Output Results Within 30 Minutes. National University of Singapore/MIT and Others Build a Microbial Contamination Detection Model Based on SVM
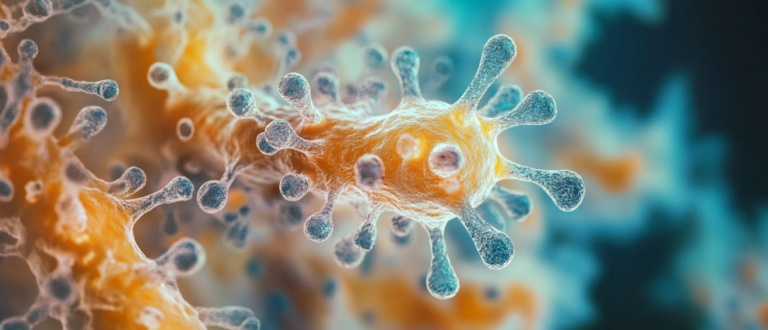
As an important part of advanced therapeutic drugs (ATMPs), cell therapy products (CTPs) are bringing hope to patients with rare diseases and difficult and complicated diseases. However, its production process is extremely vulnerable to microbial invasion, and microbial contamination has always been like a haze over this ray of hope. Traditional sterility testing methods, such as USP <71> (sterility testing methods recorded in Chapter 71 of the United States Pharmacopeia), which has been used for half a century, seem to be unable to cope with the new demands of precision medicine: the two-week culture cycle, cumbersome pretreatment steps, and turbidity observations that rely on subjective judgment not only seriously lag behind the short shelf life of cell preparations, but may also expose patients to infection risks due to misjudgment.
With the booming development of cell therapy products, it has become increasingly urgent to detect microbial contamination quickly and accurately. An innovative detection method, machine learning-assisted ultraviolet absorption spectroscopy, has emerged. It combines optical technology with powerful machine learning methods. It does not require a large amount of training data or growth enrichment steps. It only requires a small amount of sample to output the test results in just 30 minutes, providing a strong guarantee for the safety of cell therapy products.
Recently, a joint research team from the Singapore-MIT Research Alliance, Singapore A*SRL Laboratory, National University of Singapore, and Massachusetts Institute of Technology proposed a detection method that combines ultraviolet absorption spectroscopy with machine learning, which can complete the detection of microbial contamination in cell culture supernatants within 30 minutes. This method uses a one-class support vector machine (one-class SVM) to analyze the characteristic differences between nicotinamide and nicotinic acid in the ultraviolet spectrum, and showed an average true positive rate of 92.7% when testing 7 common contaminating microorganisms. Even after excluding donor samples with abnormal niacin metabolism, the true negative rate still reached 92%, far exceeding the accuracy of traditional empirical judgment.
The relevant research results have been published in Nature's journal Scientific Reports under the title "Machine learning aided UV absorbance spectroscopy for microbial contamination in cell therapy products".

Paper address:https://hyper.ai/en/sota/papers/s41598-024-83114-y
Follow the "HyperAI Super Neural" public account and reply "Microbial Contamination Monitoring" to get the complete PDF
Dataset: Data of sterile MSC culture samples collected using a commercial spectrometer
In this study, the construction and analysis of the data set closely revolved around mesenchymal stromal cell (MSC) culture. In view of the wide application of MSC therapy in the treatment of acute tissue injury, inflammatory diseases and chronic degenerative diseases, the research team selected it as a demonstration object and used a commercial spectrometer to collect the absorption spectra of sterile MSC culture samples as the basic data for training a one-class support vector machine (one-class SVM) model. The study adopted an anomaly detection strategy to predict the contamination status of cell culture samples by capturing the differences in spectral features, and explored the potential mechanism of SVM model identification of contamination based on the hypothesis of spectral differences between niacin (NA) and nicotinamide (NAM) metabolites.
During the experiment, the researchers inoculated 10 CFU of E. coli into the MSC culture system of donor A and successfully detected the contamination signal in 21 hours. After comparing the detection performance of samples from 7 commercial donors, donor A performed well in identifying sterile samples from other donors, so it was selected as the initial training data source. In subsequent studies, this method not only achieved effective detection of 7 microorganisms and contamination levels as low as 10 CFU, but also verified the robustness of the technology through cross-donor testing.
In order to deeply analyze the performance of the SVM model, the principal component analysis (PCA) technology was introduced in the study. The Pseudomonas aeruginosa inoculated samples were used as the research object because they showed the highest NA concentration in liquid chromatography-mass spectrometry (LC-MS) detection. The distribution of the training data set and the contaminated samples was visualized by PCA. The results showed that the contaminated samples and sterilized samples were significantly separated in space, effectively distinguishing the sterile and contaminated states. The load vectors of principal component 1 (PC 1) and principal component 2 (PC 2) were further analyzed, and the UV absorption spectra of 100μg/mL NA and NAM in PBS were normalized to intuitively present the association between spectral features and principal components.
In the model robustness verification phase, the study collected discarded culture media and PBS sterilized samples from 6 commercial donors (donor BG) to construct a cross-donor test data set. By training SVM models from different donor sources and cross-validating, it was found that the prediction accuracy of the model trained based on donors A and B was higher. Analysis of 418 test samples showed that the true positive rate of the model reached 92.7%, and the detection limit was stably maintained at 10 CFU; however, the true negative rate of 77.7% indicated that there was still room for improvement, among which the false positive problem caused by abnormal NA levels in donor F samples was particularly prominent, which pointed out the direction for the subsequent optimization of the model's adaptability to metabolic differences between donors.
Machine learning-assisted ultraviolet absorption spectroscopy: Support vector machine as the core algorithm
In order to make machine learning better assist in microbial contamination detection, this study innovatively combined it with ultraviolet absorption spectroscopy and proposed a fast, sensitive and cost-effective method for microbial contamination detection. This method uses support vector machine (SVM) as the core algorithm and accurately identifies microbial contamination by analyzing the ultraviolet absorption spectrum characteristics of cell culture medium.
In terms of model construction, the researchers used a single-class support vector machine and selected the radial basis function (RBF) as the kernel function, setting the γ value to 0.002 and the ν value to 0.2. The training data set consisted of donor 5 from the 2nd to the 7th day of the 2nd, 4th, and 6th generations, and PBS-doped sterile samples of donor 8. These samples were all marked as 1, representing a sterile state. The absorbance value data of each sample covers a wavelength range of 237 nm to 300 nm to focus on the significant spectral features of niacin (NA) and nicotinamide (NAM) to avoid interference from noise in other bands. All samples were mean-centered before model training to correct for spectral deviations caused by factors such as instrument drift, thereby improving the accuracy of the model.
In terms of model validation and application, machine learning-assisted UV absorption spectroscopy was used to demonstrate the ability of this method to detect low-concentration pollutants by adding 10 CFU of E. coli to the MSC culture of donor A and extracting supernatant samples three times every 3 hours between 9 and 24 hours. The results are shown in the figure below. The SVM model can accurately predict that the sample is contaminated after 21 hours, and the total detection time is about 21.5 hours.

Furthermore, the study compared machine learning-assisted UV absorption spectroscopy with existing methods. The results showed that the detection time (TTD) of machine learning-assisted UV absorption spectroscopy was 21 hours, which is comparable to the USP <71> test (24 hours for turbidity to be observed) and the method of calculating the NA/NAM ratio by LC-MS (18 hours). However, both BacT/Alert® 3D and USP <71> require trained operators to extract samples from cell cultures and inoculate them into various growth enrichment media. The workflow of UV absorption spectroscopy is relatively simple, and does not require additional inoculation into growth enrichment media, nor does it require additional incubation time and sample preparation, thereby eliminating the additional resources and costs required for the observed growth enrichment inoculation step.

To determine whether the proposed machine learning-assisted UV absorption spectroscopy method can be applied to other microorganisms, the researchers used PBS-sterilized samples of donor A and applied the method to the detection of other slow-growing microorganisms such as Staphylococcus aureus (S. aureus), Pseudomonas aeruginosa (P. paraeruginosa), Bacillus subtilis (B. spizizenii), Clostridium perfringens (C. sporogenes), yeasts such as Candida albicans (C. albicans), Escherichia coli K-12 (E. coli), and Propionibacterium acnes (C. acnes), with a detection limit (LoD) as low as 10 CFU. In addition, the performance of the SVM model was visualized by principal component analysis (PCA), and it was found that the P. aeruginosa inoculated samples were clearly distinguished from the PBS-sterilized samples in the PCA plot, indicating that the model can effectively capture the differences in spectral features.

In terms of model robustness research, considering that differences between donors may affect model performance, the researchers collected waste culture medium samples from 6 commercial donors (donors BG) and prepared PBS sterilized samples. By training SVM models based on different donors and evaluating their prediction accuracy for other donor samples, it was found that the models of donors A and B had a higher average prediction rate. Therefore, the SVM model trained based on donors A and B was subsequently applied to samples from the other 6 donors. The results showed that machine learning-assisted UV absorption spectroscopy achieved a true positive rate of 92.7%, and the limit of detection (LoD) remained at 10 CFU for the 7 microorganisms tested. However, the true negative rate of the model was 77.7%, and the false positive samples mainly came from donor F. Analysis found that the NA level in donor F samples was high, suggesting that the model needs to be further optimized in the future to improve its adaptability to differences between donors.

AI4S empowers cell therapy: scientific research, industry and policy advance in synergy
In recent years, from scientific research to the business world, from laboratories to industrialization, cell therapy products (CTP) have achieved great success.
In the field of basic research, the TJ-AI4S team of Tongji University in Shanghai, China won the championship in the Global AI Drug Development Algorithm Competition. The molecular feature extension strategy proposed by them improved the generalization ability of the model and provided new ideas for the construction of the molecular fingerprint library of CTP pollutants. The UniBind framework jointly developed by Beijing University of Posts and Telecommunications and Peking University in China analyzes protein interactions through multi-scale graph neural networks, providing a computational basis for studying the dynamic association between cytokines and microbial metabolites in CTP.
Paper link: https://www.nature.com/articles/s41591-023-02483-5
In addition, the team of ShanghaiTech University in China developed the CAR-Toner platform, which uses AI algorithms to optimize the charge distribution of CAR molecules, successfully improving cell amplification efficiency and reducing batch differences. This innovation not only improves production efficiency, but also provides new technical support for the standardized production of CTP.
Paper link: https://www.nature.com/articles/s41422-024-00936-1
The industry has also received good news. The CAR-T drug "Yikaida" of Chinese biotechnology company Fosun Kairui has achieved the first cross-border supply in China in 2025. Behind this is the AI-driven cold chain logistics management system, which ensures the activity of cells during ultra-low temperature transportation. In addition, the American biotechnology company A2 Bio has achieved large-scale pre-production of CAR-T drugs through AI screening of universal donor cells, which has greatly reduced production costs and shortened the waiting period for treatment, bringing more hope to patients.
Overseas research institutions have also achieved fruitful results in the field of AI-enabled cell therapy. In 2025, the biomedical engineering team at Duke University in the United States developed the PepPrCLIP technology, which designed functional short peptides based on the ESM-2 protein language model, providing a new strategy for precision cancer treatment. The AI prediction model built by IBM Watson Health in collaboration with Stanford University in the United States, by integrating patient genome, proteome and clinical multi-dimensional data, has increased the prediction accuracy of cytokine release syndrome (CRS) in CAR-T therapy to 89%, helping clinical intervention in high-risk patients in advance. These have injected new vitality into the development of the global cell therapy field.
At present, AI4S has built a complete chain from basic research to clinical application in the field of CTP, but it still needs to be further improved in data standardization and cross-institutional collaboration mechanisms. Looking to the future, with the increase in policy support and the deep integration of industry, academia and research, AI4S is expected to make greater breakthroughs in the fields of personalized CTP preparation, real-time quality traceability, and cross-border logistics optimization.
Reference articles:
- https://mp.weixin.qq.com/s/VZI7pm-kO7CxNJyrn9-qQw
- https://mp.weixin.qq.com/s/0AoP5XSLOLzcTChQoIIfmw
- https://mp.weixin.qq.com/s/Avu5SbLetCFBVUwnDybfsw
- https://mp.weixin.qq.com/s/WoruStfCdYNDskAn_iFYtQ
- https://www.nsfc.gov.cn/publish/portal0/tab1128/info90687.htm