The Performance Far Exceeds That of the SAM Series Model. The University of Zurich and Others Developed a General 3D Blood Vessel Segmentation Basic Model, Which Was Selected for CVPR 2025.
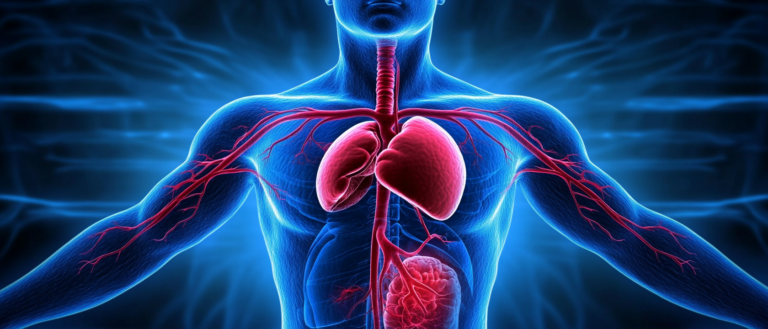
If the human body is compared to a huge city, then blood vessels are undoubtedly the "roads" of this city. Arteries, veins and capillaries correspond to highways, urban roads and country roads. They work together to transport nutrients, oxygen and other substances to various parts of the body through blood, thus maintaining the efficient and stable operation of this "city". When these roads have problems, people's bodies will naturally develop diseases.
Blood vessel segmentation is an important means to check whether there are problems with these "roads". Just like discovering problems through traffic images in urban construction, it is a key task in medical image processing.Blood vessel segmentation can accurately identify and extract the structure of blood vessels from medical images.It can be applied to the analysis, diagnosis and treatment of various vascular diseases. For example, in cardiovascular disease, the segmentation of coronary arteries helps doctors assess the degree of stenosis of the blood vessels and thus formulate appropriate treatment plans for patients.
In recent years, vascular segmentation has made significant progress with the support of computer and medical imaging technology. However, when faced with imaging for specific tasks, accurate and robust segmentation of fully connected blood vessels remains a challenging problem, especially in 3D vascular segmentation. On the one hand, due to the limitations of the blood vessels themselves, the complex and tiny vascular geometry brings about a sharp increase in the difficulty of segmentation;On the other hand, there are significant domain gaps caused by the limitations of imaging methods and protocols, specific signal-to-noise ratios, vascular patterns, imaging artifacts, and changes in background tissue.
Although there are many medical image segmentation methods based on basic models, such as SAM (Segment Anything Model) and SAM-Med3D and VISTA3D for 3D medical images,However, these models still have limitations in the task of vessel segmentation.Therefore, 3D vessel segmentation remains a labor-intensive task for medical staff and researchers, requiring extensive manual voxel-level annotation to further achieve accurate vascular image analysis.
To address this problem, a team from the University of Zurich, ETH Zurich and the Technical University of Munich proposed a basic model designed specifically for 3D blood vessel segmentation, vesselFM. The model is trained on a large-scale dataset (Dreal) and synthetic data generated by domain randomization (Ddrand) and a generative model based on flow matching (Dflow).It can achieve segmentation and generalization capabilities that are superior to existing advanced models in zero-shot, single-shot, and few-shot scenarios.
The related research was published under the title "vesselFM: A Foundation Model for Universal 3D Blood Vessel Segmentation" and was selected for CVPR 2025.
Research highlights:
The study proposes a universal base model for 3D vascular segmentation with zero-shot and generalization capabilities, which can be used "out of the box" by researchers and medical staff
* The team curated the largest 3D vessel segmentation dataset, including carefully processed real 3D vessel images and matching voxel-level annotations
* The study proposes a fine-grained neighborhood randomization strategy for 3D vessel segmentation and introduces flow matching into 3D medical image generation.

Paper address:
https://go.hyper.ai/lVad9
Dataset: 3 heterogeneous data sources
The researchers used three heterogeneous data sources to train it.The first is a dataset Dreal (Diverse Real Data) containing different real data; the second is 2 synthetic data sources,They are the domain random dataset Ddrand (Domain Randomization) and the data collected from the flow matching-based generation model Dflow (Flow Matching-Based).
in,Dreal is the largest real dataset used in 3D blood vessel segmentation tasks to date.A wide range of imaging modalities covering various anatomical regions from different organisms,Contains over 115,000 3D patches of 128³ shapes from 17 annotation sources.Specifically, its extensive clinical imaging includes MRA, CTA, X-rays, two-photon microscopy, and vEM, and biological samples come from the brain, kidneys, and liver of humans and experimental mice, which provide vascular patterns with different structural and functional characteristics for research, as shown in the figure below.

The researchers then looked at the different types of tissue, imaging methods, and protocols.Dreal is further divided into 23 data sets.Each dataset is preprocessed and finally patches with target shape of 128³ are extracted from the images and their corresponding labels.

The researchers introduced a domain randomization strategy to create Ddrand, as shown in the figure below. This method generates a large number of diverse synthetic image-mask pairs by applying a series of spatial transformations and artificial artifacts to real vascular data, thereby improving the model's robustness to real data.Specifically, it can be divided into three steps, namely foreground generation, background generation and background fusion.

In the prospect generation phase,The researchers used 1,137 vascular patches v with a shape of 250³ provided by Wittmann et al. as the basis for the synthetic mask.These vascular plaques are derived from graphical representations of corrosion casts with high fidelity.
The main method used by the researchers is to generate a wide range of realistic vascular patterns through spatial transformations such as random cropping, flipping, dilation and scaling, random elastic deformation, and binary smoothing. Next, the researchers simulated various foreground artifacts present in real vascular images by selecting artifact transformations such as bias field, Gaussian noise, Gaussian smoothing, dropout, offset, blur, etc.
In the background generation phase,The researchers modeled background images containing a variety of background geometries with different textures.There are three main variants: Sphere (non-overlapping spheres), Polyhedron (the image is split into multiple polyhedral regions using Voronoi), and Geometric Image without any background.
In the background fusion stage,The researchers merged the foreground into the background by voxel addition and subtraction or by replacing background intensity values with mask intensity values.To expand the image domain, the researchers continuously enhanced the image by random bias field, added Gaussian noise, applied random local peaks in k-space, randomly adjusted the image contrast, performed Gaussian smoothing on individual or shared σ values of all spatial dimensions, added Rician noise and Gibbs noise, performed random Gaussian sharpening, and randomly transformed the intensity histogram, finally obtaining the synthetic image Xdrand (as shown in Figure b above).
In order to further enrich the distribution of real data Dreal, the researchers further trained and sampled a conditional generation model F based on Flow Matching to generate a third data source Dflow, as shown in the figure below.

Model architecture: Introducing deep generative models and domain randomization strategies
Overall,vesselFM is a general base model designed specifically for 3D vessel segmentation that can accurately segment 3D vasculature in a variety of modalities and tissue types.During the model design process, the researchers trained it through three heterogeneous data sources to achieve its powerful segmentation and generalization capabilities.

In this process, the researchers introduced two key steps:They are the deep generative model and the domain randomization strategy respectively.The above dataset introduction section elaborates on the domain randomization strategy. This section focuses on the deep generative model used to generate the Dflow dataset.
Deep generative models are an important means of generating synthetic medical images, mainly based on diffusion models, which are used to generate a large amount of high-fidelity synthetic data. In order to perform segmentation tasks on these data, it is necessary to accurately match image-mask pairs. Methods such as Med-DDPM and SegGuidedDiff are designed to meet this challenge.They incorporate semantic conditioning by channel-wise concatenating segmentation masks into the model input, resulting in image-mask pairs that adhere to consistent anatomical constraints.The former is tailored for 3D brain imaging synthesis, while the latter is designed for 2D breast MRI and abdominal CT generation.
In this study,The researchers took a different approach from the diffusion model and used a generative modeling approach called Flow Matching.It generates new samples by learning the continuous transformation process of data from a known distribution to a target distribution, which shows superior performance on natural images compared to the diffusion model.
Specifically in the study, the generative model F uses a θ-parameterized network to represent a learning time-dependent velocity field v, and then maps the sample x₀~N(0,I) to the sample x₁ of the data distribution through an ordinary differential equation (ODE). At the same time, in order to train the model F, the researchers also optimized the flow matching objective to minimize the loss of the predicted speed and the sampled true value speed on the time scale.
In addition, the training model F also adopts mask and category conditions,Mask conditioning is achieved by concatenating the mask channels with the input image xₜ.The researchers added the class embedding to the temporal embedding to incorporate the class information, and then injected it into the intermediate feature layer through addition. To generate Dflow, the researchers finally discretized x₁ through Euler integration to sample a large number of images Xflow, as shown in the figure below.

Experimental results: performance is better than the current most advanced model
In order to verify the validity and reliability of vesselFM,The researchers conducted a comparative evaluation.And demonstrated its segmentation capabilities in zero-sample, single-sample and few-sample scenarios.
Specifically,Four clinical datasets were used in the validation phase: SMILE-UHURA, MSD8, OCTA, and BvEM.The researchers extracted 3 patches of shape 128³ from these evaluation datasets and used them to define single-shot and few-shot segmentation tasks on one or all three patches to fine-tune the model. The rest of the data was used for testing and validation. For zero-shot evaluation, the model was directly applied to the test data without prior fine-tuning.
At the same time, 4 basic models designed specifically for 3D blood vessel segmentation were used as comparison objects.They are: tUbeNet, VISTA3D, SAM-Med3D and MedSAM-2.
Using a single NVIDIA RTX A6000 GPU, the researchers sampled 10,000 image masks from model F and generated Dflow in 3 days. To manage Ddrand, the researchers extracted 500,000 image mask pairs from the domain randomization generation pipeline, each with a shape of 128³. The weights of the three data sources used were roughly set according to their size, namely Ddrand (70%), Dreal (20%), and Dflow (10%).
The specific results are shown in the figure below. vesselFM demonstrates excellent generalization and superior performance on all 4 datasets and tasks.In the zero-shot task, on the MSD8 dataset, the Dice score of vesselFM is 5.86 points higher than that of VISTA3D (VISTA3D is trained on 11,454 CT data, which itself includes data from MSD8), which further highlights the powerful inductive bias of vesselFM.

In contrast, the general 3D vessel segmentation model tUbeNet does not perform well in more complex imaging modalities, and the other two general segmentation models SAM-Med3D and MedSAM-2 are unable to segment vessels in zero-shot settings. It is worth mentioning that on the SMILE-UHURA dataset, the Dice and clDice scores of vesselFM in zero-shot scenarios even exceed those of the baseline models in few-shot scenarios.Qualitative results demonstrate the superior generalization capability of vesselFM in zero-shot contexts without suffering from annotator-specific biases.As shown in the figure below.

Deep learning opens a new path for blood vessel segmentation research
In summary, the research related to vesselFM has undoubtedly promoted the progress of 3D vascular segmentation research, provided a new path for the treatment and research of vascular diseases, and is expected to promote the birth and application of new advanced tools, and ultimately achieve the goal of benefiting patients.
Fortunately,vesselFM is not alone in this endeavor.With the development of deep learning technology and the increasing abundance of medical data, processing medical images through artificial intelligence has become an important direction of modern medical reform. More and more laboratories and research institutions have focused on this field and hope to solve the challenges of vascular diseases faced by humans through their own research.
For example, a team from the Chinese Academy of Sciences published a study titled “VesselSAM: Leveraging SAM for Aortic Vessel Segmentation with LoRA and Atrous Attention.” The study proposed an enhanced version of SAM, named VesselSAM.Specifically designed for aortic vessel segmentation.The model integrates the hole attention module and low-rank adaptation (LoRA).The key limitations of SAM are addressed and its ability to capture complex hierarchical features in medical images is enhanced.
Paper address:https://arxiv.org/abs/2502.18185
A team from Shanghai Jiao Tong University in China, together with teams from Shanghai First People's Hospital, Queen's University Belfast and Louisiana State University, published a study titled "Self-Supervised Vessel Segmentation via Adversarial Learning".This study proposes to train two generators through adversarial learning, one is an attention-guided generator and the other is a segmentation generator.They are asked to synthesize fake blood vessels and segment blood vessels from coronary angiography images, thereby learning the feature representation of blood vessels. This paper was also selected for CVPR 2021.
A team from the University of Lisbon in Portugal and a team from the Catholic University of Portugal also published research on vascular segmentation.They proposed 3DVascNet, a 3D retinal vascular network automatic segmentation and quantification software developed based on deep learning.The software not only achieves accurate segmentation of blood vessels, but also can quantify vascular morphological measurement parameters, such as vessel density, branch length, vessel radius, and branch point density. More importantly, the software is free.At the same time, its powerful generalization ability further promotes the ability of medical staff to study three-dimensional vascular networks.The related research was published under the title “3DVascNet: An Automated Software for Segmentation and Quantification of Mouse Vascular Networks in 3D”.
Paper address:https://www.ahajournals.org/doi/10.1161/ATVBAHA.124.320672
In summary, as a critical and challenging task in medical image processing, blood vessel segmentation still has many problems to be solved, but the repeated attempts of researchers have undoubtedly shown us that these problems are crumbling. What is more convincing is that in the near future, vascular diseases may also be gradually conquered with the deepening of artificial intelligence applications.