Don’t Go Back to School, the Teachers’ Tactics Have Become More Sophisticated.
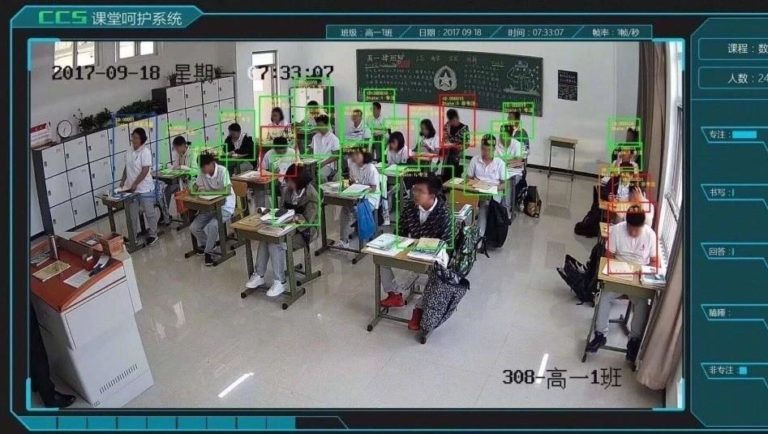
By Super Neuro
Today is International Teachers' Day. My friend circles have been flooded with blessings for teachers. Everyone is paying tribute to teachers and missing the campus. But if you knew the "dire straits" living environment of middle school students today, I wonder what you would think.
Recently, Hangzhou No. 11 Middle School has piloted the Smart Eye system, which installs a combination of cameras in the classroom to capture students' expressions and movements in class. With the help of facial recognition technology, it collects students' 6 types of classroom behaviors and 7 types of classroom expressions.

The teaching system that caused a stir is called the "Smart Classroom Behavior Management System", or "Smart Eye" for short.
It is said that it can accurately capture studentsRead, stand up, raise your hand, lie on the table, listen and writeAnd other 6 behaviors, combined with the students' facial expressions to analyzeNeutral, happy, sad, angry, scared, disgusted, and surprised 7 emotions.
Smart Eyes - The student who loves to tell tales the most in the class
After the information is simply processed by the system, a string of codes will be generated, such as listening carefully to the class is A, lying down to sleep is B, answering questions is C, and the status of the students will be judged based on this. A good status, such as listening carefully to the class, will add points, and a bad status, such as sleeping in class, will deduct points.

These data will be pushed directly to the teachers after class, so that they can understand the class effect and students' reactions.
Teachers can use this to optimize their teaching methods, and schools can also use it to judge the quality of teachers' teaching. However, due to the presence of cameras, it is inevitable that students will feel a little uncomfortable, which is understandable.
Regarding the privacy and data leakage issues that the public is concerned about, it is said that the data information generated by this system can only be viewed by the teachers and school seniors, and other teachers or outsiders have no right to view it.

(The red circle above is the facial recognition device, and the red circle below is the camera that collects information)
The hardware part consists of a rotatable camera and a facial recognition device. There are about three in each classroom, which scan every 30 seconds.
All cameras are only used to collect behavioral information and will not be used for surveillance or recording. There will be no issues of privacy or data leakage.
The 11th Middle School plans to install the system in all classrooms this year, which can monitor students' emotions in class and record their attendance by face recognition. However, the system is still in its early stages and needs more data for it to learn and improve the accuracy of emotion recognition.
In the future, they plan to replace all card swiping in the school with face scanning, creating the country's first cardless campus. I wonder if this was inspired by Alibaba's Jack Ma next door.
Classroom monitoring may be a good thing
Hangzhou No. 11 Middle School is the first to bring high technology to campus, but we hope that the school will not use emotion recognition, which is used to improve teaching quality, as a real-time monitoring tool. After all, even though the campus is a public place, every student should still retain some personal privacy within certain limits.
Ultimately, the reason why the Smart Eye system has attracted attention and discussion is because people feel that students are being monitored. But think about it the other way around, Smart Eye claims that data is not stored or made public, which is actually similar to the traditional teacher's eyes.
Therefore, the most important thing is the "User Agreement". What the school should clarify now is the usage and authorization scope of the video content in Huiyan.
Technical Implementation of Emotion Recognition
It has become a reality that machines can recognize human emotions, but the complexity of massive training data and model building has daunted many researchers.
Now, five big guys have come up with a lightweight emotion recognition model that can not only automatically complete the classification of training sets, but also makes the model building process simple, greatly reducing the threshold for creating emotion recognition models.
Emotion recognition is based on technologies such as image and face recognition, and identifies human emotional states by analyzing people's physical behaviors (such as facial expression recognition, voice, and posture).

The diversity of human facial emotions
Although neural networks for emotion recognition have been widely used in fields such as healthcare and customer analysis, most emotion recognition models still cannot deeply understand human emotions, and building such a model is very costly and difficult to develop.
Lightweight emotion recognition model, let’s take a look
To solve this problem, five engineers from Orange Labs and the University of Caen Normandy (UNICAEN) in France jointly published a paper "An Occam's Razor View on Learning Audiovisual Emotion".
In their paper, they proposed a lightweight deep neural network model based on audio-visual emotion recognition (i.e. emotion recognition using audio and video). It is said that the model is easy to train, can automatically classify the training set, and has high accuracy, and can achieve good performance even with a small training set.
The model proposed in this paper followsOccam's razor, and trained based on the AFEW dataset. Through multiple processing layers (for feature extraction, analysis, etc.), audio and video are preprocessed and feature analyzed at the same time, and finally the two are combined to output the emotion recognition result.
AFEW stands for "Acted Facial Expressions In The Wild". It is a collection of expression recognition data that provides test data for emotion recognition model training and the EmotiW series of emotion recognition challenges.
All data comes from video clips containing facial expressions edited from film and television dramas, including the six basic expressions of "happiness, surprise, disgust, anger, fear and sadness", as well as neutral expressions.
In order to make the model better recognize the training data in AFEW, the five R&D personnel also made some innovations to the model:
1) Reduce feature dimensions and simplify model analysis processes through transfer learning and low-dimensional space embedding;
2) Sampling by scoring each frame to reduce the size of the training set;
3) Use a simple frame selection mechanism to weight the image sequence;
4) Different forms of feature fusion are performed in the prediction stage, that is, the video and audio are processed separately and then fused.
This series of innovations has greatly reduced the number of parameters that characterize the characteristics of the data set, simplifying the model training process while improving the accuracy of emotion recognition. In the 2018 emotion recognition competition EmotiW (Emotion Recognition In The Wild Challenge), the recognition accuracy of the model reached 60.64%, ranking fourth.

The model ranked fourth in the 2018 EmotiW audio-visual emotion recognition model accuracy rankings.
The top-ranked emotion recognition model was developed by SEEK TRUTH, a domestic AI financial technology company, with an accuracy rate of 61.87%. The second-ranked model was developed by DeepAI, an AI startup in California, with an accuracy rate of 61.10%.
The model can automatically add labels to the training set
Compared with other emotion recognition models, the biggest advantage of this model is that it is easy to build and train. It can make it easier for computers to perceive human micro-expressions, including body language.
At present, most emotion recognition models are trained through the facial expressions of characters in film and television dramas. Firstly, the acquisition cost is low, and secondly, the expressions are rich.

However, when these data are input into the training set, they are not classified (i.e., emotion labels are added to each video). Therefore, before training, they need to be classified manually or through other methods, which is difficult to operate and can easily lead to distortion of the training set.
The audio-visual emotion recognition model developed by Frédéric Jurie and others can automatically classify expressions in the training set through a deep neural network for use by the model.
In this way, the difficulty of model training is reduced while the recognition accuracy is improved.
This model also proves that lightweight neural network models can achieve good results and are easier to train, in stark contrast to the increasingly complex neural network models today.
In the future, they will further study how to better integrate data in non-video formats and recognize data with fewer or even no classifications.