EasyControl, a Tool for Generating Ghibli-style Paintings, Is Launched With One Click; a Single Image Can Be Transformed Into a 3D Model in Seconds, TripoSG Revolutionizes 3D Asset Generation
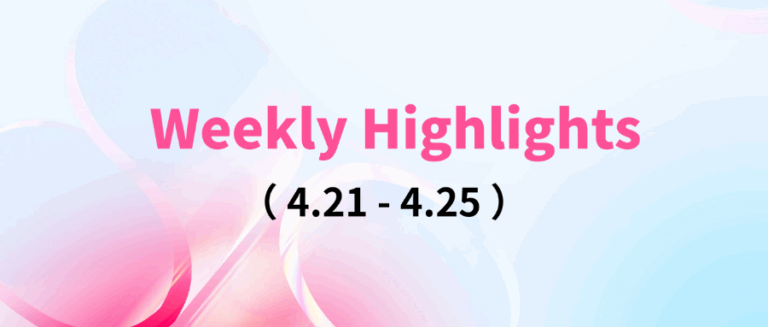
Recently, Ghibli-style images have swept social media. When people add Hayao Miyazaki's animation-style filters to movies or TV series, they seem to be brought into a fairy tale world full of fantasy. However, for many users who want to create Ghibli-style pictures by themselves, the existing generation models often have problems such as complex operation, high threshold for use, or unsatisfactory generation effects.
EasyControl greatly reduces the threshold for creation with its advanced technology and simple and easy-to-use interface.It introduces lightweight conditional injection LoRA Module, location-aware training paradigm, andCausal Attention Mechanismand KV Cache Technology, significantly improving model compatibility, supporting plug-and-play functionality and non-destructive style control.
at present,HyperAIThe "EasyControl Ghibli-style image generation Demo" tutorial has been launched.Demo uses the stylized Img2Img control model to convert a portrait intoMiyazaki styleArtworks, come and try it~
Online use:https://go.hyper.ai/jWm9j
From April 21st to April 25th, hyper.ai official website updates:
* High-quality public datasets: 10
* High-quality tutorials: 12
* Community article selection: 4 articles
* Popular encyclopedia entries: 5
* Top conferences with deadline in April: 1
Visit the official website:hyper.ai
Selected public datasets
1. Spanish Traffic Aerial Image Dataset
The dataset contains 15,070 frames of images taken by unmanned aerial vehicles (UAVs) covering a variety of traffic scenarios, including regional roads, urban intersections, rural roads, and different types of roundabouts. 155,328 objects are annotated in the images, of which 137,602 are cars and 17,726 are motorcycles. YOLO formatStorage is convenient for training machine vision algorithms based on convolutional neural networks.
Direct use:https://go.hyper.ai/VJoXE
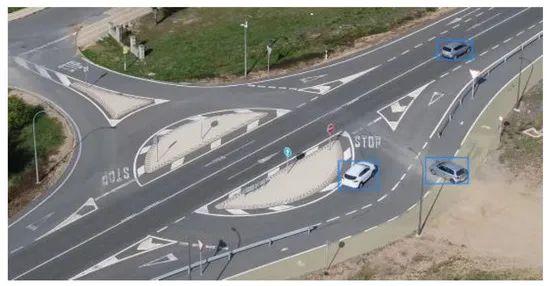
2. CAMUS Cardiac Ultrasound Image Dataset
The dataset contains two-dimensional apical four-chamber and two-chamber view sequences obtained from 500 patients. The data was collected at the University Hospital of Saint-Etienne in France and has been completely anonymized to ensure patient privacy and data compliance. Each image has been accurately annotated by professional medical personnel, covering the contour information of the left ventricular endocardium, left ventricular epicardium, and left atrium. These detailed annotations provide researchers with rich training and verification resources.
Direct use:https://go.hyper.ai/iYtn2
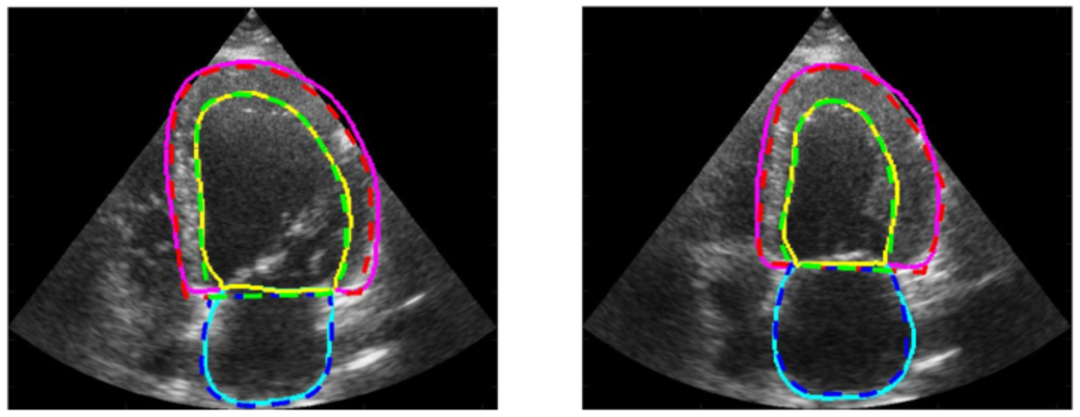
3. Ships/Vessels in Aerial Images
The dataset contains 26.9k images with only the image category "ship" that are carefully annotated specifically for ship detection. The bounding box annotations are presented in YOLO format, which can accurately and efficiently detect ships in images.
Direct use:https://go.hyper.ai/s03Tk
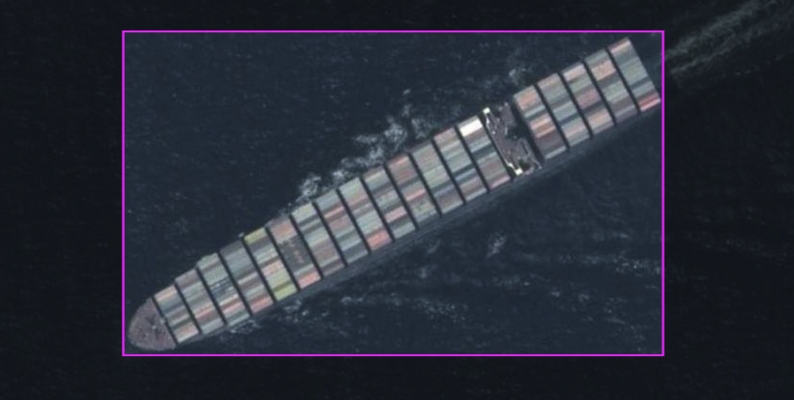
4. SkyCity Aerial City Landscape City Landscape Aerial Photography Dataset
This dataset is a curated dataset for aerial landscape classification, with a total of 8k images, 10 different categories, each category contains 800 high-quality images, and a resolution of 256 × 256 pixels. This dataset brings together urban landscapes from the public AID and NWPU-Resisc45 datasets, aiming to facilitate urban landscape analysis.
Direct use:https://go.hyper.ai/eCRdN
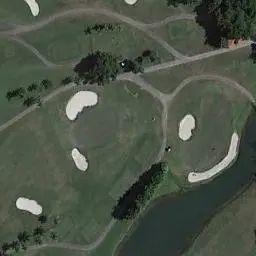
5. 302 rare disease case data sets
The dataset contains 302 rare diseases, with 1 to 9 rare diseases randomly selected from each category. These rare diseases were selected from 7k+ rare diseases of 33 types in the Orphanet database, a comprehensive rare disease database co-funded by the European Commission.
Direct use:https://go.hyper.ai/LwqME
6. DRfold2 RNA structure test dataset
This dataset is an independent test dataset built to objectively evaluate the performance of DRfold2 in research. It contains 28 RNA structures, all of which are less than 400 nts in length and come from the following 3 categories: the latest RNA-Puzzles target sequences; RNA target sequences in the CASP15 competition; and the latest RNA structures published in the Protein Data Bank (PDB) database as of August 1, 2024.
Direct use:https://go.hyper.ai/shkp6
7. HMC-QU Cardiac Medical Image Dataset
This dataset contains two-dimensional echocardiographic (echo) recordings of the apical four-chamber (A4C) and apical two-chamber (A2C) views acquired during 2018 and 2019. These recordings were acquired with equipment from different manufacturers (such as Phillips and GE Vivid ultrasound machines) with a temporal resolution of 25 frames/s and spatial resolutions ranging from 422×636 to 768×1024 pixels.
Direct use:https://go.hyper.ai/gQN8a
8. Reasoning-v1-20m reasoning dataset
Reasoning-v1-20m contains about 20 million reasoning traces, covering complex problems in multiple fields such as mathematics, programming, science, etc. This dataset aims to help the model learn complex reasoning logic and improve its performance in multi-step reasoning tasks by providing rich examples of the reasoning process.
Direct use:https://go.hyper.ai/c2RqP
9. II-Thought-RL-v0 Multi-Task Question Answering Dataset
II-Thought-RL-v0 is a large-scale, multi-task dataset designed for reinforcement learning and problem answering. It contains high-quality question-answer pairs that have been strictly filtered in multiple steps, covering multiple fields such as mathematics, programming, and science. The question pairs in the dataset are not only from public datasets, but also include customized high-quality question pairs to ensure the diversity and practicality of the data.
Direct use:https://go.hyper.ai/9eSSq
10. AM-DeepSeek-R1-Distilled-1.4M Large-Scale General Reasoning Task Dataset
The dataset contains about 1.4 million data entries, covering a variety of types of questions such as mathematics, code, scientific Q&A, and general chat. The data has been carefully selected, semantically deduplicated, and strictly cleaned to ensure the high quality and challenge of the data. Each entry in the dataset contains rich thinking traces, which not only provide examples of the reasoning process for the model, but also help the model better understand and generate complex reasoning task solutions.
Direct use:https://go.hyper.ai/2PSxR
Selected Public Tutorials
1. YOLOE: See everything in real time
YOLOE is a new real-time visual model proposed by a research team from Tsinghua University in 2025, which aims to achieve the goal of "seeing everything in real time". It inherits the real-time and efficient characteristics of the YOLO series of models, and on this basis deeply integrates zero-sample learning and multimodal prompting capabilities, and can support target detection and segmentation in multiple scenarios such as text, vision and silent prompting.
The relevant models and dependencies of this project have been deployed. After starting the container, click the API address to enter the Web interface.
Run online:https://go.hyper.ai/rOIS1
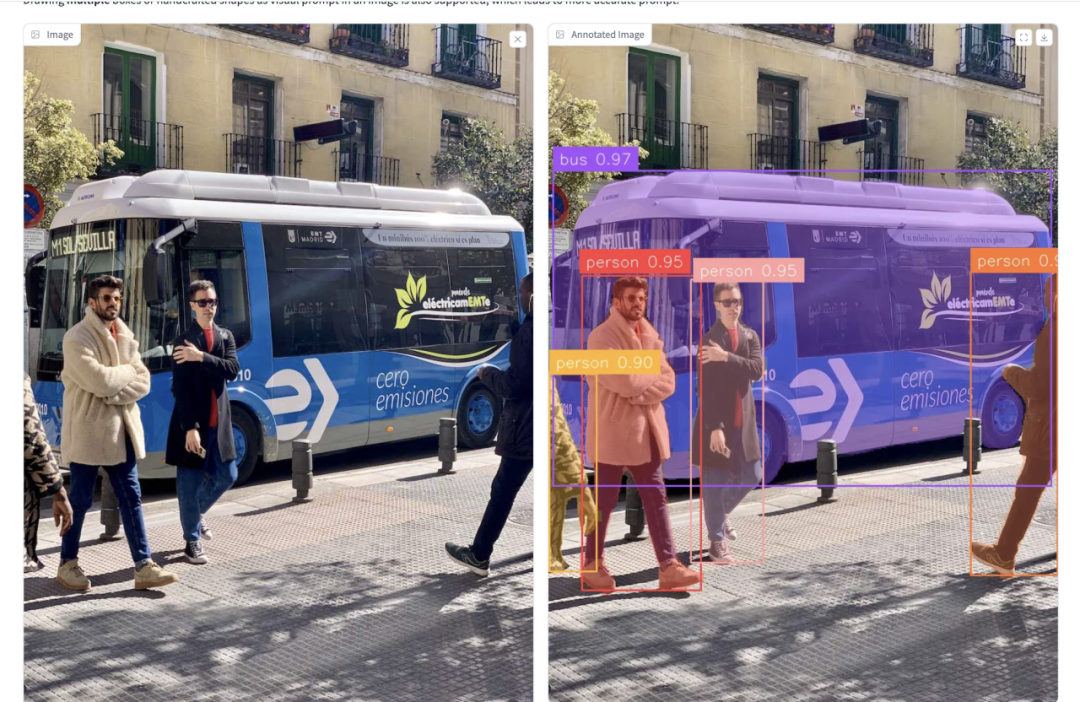
2. One-click deployment of R1-OneVision
R1-OneVision is a multimodal reasoning model released by the Zhejiang University team. The model is fine-tuned on the R1-Onevision dataset based on Qwen2.5-VL. It is good at handling complex visual reasoning tasks, seamlessly integrating visual and textual data, and performs well in mathematics, science, deep image understanding, and logical reasoning. It can be used as a powerful AI assistant to solve various problems.
This tutorial uses R1-Onevision-7B as a demonstration, and the computing resource uses RTX 4090. After starting the container, click the API address to enter the web interface.
Run online:https://go.hyper.ai/7I2pi
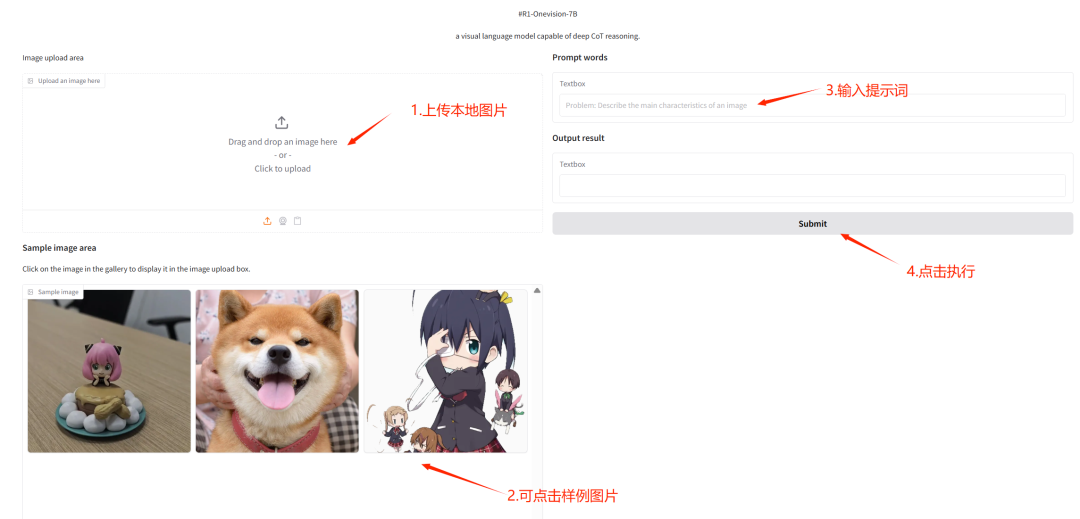
3. UNO: Universal Customized Image Generation
The UNO project can support both single-subject and multi-subject image generation, unifying multiple tasks with one model and demonstrating strong generalization capabilities.
This project is based on FLUX.1-dev-fp 8 and can quickly recognize text and generate images based on text descriptions.
Run online:https://go.hyper.ai/r8JZo
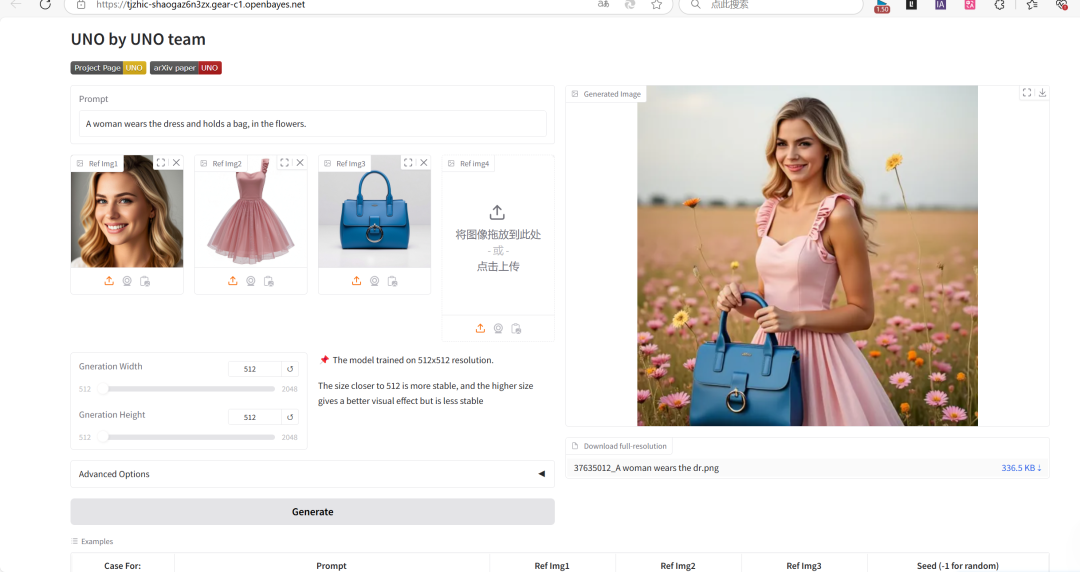
4. TripoSG: Transform a single image into high-fidelity 3D in seconds
TripoSG is an advanced high-fidelity, high-quality, and high-generalizability image-to-3D generative base model. It leverages large-scale rectified transformers, hybrid supervised training, and high-quality datasets to achieve state-of-the-art performance in 3D shape generation.
The relevant models and dependencies of this project have been deployed. After starting the container, click the API address to enter the Web interface.
Run online:https://go.hyper.ai/rcWwu
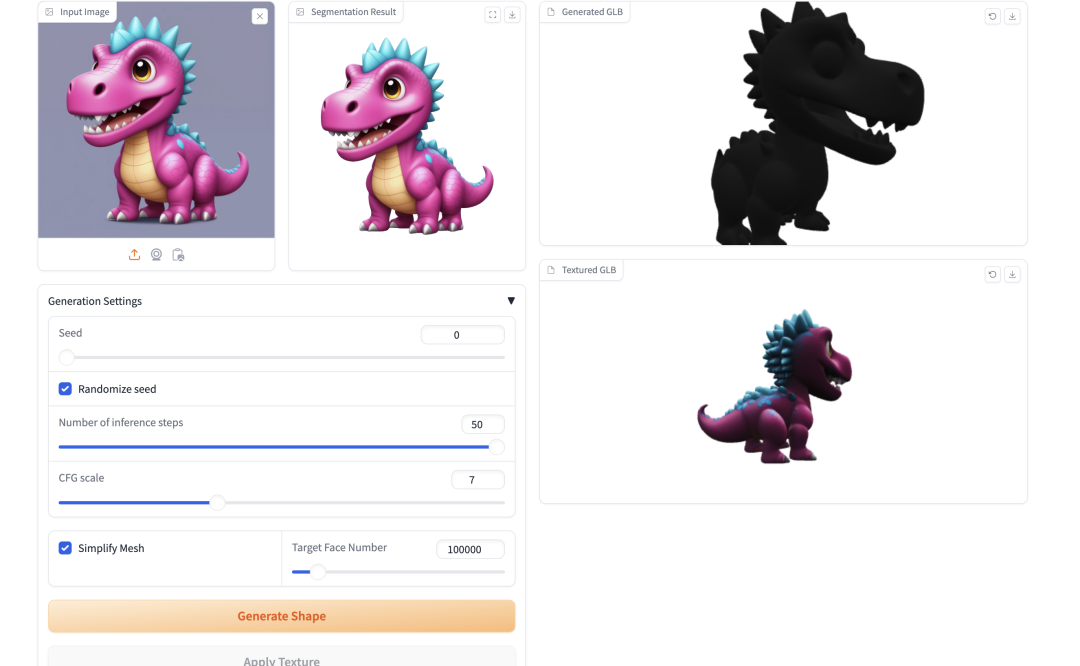
5. InfiniteYou High-Fidelity Image Generation Demo
InfiniteYou, or InfU for short, is an identity-preserving image generation framework based on Diffusion Transformers (such as FLUX) launched by ByteDance's intelligent creation team in 2025. Through advanced technology, it can maintain the consistency of the person's identity while generating images, solving the shortcomings of existing methods in identity similarity, text-image alignment, and generation quality.
This tutorial uses InfiniteYou-FLUX v1.0 as a demonstration, and the computing resources use A6000. Click the link below to quickly clone the model.
Run online:https://go.hyper.ai/K5Yl5
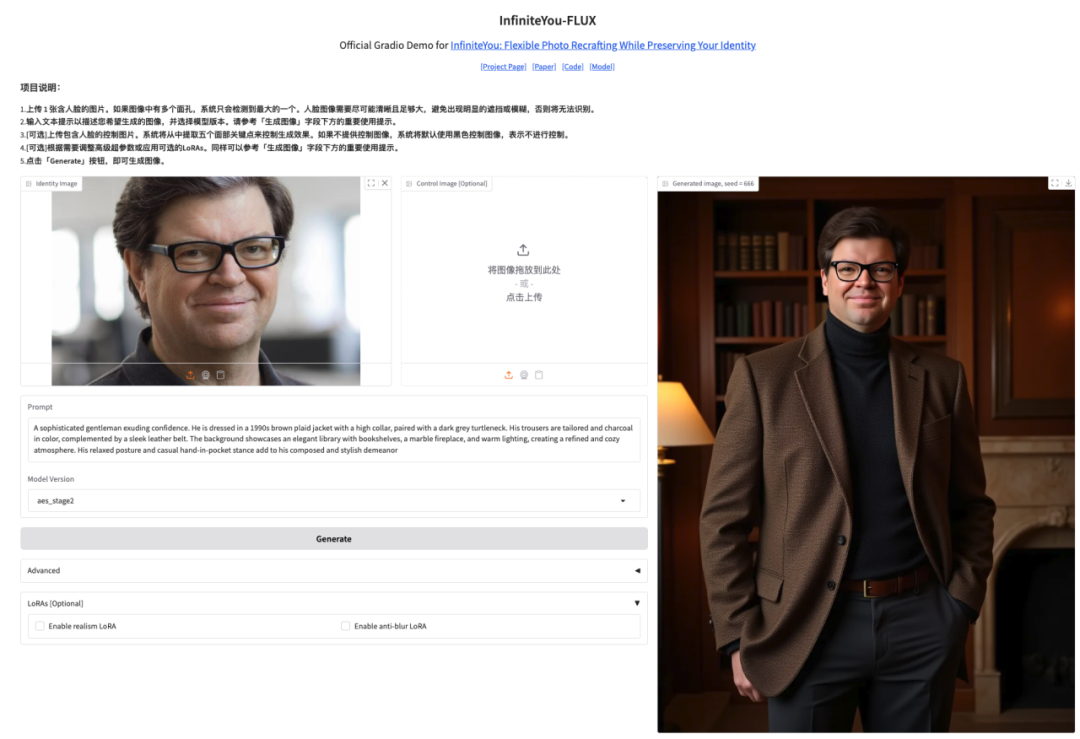
6. One-click deployment of DeepCoder-14B-Preview
The DeepCoder-14B-Preview project is a 14B coding model released by AGENTICA on April 8, 2025. The model is fine-tuned from DeepSeek-R1-Distilled-Qwen-14B LLM for code reasoning, using distributed reinforcement learning (RL) to scale to long context lengths. The model achieved a Pass@1 accuracy of 60.6% on LiveCodeBench v5 (8/1/24-2/1/25), an improvement of 8% over the base model (53%), and achieved similar performance to OpenAI's o3-mini with only 14B parameters.
This tutorial uses the DeepCoder-14B-Preview model as a demonstration case and adopts the 8-bit quantization method provided by bitsandbytes to optimize video memory usage.
Run online:https://go.hyper.ai/17aD2
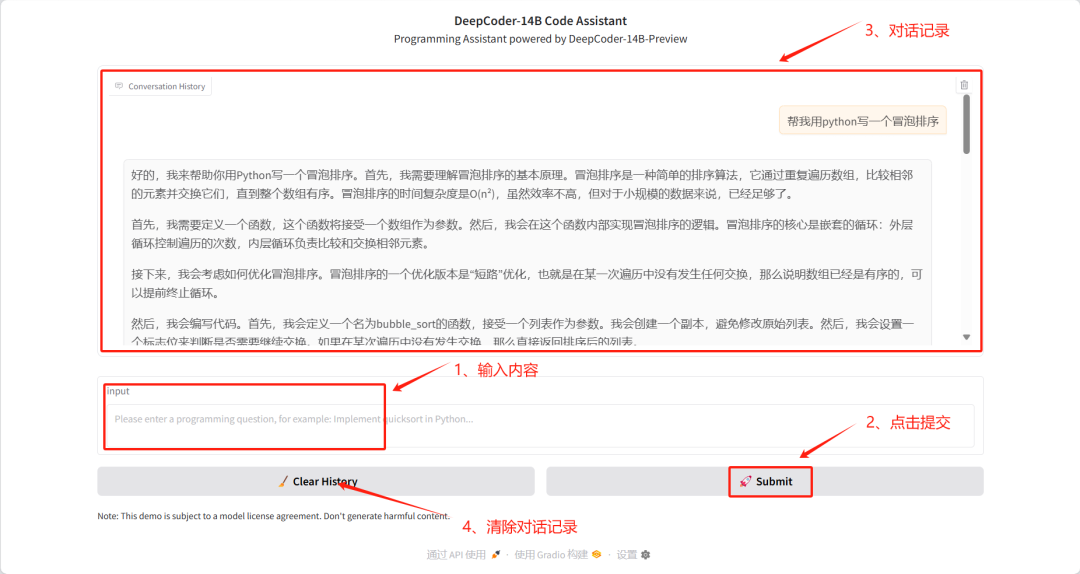
7. EasyControl Ghibli style image generation demo
EasyControl is a project that aims to add efficient and flexible control to the Diffusion Transformer. The project significantly improves model compatibility by introducing lightweight conditional injection LoRA modules, location-aware training paradigms, and combining causal attention mechanisms and KV cache technology, supporting plug-and-play functionality and lossless style control.
This tutorial uses the Stylized Img2Img Controls model, which is able to transform a portrait into a Hayao Miyazaki-style artwork, while preserving facial features and applying the iconic anime aesthetic.
Run online:https://go.hyper.ai/jWm9j
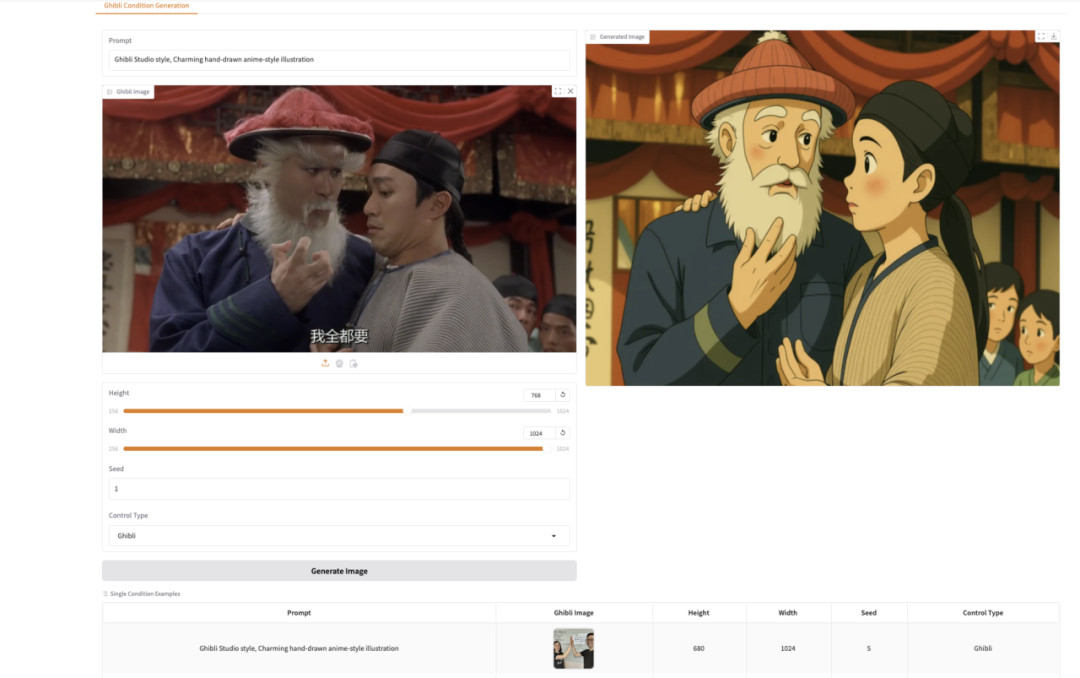
8. Qwen2.5-0mni: Full-mode support for reading, listening, and writing
Qwen2.5-Omni is the latest end-to-end multimodal flagship model released by Alibaba's Tongyi Qianwen team. It is designed for comprehensive multimodal perception and seamlessly processes various inputs including text, images, audio, and video, while supporting streaming text generation and natural speech synthesis output.
This tutorial uses Qwen2.5-Omni as a demonstration, and the computing resources are A6000.
Run online:https://go.hyper.ai/eghWg
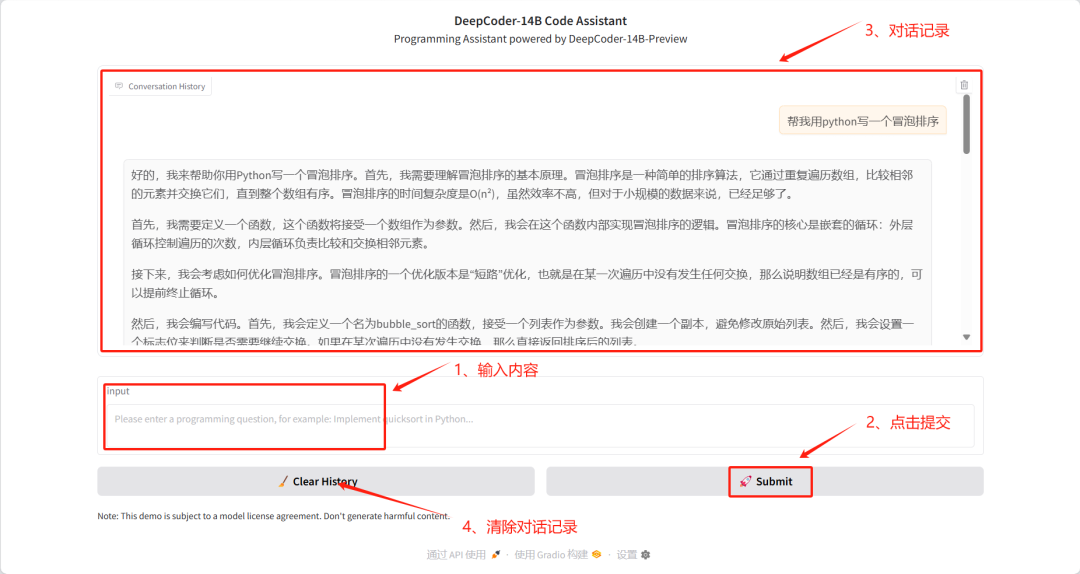
9. One-click deployment of Qwen2.5-VL-32B-Instruct
Qwen2.5-VL-32B-Instruct is an open-source multimodal large model developed by the Alibaba Tongyi Qianwen team. Based on the Qwen2.5-VL series, this model is optimized through reinforcement learning technology and achieves a breakthrough in multimodal capabilities with a 32B parameter scale.
This tutorial uses Qwen2.5-VL-32B as a demonstration, and the computing resources use A6000*2. Functions include text understanding, image understanding, and video understanding.
Run online:https://go.hyper.ai/Dp2Pd
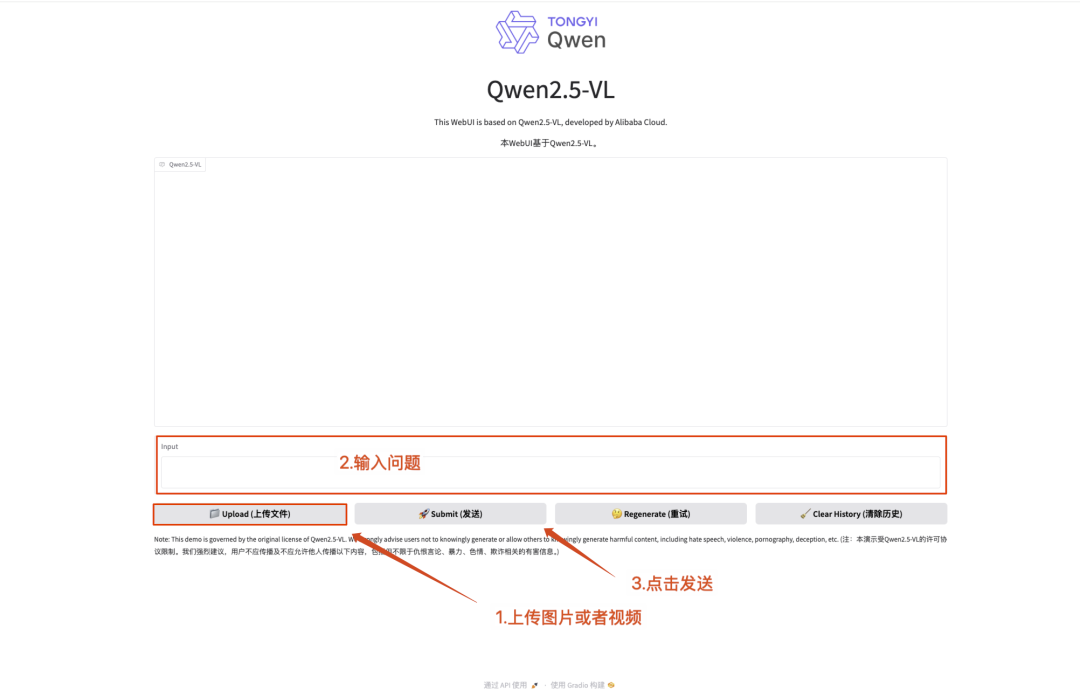
10. One-click deployment Qwen2.5-VL-32B-Instruct-AWQ
Qwen2.5-VL-32B-Instruct-AWQ is a quantized version of Qwen2.5-VL-32B-Instruct, which significantly enhances programming and mathematical computing capabilities. The model supports 29 language interactions, can process 128K tokens long text, and has core functions such as structured data understanding and JSON generation. Developed based on the transformers architecture, it achieves efficient deployment through quantization technology and is suitable for large-scale AI application scenarios.
The relevant models and dependencies of this project have been deployed. After starting the container, click the API address to enter the Web interface.
Run online:https://go.hyper.ai/fAYEK
Since its release, DeepCoder-14B-Preview has received over 3k stars on GitHub for its outstanding performance in code comprehension and reasoning tasks. The model has demonstrated capabilities comparable to o3-mini in multiple evaluations, and has efficient reasoning performance and good scalability.
To help developers quickly experience and deploy the model, the tutorial section of HyperAl's official website has launched the "One-click Deployment of DeepCoder-14B-Preview" tutorial. Click the link below to quickly start.
Run online:https://go.hyper.ai/V42RT
To promote the application and development of AI protein engineering, the research group of Professor Hong Liang from Shanghai Jiao Tong University has developed a one-stop open platform, VenusFactory, tailored for protein engineering. Researchers can easily call more than 40 cutting-edge protein deep learning models without writing complex codes.
Currently, the HyperAI official website has launched a one-click deployment tutorial for the "VenusFactory Protein Engineering Design Platform". Click clone to start it with one click.
Run online:https://go.hyper.ai/TnskV
Community Articles
The Shanghai Artificial Intelligence Laboratory and several universities proposed MaMI Model, innovatively introduced a continuous modality parameter adapter, breaking the limitations of the traditional single modality, allowing the unified model to adapt to multiple input modalities such as X-ray and CT in real time. After evaluation on 11 public medical imaging datasets, MaMI demonstrated the most advanced re-identification performance, providing strong support for accurate and dynamic historical image retrieval in personalized medicine. This article is a detailed interpretation and sharing of the research.
View the full report:https://go.hyper.ai/e8Eat
Phaseshift Technologies, a Canadian advanced materials company, is committed to developing the next generation of alloys and composite materials using AI technology and multi-scale simulation. Its Rapid Alloy Design (RAD™) platform can develop customized alloys for specific needs and scenarios for various industries, increasing the material development speed to 100 times that of traditional methods while reducing costs. This article is a detailed report on the company.
View the full report:https://go.hyper.ai/da4VH
Professor Luo Xiaozhou of the Shenzhen Institute of Advanced Technology of the Chinese Academy of Sciences gave an in-depth presentation on the topic of "Artificial Intelligence-driven Enzyme Engineering" at the "Future is Here" AI Protein Design Summit hosted by Shanghai Jiao Tong University. From multiple perspectives such as the UniKP framework and ProEnsemble machines, he explained the innovative application of AI in the field of enzyme engineering and its biomanufacturing practice. This article is a transcript of Professor Luo Xiaozhou's sharing.
View the full report:https://go.hyper.ai/de1KW
Teams from top universities such as MIT/UC Berkeley/Harvard/Stanford jointly proposed the innovative algorithm DRAKES. By introducing a reinforcement learning framework, they realized the differentiable reward backpropagation of the complete generated trajectory in a discrete diffusion model for the first time, while maintaining the naturalness of the sequence and significantly improving the performance of downstream tasks. This article is a detailed interpretation and sharing of the research paper.
View the full report:https://go.hyper.ai/YyEof
Popular Encyclopedia Articles
1. DALL-E
2. Reciprocal sorting fusion RRF
3. Pareto Front
4. Large-scale Multi-task Language Understanding (MMLU)
5. Contrastive Learning
Here are hundreds of AI-related terms compiled to help you understand "artificial intelligence" here: