Microsoft Team Wins Data Competition by Significantly Improving Weather Forecast Accuracy
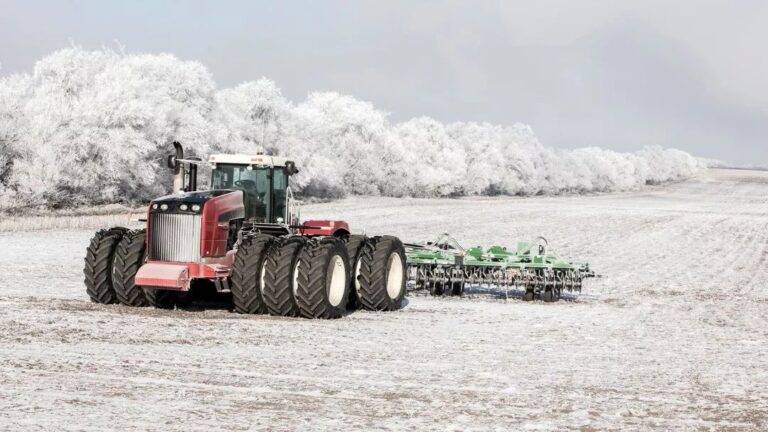
By Super Neuro
Scenario description:The Microsoft research team has used machine learning technology to greatly improve the accuracy of temperature and precipitation forecasts in seasonal climate forecasts, providing convenience for places that rely on weather forecasts.
Keywords:Machine learning, weather forecasting, AI for Earth
You must have been fooled by the weather forecast. But no matter how unreliable the weather forecast is, people are still keen on it because the weather is closely related to us. For example, in agricultural planning, it is necessary to make the most accurate predictions possible to prepare for the next climate conditions.
Lester Mackey, a machine learning researcher at Microsoft Research Cambridge, pointed out that the current common forecasts for the next two to six weeks are "just a matter of luck." To address this situation, he applied his expertise in AI to weather forecasting to increase the chances of accurate predictions.

Using machine learning techniques, Mackey and his team developed a state-of-the-art technology.In a sub-seasonal weather forecast competition held by the U.S. Bureau of Reclamation, their team's model was far more accurate than the previous standard model.The purpose of this competition is to solve water resource management problems, including responding to changes in hydrological conditions and preventing droughts or floods in advance.
Mackey's team's research project also received support from Microsoft's AI for Earth program to encourage them to use technology to make greater contributions to society.
When machine learning and weather prediction collide
Mackey knew little about weather and climate forecasting until he met his future collaborator, climatologist Judah Cohen of Atmospheric and Environmental Research.

Cohen previously worked in climate risk consulting and was passionate about weather forecasting, but the shortcomings of traditional methods slowed his progress, so he tried to improve it through machine learning.
Cohen contacted Mackey because he learned that Mackey was good at machine learning technology, which could collect effective information from historical weather and climate data to help him improve his forecasting methods.
Encouraged by Cohen, Mackey gradually realized the applicability and advantages of machine learning in this field. They then started an in-depth collaboration, combining their areas of expertise.
For a period of time, it was common to analyze and predict the weather from historical data, but by the 1980s, this method gradually fell into disuse. Since then, physical models simulating the evolution of the atmosphere and ocean have begun to dominate the industry. With the exponential growth of computing power, these models have become more and more mature and popular.

“Today, major climate centers use large supercomputers to simulate the movement of the atmosphere and ocean,” Mackey said. “As technology has improved, predictions have improved, but people have paid less attention to historical data. The common approach now is to take the current weather conditions and extrapolate their differential equations to make predictions.”
But Mackey and Cohen had other ideas. They wanted to incorporate the vast amount of historical data into the sub-seasonal forecast.
Lucas Joppa, Microsoft’s chief environmental officer for AI for Earth, said:“Mackey is working on a hard problem, and the advantage is that the technology he’s exploring happens to have great utility in weather forecasting and has great applicability in broader social and economic areas.”
The competition was used to test the waters, but the results were…
As Mackey and Cohen's research collaboration unfolded, Cohen received notice of a competition sponsored by the U.S. Bureau of Reclamation to improve the accuracy of weather forecasts such as temperature and precipitation in the western United States.

Mackey said that current models have relatively mature methods for both short-term and long-term: for weather models within seven days, the performance is already quite good; and for very long-term forecasts, such as one to more than ten years, climate prediction models are also well developed.
But subseasonal forecasts are an intermediate stage that rely on a combination of variables that influence short-term weather, such as daily temperature and wind, as well as seasonal factors such as El Niño and Arctic sea ice.Because of this, it is difficult to predict.
During the 13-month competition, they need to continuously make weather forecasts for the next 3-4 weeks, or 5-6 weeks. The competition items include forecasts for precipitation and temperature.
In order to make better predictions, they used two different machine learning methods. One is to apply a variety of data, including temperature and precipitation records in historical data, as well as a collection of physical forecast models such as sea ice concentration and El Niño phenomenon (corresponding to MultiLLR). The other is to use only a single historical data of the project to be processed (corresponding to AutoKNN).
“We do a forecast every two weeks, and in between, it’s mostly acquiring new data, processing the data, building infrastructure to test new methods, and then developing new methods and evaluating them,” Mackey explains. “Then we stop, make a forecast, and repeat for the next two weeks.”
In the later stages of the competition, Mackey's team tried to combine the two methods and achieved the best prediction results. The results of the competition were also announced on March 7, US time.

Mackey and Cohen's team achieved impressive results, ranking first in predicting temperatures three to four weeks out and second in predicting total precipitation five to six weeks out.
The weather is predicted, but what about their future?
The relevant technical details and research results were published by the team in a paper on arXiv.org. In order to encourage more people to join this challenge, they also made the created dataset public.
Paper address: https://arxiv.org/pdf/1809.07394.pdf
Dataset address: https://dataverse.harvard.edu/dataset.xhtml?persistentId=doi:10.7910/DVN/IHBANG
Microsoft’s AI for Earth program is providing funding to Mackey and his colleagues to encourage more people to expand and improve their machine learning-based predictive techniques.
In addition, Microsoft's AI for Earth intends to provide Mackey and his team with more funding to expand the team and improve their research.
Kenneth Nowak, a water resources researcher at the U.S. Bureau of Reclamation, said:"We hope that this method will be further improved and applied in a wider range of fields."He added,The government agency will "look for more opportunities to use machine learning" in weather forecasting.

Chohen and Mackey made a breakthrough study out of curiosity about the potential value of AI in sub-seasonal climate forecasting. Chohen is also full of expectations for their next work.“I see the advantages of machine learning. This is not the end, it’s more like the beginning, and there is still a lot of work waiting for us to do.”
