Stable Virtual Camera Redefines 3D Content Generation and Unlocks New Dimensions of Images; BatteryLife Helps Predict Battery Life More Accurately
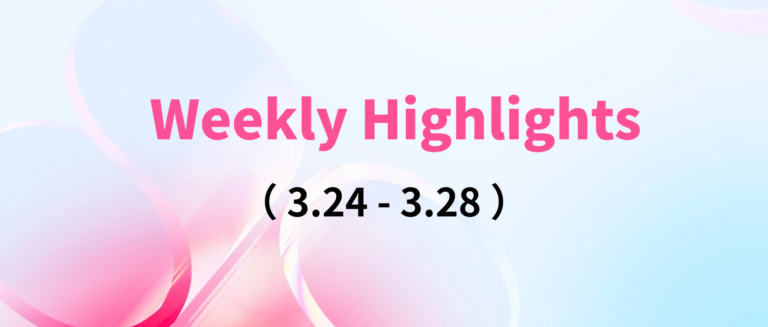
In the fierce competition of digital content creation, Stability AI is standing at the crossroads of fate. The company that once ignited the image generation revolution with Stable Diffusion has fallen into crisis due to upper management problems. Recently, Stability AI launched the Stable Virtual Camera model, and it is unknown whether it can break the deadlock with a heavy punch.
Stable Virtual Camera is a multi-view diffusion model, which combines the control capabilities of a traditional virtual camera with the creativity of generative AI. The technology is able to transform ordinary 2D images into 3D videos with realistic depth and perspective effects, without the need for complex scene reconstruction or specialized skills.
Compared with traditional 3D video models,The model does not require a large number of input images or complex pre-processing steps, making the generation of 3D content much simpler and more feasible.And this technology performs well on the Novel View Synthesis (NVS) benchmark.The performance exceeds some existing models.
Currently, HyperAI Super Neural is online 「Stable Virtual Camera images turn into 3D videos in seconds」Tutorial, come and try it~
Online use:https://go.hyper.ai/N2u9l
From March 24 to March 28, hyper.ai official website updates:
* High-quality public datasets: 10
* Selection of high-quality tutorials: 3
* Community article selection: 3 articles
* Popular encyclopedia entries: 5
* Top conferences with deadline in April: 10
Visit the official website:hyper.ai
Selected public datasets
The dataset covers 17,966 characters and 29,798 real dialogues, including not only character overviews and dialogues, but also plot summaries, character experiences, and dialogue backgrounds. In addition, the dialogues cover three dimensions: language, action, and thought, making the character performance more three-dimensional.
Direct use:https://go.hyper.ai/1WbXV
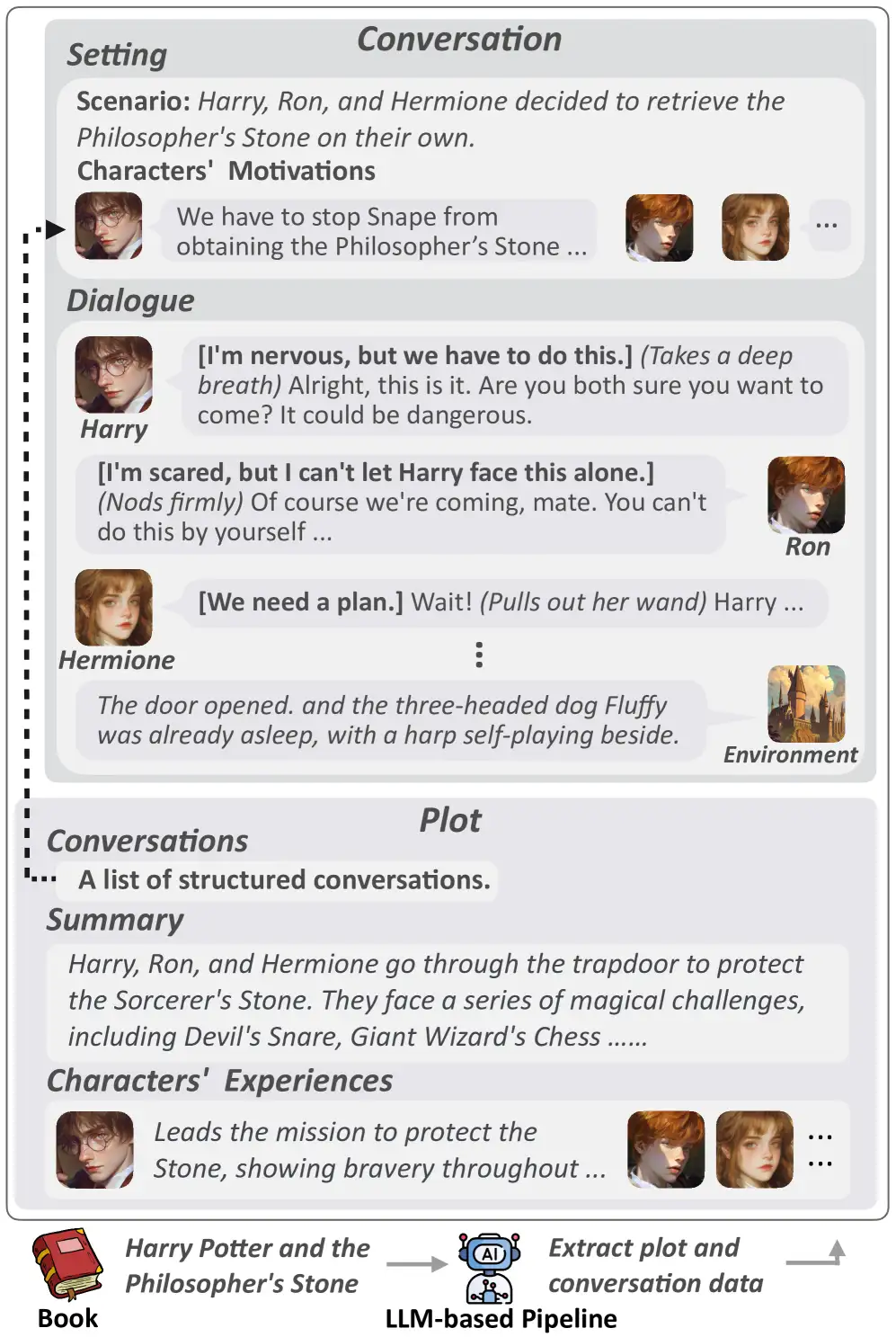
2. MV-MATH Mathematical Reasoning Annotation Dataset
The MV-MATH dataset contains 2,009 high-quality math problems, which are divided into three types: multiple-choice questions, fill-in-the-blank questions, and multi-step questions. The dataset contains multiple visual scenes, and each question is accompanied by 2 to 8 images. These images are intertwined with text to form complex multi-visual scenes, which are closer to math problems in the real world and can effectively evaluate the model's reasoning ability to process multi-visual information.
Direct use:https://go.hyper.ai/tRQsA
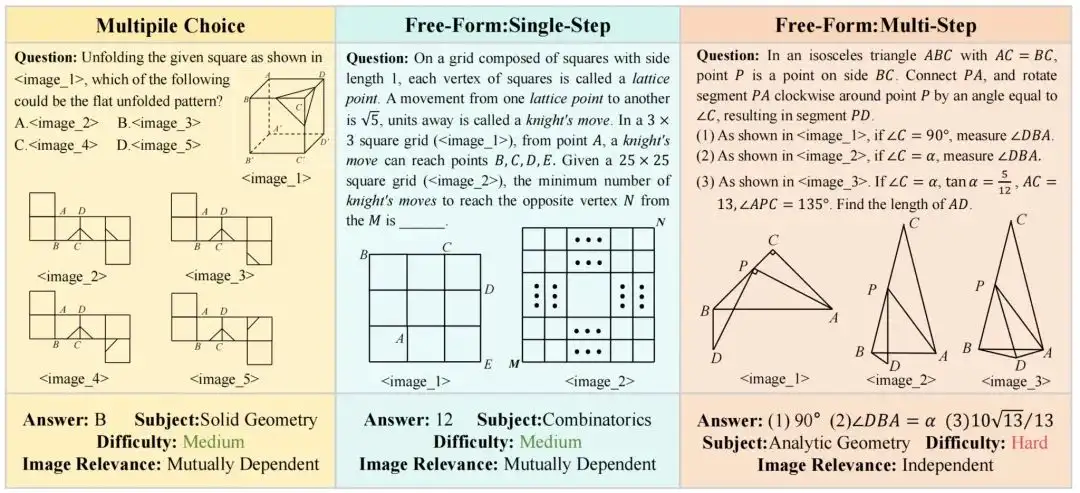
3. WideRange4D Multi-view Scene Dataset
This dataset fills the gap in existing 4D reconstruction datasets in complex dynamic scenes by introducing 4D scene data with a large range of spatial motion. It excels in scene richness, motion complexity, and environmental diversity, including real-world scenes (such as city streets and rural roads) and virtual scenes, covering short-distance, medium-distance, and long-distance motion, as well as complex motion trajectories, while also simulating a variety of weather conditions such as sunny days, rainy days, and sandstorms.
Direct use:https://go.hyper.ai/9KszI
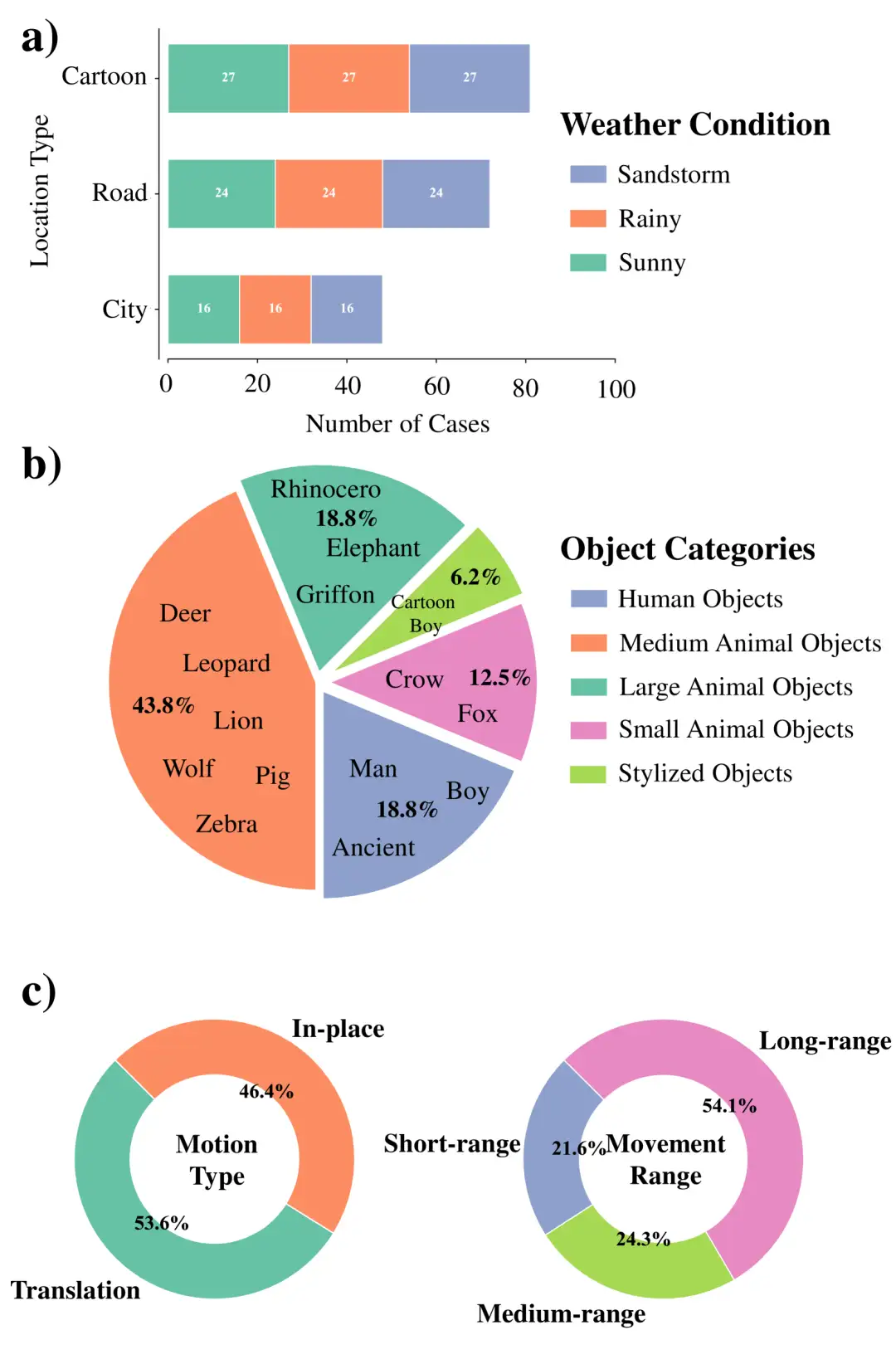
4. TacQuad Multimodal Multisensor Tactile Dataset
TacQuad is an aligned multimodal multi-sensor tactile dataset collected from 4 types of visual tactile sensors (GelSight Mini, DIGIT, DuraGel and Tac3D). It provides a more comprehensive solution to the low standardization of visual tactile sensors by providing multi-sensor aligned data with text and visual images. This explicitly enables the model to learn semantic-level tactile properties and sensor-independent features, thereby forming a unified multi-sensor representation space through a data-driven approach.
Direct use:https://go.hyper.ai/uL0Zd
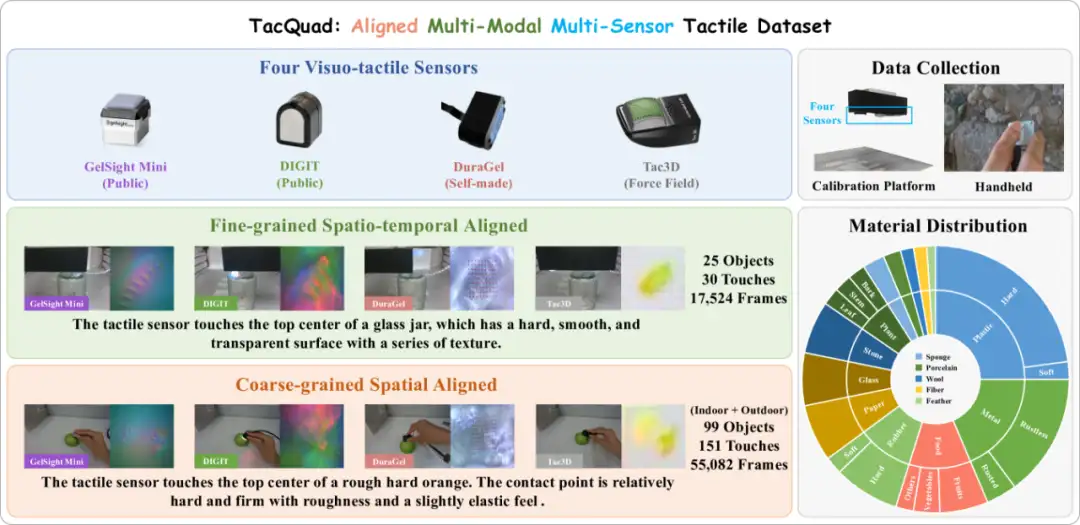
5. Physical AI Robotics and Autonomous Driving Video Dataset
This dataset is a physical AI dataset released by NVIDIA at the GTC25 conference. It contains 15 TB of data, more than 320,000 trajectories for robot training, and up to 1,000 general scene description (OpenUSD) assets, including the SimReady collection, covering different types of roads and geographical environments, different infrastructures, and different weather environments.
Direct use:https://go.hyper.ai/LEHa5
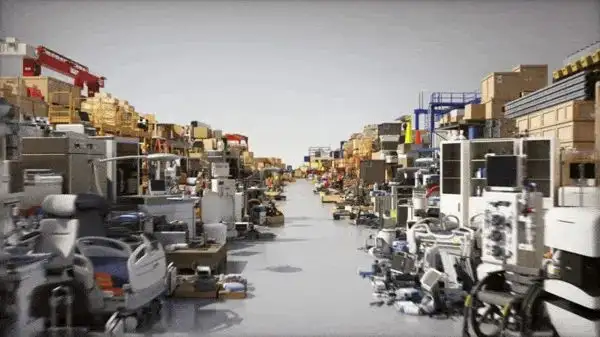
6. Aerial Landscapelmages Aerial Landscape Dataset
Skyview is a curated dataset for aerial landscape classification, with a total of 12k images, 15 different categories, each category contains 800 high-quality images with a resolution of 256×256 pixels. This dataset combines images from the publicly available AID and NWPU-Resisc45 datasets. This compilation aims to promote research and development in the field of computer vision, especially in aerial landscape analysis.
Direct use:https://go.hyper.ai/mne9z
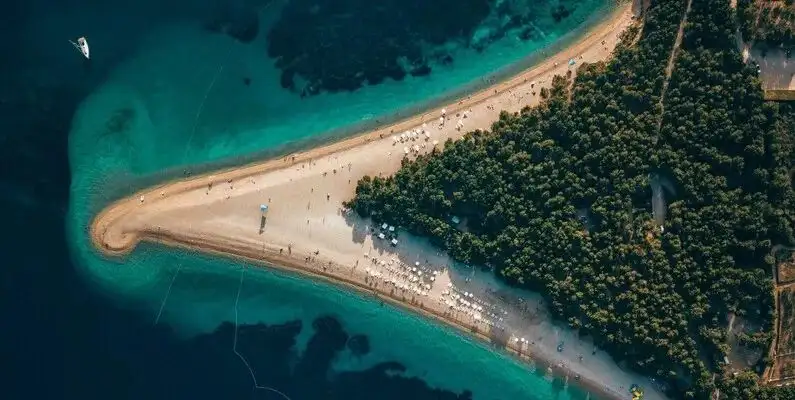
7. EMM-AU Driving Accident Video Dataset
This dataset is the first dataset designed specifically for driving accident reasoning tasks, and extends the MM-AU dataset by leveraging advanced video generation and enhancement techniques. The dataset contains 2k newly generated detailed accident scene videos, which are generated by fine-tuning the pre-trained Open-Sora 1.2 model, aiming to provide richer and more diverse training data for accident understanding and prevention.
Direct use:https://go.hyper.ai/gy0mb
8. BatteryLife Battery Life Prediction Dataset
This dataset was originally created to support research on battery life prediction. It integrates 16 different datasets and provides more than 90,000 samples from 998 batteries, all with life labels. The size of the BatteryLife dataset is 2.4 times that of the previous largest battery life resource, BatteryML.
Direct use:https://go.hyper.ai/0PzfZ
9. VenusMutHub protein mutation small sample dataset
VenusMutHub is the first small sample dataset of protein mutations for real application scenarios. The research team carefully compiled 905 small sample experimental mutation datasets for real application scenarios, covering 527 proteins (of which 98% has 5-200 mutations), and covering various functional measurement data such as stability, activity, binding affinity and selectivity. All data are measured using direct biochemical measurements rather than alternative fluorescence readings to ensure the accuracy of the evaluation.
Direct use:https://go.hyper.ai/8y20R
10. Bird vs Drone Bird and drone image classification dataset
The dataset contains a diverse collection of images from the Pexel website, representing birds and drones in motion. These images are captured from video frames, segmented, enhanced, and pre-processed to simulate different environmental conditions, thereby enhancing the model training process.
Direct use:https://go.hyper.ai/RdN4d
Selected Public Tutorials
1. Image dehazing using MATLAB
In the field of computer vision, image dehazing is an important preprocessing task, especially in autonomous driving, remote sensing image analysis and monitoring systems. Dehazing can effectively improve image quality and make the target more clearly visible.
This project uses the Retinex algorithm to remove fog from images, and combines it with GPU acceleration to improve computational efficiency. You can complete the image dehazing process by entering the relevant code according to the tutorial.
Run online:https://go.hyper.ai/wu1fE
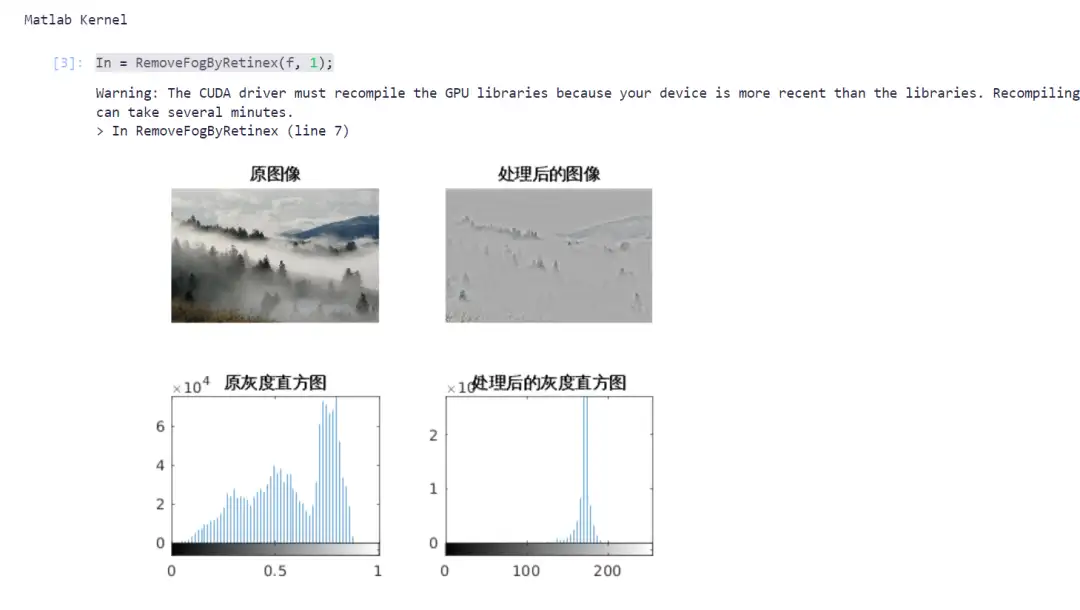
2. Stable Virtual Camera turns images into 3D videos in seconds
Stable Virtual Camera (Seva) is a general diffusion model launched by Stability AI in March 2025. Seva is able to generate new views of a scene based on any number of input views and target cameras. Its design overcomes the limitations of existing methods in generating samples with large view changes or smooth temporal motion, without relying on a specific task configuration.
A notable feature of the model is that it can maintain highly consistent sample generation without the need for additional 3D representation learning, thereby simplifying the perspective synthesis process in practical applications. In addition, Seva can generate high-quality videos up to half a minute long and achieve seamless looping. Extensive benchmark tests show that Seva outperforms existing methods on different datasets and settings.
The relevant models and dependencies of this project have been deployed. After starting the container, click the API address to enter the Web interface.
Run online:https://go.hyper.ai/N2u9l
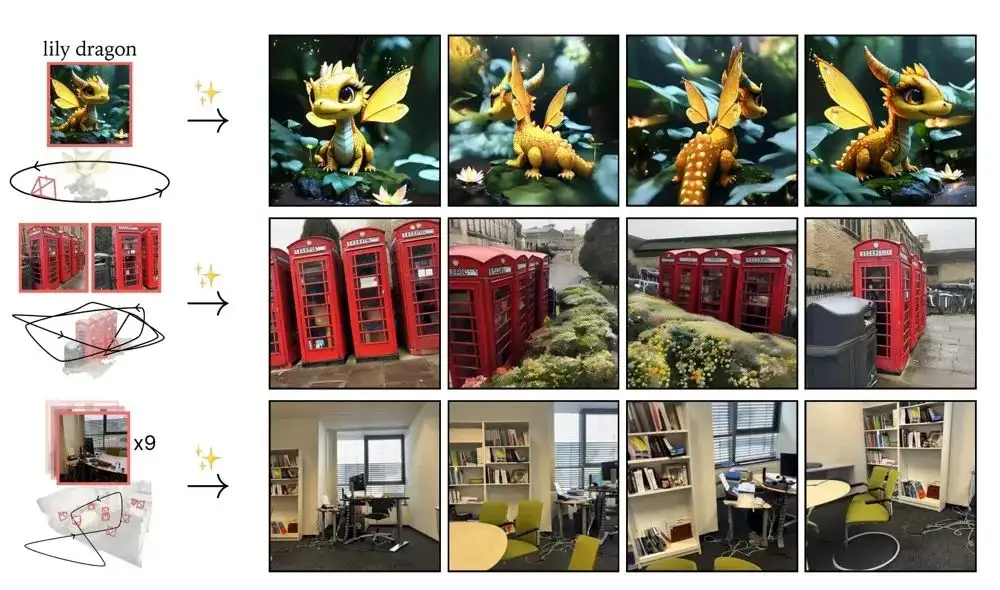
The speech generation model CSM (Conversational Speech Model) launched by the Sesame team can output smooth, natural, and emotional speech based on text and audio input. Compared with traditional AI speech generation models, CSM has stronger emotional understanding capabilities, more natural conversation rhythm, and almost zero-delay real-time interaction, without any drone feeling.
Run online:https://go.hyper.ai/bxOoN
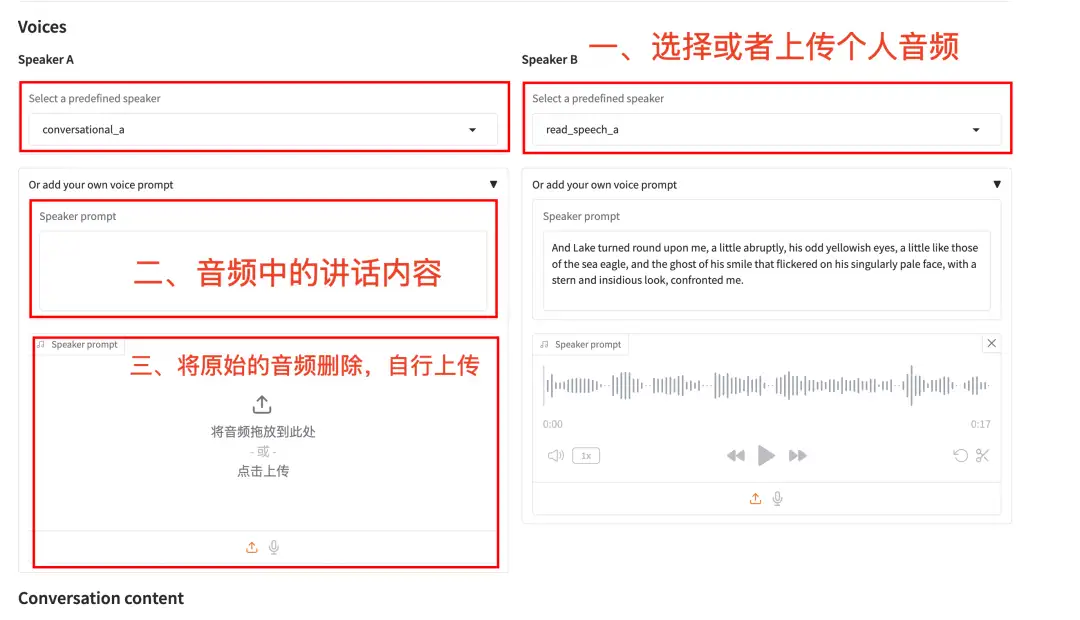
Community Articles
The AcneDGNet deep learning algorithm developed by the Peking University International Hospital team can accurately identify acne lesions and automatically determine the severity, with a diagnostic accuracy comparable to that of senior dermatologists. It provides strong support for online consultations and offline medical treatment, and helps to manage acne more efficiently. This article is a detailed interpretation and sharing of the research.
View the full report:https://go.hyper.ai/qAjYK
A research team from the University of Cambridge proposed a method called AlphaFold-Metainference. This method uses the correlation between the alignment error map predicted by AlphaFold and the distance change matrix in the molecular dynamics simulation to construct a set of structures of disordered proteins and proteins containing disordered regions, providing new ideas for the prediction of disordered protein structures based on deep learning methods, and further broadening the scope of application of AlphaFold. This article is a detailed interpretation and sharing of the research.
View the full report:https://go.hyper.ai/6Bbhc
Studies have shown that after long hours of working with medical images, professionals' visual fatigue can cause boundary errors up to 12%. To solve this problem, NVIDIA recently teamed up with other research teams to propose a VISTA3D multimodal medical image segmentation model. This model pioneered a three-dimensional supervoxel feature extraction method, which achieves the coordinated optimization of three-dimensional automatic segmentation and interactive segmentation through a unified architecture. In a comprehensive benchmark test involving 23 data sets, its segmentation accuracy is 5.2% higher than the existing optimal expert model. The relevant results have been published in preprint form on arXiv. This article is a detailed interpretation and sharing of the research.
View the full report:https://go.hyper.ai/D19LU
Popular Encyclopedia Articles
1. DALL-E
2. Reciprocal sorting fusion RRF
3. Pareto Front
4. Large-scale Multi-task Language Understanding (MMLU)
5. Contrastive Learning
Here are hundreds of AI-related terms compiled to help you understand "artificial intelligence" here:
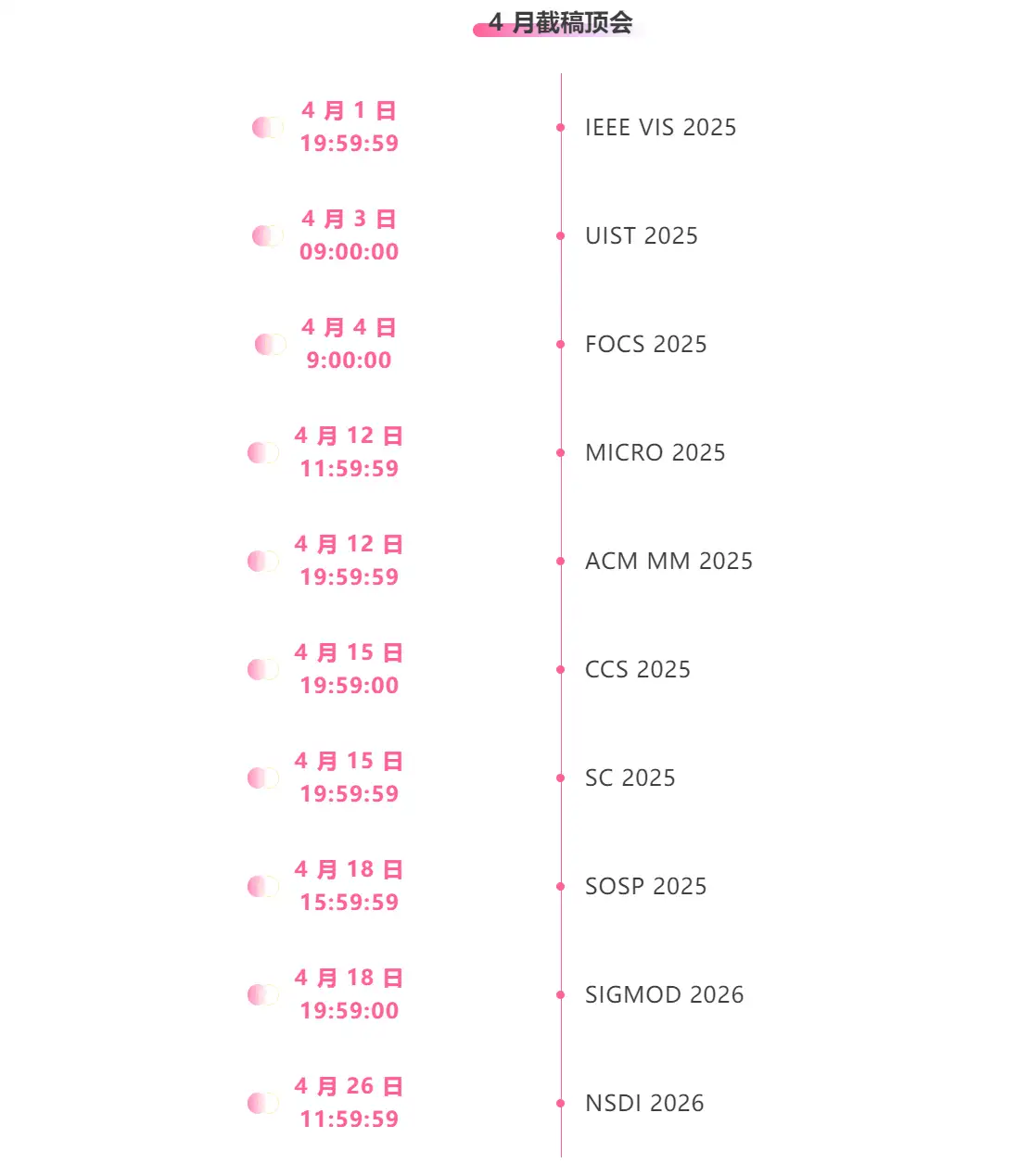
One-stop tracking of top AI academic conferences:https://go.hyper.ai/event
The above is all the content of this week’s editor’s selection. If you have resources that you want to include on the hyper.ai official website, you are also welcome to leave a message or submit an article to tell us!
See you next week!