Efficiency Increased 73 Times! Japanese Research Team Successfully Prepared 10 Light-driven Organic Crystals Based on Machine Learning
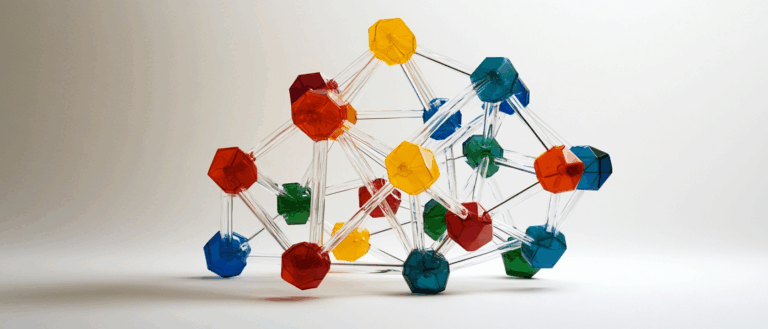
Photo-actuated organic crystals are able to deform when exposed to light. This ability to convert external stimuli into mechanical motion makes them an ideal candidate for the preparation of actuator materials, especially for the manufacture of lightweight and remotely controllable actuators.It is expected to be widely used in fields such as robots and medical equipment.
As mentioned above, when light-driven crystals are deformed, objects can exert force and do work. In this case, when the free deformation of the crystal is completely prevented, a maximum force is generated, which is defined as the blocking force. Effectively controlling the blocking force of light-driven crystals is crucial for their practical applications. For example, maximizing the blocking force can broaden the potential applications of light-driven crystals. Today, researchers can generate smaller forces by adjusting the light intensity, but how to increase the maximum force remains a challenge.Because the maximum force is related to many factors such as crystal properties, crystal size and experimental conditions,The relationship between these parameters and the resulting forces is not yet fully understood.
Recently, a team from Waseda University in Japan used machine learning technology to perform molecular design and experimental optimization of light-driven crystals, successfully maximizing the blocking force. The research was published in Digital Discovery under the title "Machine learning-driven optimization of the output force in photo-actuated organic crystals".
Specifically, the researchers first used LASSO (least absolute shrinkage and selection operator) regression for molecular design, and based on this, synthesized compound crystals and constructed a material library with different Young's moduli and crystal sizes. Secondly, Bayesian optimization was used to efficiently sample from the material library, and the maximum blocking force was experimentally measured to be 37.0mN. This method is 73 times more efficient than the traditional grid search method, greatly improving the applicability of optoelectronic mechanical molecular crystals and helping to optimize other properties of functional crystals.
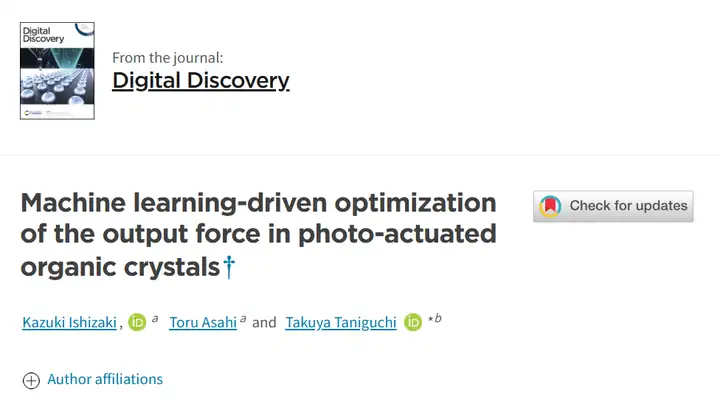
Paper address:
The open source project "awesome-ai4s" brings together more than 100 AI4S paper interpretations and also provides massive data sets and tools:
https://github.com/hyperai/awesome-ai4s
393 data points collected from 118 papers
The researchers searched Google Scholar using organic crystal, Young's modulus and molecular crystal, Young's modulus as keywords. When sorting the data, they found 118 papers that mentioned at least one Young's modulus value and determined that these values were mainly obtained by nanoindentation.
* Young's modulus is a physical quantity that measures a material's ability to resist deformation. The larger its value, the less likely the material is to deform.
* Youngs Modulus Young's modulus dataset download address:
https://go.hyper.ai/igaoF
Since in many cases the literature provided measurements in multiple directions of the material (e.g., along different crystallographic axes), the researchers extracted every relevant data point and also included bending test data and other measurements to ensure the comprehensiveness of the dataset. The final result was a dataset containing 393 data points.
* The anisotropy of a material can be characterized by measuring the Young's modulus of the crystal in different directions.
Two types of machine learning include LASSO regression and Bayesian optimization
As shown in the figure below, based on the collected data set,The researchers used the LASSO method to design crystal molecules and prepare the designed crystals (Crystal preparation).Through a series of experiments, such as X-ray analysis, UV-visible light absorption measurement, nanoindentation experiment, bending experiment, etc., the structural characteristics of the prepared crystals were analyzed, their Young's modulus was measured, and a material library with different Young's moduli and crystal sizes was established.Bayesian optimization is used to perform crystal sampling and iteratively measure the maximum blocking force of different crystals.
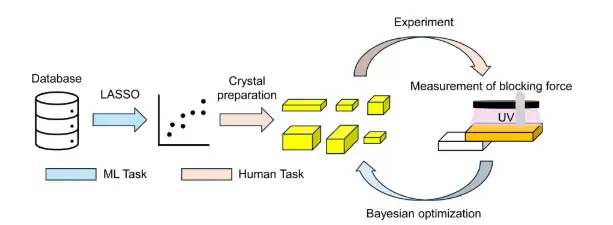
In summary, the two machine learning methods studied the relationship between Young's modulus and functional groups to determine the best experimental conditions, while the human researchers were tasked with preparing crystal samples, conducting experimental analysis, and testing the maximum blocking force of crystals in the real world.
Synthesize 10 light-driven organic crystals and maximize the crystal blocking power
Successful design of light-driven organic crystals using LASSO method
The researchers targeted the salicylideneamine molecule because of its ability to drive crystals with light. In the initial design of the salicylideneamine molecule, the researchers explored the relationship between the molecular substructure and Young's modulus.The goal is to establish a library of salicylaldehyde crystalline materials with different Young's moduli and crystal sizes.
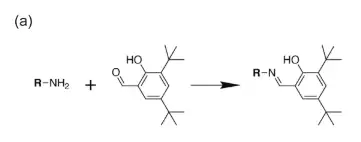
Specifically,The researchers vectorized the molecular structures of the collected dataset of 393 data points.After removing the variables with higher correlation among the explanatory variables, it was found that 18 and 27 substructures were positively and negatively correlated with Young's modulus, respectively. For example, the substructure that forms hydrogen bonds is highly positively correlated with Young's modulus.
To further optimize molecular design, enhanced interpretability is necessary.The researchers used LASSO regression, a method that performs both variable selection and linear modeling.After performing hyperparameter optimization, evaluating LASSO accuracy, measuring experimental errors and other measures, as shown in the figure below, the LASSO model finally retained 7 variables with positive and negative correlations, of which 4 variables (aliphatic hydroxyl groups, nitro groups, carboxylic acids, and amino groups) are substructures that may increase Young's modulus, that is, positive correlation, and the other 3 variables (aromatic hydroxyl groups, halogens, and benzoene rings) are substructures that may reduce Young's modulus, that is, negative correlation.
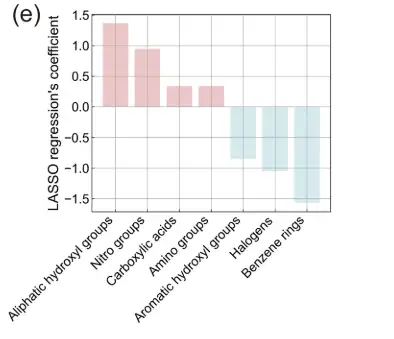
Based on the positive and negative relationships obtained from LASSO regression, the researchers synthesized salicylaldehyde amine molecules (appropriately replacing the above different substructures).Finally, 10 different compounds were obtained.
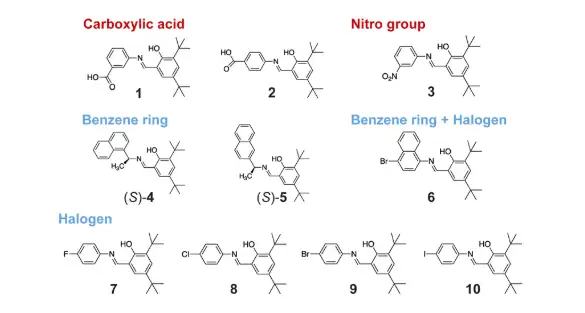
All the prepared compounds crystallized in plate-like form with different crystal sizes. The researchers conducted X-ray analysis on these crystal structures, and the results are shown in the figure below.
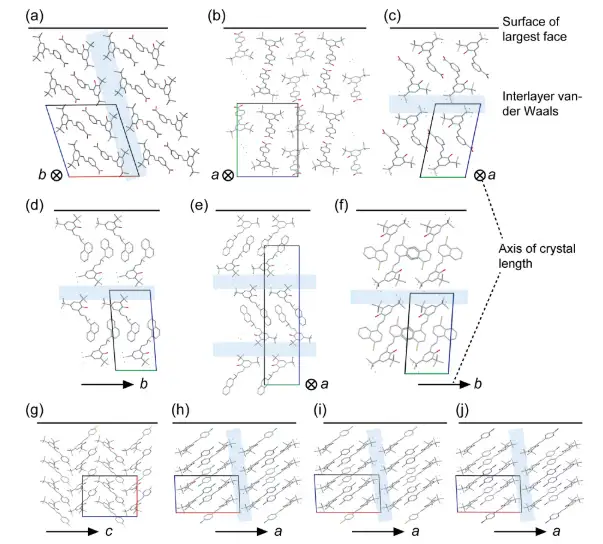
In addition to UV-visible absorption measurements of these compounds, the researchers also used nanoindentation and bending tests to measure the Young's modulus of the crystals along the thickness and longitudinal directions, respectively. The results are shown in the figure below.
Figure a on the left shows that the Young's modulus of crystalline compounds 1–3 is greater than that of compounds 4–6, which is consistent with the prediction of LASSO. However, the halogenated crystals (compounds 7–10) are inconsistent with the prediction, and the crystals of compounds 7–9 have a relatively large Young's modulus. This is because the collected data set includes halogenated crystals that form halogen bonds and have relatively small Young's moduli, but the halogenated crystals in this study do not form halogen bonds.
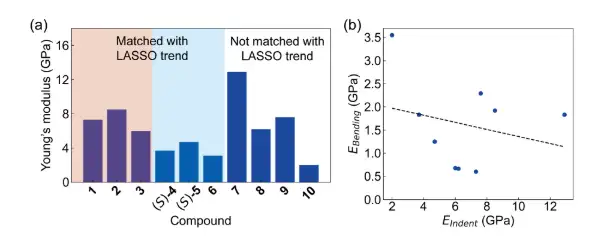
Figure b above shows that crystals with a larger Young's modulus in the thickness direction tend to have a smaller Young's modulus in the length direction, which can be attributed to the anisotropy of organic crystals. When a strong interaction is formed in one direction, weaker interactions will become the main intermolecular interactions in other directions.
all in all,The researchers revealed the relationship between molecular structure and Young's modulus, constructing a design guide for salicylaldehyde molecules.
Bayesian optimization for efficient sampling, maximizing the crystal blocking force to 37.0 mN
After establishing a material library with different Young's modulus and crystal size,The researchers sampled from this material library using Bayesian optimization and tested the crystal blocking force under light illumination.The measurement process is shown in the figure below.

Initially, the researchers measured 10 points (five light intensity conditions for two crystals), with the maximum value being Fmax = 9.8 mN (Fmax is defined as the maximum blocking force minus the initial load). Subsequently, the researchers used Bayesian optimization to sample crystals and iteratively measure Fmax.It was found that during the 100 experiments, Fmax gradually increased.
As shown in the figure below, Fmax gradually increased from 9.8 mN to 16.0 mN, 26.8 mN, 34.4 mN, and finally reached 37.0 mN. It is worth noting that the growth of Fmax in the last three values showed a gradually slowing trend: the increase from 26.8 mN to 34.4 mN was 7.6 mN, and the increase from 34.4 mN to 37.0 mN was 2.6 mN. Although continued experiments may still bring about a certain increase in force value, the increase will be increasingly limited. In addition, the last three Fmax were achieved by crystals of compound 3 under similar conditions.This also strongly indicates that the optimization within the current parameter space is close to the global maximum.
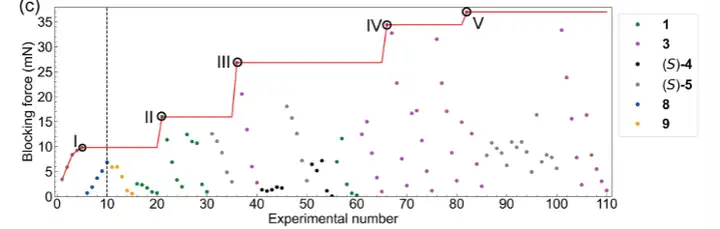
It is worth mentioning that the material library used for this force measurement contains 8 crystals (excluding compounds 6 and 7), but compound 3 showed the most outstanding performance and was also selected the most during the optimization process.
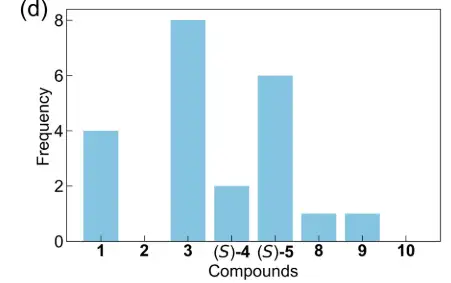
The above may be attributed to the unique structural features of compound 3:
* The large dihedral angle (51.58°) between the salicyl plane and the phenyl plane enables efficient photoisomerization, while the nitro group enhances the photoresponsiveness.
* Stacked molecular layers along the thickness direction promote anisotropic thermal expansion.
* Compound 3 exhibits enol-keto photoisomerization and thermal effect under light irradiation.
* In thin crystals, photoisomerization causes the crystal to bend away from the light source, while in thick crystals thermal expansion dominates.
* The negative thermal expansion coefficient of the crystal along the length direction close to room temperature may contribute to the overall mechanical response.
* In areas of sufficient light intensity, force generation increases with crystal size.
In summary, by applying two machine learning models,This study successfully raised the stopping force of light-driven crystals to a new level, exceeding the previously reported maximum value (about 10mN).
in,LASSO regression reveals the positive and negative correlations between certain substructures of light-driven organic crystals and Young's modulus.For example, substructures that easily form hydrogen bonds are considered to be positively correlated factors, while benzene rings and halogens are considered to be possible negatively correlated factors. These findings enable researchers to select appropriate substructures of salicylaldehyde derivatives.
The Bayesian optimization method samples the force measurements on the selected crystals and achieves a blocking force of up to 37.0 mN, assuming that this value represents the global maximum force in the parameter space.The exploration efficiency of this study is at least 73 times that of the traditional grid search method.
The revolutionary potential of Bayesian optimization in materials science
The Bayesian optimization used in this study converged to a specific combination of crystal properties and size, indicating that there is an optimal balance between crystal properties and size in maximizing blocking force, and simply choosing the largest crystal size is not the optimal solution to maximize blocking force. This method sets a new benchmark for light-driven crystal force output and also provides a general framework for material optimization of other molecular crystal systems.
In addition to the above research, Bayesian optimization has also demonstrated revolutionary potential in many fields of materials science due to its efficient data utilization capabilities, global optimization characteristics and adaptability to complex constraints.
for example,The University of Toronto team used a multi-objective Bayesian optimization (MBO) algorithm combined with highly sp²-bonded nanoscale pyrolytic carbon to create lightweight carbon nanolattices with ultra-high specific strength and scalability.The research was titled “Ultrahigh Specific Strength by Bayesian Optimization of Carbon Nanolattices” and published in Advanced Materials.
* Paper address:
https://advanced.onlinelibrary.wiley.com/doi/10.1002/adma.202410651
In addition, the Swedish team successfully explored an organic aqueous mixed electrolyte system containing four co-solvents and two lithium conductive salts by integrating automated button cell assembly, LiFePO4||Li4Ti5O12 and Bayesian optimization, demonstrating the potential of combining Bayesian optimization with autonomous full-cell experiments and providing new electrolyte design insights for the next generation of aqueous batteries. The study was published in Cell Press under the title "Accelerating aqueous electrolyte design with automated full-cell battery experimentation and Bayesian optimization".
Virginia Tech has developed a Bayesian reaction optimization and analysis framework that enables polymer chemists to integrate advanced optimization algorithms into the screening and optimization of polymer stereoselective catalysts, marking a major breakthrough in Bayesian optimization in polymer chemical synthesis. The study, titled "Bayesian-optimization-assisted discovery of stereoselective aluminum complexes for ring-opening polymerization of racemic lactide," was published in Nature Communications.
* Paper address:
https://www.nature.com/articles/s41467-023-39405-5
In summary, by using Bayesian optimization, or combining Bayesian optimization with other methods, material research and development is expected to break through the efficiency bottleneck of traditional methods and achieve efficient exploration and precise design at multiple scales and multiple objectives.