Selected for ICLR 2025, MIT/UC Berkeley/Harvard/Stanford and Others Proposed the DRAKES Algorithm to Break Through the Bottleneck of Biological Sequence Design
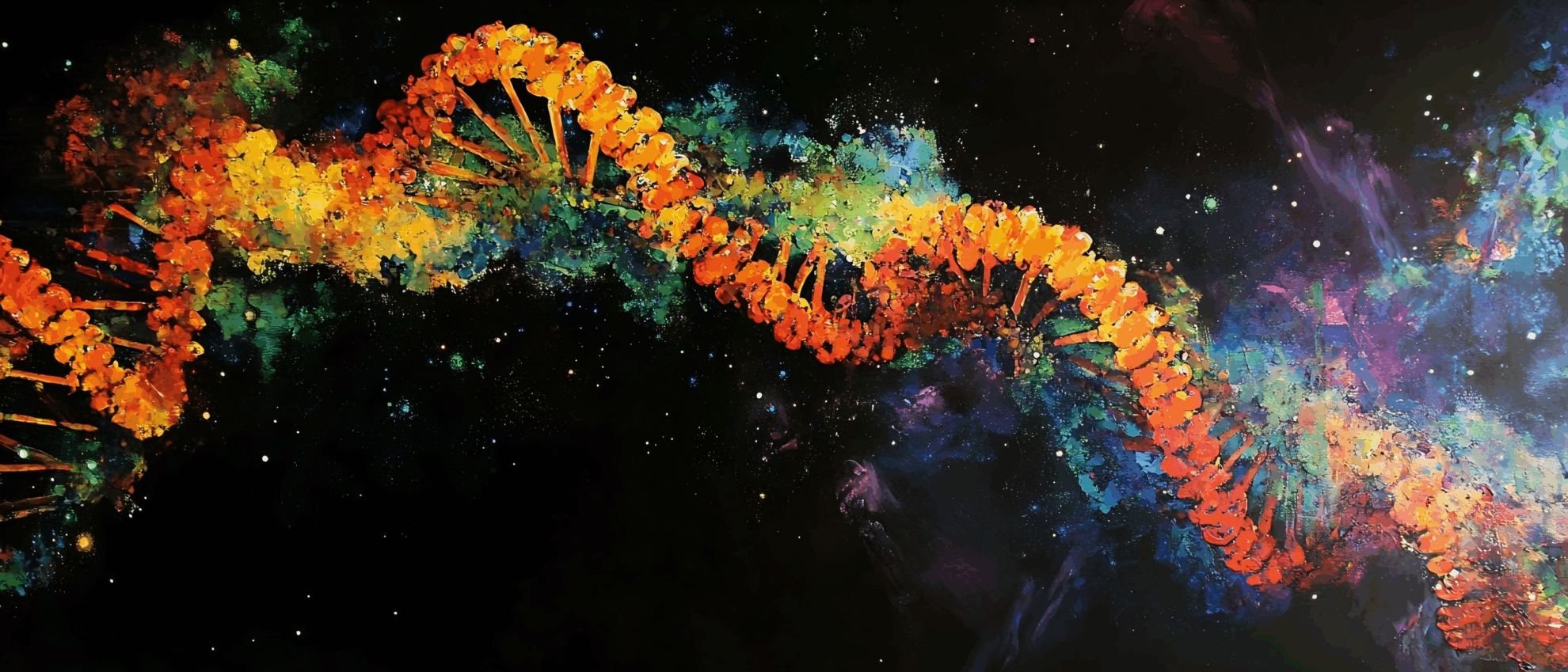
For a long time, the core bottleneck in the field of protein design has not been broken through: the combinatorial space of amino acid sequences has grown exponentially, and traditional computational methods often lose sight of one while optimizing the naturalness and stability of the sequence. In the field of gene therapy, scientists are also faced with the challenge of designing DNA elements that efficiently regulate gene expression; in the development of mRNA vaccines, the contradiction between sequence optimization and translation efficiency improvement always exists; even in natural language generation tasks, engineers need to find a balance between grammatical correctness and content security. These seemingly scattered challenges actually point to the same technical bottleneck:How to optimize specific task objectives while generating discrete sequences that conform to statistical distributions?
To address this key challenge, researchers from the Massachusetts Institute of Technology, Harvard University, Stanford University, the University of California, Berkeley, and the American genetic engineering technology company Genentech jointly proposed an innovative algorithm DRAKES.By introducing a reinforcement learning framework, the algorithm realizes for the first time the differentiable reward backpropagation for the complete generated trajectory in a discrete diffusion model.Experiments show that DRAKES can significantly improve the performance of downstream tasks while maintaining the naturalness of the sequence. Its theoretical analysis further reveals the optimal solution path for this method in balancing distribution fidelity and task optimization.
The relevant research results were selected for ICLR 2025 under the title "Fine-Tuning Discrete Diffusion Models via Reward Optimization with Applications to DNA and Protein Design". For a long time, the core bottleneck in the field of protein design has not been broken through: the combinatorial space of amino acid sequences has grown exponentially, and traditional computational methods often lose sight of one while optimizing the naturalness and stability of sequences. In the field of gene therapy, scientists are also faced with the challenge of designing DNA elements that efficiently regulate gene expression; in the development of mRNA vaccines, the contradiction between sequence optimization and translation efficiency improvement always exists; even in natural language generation tasks, engineers need to seek a balance between grammatical correctness and content security. These seemingly scattered challenges actually point to the same technical bottleneck:How to optimize specific task objectives while generating discrete sequences that conform to statistical distributions?
To address this key challenge, researchers from the Massachusetts Institute of Technology, Harvard University, Stanford University, the University of California, Berkeley, and the American genetic engineering technology company Genentech jointly proposed an innovative algorithm DRAKES.By introducing a reinforcement learning framework, the algorithm realizes for the first time the differentiable reward backpropagation for the complete generated trajectory in a discrete diffusion model.Experiments show that DRAKES can significantly improve the performance of downstream tasks while maintaining the naturalness of the sequence. Its theoretical analysis further reveals the optimal solution path for this method in balancing distribution fidelity and task optimization.
The related research results were selected for ICLR 2025 under the title "Fine-Tuning Discrete Diffusion Models via Reward Optimization with Applications to DNA and Protein Design".

Paper address:
https://doi.org/10.48550/arXiv.2410.13643
Follow the "HyperAI Super Neural" public account and reply "DRAKES" to get the complete PDF
Open source project "awesome-ai4s"It brings together more than 100 AI4S paper interpretations and provides massive data sets and tools:
https://github.com/hyperai/awesome-ai4s
Dataset: Multiple datasets are used in combination to achieve multi-dimensional performance evaluation of DRAKES
This study revolves around the design of regulatory DNA sequences and protein sequences, and uses multiple public data sets to support experimental verification. In the design of regulatory DNA sequences, the study used a large-scale enhancer dataset, which contains about 700,000 DNA sequences of 200 bp in length. Through massively parallel reporter gene assays (MPRAs), enhancer activity in human cell lines was measured, providing basic data for model pre-training and the construction of reward oracle.
The experiment also introduced the chromatin accessibility data of the HepG2 cell line.The chromatin accessibility of synthetic sequences was independently assessed to verify the reliability of predicted activity. In addition, the JASPAR transcription factor binding profile was used to scan the generated sequences for potential transcription factor binding motifs, assisting in the analysis of key features of enhancer activity.
In the protein sequence design task, the pre-trained inverse folding model is based on the PDB training set, which covers the structure and sequence data of natural proteins. The training of the reward oracle relies on the Megascale dataset.The dataset contains approximately 1.8 million sequence variants from 983 natural and designed domains.Stability measurements are provided to evaluate the functional properties of generated sequences. After the data is screened and split by standard processes, approximately 500,000 sequences of 333 domains are formed for building reward models for fine-tuning and evaluation. The combined use of these data sets ensures that the functionality, natural similarity, and stability of model-generated sequences can be effectively verified in different biomolecule design tasks, providing multi-dimensional empirical support for the performance evaluation of the DRAKES method.
DRAKES algorithm: adopts a two-stage architecture and double experiments to verify its potential for application in biomedical scenarios
The researchers proposed an algorithm called DRAKES for fine-tuning discrete diffusion models to optimize the reward function for specific task objectives.The algorithm combines the reinforcement learning (RL) framework and Gumbel-Softmax.It solves the balance problem between maximizing rewards and maintaining naturalness in discrete diffusion models. The core idea of DRAKES is to ensure that the generated sequence remains similar to the pre-trained model distribution while optimizing the rewards by introducing the KL divergence constraint.
Specifically, DRAKES adopts a two-stage architecture, which is designed for the sampling process and the optimization process respectively. In the data sampling stage, the algorithm generates trajectories through a continuous time Markov chain (CTMC) and uses the Gumbel-Softmax technique to convert the discrete sampling process into a differentiable operation. This technique approximates the classification distribution through softmax, maintaining the sampling authenticity and retaining the gradient information under low temperature parameters.This design breaks through the limitation of non-differentiability in traditional discrete diffusion models.It provides a theoretical basis for subsequent optimization.
In the optimization phase,The algorithm updates parameters by maximizing the empirical objective function.Combining Truncated Back-Propagation and Straight-Through Gumbel Softmax technology effectively improves training efficiency. This architecture not only ensures the naturalness of the generated sequence, but also avoids the risk of over-optimization through KL divergence constraints, thereby achieving a dynamic balance between reward maximization and distribution fidelity.

To verify the effectiveness of the DRAKES algorithm, the researchers conducted a comprehensive experimental evaluation in two key tasks: regulatory DNA sequence design and protein sequence design.Experimental results systematically demonstrate the ability of DRAKES to significantly optimize target properties while maintaining sequence naturalness.
In the regulatory DNA sequence optimization task, the enhancer sequences generated by DRAKES showed a synergistic improvement in predictive activity (Pred-Activity=0.78) and chromatin accessibility (ATAC-Acc=0.81) in the HepG2 cell line, while maintaining a triplet nucleotide correlation (0.92) and JASPAR motif correlation (0.88) close to the natural sequence. It is worth noting that although the version without KL regularization obtained higher predictive activity (Pred-Activity=0.85), it performed worse on the independent validation indicator ATAC-Acc (0.72), revealing the risk that over-optimization may cause the generated sequence to deviate from the natural distribution.

In the protein stability optimization task, the sequence generated by DRAKES achieved the optimal balance between predicted stability (Pred-ddG=-1.23 kcal/mol) and structural consistency (83% success rate of scRMSD<2). Comparative experiments show that although the version without KL regularization performs better in predicted stability (Pred-ddG=-1.45 kcal/mol), its structural consistency is significantly reduced (scRMSD<2 success rate is only 61%). Verified by PyRosetta physical simulation, the Gibbs free energy (ΔG=-15.2 kcal/mol) of the sequence generated by DRAKES under the target main chain structure is 21% lower than the baseline method, further confirming the physical rationality of its optimization results.

The experimental results show that the DRAKES algorithm maintains the naturalness of the sequence (log-likelihood App-Log-Lik=-1.05).Significantly improved the optimization capabilities of target attributes.In gene regulatory element design, enhancer activity was improved by 35%; in protein drug design, stability was improved by 28%. These results not only verify the application potential of DRAKES in key biomedical scenarios, but also establish a new technical paradigm for sequence optimization tasks based on discrete diffusion models.
China's innovative breakthroughs in discrete diffusion models and biological sequence design
In recent years, China has built a complete technical system from theoretical innovation to industrial application in the field of discrete diffusion models and biological sequence design, and has proposed a number of original methods in the theoretical framework of discrete diffusion models. For example, the three-dimensional RNA hyperbolic discrete diffusion model developed by Shanghai Yuanma Intelligent Medicine embeds RNA geometric features into hyperbolic space and uses the exponential growth characteristics of hyperbolic geometry to achieve accurate structure-sequence mapping under finite sample conditions. Experimental data show thatThe similarity between the generated sequence and the target structure is 23% higher than that of the traditional method.It shows significant advantages, especially in the prediction of complex pseudoknot structures.This innovative approach of integrating differential geometry with generative models marks that China has entered a new stage of "self-defined paradigm" in the field of biomolecular computing.
In the field of gene therapy,The drug for the treatment of hereditary deafness developed by Li Huawei's team at Fudan University.By precisely regulating the functional expression of DNA sequences, a hearing improvement rate of 68% was achieved in clinical trials.The core of its technology lies in establishing a three-level optimization system of "sequence editing-epigenetic regulation-functional verification".This is in deep agreement with the directional optimization concept of discrete diffusion models at the methodological level. This breakthrough is due to the policy support of the "China (Beijing) Pilot Free Trade Zone Changping Group Pharmaceutical and Health Industry Support Measures" (2023), which clearly lists cell and gene therapy as a key direction and requires collaborative innovation of the entire chain of "algorithm design-experimental verification-clinical transformation".
Article link:
https://doi.org/10.1016/S0140-6736(23)02874-X
The dedicated computing platform deployed by the China National Center for Bioinformatics (CNCB) provides a strategic infrastructure for large-scale biological sequence design, and can quickly complete protein folding simulations that would take months in traditional laboratories. The first phase of the Chinese Population Pan-Genome Consortium (CPC) research progress jointly released by 26 institutions including Fudan University, Xi'an Jiaotong University, and the Chinese Academy of Medical Sciences has initially constructed the first pan-genome reference map exclusively for the Chinese population, laying the foundation for deciphering the genetic code of the Chinese population.This dual-wheel drive model of "computing power + data" effectively solves the two major pain points in biological sequence design: the problem of population specificity and the breakthrough of the long-tail effect.
In the face of the potential risks of AI-generated biological sequences, the National People's Congress revised the "Biosafety Law of the People's Republic of China" in 2024, emphasizing "preventing biosafety risks caused by the abuse of artificial intelligence technology".It is required to implement full-chain supervision on technologies such as gene editing and synthetic biology.Set safe boundaries for technological development.
At present, China has formed a complete innovation chain of "theory-application-facility-standard" in the field of discrete diffusion model and biological sequence design. These advances will not only reshape the underlying logic of biomedical research and development, but are also likely to give birth to a new generation of biotechnology industry revolution. As the Saudi media "Mecca News" said: "China is not only catching up with the West, but also establishing its own innovative characteristics. The young generation of innovators focuses on advanced technologies, which has made China a world-leading biotechnology force and is expected to become a global biotechnology power."
References:
1.https://export.shobserver.com/baijiahao/html/709277.html
2.https://www.ncsti.gov.cn/kjdt/yqdy/cpy2/zchj/202410/t20241012_181850.html
3.https://sghexport.shobserver.com/html/baijiahao/2023/06/15/1051928.html
4.http://news.china.com.cn/2025-01/03/content_117643069.shtml