CrowdAI Uses Satellite Images to Assess California Wildfire Losses
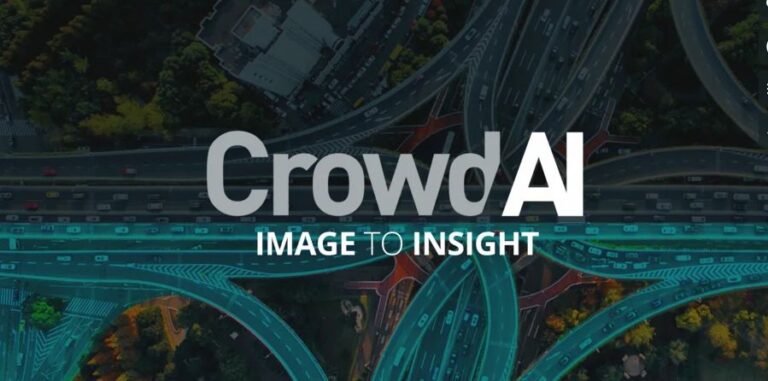

Scene description:By comparing satellite images of the disaster-stricken areas before and after, we can mark the buildings and determine the extent of the disaster in the area, thus helping to rationally allocate rescue resources.
Keywords:Natural disaster prevention, image recognition, satellite imagery, convolutional neural networks, object recognition
Natural disasters have caused huge losses
The wildfires that raged across California for three weeks last fall were the deadliest wildfire disaster in the United States in a century, claiming 85 lives and destroying an estimated 140,000 homes.
According to statistics, hundreds of natural disasters occur around the world every year, killing nearly 50,000 people, displacing tens of millions of people, and causing economic losses of more than US$100 billion each year.

After a natural disaster occurs, the traditional response method is for rescue workers to determine how to deploy rescue resources through on-site observation, rescue calls, etc., which is time-consuming and inefficient.
In disaster relief work, it is crucial to obtain comprehensive and accurate information in a timely manner to achieve the rational allocation of resources. Now, machine learning can help us race against time and save more lives and losses.
It only takes 1 second to locate the disaster site and assess the extent of the disaster.
During the California wildfire, a company called CrowdAI participated in the rescue by using satellite data and integrated image vision technology.
CrowdAI uses satellite imagery from Spacenet and Deepglobe, as well as data from DigitalGlobe and Planet Labs, to train convolutional neural networks.
It only takes one second to predict and assess the extent of the disaster, and then report the assessment results to the rescue command center to help scientifically allocate rescue resources and formulate more scientific rescue plans.

With the help of CrowdAI's customized deep learning model, in addition to labeling conventional house buildings, it has also expanded to independent structures such as carports, utility sheds and barns.
In this fire, after identifying the structure from the satellite imagery, the AI model marked the location of the damage with red dots based on a comparison of images before and after the disaster.

Expanding to the entire area, the severity of the disaster can be determined by the number of marked points, and different colors can be used to distinguish the severity of the disaster.
Finally, marking them on Google Earth or ArcGIS can provide guidance for disaster relief and reconstruction work.

Devaki Raj, founder and CEO of CrowdAI, said of the ongoing pursuit of evaluation speed:“When disasters happen, we have to predict them quickly, and that’s why we need this speed.”
Many rescue workers and government officials use this rapidly generated data to more rationally coordinate rescue efforts, which improves the efficiency of solving critical problems.
“Over the past decade, massive amounts of satellite and drone data have become available online,” said Devaki Raj, founder and CEO of CrowdAI. “We are using the latest advances in computer vision to process this data.”
In order to complete the disaster assessment as accurately as possible, previous methods required a large amount of data training, but Jigar Doshi, head of machine learning at CrowdAI, mentioned that "because machine vision technology is already very mature, we don't need to train a large model (for disaster conditions) to conduct effective assessments."
Satellite data for humanistic care under the guidance of AI
CrowdAI is similar to other companies that use satellite imagery and other technologies to provide data services, but the difference is that they focus their efforts on natural disasters.
After Hurricane Michael last year, CrowdAI worked with telecommunications provider WOW to assess building damage in Panama City, Florida. Based on satellite images of the city provided by NOAA, the data they provided helped WOW to deploy workers appropriately according to the degree of damage to achieve rapid recovery.
CrowdAI has also collaborated with Facebook AI to study the assessment of damage caused by hurricanes and wildfires. "From Satellite Imagery to Disaster Insights"It was also accepted by the NeurIPS conference.

In the paper, their research achieved good results: it achieved 88.8% accuracy when identifying roads damaged by Hurricane Harvey near Texas in 2017, and 81.1% accuracy when identifying damaged buildings in the Santa Rosa fire.

In addition, another article from CrowdAI 《Residual Inception Skip Network for Binary Segmentation》, which is about identifying road networks from satellite images, was accepted by another top conference, CVPR.
In terms of disaster prediction, CrowdAI is also exploring predictive disaster models. It is said that they are trying to actively develop deep learning tools that can surpass satellite images by integrating data such as wind, precipitation and social media.
Google and McKinsey Global Institute have produced a report on cases where AI benefits humanity. The report states that “AI can provide more accurate rescue and emergency preparedness, is faster than human rescue, and has a wider range of applications.”
Undoubtedly, among the many current machine learning cases targeting satellite data, most are aimed at industrialization and commercialization. CrowdAI provides satellite data with more humanistic application scenarios and makes intelligence more humane.