A Joint Team From China and Japan Used a Large Model to Analyze the Conduction Mechanism of Hydride Solid Electrolytes and Established a Reliable Activation Energy Prediction Model
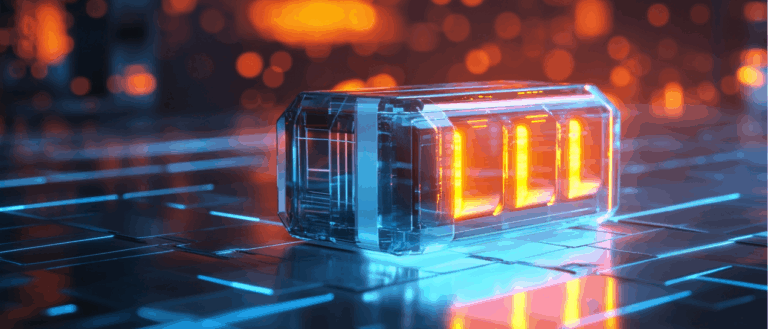
With the rapid development of industries such as electric vehicles and electronic devices, the demand for high-performance batteries has shown a blowout growth. As the core of the next generation of energy storage technology, solid-state batteries have become the focus of global scientific research competition due to their higher safety, energy density and cycle stability. Solid-state electrolytes (SSEs) are key components of all-solid-state batteries (ASSBs). Their performance parameters directly determine the output efficiency and service life of the battery, and have become a technical fortress that scientific research teams are competing to overcome.
The research and development of traditional SSEs has long relied on tedious trial and error methods.Take Japan's Toyota Motor Corporation as an example. This industry giant with more than 1,300 solid-state battery patents once ambitiously planned to commercialize all-solid-state batteries in 2027, but due to the interface stability problem of solid-state electrolytes, it had to postpone the mass production schedule to after 2030. Coincidentally, the huge investment of the U.S. Department of Energy in the "sodium ion battery + solid electrolyte" technology path has also hit a bottleneck due to the complex ion migration mechanism of hydride materials. This fully exposes the limitations of the traditional R&D model——The strong electrostatic interaction of divalent cations in hydride SSEs makes it difficult to capture their dynamic migration behavior using traditional experimental characterization techniques.The inefficiency of the trial and error method has further slowed down the process of technological breakthroughs.
In this context, artificial intelligence has injected transformative power into the research and development of solid-state electrolytes. The rise of large language models (LLMs) has expanded the boundaries of data-driven methods and provided more accurate tools for theoretical predictions. However, the complexity of SSE material systems, especially the multi-scale ion migration mechanisms in hydrides, still poses a challenge to the accuracy of theoretical models. The methodological fragmentation problem that is prevalent in current research also limits the systematic understanding of material systems.
To break this deadlock, a joint research team composed of Tohoku University in Japan, Sichuan University in China and Shibaura Institute of Technology in Japan proposed an innovative framework integrating artificial intelligence and multi-scale simulation.The team successfully revealed the unique "two-step" ion migration mechanism in hydride SSEs by combining a comprehensive SSE database with a large language model and ab initio metadynamics (MetaD) simulations.
The relevant research results have been published in the top international journal Angewandte Chemie-International Edition under the title "Unraveling the Complexity of Divalent Hydride Electrolytes in Solid-State Batteries via a Data-Driven Framework with Large Language Model".
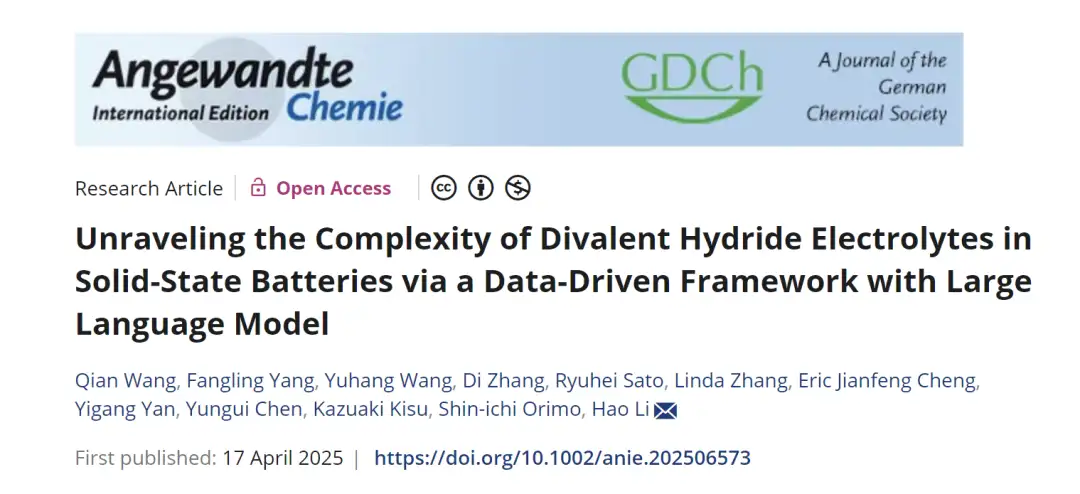
Paper address:
Data-driven and AI-accelerated workflows, an acceleration engine for high-performance solid electrolytes
In the field of divalent solid electrolyte (SSE) research, although experiments and calculations continue to deepen, the diversity of neutral molecules and the slow migration of ions complicate the research due to the limitations of material characterization technology and simulation methods. To this end, the research team developed an innovative big data-driven workflow, using large language models (LLMs) to deeply understand SSE and analyze cation migration.Aims to design fast ion-conducting SSEs for high-performance solid-state batteries (SSBs).
As shown in Figure a below,The process starts with big data analysis, using the Dynamic Database of Solid Electrolytes (DDSE) developed by the team, which covers 2,556 experimental SSE materials, 18,635 sets of ionic conductivity measurement data, and 657 computational SSE materials.Thus capturing and establishing key features.
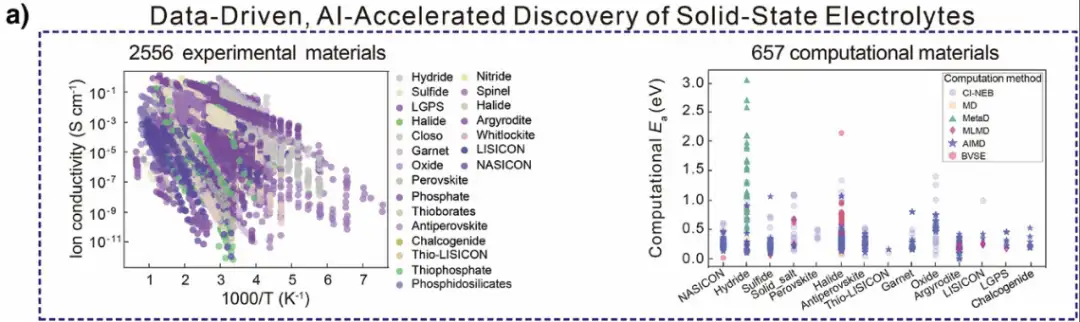
Based on this database, the researchers gained two important insights:
Big Data Analysis of Hydride SSE
As shown in Figure b below, the researchers analyzed the temperature-dependent ionic conductivity of 158 metal hydrides in the experiment and found that in SSEs without neutral molecules, the conductivity of monovalent electrolytes is usually between 10⁻⁷ and 10⁻¹ S cm⁻¹, and the activation energy (Eₐ) is less than 1.0 eV; while divalent ions have strong electrostatic interactions with the surrounding environment and weak conductivity, and there are fewer divalent SSEs without neutral molecules. However, adding neutral molecules to the divalent SSE lattice can promote the migration of divalent ions.Significantly improves conductivity, making its performance comparable to monovalent electrolytes.
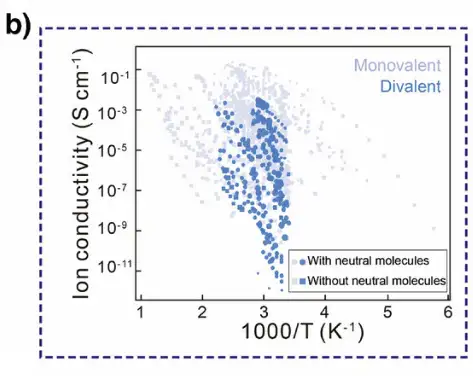
Improvements to traditional simulation methods
To gain a deeper understanding of ion migration in SSE materials, the researchers systematically analyzed the commonly used computational methods in the past 10 years. The benchmark analysis of experimental and theoretical calculations based on LLM overturned the understanding of the commonly used transition state search methods for SSE cation migration, revealing that the traditional simulation method is significantly different from the experimental Eₐ, indicating that more reasonable methods, such as MetaD, should be used first, especially for SSEs with high Eₐ. As shown in Figure d below, the researchers subsequently conducted a series of MetaD simulations to further reveal the mechanism of cation migration, introduced multiple theoretical descriptors to describe Eₐ, and used a global optimization strategy to clarify the structure of the predicted high ionic conductivity SSE composition.
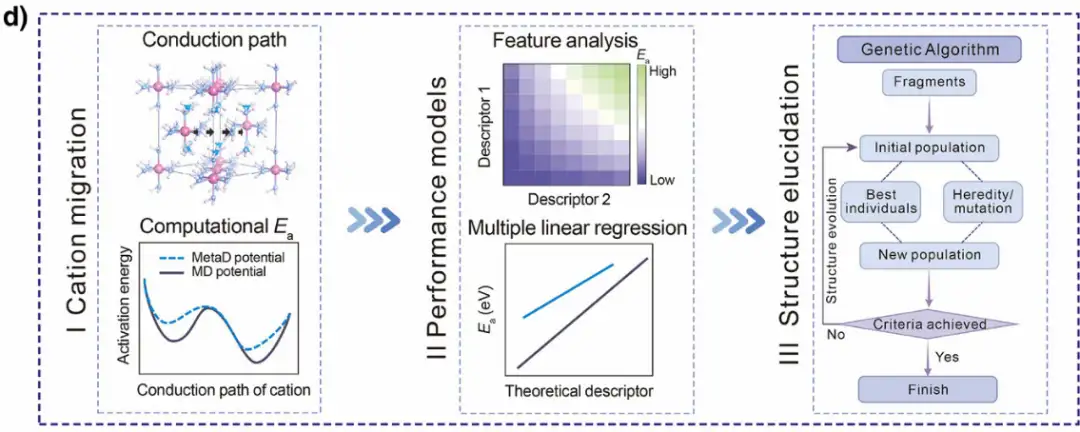
The researchers also conducted a series of MetaD simulations to explore the mechanism of cation migration, introduced multiple theoretical descriptors to describe Eₐ, and used global optimization strategies to clarify the structure of the composition of high ionic conductivity SSE. On this basis, the structure-performance relationship was derived and the new SSE was successfully predicted. Overall,This workflow provides a powerful tool for predicting the kinetics of hydride cation migration and identifying promising new SSE candidate materials.It effectively solves the problem of difficulty in clarifying limitations caused by data and method limitations in the research of divalent solid electrolytes.
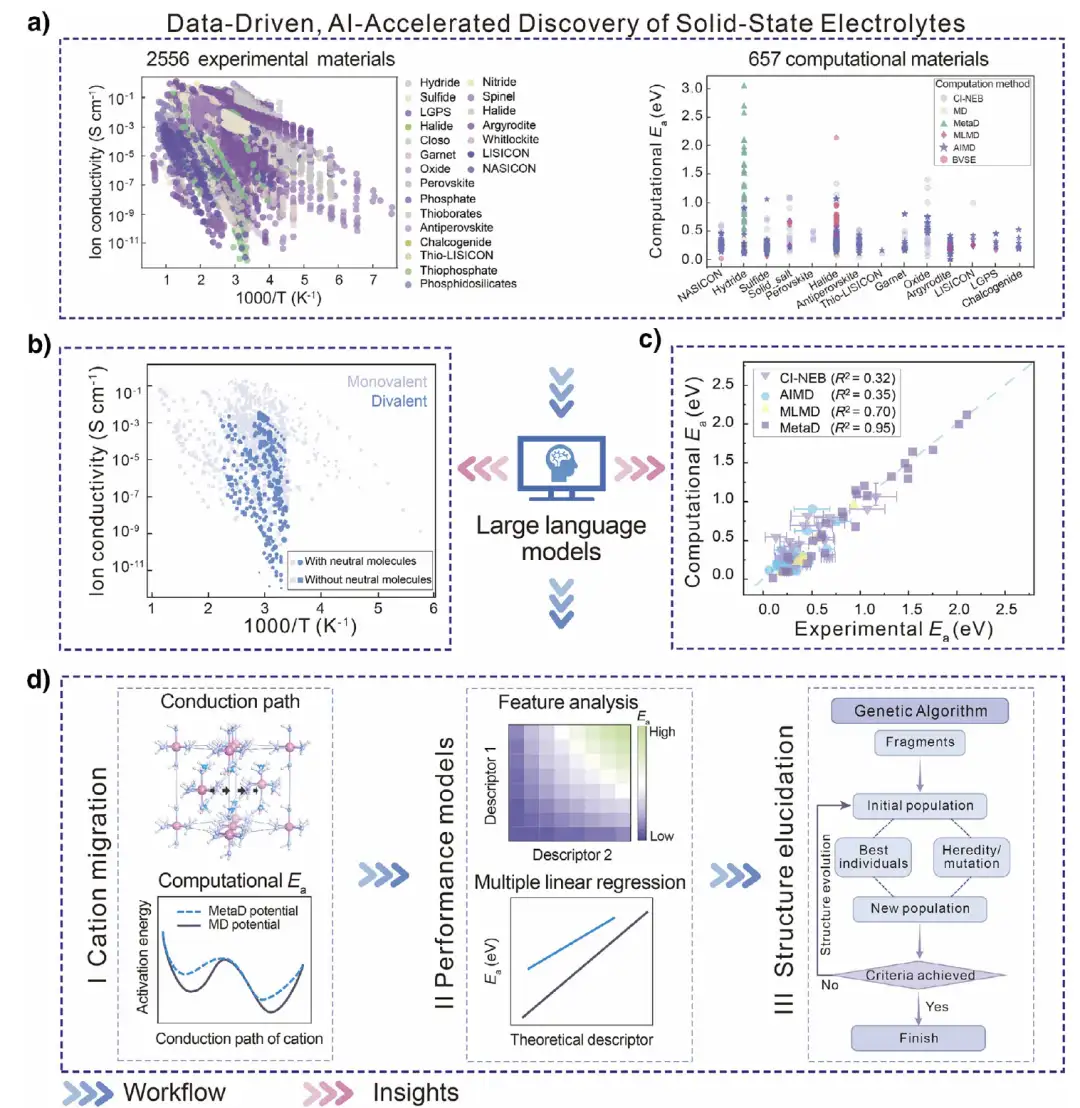
Hydride SSE research: revealing a new mechanism of SSE cation migration and establishing a reliable activation energy prediction model
In the research field of hydride solid electrolytes (SSEs), the research team built a systematic model system to accurately predict cation migration and explore new mechanisms.As shown in Figure a below, the model covers 21 different SSEs, involving various cations (Cations, monovalent such as Li⁺, Na⁺, K⁺, divalent such as Ca²⁺, Mg²⁺, Zn²⁺), anions (Anions, small nest BH₄⁻, network B₃H₈⁻, "cage-like" closed BₙHₙˣ⁻, CBₙHₙˣ⁻) and neutral molecules (Neutral molecules, such as H₂O, NH₃, etc.). The latter promote migration in the system by reducing cation coordination and expanding the crystal lattice.
The model is trained based on the MetaD simulation method.At the corresponding phase temperature, the cations, anions and neutral molecules in the system are systematically evaluated to obtain key data such as the free energy surface.The simulated activation energy (MetaD Eₐ) was compared with the experimental value, and the agreement was very high (R²=0.95), especially the divalent SSE containing neutral molecules performed well, which verified the reliability of the model.
In the study of cation migration mechanism,MetaD simulation captures the novel migration process.As shown in Figure cd below, taking Mg ion as an example, it dissociates from the original tetrahedral coordination, moves toward the neutral molecule NH₃ and transfers to the interstitial site, migrates along the interstitial site, and alternately coordinates with [BH₄]⁻ to complete the migration. In the process, two quasi-stable coordination states of "coordination unlocking" and "rotating wheel" are presented. A similar process is also seen in the migration of Li⁺ in LiBH₄·NH₃. Further analysis found that, as shown in Figure eg below, this type of SSE has two activation energy values (Eₐ₁ and Eₐ₂), corresponding to the process of cations moving out of the coordination environment and migrating to vacancies, respectively. Eₐ₂ is close to the experimental measurement value, indicating that it may be the rate-determining step.
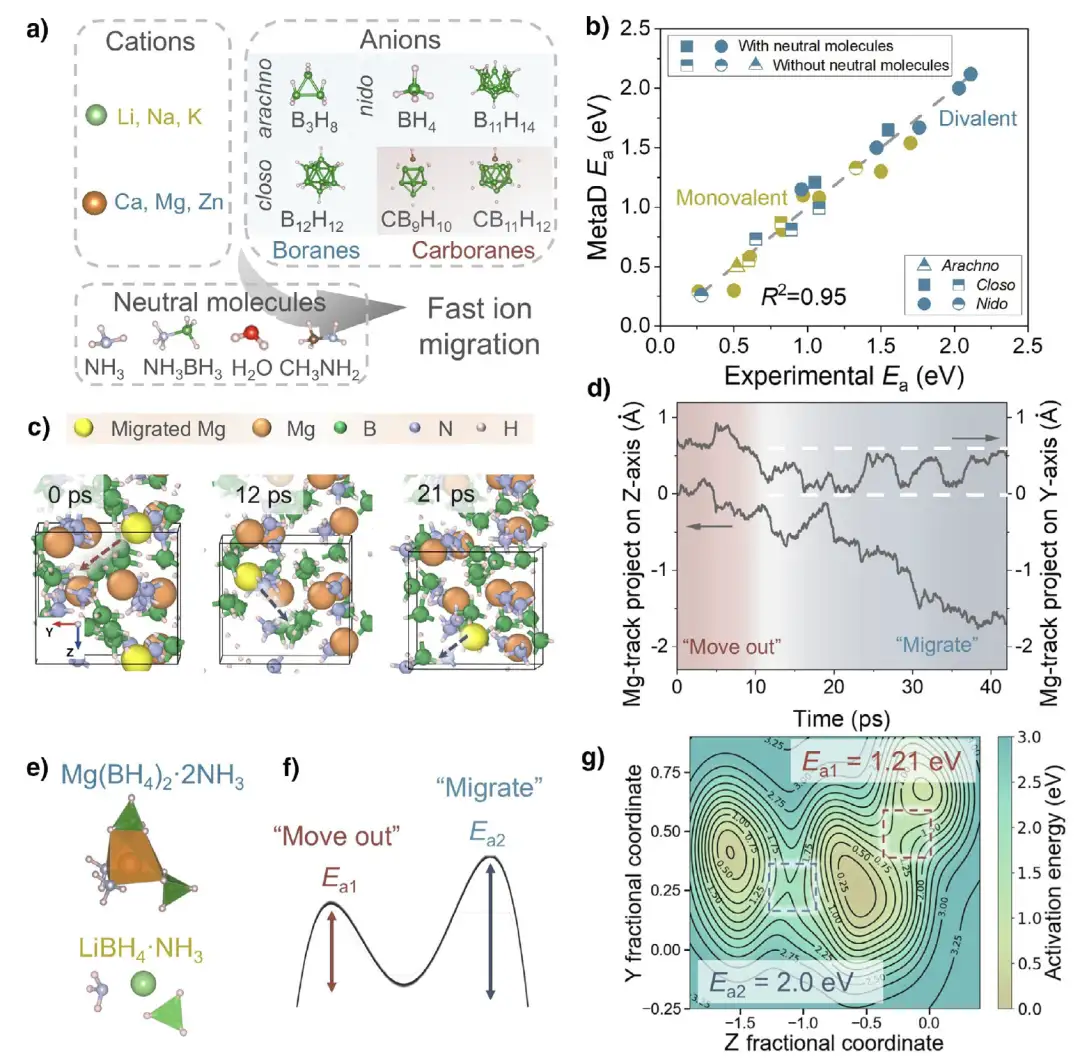
In the study of activation energy prediction, the research team introduced 8 theoretical descriptors: unit cell volume (V), electronegativity (X), atomic number (Z), binding energy (bₘ), anion distance (d), number of neutral molecules (n), atomic radius (ratom) and ionic radius (rion), and performed single linear regression and multiple linear regression analysis on closed (Closo-SSEs) and nested (Nido-SSEs) systems. The nested system (BH₄ system) has a higher R² value, and the multiple linear regression model (such as Eₐ=a₁P₁+a₂P₂+b) can effectively unify different SSE types, as shown in the figure below.The prediction ability is strong in divalent SSEs containing neutral molecules, with R² values reaching 0.91 and above.
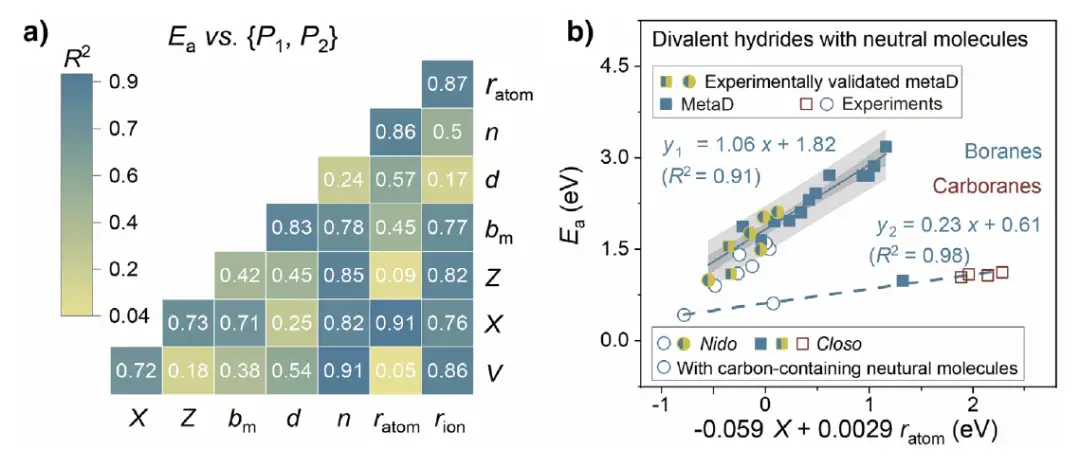
Based on the above models and analysis,The research team predicts high-performance divalent hydride SSE,Combining genetic algorithm (GA) with USPEX software package to carry out crystal structure prediction, we explored stable structures. For example, M(BH₄)₂・ 6(CH₃)₂CHNH₂「MBCCN」, whose cation is coordinated with two [BH₄]⁻ and two neutral molecules,The formation energy indicates that the structure is stable, and MetaD simulation verifies that its Eₐ value is close to the predicted value with an error of about 0.05 eV.
Artificial intelligence drives solid electrolyte research and development: from innovation to industrialization
In the field of solid electrolyte research, AI is undergoing a profound transformation from an auxiliary tool to a core driving force, leading to a fundamental change in the paradigm of material research and development. Many scientific research teams and companies around the world have achieved remarkable results in this transformation. The team of Academician Ouyang Minggao of Tsinghua University in China has built a full-chain intelligent research and development system of "AI reading of literature, AI writing of reports, AI calculation of models, and AI optimization", which has greatly shortened the development cycle of traditional battery materials from several years to several months.The Dynamic Database of Solid Electrolytes (DDSE) developed by the company has integrated data of more than 25,000 experimental materials.Combined with the large language model, a breakthrough was achieved in which the prediction error of ionic conductivity was less than 5%.
Toyota cooperated with Idemitsu Kosan Co., Ltd. of Japan to use AI algorithms to optimize the stability of sulfide electrolyte interfaces. The all-solid-state battery samples developed have a cycle life of over 2,000 times and an energy density of 400 Wh/kg. Mass production is planned for 2027. Microsoft's Azure Quantum team used AI models to screen 32 million material combinations and found a sodium-based solid electrolyte with ion conductivity comparable to lithium-based materials, but with a 70% reduction in cost. Related results have entered the pilot stage. DeepMind's GNOME model predicts 2.2 million new materials, including 528 lithium fast ion conductors.Its independently developed robotic laboratory has successfully synthesized 736 predicted materials with a verification accuracy rate of 80%.
Paper link:
https://www.nature.com/articles/s41586-023-06735-9
These breakthroughs not only significantly improve material performance, but also promote profound innovation in R&D models.A team from Shanghai Jiao Tong University used machine learning to screen out 12 highly stable electrolytes from 29,000 garnet structures.The computational efficiency is 95 times higher than that of traditional DFT methods.
Paper link:
https://www.sciencedirect.com/science/article/abs/pii/S2211285521005929
The dynamic database (DDSE) built by Tohoku University in Japan updates global experimental data in real time and provides material design guidance for more than 100 companies in combination with AI prediction. CATL, a leading Chinese new energy innovation technology company, has established an intelligent design platform for battery materials.It has over 180 million pieces of molecular data and 1 million pieces of crystal data, as well as more than 10 special research and development databases such as positive electrodes, negative electrodes, and electrolytes.It has a computing power of more than 1 PFlops and can complete material screening and closed-loop verification within 90 days. In addition, AI has shown unique advantages in solving solid-solid interface problems. The nano-wetting model developed by Zhang Qiang's team at Tsinghua University optimizes the interface stress distribution through AI, which increases the battery cycle life by 3 times.
Paper link:
https://pubs.acs.org/doi/10.1021/jacs.4c08115
Currently, the deep integration of AI and solid electrolytes is accelerating the industrialization process. Toyota plans to launch an all-solid-state battery equipped with an AI-designed electrolyte, which is expected to have a range of more than 1,000 kilometers; CATL's sulfide electrolyte pilot line has a yield rate of 95%, and its production capacity is expected to exceed 1,000 tons in 2025; the open source nature of large vertical models such as DeepSeek enables small and medium-sized enterprises to access AI R&D capabilities at low cost.Promote industry-wide efficiency improvement by 1-2 orders of magnitude.These advances indicate that solid-state battery research and development has entered the era of "intelligent creation" from "trial and error exploration", and AI is becoming the core engine for breaking through material bottlenecks and accelerating technological iteration.
Reference articles:
1.https://baijiahao.baidu.com/s?id=1824647127596448596&wfr=spider&for=pc
2.https://stock.stockstar.com/RB2025012400049753.shtml
3.https://www.toyota.com.cn/toyotatimes/tinfo/index.php?t_id=559&lmid=100
4.http://www.istis.sh.cn/cms/news/article/45/26764
5.https://baijiahao.baidu.com/s?