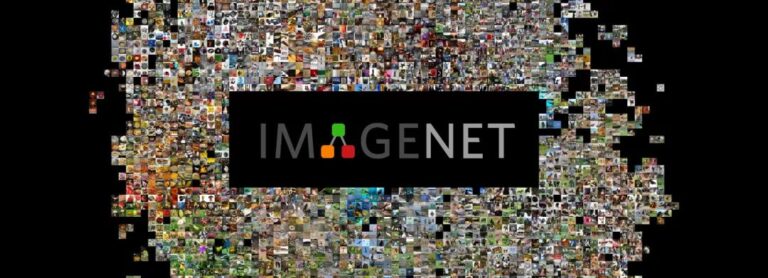
As the most classic dataset, ImageNet has led to the rapid development of computer vision. What challenges did this dataset face in its creation? How did it affect the development of deep learning? What inspiration can it bring us in today's hot machine learning world?
The famous computer scientist Fei-Fei Li has said on many occasions:Artificial intelligence will change the world, but who will change artificial intelligence?
The reason why Fei-Fei Li plays such an important role in the industry and every word she says can cause a stir in the industry is not only because of her many important research results. A very important point is that she started the ImageNet project, which played an important role in promoting the entire industry.
ImageNet: The dataset that changed the development of AI
Computer vision is the best direction for AI development at present. ImageNet is a classic data set in this field. It is no exaggeration to say that without ImageNet, facial recognition would be a luxury.
ImageNet was introduced by Fei-Fei Li et al. in a paper at CVPR 2009. The number and quality of ImageNet are unprecedented.It contains 15 million annotated images covering 22,000 categories, aiming to teach computers to recognize the diversity of the world..

In the last decade, the introductory paper on ImageNet has been published. 《ImageNet: A Large-Scale Hierarchical Image Database》The paper has a huge impact. On Google Scholar,Cited 11914 times.
Another paper describes the ImageNet data challenge and research progress in the field of object recognition. 《ImageNet Large Scale Visual Recognition Challenge》, the number of citations has also reached an astonishing 11056 times.

ImageNet has become a benchmark in the field of computer vision recognition, and it has also led the industry into an era of high-quality data sets: after 2010, major companies such as Google, Microsoft, and multiple research institutes have begun to launch high-quality data sets.
ImageNet has also stood the test of time. At the 2019 top conference CVPR,Awarded for the most far-reaching contribution to computer vision over the past decade——The Longuet-Higgins Award was awarded to the paper that released ImageNet without any suspense.
Ten years ago, she foresaw the importance of data
Back in 2009, the mainstream thinking in the industry was still about models, reflected in theoretical, hand-coded machine learning, and using mathematical methods to solve common problems.
But Fei-Fei Li did something very "different", as she later said in an interview,“Research should be long-term and have an impact. Don’t just look at the current trends. You should be committed to doing solid and influential research.”

In 2006, Fei-Fei Li was a professor of computer science at the University of Illinois at Urbana-Champaign. She found that the entire community was studying better algorithm specification strategies, but underestimated the role of data.
Through calm analysis, she saw the disadvantages of doing so:If the data used is produced for research purposes and cannot reflect the real world, even the best algorithm will be meaningless.
This made her determined to work on the data.
Ten years ago, computers still recognized objects by capturing features and then giving results. However, this method has many disadvantages. For example, for the same object in multiple postures and angles, the models abstracted by the computer often make mistakes.
The biggest problem is the singleness of the training data. If the computer is fed only one type of picture, it will be trained to have "stereotyped" cognition, and once there is a slight change, it will not be able to recognize it.
Fei-Fei Li keenly discovered that this problem would be the biggest bottleneck in computer vision.
The birth of ImagNet: a series of twists and turns
To solve this problem, Fei-Fei Li's thinking returned to people. In her understanding,A three-year-old child is able to recognize and distinguish objects because he has seen a large number of objects through his eyes and collected a large number of images.
If a large number of labeled images are “fed” to computers, AI may be able to learn to recognize images. According to this idea, the key is still data, but how to establish a comprehensive system?
At this time, a WordNet The project appeared in Fei-Fei Li's vision.
This is an English architecture built based on vocabulary classification. Each word will be displayed according to its relationship with other words. The entire project covers words for a large number of objects in the world.

After meeting WordNet researcher Professor Christiane Fellbaum in 2006, Fei-Fei Li had the answer: she wanted to imitate WordNet's approach and build a large data set to provide example pictures for each word.
The following year, while working at Princeton University, Li Feifei launched ImageNet project, and began to form a team to complete this huge work. Their goals are:Get enough annotated images to build a complete and huge image system.
But the task was so huge that they initially wanted to hire college students to find, filter and label online images and add them to the dataset.
But Fei-Fei Li soon realized that this speed of collecting images was too slow. According to a rough estimate, if a person kept marking without stopping, eating or drinking, it would take decades.
By chance, Li Feifei found another opportunity.Amazon Mechanical Turk is an online crowdsourcing method.On this platform, employers can hire many people online to complete some simple marking.

Ultimately, by using Amazon's crowdsourcing service,49,000 people from 167 countries spent two and a half yearstime to complete this huge project.
Despite facing multiple challenges such as lack of support, insufficient funding, and shortage of manpower, ImageNet was still born with their persistence.
As a new thing, ImageNet was not taken seriously at first. At the 2009 CVPR conference, the ImageNet paper was only used as a research poster and posted in an inconspicuous place.
This situation has been completely reversed with the ImageNet-derived challenge competition.
ILSVRC Competition: Let ImageNet Become a Success
One year after the release of ImageNet, thanks to the efforts of Fei-Fei Li and others, ImageNet Large Scale Visual Recognition Challenge (ILSVRC) is launched.
ILSVRC is also known as the ImageNet competition, which has been held annually since 2010. In this competition, contestants use the ImageNet dataset as a benchmark to evaluate their performance in large-scale object detection and image classification.

Soon, this competition became the Olympics of algorithm selection competition. Major institutions used it as a training ground to test the pros and cons of their own algorithms. At one time, various breakthroughs and achievements emerged.
But the highlight of the ImageNet competition is that it promoted the rise of neural networks and deep learning.
In the 2012 ImageNet competition, Hinton led his team to participate. In that year, the deep learning method used by Hinton's team was far ahead of all other methods in the image recognition competition. They submitted a deep convolutional neural network structure model. Alexnet improved the performance by 10.8 %, which is 41% higher than the second place.

What does this mean? At that time, the performance improvement of 1% would be a "Major Contribution", and the neural network, a method that had been dormant for more than ten years, actually exceeded 10 percentage points, which instantly caused a huge earthquake.

Before this, deep neural networks had never been trained with data on such a scale. After AlexNet, the excellent capabilities of deep neural networks were fully demonstrated with the help of ImagNet.
Two years later, all teams participating in the ImageNet Challenge used deep learning.
Competition ends, research continues
In 2017, after eight years, the ImagNet Challenge completed its mission: the recognition error rate of computers was lower than that of humans. The mature image identification was no longer challenging, and the new journey pointed to image understanding, so the competition came to a successful conclusion.
Driven by ImageNet and the Challenge,The computer's accuracy in classifying objects increased from 71.8% to 97.3%., far exceeding the human level.
Looking back at the process of establishing ImageNet, it was not a mainstream task at the time, but this "counter-trend" work, due to the persistence of Fei-Fei Li and others, eventually promoted the historical process of AI. At the same time, Fei-Fei Li also left the strongest mark in the field of computer vision because of ImageNet.

If data is likened to the "rocket fuel" of machine learning, ImageNet is undoubtedly the first and most substantial barrel of fuel.
As Fei-Fei Li's team said,“You don’t have to do the most popular thing, but you must do something that you believe in and that will have an impact.”

References:
1. The data that transformed AI research—and possibly the world
2. How we'reteaching computers to understand pictures
3. CVPR 2019 Attracts 9K Attendees; Best Papers Announced; ImageNet Honored 10 Years Later
4. There's only been one AI breakthrough
5. ImageNet Publications and Citations
6. Large ScaleVisual Recognition Challenge
-- over--