618 Shopping Spree | Uncovering the Secrets of Amazon and Taobao: How to Build Algorithms to Become the Best Shopping Guide
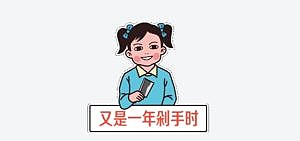
Following the "Double Eleven" event, JD.com also took advantage of its store anniversary to create the "618" mid-year shopping carnival. In addition to using various marketing methods to attract customers, major e-commerce companies are also using intelligent recommendations to continuously influence users' shopping choices. The recommendation system has made a great contribution to the growth of transaction volume.
In 2009, Taobao launched the “Double Eleven” event, turning the original Singles’ Day intoShopping carnival.Since then, the historical curtain of major e-commerce companies creating festivals has gradually opened.
In recent years, various online shopping festivals have been held almost throughout the year.
Shopping Festival: Consumers’ Carnival, E-commerce Wars
Starting from New Year's Day, a series of shopping festivals follow one after another.
From the "New Year's Goods Festival" during the Spring Festival, to the "Goddess Festival" on March 8, to the "Mother and Baby Festival" in late May, to the "618" carnival in the middle of the year, and then to the "Double Eleven" and "Double Twelve" in the second half of the year... the list is endless. Major e-commerce companies have taken turns to change their ways to give the majority of online shoppers a chance to shop.Buy Buy Buyreason.
Recently, seeing that JD.com's "618" is about to come, major e-commerce companies and online shoppers are gearing up.
Since the end of May, major e-commerce companies have been warming up for this mid-year carnival, with advertisements everywhere and various marketing strategies: 50% off in the first hour, as low as 6.18 yuan, discounts for various amounts... and shopaholics have also started to make careful selections early, filling their shopping carts.
For employees of major e-commerce companies, this shopping festival seems to beA war without gunpowderGenerally, "the battle line is too long, so we just have to wait until June 18th to start."
For the protagonists of this "war" - the majority of online shoppers, as the level of consumer spending increases, price is no longer the only guide for purchasing, so major e-commerce companies are no longer simply competing on price. Brand, quality, reviews and other factors will become reference factors. "Guess you like" and "Good stuff"Things like these are constantly influencing the choices of online shoppers and constantly breaking their self-control.
Therefore, a smarterRecommendation SystemIt has also become an indispensable weapon for e-commerce platforms.
Personalized recommendations everywhere
Nowadays, intelligent recommendation systems are everywhere.
Toutiao stood out from many news clients by relying on algorithms to interpret readers' interest DNA and provide users with accurate news recommendations. For example, the "People who like this movie/book also like..." on the film review platform, the playlist recommendations on music software, and the "Jobs you may be interested in" on job search software are all based on intelligent recommendation systems.
Various e-commerce platforms are inseparable from recommendation systems. According to Amazon, the originator of intelligent recommendation, 40%'s revenue all comes from the personalized recommendation system.
In fact, the recommendation system first became popular in the retail industry and has been 20 years of history. It has gone through the stages of simple association recommendation to personalized recommendation.
From the initial user-based collaborative filtering, to the later content-based filtering algorithm, and finally to the hybrid recommendation algorithm,Machine Learning, Deep LearningTechnologies such asPersonalization.
Initially, the recommendation system mainly relied on product data to recommend similar products to the product being searched. "Everyone looks the same"Later, personalized recommendations based on the connections between users, using data mining, machine learning and other technologies, have been achieved. "Thousands of people, thousands of faces".
Taobao's intelligent recommendation algorithm revealed
Nowadays, smart recommendations have brought great convenience to online shoppers.Algorithms have become shopping guides who know themselves better than themselves.However, you should know that behind these products that constantly attract users to click are complex algorithms involving machine learning, big data, natural language processing, etc.
Take Taobao, which is familiar to most online shoppers, as an example. Taobao's recommendation system has also gone through several stages of development.
In 2011, Taobao started toThe first experimental project of recommendation algorithmAt that time, it was a personalized project called "Mother and Baby Files", which mainly recommended suitable products for mother and baby customers. The original intention of setting up this project was toImprove search efficiency so that users can find products that meet their needs more quickly.IncreaseEasy to measure.Before this, traditional e-commerce searches all used the same set of algorithms.
Around 2013, as the number of products on the platform increased, using the same search algorithm for all users could no longer meet user needs.Personalized recommendations and searchesIt has been officially put on the agenda to cater to users' increasingly diversified demands.
Taobao made a crucial move with JuhuasuanPersonalized recommendation test——In the past, the sorting of Juhuasuan’s product displays was based on the sales volume calculated every hour. After personalized sorting was implemented, the product transaction volume quickly doubled.
The success of the test gave Taobao more confidence in personalized recommendations. So in 2014, following the e-commerce search team, Alibaba established a dedicated recommendation technology team.
A large amount of user data also provides sufficient basis for Alibaba's recommendation system. In addition to basic information such as age and gender, the user'sShopping history, search history, browsing history,All are captured by the machine to define its preferences.
“We can observe your browsing behavior every time. For example, we can see whether the products you browse in 10 slots are similar. When the recommended categories are too concentrated, the machine will sense user (aesthetic) fatigue through some signals, and the next push will increase the exploration degree and recommend something else.”
The person in charge of Taobao's recommendation system once said, "The worst situation for product recommendations is that users see the product, but keep scrolling the screen and don't click on it."
but,It is not easy for algorithms to find logic in user behavior.As a user, this scenario may be very common: open Taobao, browse a skirt, then browse bicycles, then go back to look at skirts, and finally buy a bag of spicy noodles and leave.
To extract rules from a mess of behaviors, algorithm engineers came up with two methods:Real-time recommendations,Let complex models and algorithms quickly understand the intention of each click and make recommendations at any time according to the user's steps;Categorize illogical behaviors.That is, classified according to categories such as clothing, electronic products, etc.
In 2018, Taobao's intelligent recommendations moved towards scenario-based. For example, when a user searches for Nordic-style dining chairs, it will not only recommend dining chairs, but also a full set of Nordic-style home furnishings. It is reported that after such improvements, the usage rate of Taobao's recommendation column has increased a lot.
When we use e-commerce platforms, we also have a purpose in mind. "search", gradually became a aimless "visit"While strolling around, you often buy a bunch of recommended products without realizing it.
Limitations and Challenges of Recommender Systems
However, recommendation systems are often criticized.Inaccurate recommendations and duplicate recommendationsIt is the most common slot.
A user once complained: I just bought a quilt, but they recommend it to me every day; another user said: I am tired of the same style of skirt being recommended to me every day.
Data shows that for products such as books and food, the repurchase rate is relatively high, so the algorithm for repeated recommendations also needs to be targeted.The same algorithm no longer applies to all users and products.
Therefore, recommendation technology needs to be continuously improved in terms of accuracy and potential demand mining. One day in the future, you may be able to buy what you want most with your eyes closed.
Finally, I wish you all a satisfying and happy shopping festival.