With the Emergence of AI, Wang Feng Will No Longer Be Able to Keep His Title As a Music Mentor
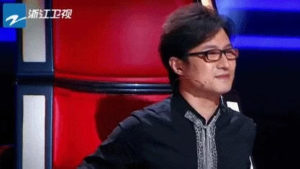
By Super Neuro
Recently, two engineers from Sweden and the Netherlands stated in a paper that they have developed an AI model that can also measure your musical talent and quantify your musical preferences with data.
Wait, are they trying to steal the business from music instructors?
"Please tell me about your musical dream." Wang Feng has asked this question many times to young people who step onto the stage and want to realize their dreams through singing. His purpose is to explore their passion for music and the stories behind it.

For ordinary people, the question "Do you have a talent for music? What type of music do you like?" is probably something most people have never had the chance to explore. Even if they like music, they can only give a vague range, or find some patterns from the "recommendations for you" on the player, but now AI can give you a clear answer.
Assessing Musical Talent with Gold-MSI
Bruce Ferwerda, assistant professor of computer science at Jönköping University (JU) in Sweden, and Mark Graus, a data scientist at Maastricht University in the Netherlands, have jointly developed an AI model that can, in theory, assess the musical talent of non-professionals using the Gold-MSI index.
The Gold-MSI index is specifically used to evaluate the potential musical attainments of ordinary people. Its full name is Goldmiths Musical Sophistication Index. It can evaluate the musical talents of non-professionals from the perspectives of psychology and behavioral science based on the user's music preferences (such as love of classical or pop music).

The specific evaluation criteria mainly involve the following five aspects:
-
level of commitment to music (e.g., money and time spent on music);
-
Musical perception (such as accuracy of musical listening);
-
Whether the candidate has received music training (such as vocal training, etc., including professional and non-professional);
-
Pitch condition;
-
and emotional cognition of music (i.e., participants described their emotional experience of the music).
For this index, the key to assessing its accuracy lies not only in the above five aspects, but also in whether it can accurately judge the user's music preferences. Because there was no system that could accurately quantify the user's music preferences, the index often came to wrong conclusions during actual testing.
Now, through this AI evaluation model, users' music preferences can be accurately quantified. It is said that the accuracy of the model can reach more than 90%. This is very helpful to improve the evaluation accuracy of the Gold-MSI index.
Give it a try if you get a chance, and then send the result to Wang Feng. Maybe there won’t be a need for auditions.
Using algorithms and psychological models to understand your music preferences
A person's musical taste and ability can be inferred and quantified, but for many non-professionals, they may not be able to accurately express their musical preferences.
The main reason is that they may be interested in multiple different types of music at the same time. When making a choice, these different types of music will interfere with their judgment of their own musical preferences, especially in modern music, where many songs incorporate some styles.
Bruce and Mark say the model can infer your favorite music genres and singers and determine your musical preferences.
On August 22, they published a paper titled "Predicting Musical Sophistication from Music Listening Behaviors A Preliminary Study" on Arxiv.or, describing the model in detail.

Interested friends can view it through this website: https://arxiv.org/pdf/1808.07314.pdf
The paper states that the model can infer the user's music preferences based on their music playback records through machine learning algorithms and psychological models, and improve the music experience through the order of music playback.
Among them, psychological models can be used to explain user behavior and emotional changes. Based on this, AI can understand the user's psychological changes towards different music through sentiment analysis, and thus provide an in-depth interpretation of music preferences.

When listening to a random playlist, if a song you don't like suddenly plays, it will affect your mood.
In terms of data collection, they built a data training set through the API interface provided by Spotify and invited 61 users to participate in the model effectiveness test.
The entire training set collected 21,080 music playback records of participants based on indicators such as music style (i.e. liveliness or liveliness), rhythm, track popularity, and popularity of music creators, in order to quantify their preferences for different styles of music.
The algorithm analyzes the user's historical music data to determine which music the user is more interested in. It also determines the user's talent in music theory based on the complexity and technicality of the music selected by the user, as well as whether the user has music creation experience.
Generally speaking, people who are more sensitive to music are more willing to appreciate complex music and have potential for music creation, such as practicing musical instruments or listening to various types of music.
So listening to symphonies often can really improve your musical sense.