Enzyme Kinetic Parameter Prediction, Bottleneck Identification... Luo Xiaozhou From the Shenzhen Institute of Advanced Technology, Chinese Academy of Sciences, Shared the Innovative Application of AI in the Enzyme Field
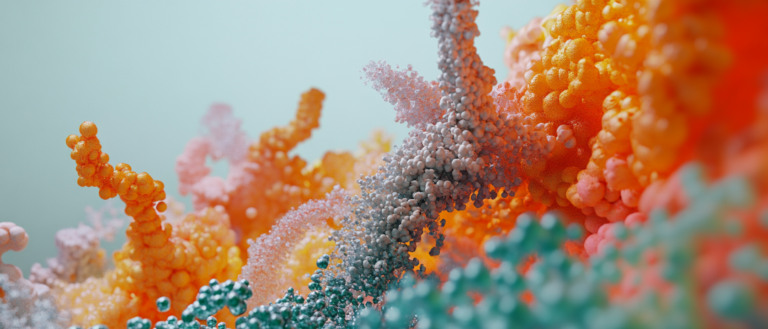
Protein, as the cornerstone of life, plays a key role in life activities. The study of its structure and function is of great significance to the fields of innovative drug development, synthetic biology, enzyme production, etc. However, traditional protein design faces many difficulties. The protein structure is complex and the sequence space is huge. The design method that relies on expert experience and high-throughput screening is not only time-consuming and labor-intensive, but also difficult to guarantee the success rate.
Today, AI for Science has become a new frontier in the development of global artificial intelligence, which is profoundly changing the paradigm of scientific research and bringing about tremendous changes in the field of protein design. Especially after the emergence of innovative results such as AlphaFold, related research has gradually entered the public eye and received more attention. At the same time, it has also further promoted more outstanding teams at home and abroad to devote themselves to this and tackle difficulties from different aspects such as technology and application.
Professor Luo Xiaozhou, a researcher at the Shenzhen Institute of Advanced Technology of the Chinese Academy of Sciences, is one of them. He previously worked in synthetic biology and has been engaged in AI protein research since returning to China in 2019. At the "Future is Coming" AI Protein Design Summit recently hosted by Shanghai Jiao Tong University in China, Professor Luo Xiaozhou shared his views on the topic of "Artificial Intelligence-Driven Enzyme Engineering". Discussing the potential applications of multimodal learning and generative AI in enzyme design,The innovative applications and practices of AI in the field of enzyme engineering are explained from multiple perspectives such as the UniKP framework and ProEnsemble machine.
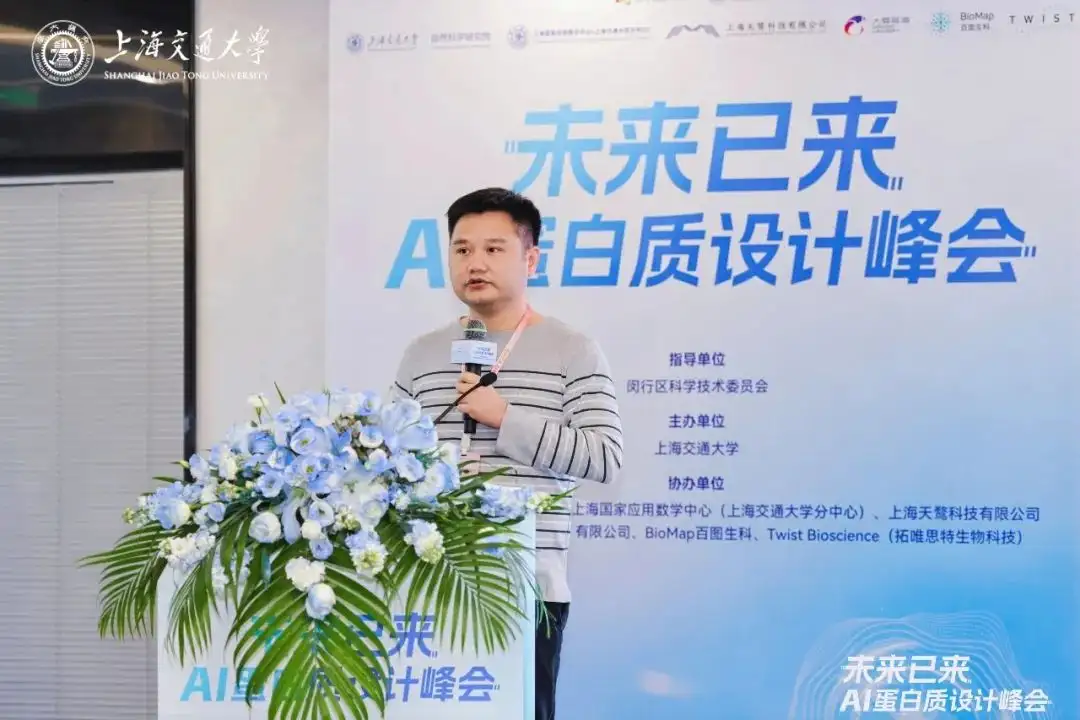
HyperAI has organized and summarized the in-depth sharing without violating the original intention. The following is a transcript of the highlights of the speech.
Automated platform construction, AI solves protein problems
As a treasure trove of medicinal products, natural products have the characteristics of wide sources, rich structures and diverse activities. However, the traditional method of extracting natural products from natural resources is inefficient. Pure chemical synthesis not only has low yields, but also requires the use of a large number of toxic and harmful reagents. For example, artemisinin was originally extracted from Artemisia annua, but faced many problems during chemical synthesis. Later, by regulating multiple genes, the expression of artemisinin was achieved in Saccharomyces cerevisiae. This breakthrough allowed us to see the potential of biosynthesis, so I began to pay attention to research in the biological field. In addition, in the field of enzyme modification, the lack of data will seriously restrict research progress. This problem makes us realize the importance of data, so I am committed to building automation and data platforms to lay the foundation for subsequent AI research.
As the basic molecules of life, nucleic acids, small lipid molecules, sugars, metabolites, ions, water and other substances are all produced from proteins. Based on this characteristic, after returning to China in 2019, I focused my research on the field of proteins and raised three scientific questions: First, can we predict the activity and function of proteins directly from their sequences? Second, can we generate or evolve the proteins people need on demand? Third, can we optimize enzymes or strains based on universal and standardized strategies?
UniKP framework predicts enzyme properties better
The textbook states: The primary sequence of a protein determines its tertiary structure and function, and the primary sequence must contain functional information. Therefore, how to extract the sequence is extremely critical. Inspired by AlphaFold, our team began to explore methods to predict protein function from sequences. In the study, we introduced the Transformer architecture, integrating traditional representation methods with machine learning features to build an integrated model.The peptide and protein function prediction framework based on fusion features and integrated models has achieved SOTA performance on 8 related prediction tasks, accurately predicting peptide and protein functions.It accelerates the screening process of anti-infection active substances such as antimicrobial peptides and reduces experimental costs.
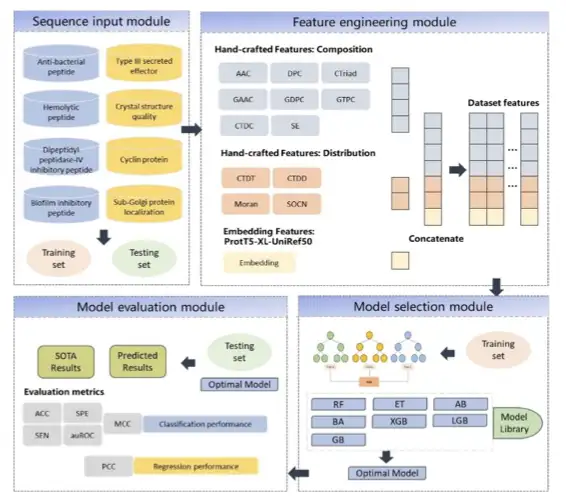
Subsequently, the team used the UniKP framework to try to predict the properties of enzymes based on the Transformer embedding enzymatic parameter prediction tool. They used ProtT5 and the traditional SMILE Transformer model to vectorize the sequence and combined it with a simple machine learning model to achieve SOTA results.
The research team selected four representative datasets to verify the performance and value of UniKP.
First is the DLkcat dataset,The researchers screened 16,838 samples, including 7,822 unique protein sequences and 2,672 unique substrates from 851 organisms. The dataset was divided into training and test sets in a 9:1 ratio.
Next are the pH and temperature datasets,The pH dataset contains 636 samples, consisting of 261 unique enzyme sequences and 331 unique substrates; the temperature dataset contains 572 samples, consisting of 243 unique enzyme sequences and 302 unique substrates. The dataset is divided into training set and test set in a ratio of 8:2.
The third is the Michaelis constant (Km) data set,It consists of 11,722 samples, including enzyme sequences, substrate molecular fingerprints and corresponding Km values. The dataset is divided into training and testing sets in a ratio of 8:2.
The fourth is the kcat/Km data set,Contains 910 samples consisting of enzyme sequences, substrate structures and their corresponding kcat/Km values.
It has been verified that UniKP is significantly better than existing models in kcat prediction and achieves kcat/Km prediction for the first time.Taking kcat as an example, on the largest publicly available data set, the determination coefficient is 20 percentage points higher than the current SOTA result. At the same time, it also performs significantly better in multiple tasks such as different data set divisions, different interval divisions, and different enzyme category divisions.
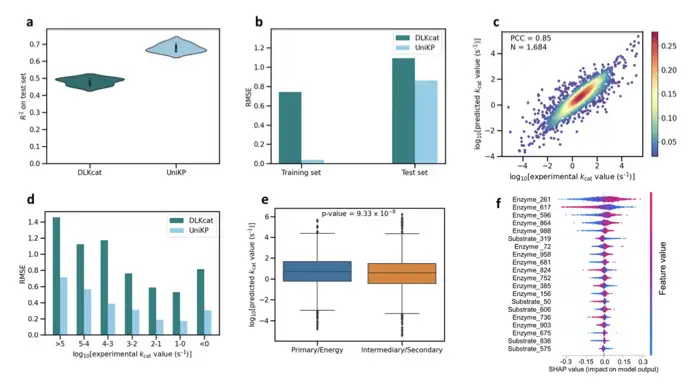
Using this architecture, we found the wild-type TAL enzyme with the highest enzyme activity to date from 1,000 Blast sequences, and obtained mutants with higher enzyme activity by predicting single-site mutations, greatly accelerating the enzyme engineering process.
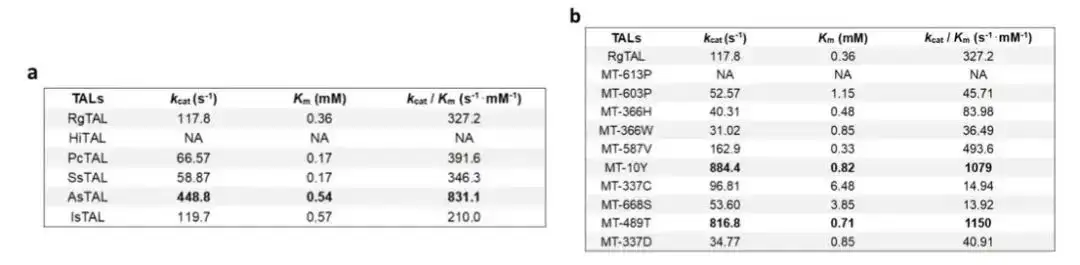
In addition, for the thermal stability of proteins, we proposed a sequence-based thermophilic protein prediction model, Thermal Finer, which achieved SOTA performance on three classification data sets and for the first time predicted the corresponding optimal catalytic temperature (regression) from the protein sequence. In other words, we have for the first time directly predicted the optimal temperature from the protein sequence, providing strong support for enzyme mining and evolution.
ProGPT-2 fine-tuning to generate or evolve proteins on demand
Currently, there are two main types of models for protein production, especially enzyme production:
* Generative Adversarial Neural Networks (GAN): ProteinGAN
* Pre-trained generative large language models (LLM): ProtGPT2, ProGen
but,These protein generation tools all have the problem of generating similar sequences, and are unable to meet the needs of generating enzymes with novel functions and novel activities.There are also some unreasonable aspects in the theoretical analysis: first, the pixel values of the image are continuous, which is more suitable for gradient optimization; second, the text (amino acid sequence) is discontinuous, and gradient optimization is meaningless for updating embeddings, and it is very inefficient.
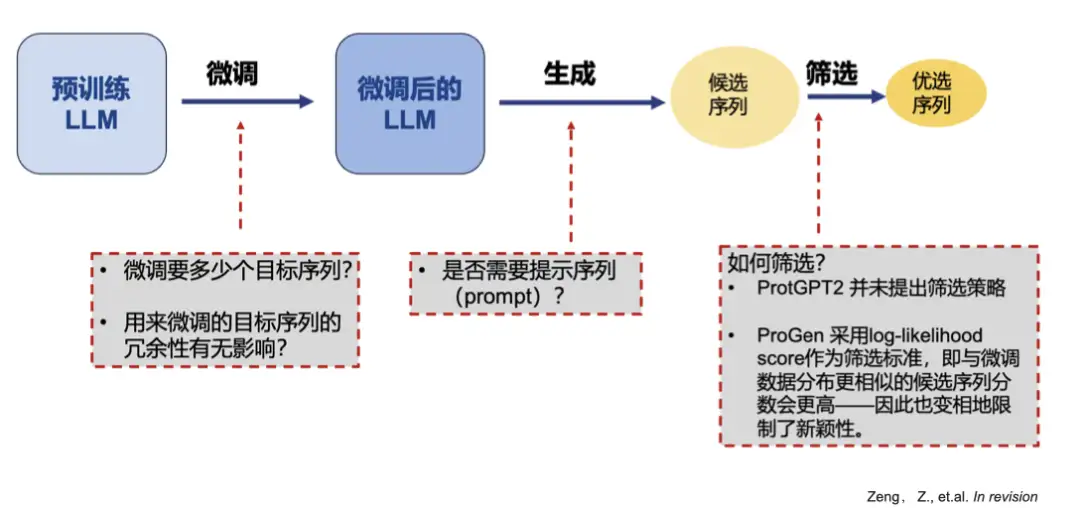
For such problems, we deeply analyze the shortcomings of existing models and propose a new optimization framework.
Our team fine-tuned ProGPT-2 and used a CNN neural network as a discriminator to filter and prioritize the generated sequences. Through experiments, we found thatFine-tuning the sequence only requires 2000 or even less, and the generated sequence without prompt words is closer to the natural enzyme. At the same time, reducing redundant data can improve the novelty of the generated sequence.
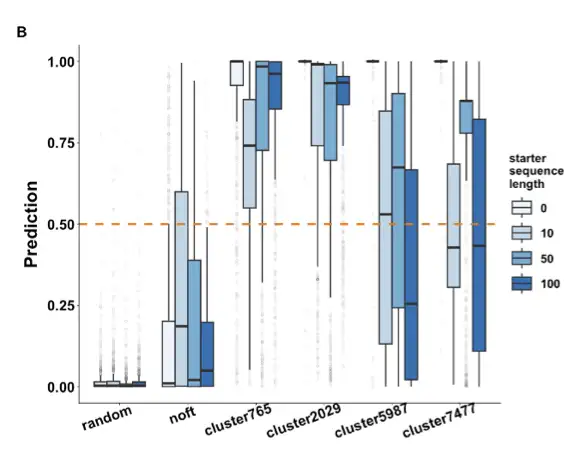
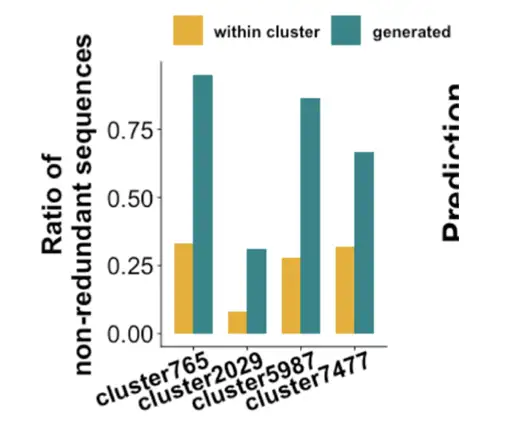
We want novel enzymes with new structures and functions, so we don’t need redundant sequences. Through predictive antimicrobial peptides, we found that this model functioned basically well, and then we did an MDH analysis and found that:The key sites are highly conserved; the prediction scores of those with signature domains are higher; and the molecular docking results are basically the same as those of MDH in nature.As shown in the following figure:
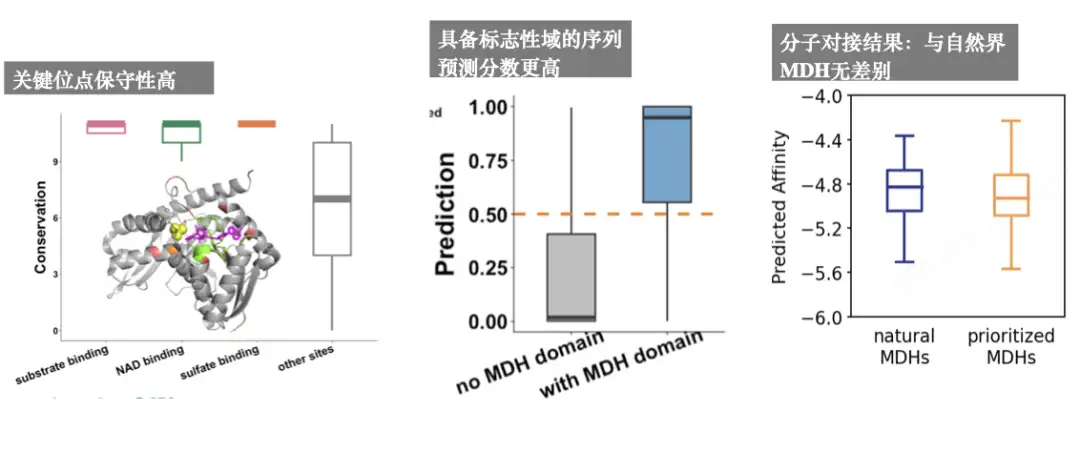
We then verified whether the enzymes produced by the model were functional. Based on the original data of ProteinGAN, the enzymes with a similarity of 80% can reach a similarity of less than 40% after the prioritized MDHs model. Compared with the 10 enzymes we randomly selected from nature, they are basically the same in insoluble, no expression, and soluble, but they still have very good enzyme activity. In other words,The enzymes generated by our team using this model have low similarity to natural enzymes, and most of them have enzymatic activity.
ProEnsemble Identify metabolic bottlenecks and optimize enzyme production
In the biosynthesis process, a series of metabolic bottlenecks such as low catalytic efficiency of multiple enzymes in the metabolic pathway and epistatic effects between enzymes make the optimization process complex and uncertain. Overexpression of pathway enzymes often affects cell growth and product expression, and some enzymes may cause negative effects. For this reason, I asked whether there is a universal and standardized strategy to optimize enzymes or strains?
Let's first verify whether overexpression is really bad?The team artificially reduced the expression levels of certain enzymes to create artificial metabolic bottlenecks, thereby obtaining controllable evolutionary space.
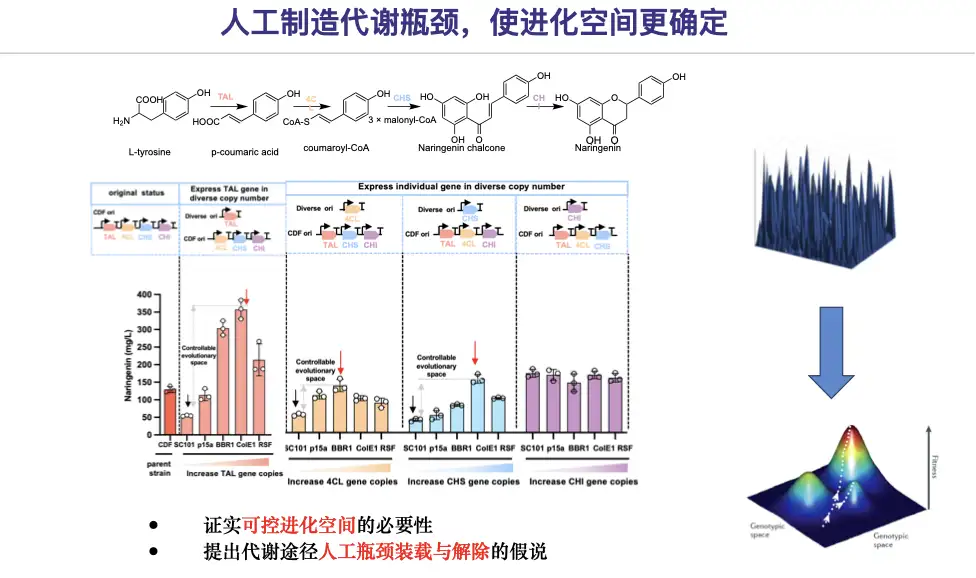
Therefore, a solution for pathway bottleneck design and elimination strategy is proposed, taking naringenin as an example:
* In the first stage, we will use the automated large-scale facility platform technology to allow the genes related to the synthesis of naringenin to be expressed at a low level (low copy number background), thereby constructing an artificial metabolic bottleneck for the synthesis of naringenin.
* In the second stage, candidate mutants 4CL-11C1 and CHS-9H9 were screened for their naringenin production comparable to that of the original mutants, thus eliminating the bottleneck of the naringenin pathway.
* In the third stage, through AI-mediated promoter engineering, mutants of single genes are placed back into the original pathway and the metabolic flux is balanced.
The results of the study showed thatArtificial bottleneck creation and removal strategies enable efficient evolution of metabolic pathways within the confines of clear trajectories.It also further confirms that epistatic effects may limit the boundaries of pathway evolution.
On this basis, we designed an automated process, including instructions, cloning, and bacterial screening tests.The results showed that there was no significant difference between it and manual operation in terms of growth, screening and product extraction.However, automation-assisted metabolic pathway evolution methodsThe time for multi-enzyme parallel evolution is greatly shortened, and one round of parallel evolution can be completed within two weeks.
Based on a large amount of data accumulation, the team developed a machine learning integrated model ProEnsemble to optimize metabolic increments. Experiments show that the machine learning-based integrated model balances metabolic pathways, and the yield of naringenin is 5.16 times higher than that of non-optimized ones, 1.21g/L in 96-well plates and 3.65g/L in fermentation tanks, reaching the highest reported level. Only by overexpressing key synthetic genes, the chassis yields of various modified compounds are higher than those reported in the literature (with the help of metabolic engineering strategies).
ProEnsemble learning strategy builds a metabolic bottleneck identification-optimization closed-loop system, successfully developing a high-yield naringenin Escherichia coli chassis, which is several times higher than the current level in the industry and provides a universal solution for the balance of complex metabolic networks.
Build a large-scale automation platform to promote industry-university-research cooperation
Finally, I would like to introduce the industrial implementation of these achievements. We have built a large-scale fully automated platform - the major scientific and technological facility for synthetic biology research in Shenzhen, China, which includes a large-scale automated platform, covering multiple platforms such as design learning, synthetic testing, and user testing. The platform has powerful functions and can perform standardized data processing and experimental design for machine learning in the cloud. Robots can assist in completing experimental operations, and the spectrum detection speed is fast. One sample can be generated in only 10 seconds, achieving high-throughput detection.
In addition, the platform also provides automated assisted software design, and users can directly select the required components in the component library to generate experimental instructions. We have now cooperated with many industries and academia. We are the first platform in the industry to realize the full process of Streptomyces automation. We welcome everyone to cooperate with us.
About Professor Luo Xiaozhou
Professor Luo Xiaozhou is a researcher, doctoral supervisor, assistant director of the Institute of Synthetic Biology at the Shenzhen Institutes of Advanced Technology, Chinese Academy of Sciences. He is a selected expert of the National Major Talent Project - Youth Project, CTO of the National Biomanufacturing Industry Innovation Center, and deputy chief process engineer of the Shenzhen Major Science and Technology Facility for Synthetic Biology, China.
He received his Ph.D. in Chemistry from the Scripps Research Institute in the United States in 2016 (supervisor: Academician Peter G. Schultz), and then completed postdoctoral research at the University of California, Berkeley (co-supervisor: Academician Jay D Keasling). He joined the Shenzhen Institutes of Advanced Technology of the Chinese Academy of Sciences in 2019. He has been selected into the National Youth Talent Program, Guangdong Province Outstanding Youth Program, and Shenzhen Excellent Youth Program.
His research focuses on the biochemical processes in living organisms in the field of synthetic biology, including directed evolution of enzymes, protein engineering, high-throughput screening, and total biosynthesis of natural and non-natural compounds. As a corresponding author, he has published 20 papers in Nature Metabolism, Advanced Science, Nature Synthesis, Nature Communications, Angew. Chem. Int. Ed., etc., and a total of more than 50 SCI papers, applied for more than 30 patents, and authorized 6.