With Neural Networks, Tom Can Track Jerry in Real Time
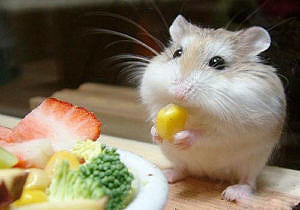
fieldBy using a large amount of animal video data to train the neural network, better animal and background segmentation can be achieved in the observation of animal behavior in complex and dynamic environments, thereby better tracking animals.
Why do birds sometimes peck their eggs? What does a squirrel wag its tail to express? Is a cat hunching its back because it is afraid or angry? Are there secrets of this group of animals hidden behind their various behaviors?

In the earliest times, some tribes and regions regarded animals as gods, and people hoped to obtain divine omens and blessings from animals.
It was not until the 20th century that scientific research on animal behavior began, and Darwin was one of the first scientists to do so.
But early behavioral research could only rely on visual observation and simple recorders.
Later, with the application of advanced technologies such as video observation and radio telemetry, animal behavior could be monitored and quantified in the field and in laboratories simulating natural conditions. Large amounts of data could be processed by electronic computers, making behavioral science subject to quantitative criteria.
In recent years, AI technology has also been reused in capturing and tracking animal "behavioral language".

Recently, researchers from the Jackson Laboratory in the United States used a modern convolutional neural network architecture to develop a scalable mouse tracking method in the open field, successfully tracking animal movements and behaviors in complex and dynamic environments with accuracy reaching human levels.
It is reported that the neural network they trained can use a simple example learning method to track mice in different environments, with different fur colors, body shapes and behaviors for a long time, and the process does not require continuous human supervision.
Humans and Nature: Understanding Each Other Through Ethology
The universe is vast, and the existence of the earth is lonely and precious. On this planet, except for humans who have a complete language system, other animals have never had language ability, which makes humans and other ethnic groups have essential differences and barriers.

They believe they will bring blessings to themselves
However, in a sense, animal behavior is their "language". All their behaviors have certain physiological basis. By observing these behaviors, humans can understand the physiological condition, emotional expression, learning behavior, etc. of animals, which will have a certain impact on disciplines such as psychology and education.
In addition, for the breeding industry, observing the behavioral responses of animals under various environmental conditions and understanding their activity patterns can help improve animal management levels and production capabilities.
For laboratories that study fly and mouse behavior with the goal of eliminating target animals, the benefits to society would be even greater if these studies could lead to the complete elimination of pests that spread epidemics and bacteria.
Observing animal videos is one of the main means of research in various animal laboratories, but it would be too time-consuming and labor-intensive if a large number of videos had to be labeled manually.
For the large amount of video data generated by tracking animal behavior, AI technology can replace manual tracking and marking work, and can even track more accurately than humans.

"DeepLabCut" can accurately and quickly track the behavior of small animals
The Jackson Laboratory team in the United States analyzed large amounts of animal video data and trained neural networks to automatically analyze, track, and even predict animal videos.
Killing pests: A neural network-based mouse tracker
Jackson Laboratory uses a neural network-based tracker toAutomatic tracking of mice is achieved without manually marking each frame of video or placing markers on the research subjects.
They compared the visual performance of three different neural network architectures for different mice and different environmental conditions. The first architecture was an encoder-decoder segmentation network, the second was a binned classification network, and the third was a regression network.

Experimental results show that the encoder-decoder segmentation neural network has high segmentation accuracy and speed with minimal training data. In addition, they provide a labeling interface, labeled training data, hyperparameter tuning, and pre-trained networks for the behavioral and neuroscience communities.
In the study, in order to capture the rich movement of mice in the video, the mice are usually abstracted into a simple point, center of mass or ellipse for analysis. In order to better use the existing methods to track mice and perform appropriate segmentation, the team simplified the experimental environment and obtained the best contrast between mice and background.
The neural network classifies the pixels in the video that belong to the mouse from the background, allowing these high-level abstract behaviors to be converted into data for mathematical calculations.
In order to better distinguish animals, researchers usually change the background color of the venue according to the color of the animal's fur, but this is likely to affect its behavior.
The tracker using neural network does not need to do this. It can track in complex and dynamic environmental conditions regardless of coating color.

In this way, we can't help but worry about the little mouse Jerry. If Tom masters this technology, can he still jump around happily?
Accurate tracking: requires extensive training
To test the neural network architecture, they established A training dataset of 16,234 training images and 568 holdout validation images. They also created an OpenCV-based labeling interface for creating training data (methods) that enables quick annotation of foreground and background.
Their network was built, trained, and tested in Tensorflow v1.0. The provided training benchmarks were conducted on an Nvidia P100 GPU architecture. The hyperparameters were tuned over several training iterations.
The end result is that of the three different architectures mentioned above, the encoder-decoder segmented network architecture is able to achieve the highest level of accuracy and functionality at high speed (over 6x real-time).
Additionally, they provide an annotation interface that allows users to train a new network for their specific environment by annotating as few as 2,500 images in about 3 hours.
Neural network tracking outperforms traditional methods
Compared with traditional tracking methods, the neural network tracking method trained by the team "wins" in the following two aspects:
1. No reliance on foreground-background visual contrast
Traditional tracking methods manipulate environmental conditions to increase the contrast between the animal and the background, thereby achieving correct foreground/background detection (segmentation). However, this does not address the fundamental problem of animal segmentation and relies on visual contrast between foreground and background for accurate tracking. Therefore, researchers must restrict the environment to achieve the best results.
That is, this video tracking technology cannot be used in complex and dynamic environments, or with genetically heterogeneous animals, which makes long-term and large-scale experiments unfeasible.
To overcome the above problems, the team usedconvolutionNetworks,neural networks, improve the segmentation quality.In addition, semantic segmentation techniques are utilized to provide generalization capabilities for dynamic environments that traditional background subtraction cannot address.

2. Tracking mice in special positions
As the environment becomes less suitable for tracking, the frequency of bad tracking instances in a single video increases. For example, when the mouse is in a corner, near a wall, or on a food cup, tracking is highly inaccurate.
In most cases, incorrect tracking is still caused by poor segmentation of the mouse from the background. This includes two types of errors: parts of the background are segmented into the foreground (e.g., shadows); and mice are misclassified as background when parts of the mouse are removed from the foreground (e.g., an albino mouse that matches the background color).
To solve this problem, they used an infrared light source to record the movements of mice under different light and dark conditions in the experiment, used an infrared beam grid to detect the current position of the mice, and collected 24 hours of video, including the time when the mice were on the food cup or in the corner. Finally, they optimized and analyzed the video data.
The team compared the trained neural network with human annotations and found that it outperformed Ctrax, an open-source, freely available machine vision program.

When this technology is used more widely, it will not only save a lot of time for researchers, but may also bring more new discoveries, such as using it to track small animals in complex environments and see a more vivid and magical animal world.
In the future, we can also use machine learning to find the source of the epidemic, understand the needs of pets at home, track the movements of rare animals, and make the world a better place!