Selected for ICLR 2025 Oral, Zhou Hao's Team From Tsinghua AIR Proposed a New Paradigm for Protein Pre-training to Decipher Protein Family Evolution
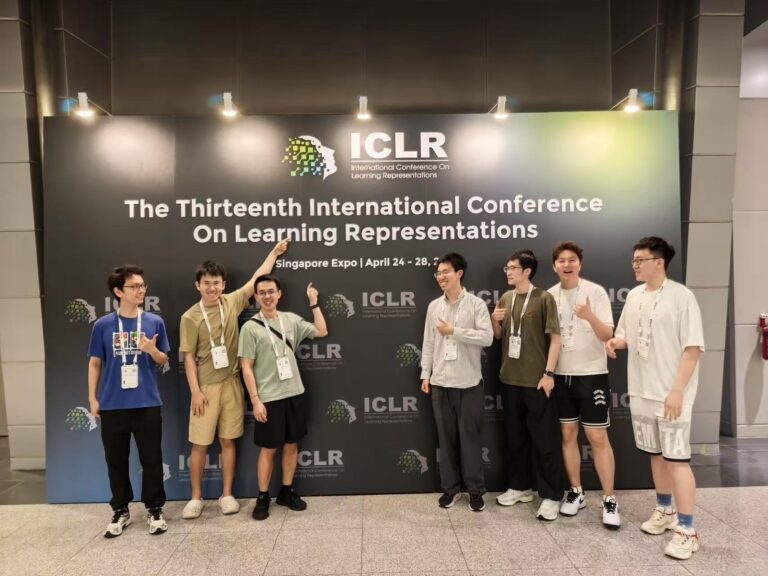
The AIR GenSI research group of Tsinghua University and the School of Pharmacy of Tsinghua University jointly proposed a tool for protein family-specific generative modeling - ProfileBFN (i.e. Profile Bayesian Flow Network). ProfileBFN can expand the discrete Bayesian flow network from the perspective of multiple sequence alignment (MSA) profile and achieve efficient protein family design. Empirical results show thatWhile generating diverse and novel family proteins, ProfileBFN is able to accurately capture the structural features of the family.
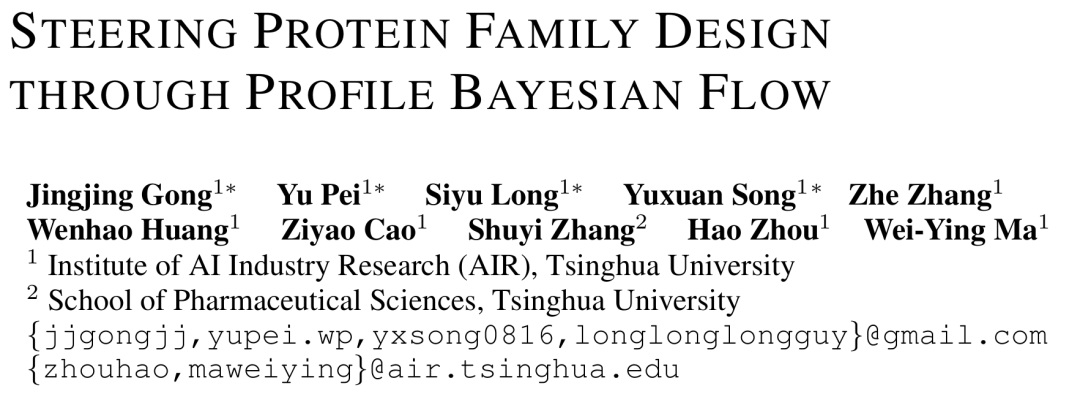
The related results were selected as oral papers in ICLR 2025 with the title of "Steering Protein Family Design through Profile Bayesian Flow". At the same time, another achievement of the team, CrysBFN, was also selected for ICLR 2025 Spotlight, and the research paper title was "A Periodic Bayesian Flow for Material Generation".
In the last session, the team proposed the Geometric Bayesian Flow Network GeoBFN, and the related results were selected for ICLR 2024 Oral under the title "Unified Generative Modeling of 3D Molecules with Bayesian Flow Networks".
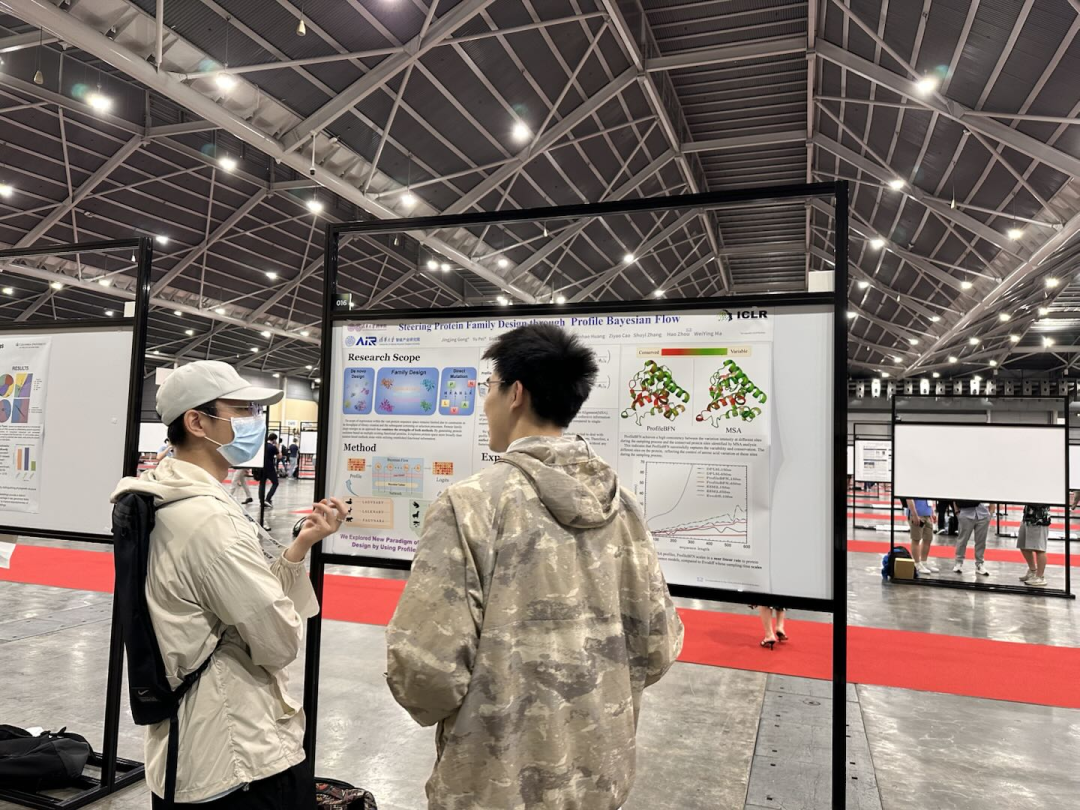
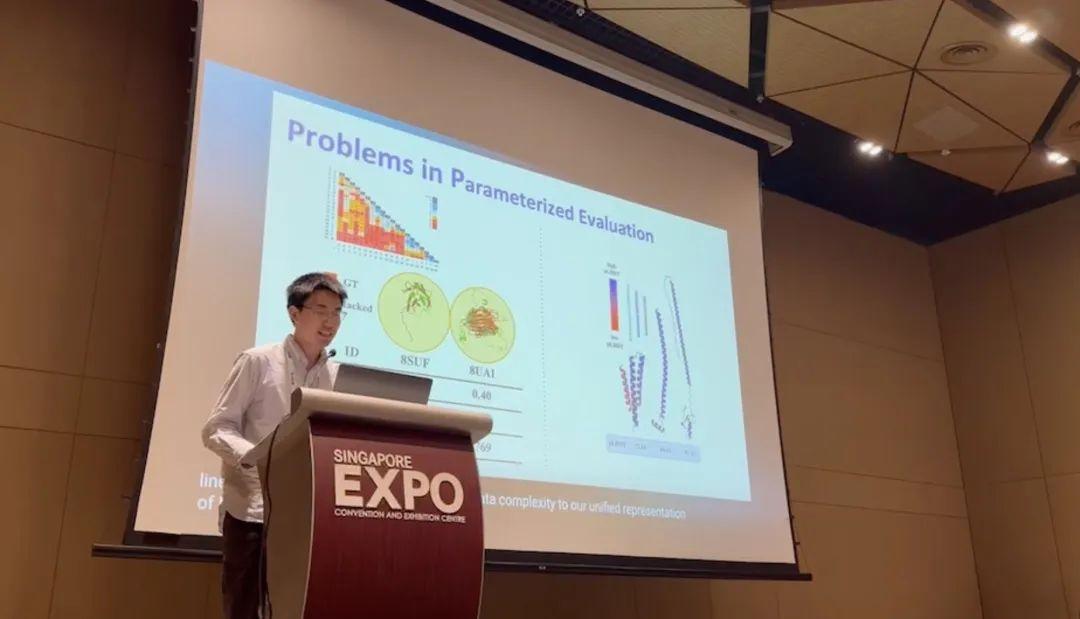
Paper link:
The open source project "awesome-ai4s" brings together more than 200 AI4S paper interpretations and provides massive data sets and tools:
https://github.com/hyperai/awesome-ai4s
Multiple sequence alignment: the cornerstone of protein structure prediction
Multiple sequence alignment (MSA) refers to the process of aligning three or more biological sequences (DNA, RNA or protein). Performing multiple sequence alignment helps to discover and identify similar regions due to functional, structural or evolutionary relationships, providing a more comprehensive perspective on the relationship between biological macromolecules.
In recent years, the use of MSA information has become an important part of protein design. In milestone works such as AlphaFold and ESM, there are special modules to encode MSA information:
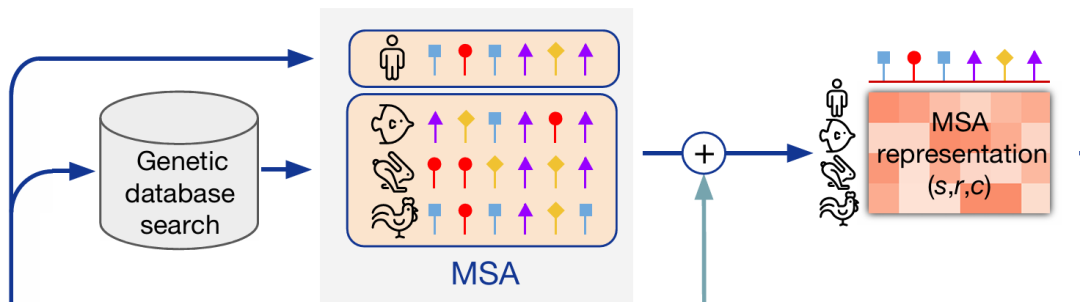
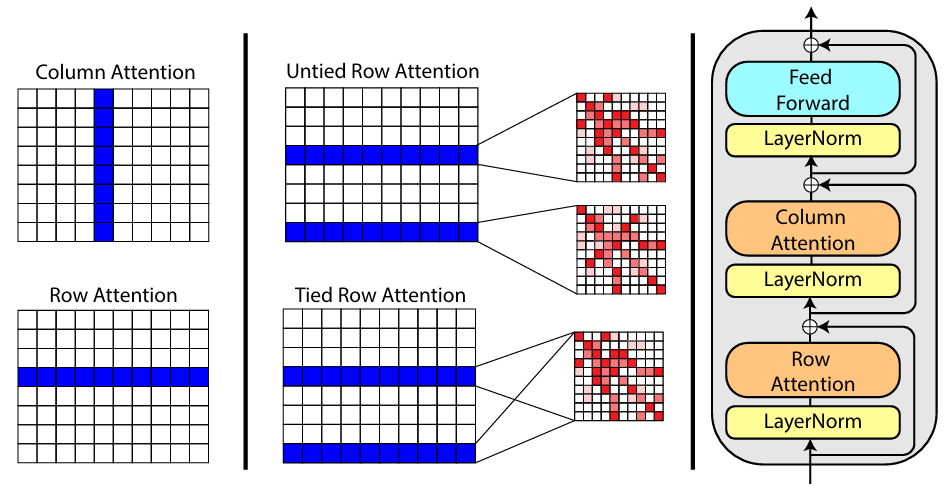
There are many sequences for success, and there are many sequences for failure.
MSA is a treasure trove of evolutionary information, but existing models seem to have overestimated their ability to mine it. With the development of technology, the depth of MSA input to deep generative models has continued to increase, but the effect has encountered a bottleneck, which has questioned the cost-effectiveness of adding MSA information. The fundamental reason is that there is serious uncertainty in both the quantity and quality of MSA:
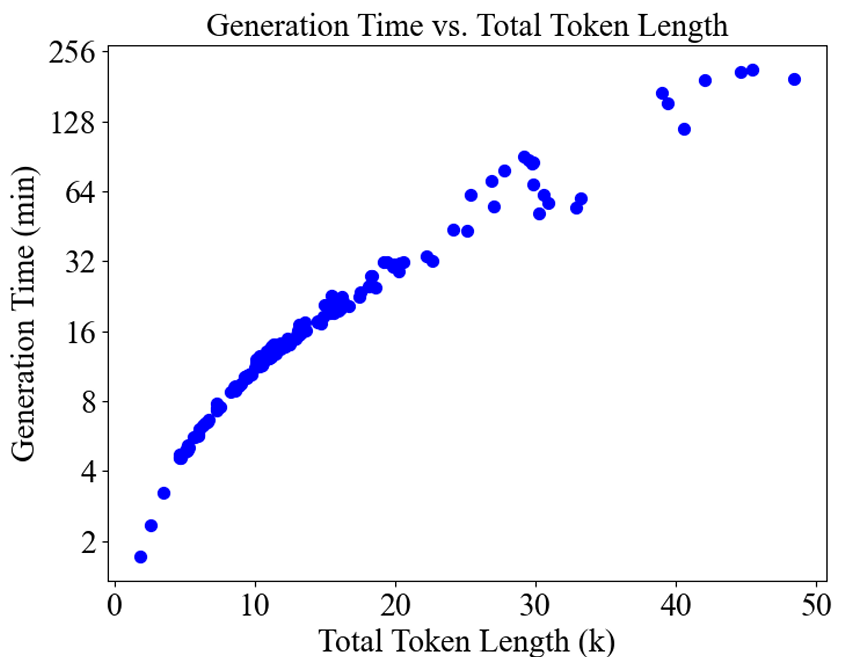
Researchers call sequences that meet a certain degree of similarity in multiple sequence alignment homologous sequences. In terms of quantity, for some "orphan" proteins, there may be no more than 10 homologous sequences, while for some proteins, more than 10,000 homologous sequences can be searched, which causes great confusion for large models, resulting in a waste of resources and an impact on efficiency.
In fact, how can humans speculate on the ingenuity of nature? In the evolution of billions of years, convergent structures reflect the effect of natural selection, while mutations provide new possibilities for evolution. For these special species in special environments, they often retain the original information at the beginning of the evolutionary tree, which is precisely the basis for the deduction of co-evolution theory. If homologous sequences are used as model input, this information is bound to be overwhelmed by a large amount of other irrelevant information, and only a high-probability representation can be modeled. To solve this problem,ProfileBFN models each cluster of homologous sequences as a unified representation that is independent of the number.
A good homologous sequence should contain as much homologous information as possible. Experiments show that in most cases, using a few homologous sequences with the largest information entropy can achieve the same effect as using hundreds of homologous sequences. Some homologous sequences differ by only a few amino acids, which brings a lot of misleading redundant information to the model.
Profile: The cornerstone of the next generation protein pedestal model
Science is based on discovery.The innovation of ProfileBFN lies in discovering the large amount of information redundancy existing in the original MSA. If 100 homologous sequences are sorted according to the information entropy method, the model can achieve the same effect by training only the first 20 sequences. To this end, a bridge between single sequence and multiple sequences needs to be established, which is why Profile appears:
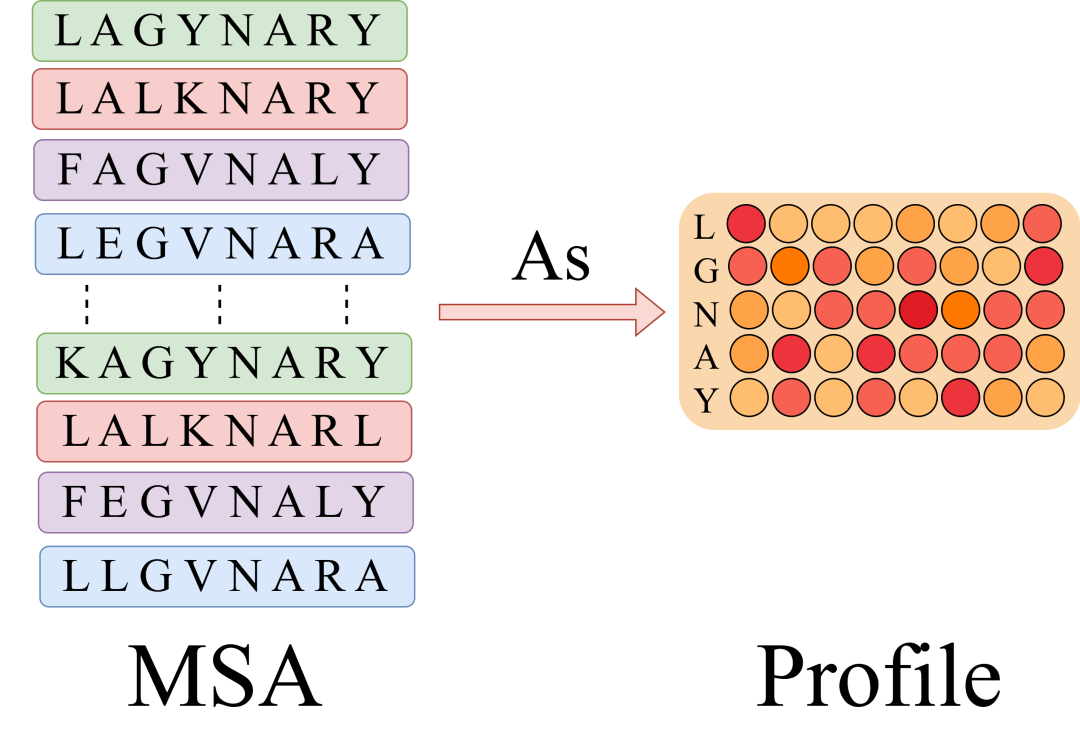
Intuitively, Profile is a column-by-column statistics of the number of amino acid occurrences in a multiple sequence alignment. Furthermore, if there are 10,000 homologous sequences, each with a length of 100, Profile will directly compress them from [10000,100] to a list of [20,100] (20 common amino acids), which greatly simplifies the computational complexity. In particular, a single sequence can also be regarded as a special Profile, except that each column has only one 1.
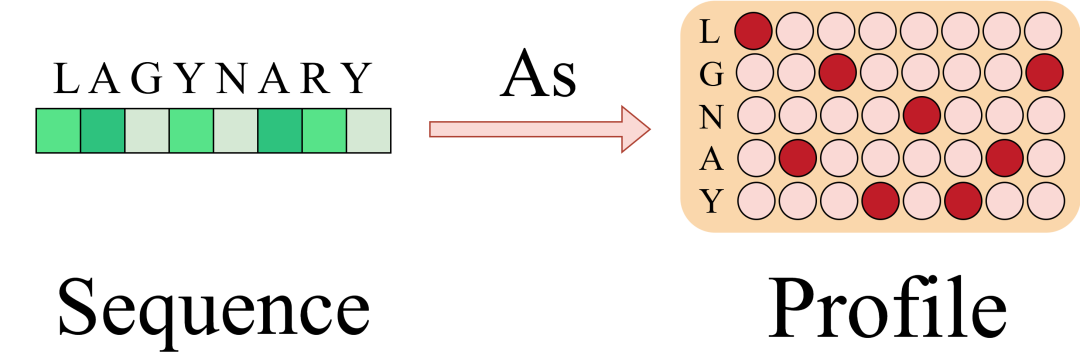
ProfileBFN found that compression from MSA to Profile not only did not cause the serious information loss that was originally expected, but also greatly improved the model performance.This can be understood as: in the great wave of building Profile,Each homologous sequence votes on the type of amino acid that appears at this position, concealing minor contradictions and highlighting the overall trend.
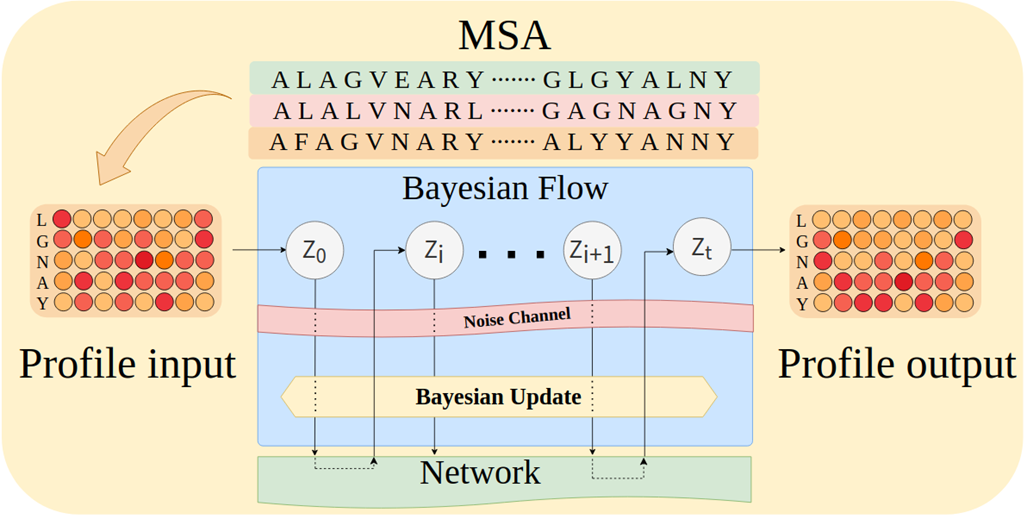
ProfileBFN's unexpectedly strong performance
Compared with the traditional method based on multiple sequence alignment,ProfileBFN relies on 10 times less data and learns 1.5 times more contextual information about protein sequences.The effect is immediate!
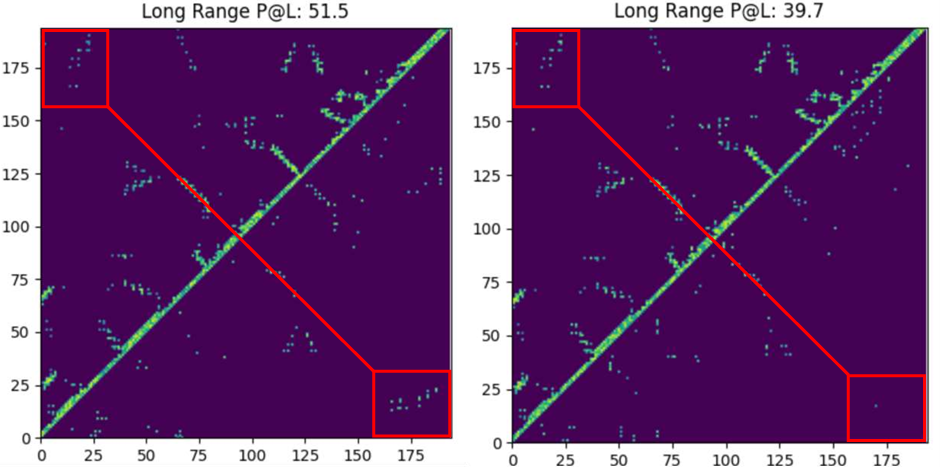
After exploration, it has been confirmed that ProfileBFN has a facilitating effect on a variety of downstream tasks:
* Enzyme classification:Improve functional fidelity and reduce screening costs
* Protein Representation Learning:Assisting in multi-task feature extraction
* Protein structure prediction:Enhance homology information and improve modeling accuracy
* Antibody production:Excellent migration effect, accurate prediction of functional areas
Enzymes are a special class of proteins with catalytic activity, and their functional specificity is usually described by EC numbers (Enzyme Commission Numbers). Studies have found that the new enzyme candidates generated by ProfileBFN highly match the wild-type enzyme in EC numbers, which means that the generated proteins maintain a high degree of functional consistency. This feature greatly reduces the difficulty of experimental screening and improves the success rate of new enzyme design.
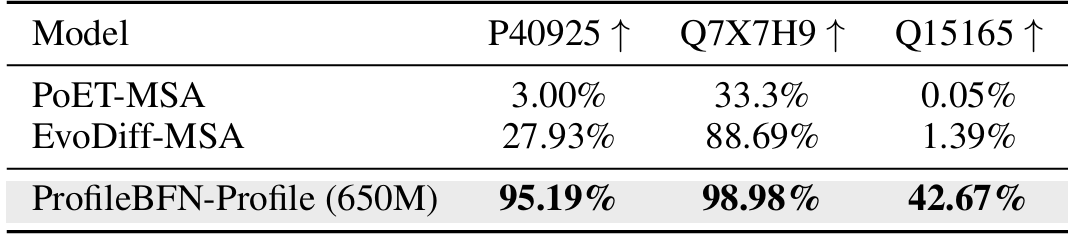
ProfileBFN builds accurate protein representations inside the model while generating proteins. The researchers extracted these representations.Fine-tuning was performed on multiple datasets such as protein thermal stability, protein interaction, and protein subcellular localization. The results showed that the representation provided by ProfileBFN can effectively improve model performance in downstream tasks such as classification. This shows that it is not only a generative model, but also a powerful feature learning tool.
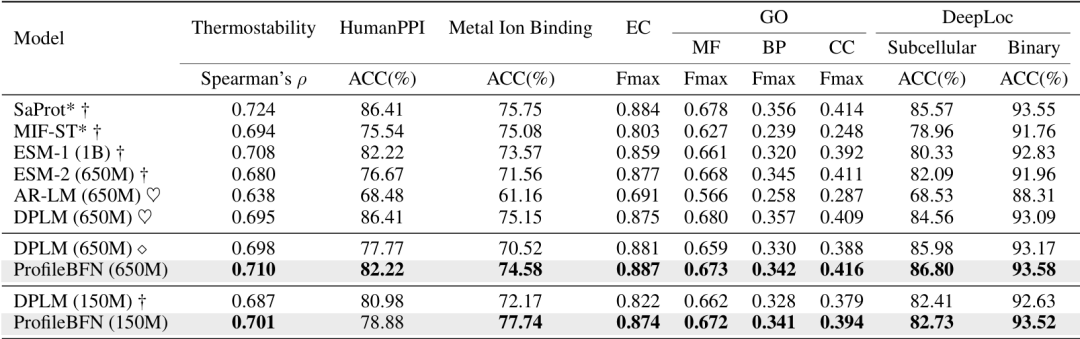
Protein structure prediction is an important issue in structural biology.Especially in the case of orphan proteins (i.e., very few homologous proteins), the accuracy of traditional methods is greatly limited. Studies have shown that ProfileBFN can be used as a homology information enhancer.With only a small amount of MSA data, more high-quality homologous proteins can be generated, thereby improving the prediction accuracy of the AlphaFold series of models. This capability gives ProfileBFN a broad application prospect in the field of structural biology.
Antibodies are functional proteins that can specifically bind to antigens and are of great significance in immune and pathological research. To explore the potential of ProfileBFN in antibody production,The researchers fine-tuned the model based on the OAS (Observed Antibody Space) antibody sequence database.The results showed that ProfileBFN performed well in generating diverse, high-quality antibody sequences.
The extraordinary effect of ProfileBFN comes from the fact that this new research provides a paradigm for generating biological sequences in the post-MSA era:
* MSA does not directly participate in the training process as input, and does not introduce additional training overhead
* In the inference phase, single sequence and MSA are modeled uniformly
* Homologous sequences are both model input and output
BFN perfectly utilizes prior information
Since Profile information is very important, even more important than the original homologous sequence, how can we use Profile information? Bayesian Flow Network (BFN) is a perfect match for Profile! This is reflected in two points:
* BFN models the process from distribution to distribution, the input profile is still the output profile
* Instead of reasoning from scratch, BFN can introduce Profile information as a priori for conditional reasoning
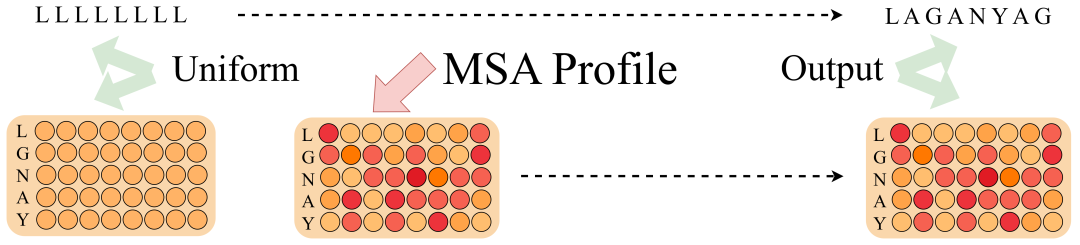
Traditional models such as the AutoRegressive model and the Diffusion model require data (Tokens) as input, and processing Profile information will increase the complexity of the algorithm.
With BFN as the model skeleton, ProfileBFN can further achieve:
* Simplification of tasks. The conditional generation of homologous information is changed to Profile information imitation.
* Improved efficiency. The sampling range is reduced and the effectiveness is improved
ProfileBFN is expected to be the savior of wet testing
In tasks such as synthetic biology, researchers often encounter long cycles, single evaluation indicators, and insufficient credibility. As a protein base model, ProfileBFN can integrate more homologous information under limited resources, make full use of specific prior information, and have a good migration effect on multiple indicators, which undoubtedly makes it the best choice for synthesizing candidate proteins and directed evolution.
About the Research Group
The research field of the Generative Symbolic Intelligence Research Group (GenSI) of Tsinghua University Institute of Intelligent Industry spans the two directions of LLM and AI for Science. It is expected that the two directions will promote each other and thus achieve the ultimate mission of AGI for Science (AI Scientist).
Specific research directions include the next generation of large-scale pre-training technology, large-scale reinforcement learning (Large Scale RL), deep generative models (Deep Generative Models) and their applications in scientific data, etc., focusing on the integration and innovation of basic artificial intelligence algorithms and scientific problems. At present, the team focuses on the cutting-edge theory of deep generative models and the exploration of Scalable Structured-based Generative Models methods, and is committed to solving realistic and challenging scientific problems in the fields of LLM and AI4Sci, such as improving the reasoning ability of LLM and surpassing AF3-level structure generation tasks.
The team can be contacted through the following channels⬇️
* homepage:https://go.hyper.ai/7ye91
* Email: gen_si@163.com
* Xiaohongshu/Zhihu: GenSI
* Twitter: @GenSI_official
* WeChat: 15805171115