Stanford Uses Algorithm to Reduce Refugee Crime Rate That Is Causing Headaches for Europe
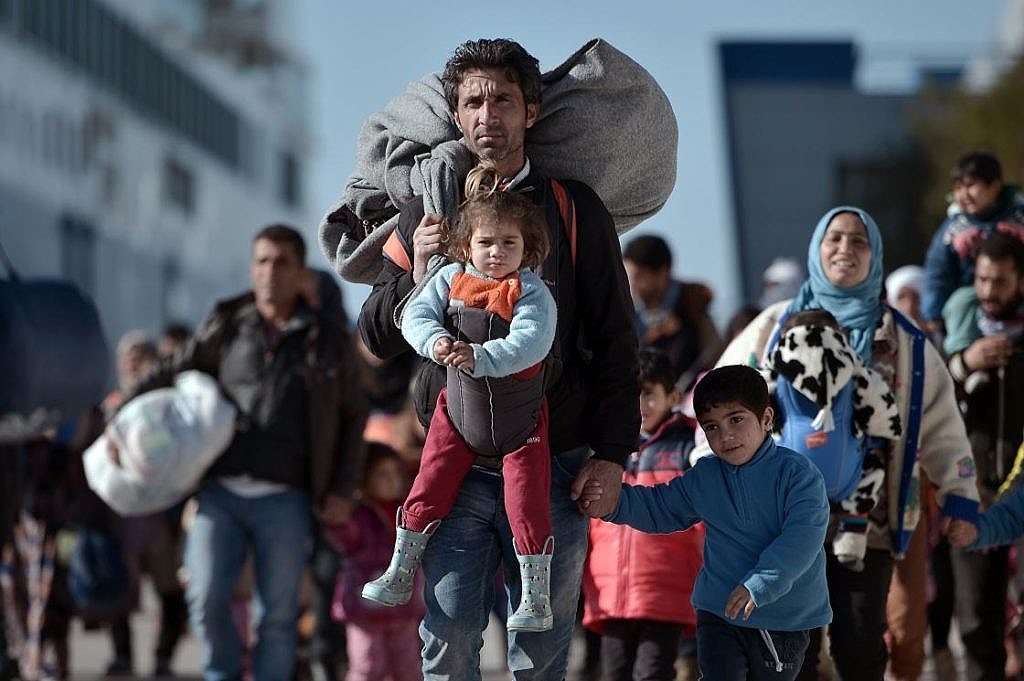
June 20th is World Refugee Day. As of 2018, the number of refugees worldwide has reached nearly 25.4 million, and how to better resettle them has become an international problem. In January 2018, researchers from Stanford University and ETH Zurich published a paper proposing the use of algorithms to help countries resettle refugees more effectively and greatly improve their employment success rate.
Over the past 60 years, the world has accumulated 750 million peopleForced to leave their homes due to war, disasters and other reasons, they become refugees. In order to draw international and social attention to refugees and raise awareness of their protection, the United Nations High Commissioner for Refugees will June 20 is designated as World Refugee Day.

The day before yesterday, the film "Capernaum" directed by Lebanese director Nadine Labaki will take place inRefugee life in Lebanon's slumsPresented to the public.
In order to escape the war, the protagonist, little boy Zain, and his family live illegally in a crowded Lebanese slum. Because of their refugee status, they can only struggle in the most unbearable corners.

Compared with modern urban life, the life of refugees in movies is shocking and sympathetic, but for war-torn countries such as Syria and Afghanistan, various heart-wrenching stories are played out every day.
Refugees: Synonymous with poverty, instability and crime
Refugees refer to people who are forced to move from their original place of residence and give up their original nationality due to war, natural disasters, etc.
Nearly 1.3 million refugees worldwide have fled their homes due to conflict or persecution 25.4 million(According to the refugee definition, internally displaced persons are not considered refugees), and more than half of these refugees are under the age of 18.

Out of compassion and humanitarian reasons, many developed countries welcome and accept refugees. Germany, Australia, Russia and other countries are major refugee host countries.

However, due to cultural differences, policy conditions, education and employment, social resources and other reasons, the influx of refugees has created some disharmonious factors.
Vicious crimes caused by refugees, deteriorating local finances, and terrorism are becoming more and more serious.All countries are beginning to be unable to bear the consequences.
Nowadays, in many places, refugees have become synonymous with "restlessness".
For some countries, it only took a few short years for them to go from initially vowing to "save refugees" to large numbers of citizens taking to the streets to protest, and even refusing to accept and expelling refugees.

According to a 2018 UN report, if the current rate of refugee resettlement continues, 18 yearsIt will take a long time to resettle all the current refugees, not to mention the increase in refugees in the future.
A great way to maintain stability: using algorithms to help refugees find jobs
Facing complex refugee groups, the most important solution is to provide betterEfficient and reasonable transportation and placement, so that they can adapt to life in their new home as quickly as possible.
In the face of this international issue, the advantages of technology emerge.
Stanford University, ETH ZurichThe research team found that the algorithm can increase the employment probability of refugees and enhance their adaptability to unfamiliar societies, thereby helping countries find more suitable areas to resettle refugees.

The researchers developed a flexible, data-driven algorithm that:It can analyze the resource status of resettlement sites and flexibly transport and allocate refugees.Thereby improving the integration effect.
This algorithm combinesSupervised learning and optimal matching,to discover and exploit synergies between refugee characteristics and resettlement sites.
Test results show that the algorithm has improved the employment rate of refugees.Increased by 40% – 70%This approach could provide governments with a practical and cost-effective policy tool that can be immediately implemented within existing institutional structures.
1. Algorithmic resettlement of refugees, three steps
Host countries have previously studied and proposed different refugee allocation schemes, such as determining optimal allocation based on matching efficiency or refugee and hosting location preferences. However, although these methods are attractive in theory, there are many obstacles to their implementation in practice.
In contrast, with a purely data-driven approach, the ensemble results can be optimized by using existing data. The algorithm is divided into three stages:Modeling, mapping and matching.
– Step 1: Modeling with historical immigration data
Modeling phase:During supervised learning, the expected success of any quantifiable indicator can be predicted, for example, whether a new, early-stage employed refugee would be a good fit for a potential resettlement location.
The researchers assigned historical immigration data to the model training, using a single refugee as the observation unit, focusing onCountry of origin, language skills, gender, age, education level, and time of arrival, place of assignment, and employment outcomesThis type of data.

These training data were then used to build a series of supervised learning models to predict refugees’ employment success expectations in relation to their background characteristics.
Refugees at each location are fitted with a separate model, resulting in different models for each location and uncovering refugee-location synergies. These fitted models are then applied to new samples to predict the expected employment success of newly arrived refugees at each new resettlement location.
– Step 2: Map individual placements to case level
Mapping phase:Convert refugee-level predictions from the modeling phase to case-level metrics. Why map to case-level metrics? Because refugees are typically not assigned to locations based on individuals, but rather on the case level, which are typically family units.
The team’s preferred case-level metric is the probability of predicting that at least one refugee in the case has found a job in that location. This metric uses the simplifying assumption that the employment probabilities of refugees in a case are independent.
– Step 3: Match each case to a specific location
Matching Phase: Assign each case to a specific location, subject to constraints, and the selected optimality criterion, and its algorithm can adapt to multiple criteria.
In the application, the optimality criterion used by the team was to maximize the mean of the case-level metric (i.e., the global mean of the probability that at least one refugee in each household is employed).
2. Improving refugee employment can stabilize the community
The team evaluated the algorithm’s application inUnited States and SwitzerlandPerformance: The United States, which allocates refugees mainly based on capacity constraints; Switzerland, which randomly allocates refugees based on a proportional allocation indicator.
In the United States, reception and resettlement services (e.g., arranging location assignments, housing for refugees, etc.) are provided by nine voluntary agencies in cooperation with the Department of State.

After refugees are assigned to one of the agencies, resettlement officers centrally distribute refugees to resettlement sites within that agency, subject to local capacity constraints.
Resettlement officers make assignment decisions before refugees arrive, without interviewing them. Refugees are given work authorization upon arrival and are encouraged to find work as soon as possible.

To track refugee resettlement success, agencies are required to report refugees’ employment status 90 days after their arrival, following the completion of the reception and resettlement period.
To evaluate whether optimizing the placement algorithm could improve outcomes, the team analyzed data on working-age refugees (18 to 64 years old) resettled by one of the largest placement agencies between 2011 and 2016. The team split the data into training and test sets. They then applied the fitted model to predict expected employment success at each location and determined the optimal allocation for the test set of refugees who arrived in the third quarter of 2016.
For the test data, the researchers focused on refugees who were freely assigned to different resettlement sites, rather than those assigned based on where their families or other ties were. They also imposed constraints on the assignments, so that under the optimal assignment, each site could only acceptLess than or equal toThe actual number of people received.
In addition, the algorithm distribution is greatlyAdded expected refugee employment rates instead of status quo allocations.

The final results show that the algorithmic allocation improves employment rates in almost every location, including locations with high and low baseline employment rates. On average, the employment rate under the actual allocation is 34%, and the employment rate under the optimized allocation is 48%, which means thatThe optimized allocation would raise the employment rate to approximately 41% above the baseline.
Compared to more expensive interventions that may take a long time to implement, such as language or vocational training programs, data-driven approaches have the potential toBetter cost-effectiveness, which can be achieved at almost no additional cost to the government or immigration authorities.
In addition, the algorithm modifies the existing policy process,Improved implementation efficiency, so that refugees can integrate into the host society more quickly. And the algorithm isDynamic, can adapt to synergy over time.
We don’t have to worry about the algorithm taking over everything; it is there to supplement, not replace, the placement person. In a computer-assisted placement process, the algorithm may provide several recommendations, and the placement person can decide on the final allocation or not take any recommendations.
Refugee issue: More than just compassion is needed
The refugee issue is not something that can be changed by so-called overflowing sympathy, nor can it be solved by technology alone. Social factors such as local peace, policies and systems are the main factors in improving this situation.
However, the application of technology will become a more effective tool for the government and immigration authorities, and provide more effective and appropriate refugee resettlement recommendations.
It is hoped that with the efforts and technical support of various countries, both refugees and host countries will be able to get out of the crisis as soon as possible.