Covers Inorganic Material Design/crystal Structure Prediction/material Property Recording, Etc., and Summarizes Open Source Data Sets and Models From Meta/Microsoft and Other Institutions
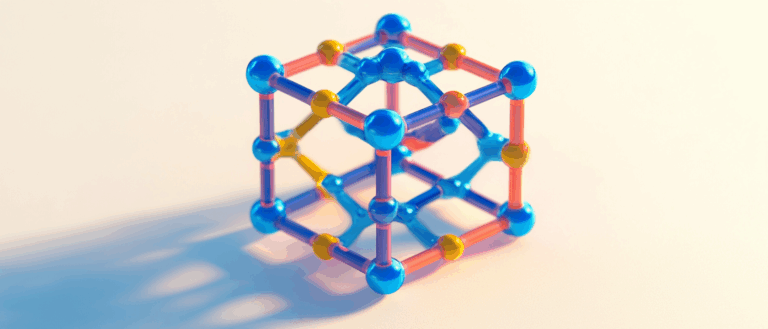
In the era of accelerating integration of artificial intelligence and materials science, data sets are gradually becoming the core engine driving the paradigm shift in materials research. The transition from traditional computational methods based on physical models to data-driven intelligent predictions depends not only on the improvement of algorithm performance, but also on the support of high-quality material data. The comprehensiveness, accuracy and repeatability of data directly determine the upper limit of the model's performance in tasks such as material property prediction, structure generation and function discovery.
Unlike images or natural languages, material data is highly structured and has complex physical constraints, multi-scale coupling, and cross-modal fusion, which makes the construction of its data set more difficult. Whether it is the first-principles calculation results or experimental measurement data, its collection, cleaning, standardization, annotation, and storage must strictly follow scientific processes to ensure the credibility and generalization ability of the data.
In particular, the systematic organization of crystal structure and material property data makes the path from basic physical modeling to machine learning modeling more feasible. The multi-dimensional information contained in the data set, such as formation energy, band gap, volume, density, etc., provides a solid data foundation for researchers to carry out property prediction, material screening, and potential application analysis. At the same time, the standardized format, unified naming system and rich metadata have also significantly improved data traceability and cross-platform usability.
In order to help scholars in related fields to better carry out research,HyperAI has compiled the material science datasets that are currently gaining widespread attention in the industry, as well as one-click deployment tutorials.Covering multiple key directions such as quantum materials, inorganic materials, crystal structures, etc., it allows complex and vast material data to truly serve researchers.
Click to view more open source datasets:https://go.hyper.ai/g9PvL
Material Dataset Summary
1. OMat24 Inorganic Materials Dataset
Estimated size:185.67 GB
Download address:https://go.hyper.ai/hptlY
In 2024, Meta released the Open Materials 2024 (OMat24) large-scale open source dataset, which contains more than 110 million DFT calculation results focusing on structural and compositional diversity, covering different atomic configurations sampled from equilibrium and non-equilibrium structures. It is currently the largest open source dataset for training DFT alternative models of materials.
2. OQMD Open Source Quantum Materials Dataset
Estimated size:32.89 GB
Download address:https://go.hyper.ai/qDyGS
The OQMD dataset contains the thermodynamic and structural properties of more than 1,226,781 materials calculated by density functional theory (DFT). The data comes from the Inorganic Crystal Structure Database (ICSD), including DFT total energy calculations of nearly 300,000 compounds and modifications of common crystal structures, with the aim of storing and sharing quantum material data.
3. Materials Project online material dataset
Download address:https://go.hyper.ai/ELmmX
Materials Project is a large open online materials dataset. The data includes crystal structure, energy properties, electronic structure and thermodynamic properties, covering multiple aspects such as material representation, optoelectronic properties, mechanical properties, physicochemical properties, stability and reactivity, thermodynamic properties and magnetic properties.
4. LLM4Mat-Bench crystal structure dataset
Download address:https://go.hyper.ai/fSTbI
LLM4Mat-Bench is a multimodal language model evaluation dataset for material property prediction. It contains approximately 1.97 million crystal structure samples from 10 public material databases, covering 45 different material physical and chemical properties. It is the largest benchmark to date for evaluating the performance of large language models (LLMs) for material property prediction.
5. Material DFT material property data set
Download address:https://go.hyper.ai/ju56p
This dataset provides a large number of high-quality material property records from the Materials Project database, covering a variety of chemical compositions and physical properties. Each record corresponds to a unique material, and all properties are obtained through density functional theory (DFT) calculations.
Classic Tutorial
In addition to high-quality data, the HyperAI official website also launched the "MatterGen Inorganic Material Design Model Demo", which supports one-click deployment, greatly reducing the threshold for use.
Tutorial address:https://go.hyper.ai/5mWaL

MatterGen is a generative AI-based inorganic material design model launched by Microsoft, which aims to directly generate new materials with specific chemical, mechanical, electronic or magnetic properties through diffusion models.
Specifically, the MatterGen model is mainly based on a diffusion architecture, which first gradually destroys the atomic type, atomic position, and periodic lattice into a random structure, and then trains a model to complete this process in reverse, allowing the model to learn how to gradually restore the original material structure from random noise. Xie Tian, the corresponding author of the paper, believes that this is very similar to the core idea of video generation.
The above is the material data set compiled by HyperAI. If you have resources that you want to include on the hyper.ai official website, you are welcome to leave a message or submit a contribution to tell us!