To Maximize the Value of Clinical MRI Data, the UCL Team Proposed the MindGlide Model to Achieve Quantification of Multiple Sclerosis Lesions
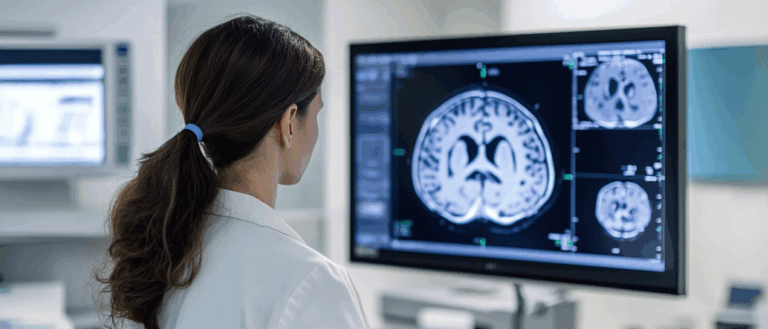
Multiple sclerosis (MS) is a chronic disabling disease characterized by inflammatory demyelinating lesions in the white matter of the central nervous system. It mostly targets young and middle-aged people, so it is also called the "invisible killer of young people." According to relevant statistics, more than 2.8 million people are affected worldwide. In Asia, about 1-5 people out of every 100,000 people suffer from MS, and the disease has become the second largest cause of disability among young and middle-aged people in China after trauma. In 2018, China included the disease in the "First List of Rare Diseases."
Brain magnetic resonance imaging (MRI) markers are crucial in the clinical treatment and research of MS. For example, multiple contrast MRI scans can capture the evolution of the disease. However, multiple contrasts require different types of specialized scanning equipment, which is time-consuming, labor-intensive and expensive, and many hospitals do not have these conditions. Therefore, how to simplify MRI analysis becomes the key to solving the problem, especially through single contrast brain volume calculation, which can reduce the need for multiple contrast acquisition and reduce the cost of clinical trials.
Based on this, a research team from University College London developed a tool called MindGlide.Key information can be extracted from MRI scans obtained during the care of MS patients.MindGlide demonstrates that conventional MRI scans lack ideal imaging contrast.There is still potential for discovering new lesions and subtle brain tissue damage.It is also expected to further help medical staff improve their ability to interpret and evaluate the treatment effects of MS patients.
The related research was published in Nature Communications under the title "Enabling new insights from old scans by repurposing clinical MRI archives for multiple sclerosis research".
Research highlights:
*Enables extraction of multiple clinically relevant MRI biomarkers from a single MRI contrast, and MindGlide outperforms the state-of-the-art in several key areas
* Clinically meaningful tissue segmentation and lesion quantification can be achieved even with limited MRI data and single contrasts not typically used for these tasks
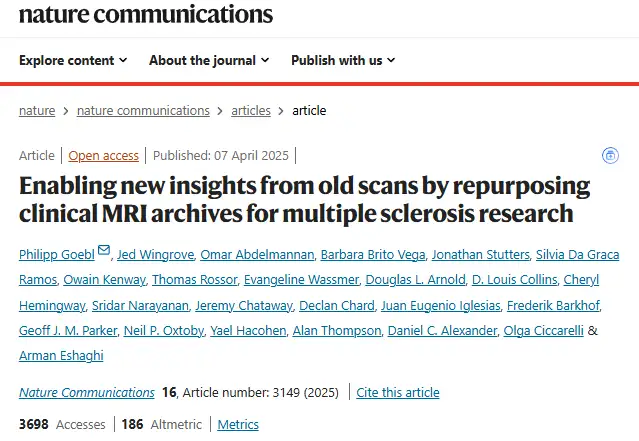
Paper address:
https://go.hyper.ai/fDEgm
The open source project "awesome-ai4s" brings together more than 100 AI4S paper interpretations and also provides massive data sets and tools:
https://github.com/hyperai/awesome-ai4s
Dataset: All data are collected from MS patients
In order to verify the reliability and validity of the model, the study used different training data sets and external validation data sets, and the data were entirely from MS patients.The study was supported by the Montreal Neurological Institute's International Progressive MS Alliance database.Relevant training data were extracted, mainly from the MS-stat trial, the ORATORIO trial, as well as routine care datasets of pediatric relapsing-remitting MS patients obtained from three hospitals in the UK and two open source lesion segmentation datasets (MS-30 and ISBI).
*MindGlide processes MRIs, including 2D and 3D scans, with MRI contrast and related tissue intensities (T1-weighted, TI-weighted, Proton Density (PD), and T2-Fluid Attenuated Inversion Recovery (FLAIR)) commonly used in hospital archives.
During the model training phase,The researchers trained MindGlide using a dataset of 8,550 real and synthetic images.Among them, a total of 4,247 real MRI scan images (including 2,092 TI-weighted and 2,155 FLAIR) were collected from 2,871 patients (including 1,082 relapsing-remitting MS patients (RRMS), 1,453 secondary progressive MS patients (SPMS) and 336 primary progressive MS patients (PPMS)).The scans were acquired using 592 professional MRI scanners at 1.5 and 3 Tesla magnetic fields.The researchers then generated an additional 4,303 synthetic scans to expand the training.
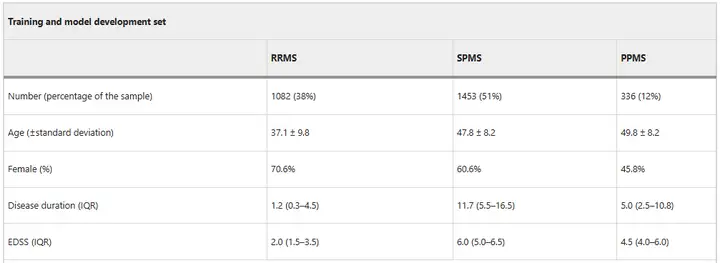
During the model validation phase, the researchers used an external validation set.The MindGlide was tested for universal use in an age range of 14-64 years, including two progressive MS clinical trials and a real-life group of pediatric relapsing-remitting MS patients.The external validation dataset included 1,001 patients (699 in the PPMS trial, 141 in the SPMS trial, and 161 in the RRMS trial) obtained from 186 MRI scanners.
in,The PPMS dataset includes 11,015 MRI scans(2,756 TI-weighted, 2,754 T2-weighted, 2,749 FLAIR, 2,756 PD), all slices were 3 mm thick (1 mm x 1 mm x 3 mm);The SPMS dataset includes 763 scans(378 TI-weighted, 385 T2-weighted), with different slice thicknesses (1mm x 1mm x 1mm for T1-weighted; 3mm x 1mm x 1mm for T2-weighted);The Pediatric RRMS Dataset includes 1,478 scans(523 T1-weighted, 475 T2-weighted, 480 FLAIR), including different slice thicknesses.
Model architecture: an efficient 3D convolutional neural network
MindGlide is developed based on "nnU-Net", a 3D convolutional neural network (CNN) based on the U-Net architecture.It has the ability to automatically self-configure, thus bypassing the expensive hyperparameter tuning process. Through training, MindGlide can simultaneously segment brain gray matter, white matter areas, and MS lesions, thereby adapting to real MRI changes and solving artifact problems in traditional image processing software. The overall architecture and strategy are shown in the figure below.
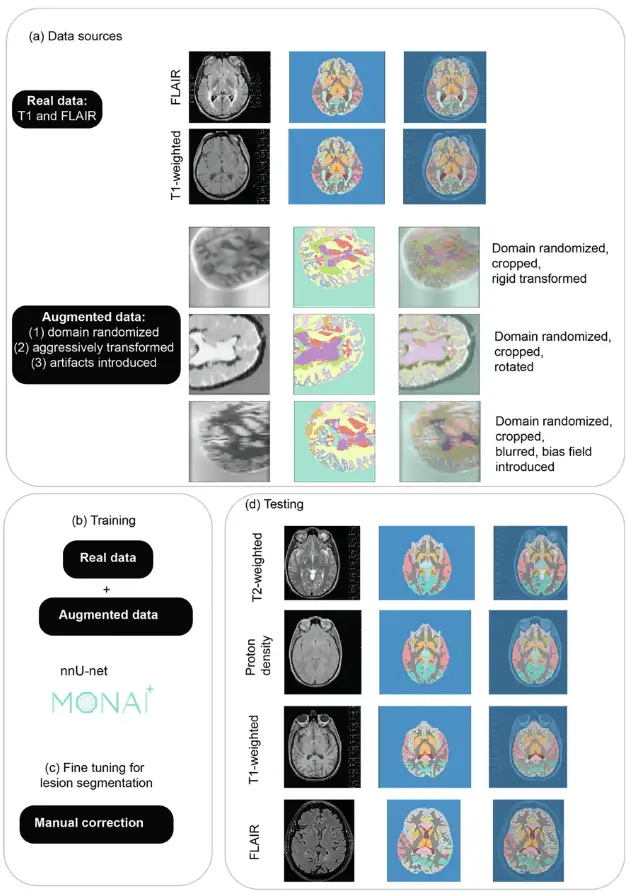
For image preprocessing for model training, the researchers used a minimal preprocessing pipeline that first normalized the image resolution to 1 mm isotropic voxels and then designed according to nnU-Net. The researchers then extracted 128 x 128 x 64 voxel patches using a sliding window technique to optimize memory and computational efficiency during training.
Data augmentation mainly involves artificially expanding the diversity of training data through random modifications, enhancing the generalizability of the model, and reducing overfitting.The researchers used two techniques to expand its adaptability: distorting the geometry and image intensity of real scans and generating synthetic scans.As shown in Fig. (a), domain randomization is used to generate intensity variations and prepare models for different MRI contrasts.
The synthetic data was generated using SynthSeg 2.0, and the researchers used MONAI 1.2.0 for enhancement during training, and then generated synthetic scans of different contrasts directly from the labels of the training dataset. Figure a shows an example of generated synthetic data, and Figure b shows training MindGlide with real data and augmented data.
In related reports, the media used intuitive data to demonstrate the capabilities of this model. It mentioned that,Complex scans that previously took neuroradiologists weeks to interpret manually now take only 5 to 10 seconds per image with MindGlide.This allows new value to be gained from images that were previously unanalyzable and from routine MRI scans, providing a more reliable basis for testing the effects of different treatments on disease progression in clinical trials and routine care.
Experimental results: Multi-dimensional comparison shows the superiority of MindGlide
To evaluate the verification effect of MindGlide, the researchers compared it with the existing state-of-the-art technologies, namely WMH-SynthSeg and SAMSEG.The former can simultaneously segment white matter high signals and brain anatomical structures from scans of different resolutions and contrasts, while the latter can robustly segment lesions and brain structures under different MRI contrasts.
First, the experiment compared the consistency of the three with human experts’ labeled lesion segmentation and disability, as shown in the figure below. The agreement between MindGlide and manually labeled real lesions was higher.The median Dice score was 0.606, SAMSEG was 0.504, and WMH-Synthseg was 0.385.
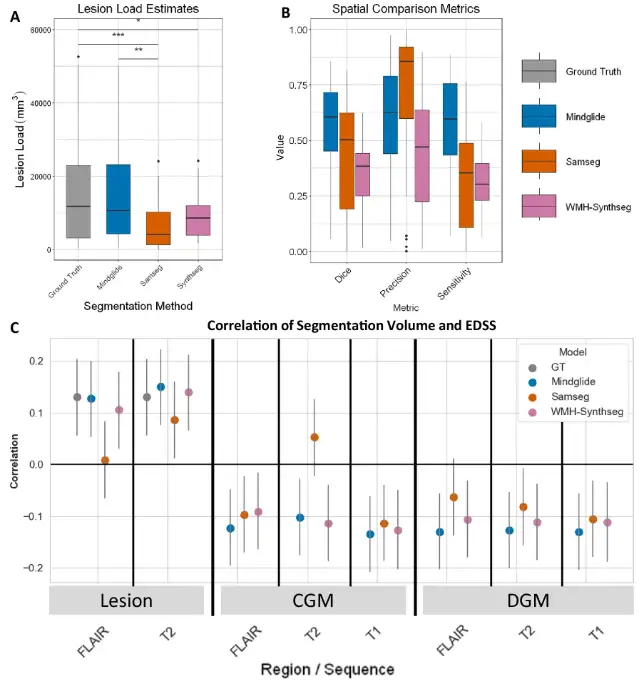
in addition,In the PPMS dataset, the correlation between the lesion load derived by MindGlide and the numerical value of the Expanded Disability Status Scale (EDSS) is also higher than that of existing technologies.
Subsequently, the researchers conducted a longitudinal validation of the lesion treatment effect involving multiple experiments, which demonstrated the effectiveness of MindGlide in detecting treatment effects. For example, in the SPMS and PPMS trials, the cumulative lesion volume in the treatment group was lower than that in the placebo group; in the pediatric cohort, MindGlide successfully detected different changes in lesion volume in different treatment groups, as shown in the figure below.
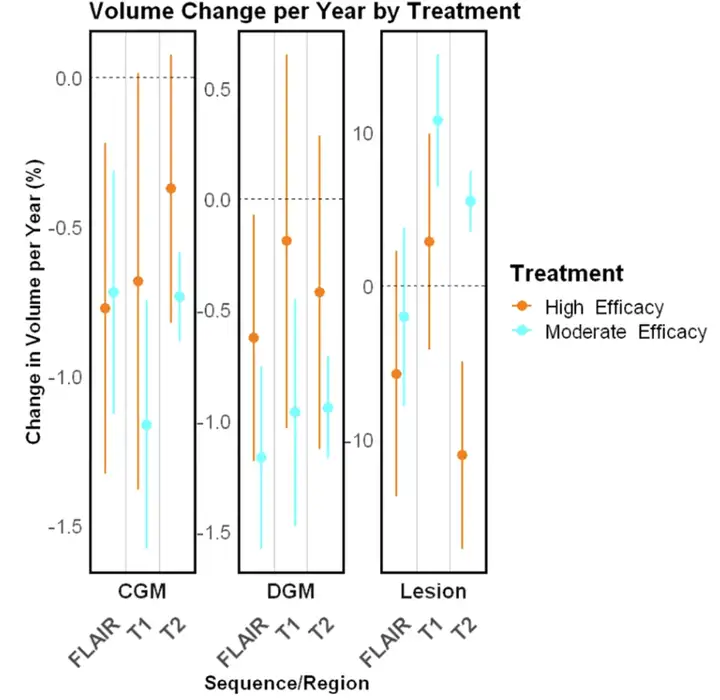
In testing the effects of treatment on brain tissue loss,MindGlide showed that the rate of cortical GM volume loss in the treatment group was significantly lower than that in the placebo group.The following figure shows various contrasting segmentation examples.
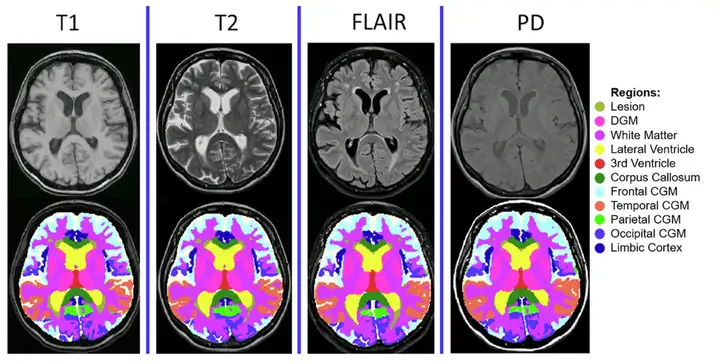
Following this, the researchers went on to compare the treatment effects of MindGlide with other segmentation tools and real lesions in a PPMS clinical trial.The experimental results show that MindGlide is very close to the ground truth. In contrast, SAMSEG overestimates the treatment effect, while WHM-Synthseg underestimates the treatment effect, indicating that MindGlide has a higher accuracy in lesion volume estimation.
The figure below shows the three assessments of regional brain volume.Results showed that MindGlide measures showed better treatment effects between treatment groups compared with measures obtained from longitudinal SAMSEG or WHM-Synthseg.
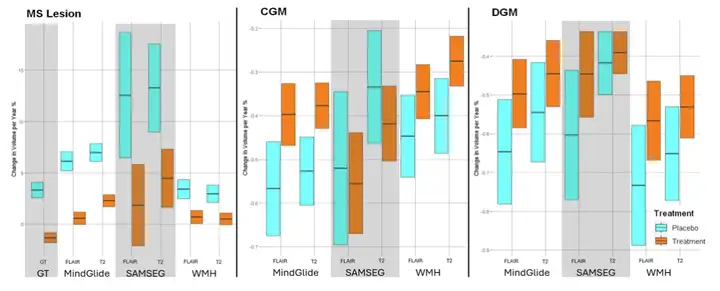
Additionally, in a routine care clinical dataset, the researchers visually inspected WMH-Synthseg and MindGlide and found that the former showed a significant failure rate, especially when the scan thickness exceeded 5 mm.Of the 433 contrasts evaluated visually, WMH-Synthseg failed to segment 65 scans, while MindGlide failed in only 6.The example is shown below.
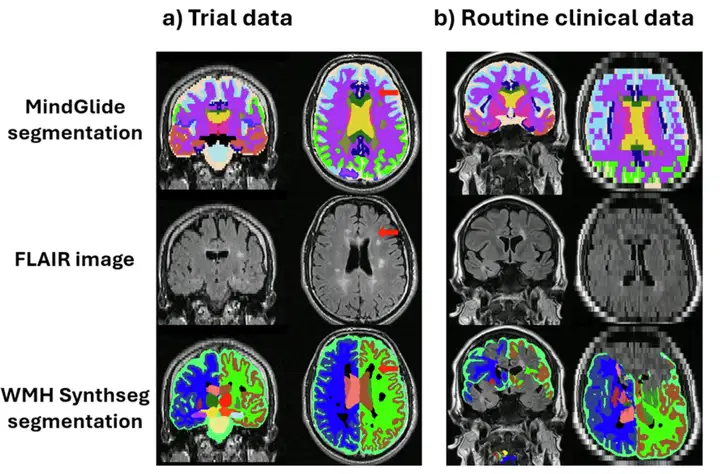
Figure (b) shows a 7mm slice from the Routine Care Clinical Dataset (RRMS) where WMH-Synthseg fails to segment. This is because most segmentation tools are designed to use superior or inferior acquisition orientations, while MindGlide allows segmentation of images acquired in any orientation.
all in all,MindGlide is superior to existing methods in many aspects and can extract multiple biomarkers from a single MRI contrast, which can effectively monitor the treatment effect and is suitable for a variety of clinical scenarios.Provide strong support for MS research and clinical decision-making.
AI has become an important tool in MS research
In recent years, the rapid development of artificial intelligence has accelerated its application in neurological diseases, and due to its unique working mechanism and efficient methods, it has provided new ideas for the medical community to conduct MS research through artificial intelligence. Coincidentally, many laboratories are trying to use artificial intelligence to overcome MS, a major problem that endangers human health.
For example, Professor Fang Liu's research group from the University of Toronto published an article in Science Advances titled "Small-molecule targeting AMPA-mediated excitotoxicity has therapeutic effects in mouse models for multiple sclerosis". This study overcame the traditional theoretical viewpoints and developed a breakthrough small molecule compound.It works by targeting a novel mechanism of regulation of AMPA receptor-mediated excitotoxicity.This study used artificial intelligence to virtually screen small molecules targeting the GluA 2 subunit of the AMPA receptor, which not only filled the gap in the international development of such drugs, but more importantly, provided a completely new idea for the treatment of MS.
Paper address:
https://www.science.org/doi/10.1126/sciadv.adj6187
In addition, a study published in NeuroImage also proposed a new open source tool for simultaneously segmenting white matter lesions and 41 neuroanatomical structures in MRI scans of MS patients.This method is based on the generative model SAMSEG mentioned above and is able to adapt to different scanning and imaging protocols, and can assess atrophy without pre-segmentation of lesions.This method overcomes some of the limitations of existing tools. The related article was published under the title “A contrast-adaptive method for simultaneous whole-brain and lesion segmentation in multiple sclerosis”.
Paper address:
https://www.sciencedirect.com/science/article/pii/S1053811920309563?via%3Dihub
All these scientific and technological achievements and cutting-edge discoveries reveal the value of artificial intelligence in MS research. Although there is still a long way to go, it also gives people hope. As Dr. Philipp Goebl, the first author of the above article, hopes, using artificial intelligence to conduct clinical trials to solve patients' conditions may become a reality in the next 5 to 10 years.