Accuracy Improved by 5.2%, NVIDIA and Others Released a Multimodal Medical Image Segmentation Model to Achieve Automatic Segmentation and Interaction of 3D Images
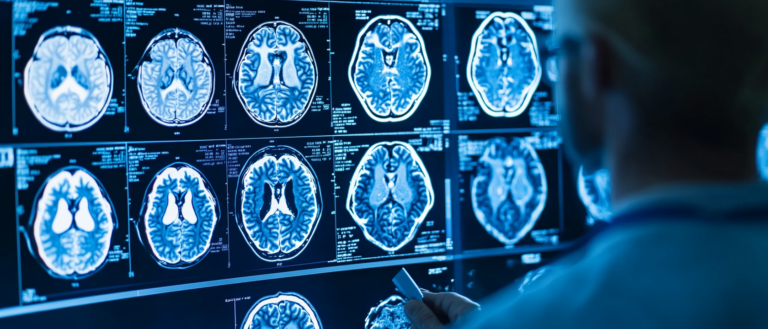
Since the birth of the first clinical CT scanner in 1971, medical imaging has undergone a revolutionary leap from two-dimensional slices to three-dimensional stereo. Modern 256-row spiral CT can collect whole-body scan data with a layer thickness of 0.16mm in 0.28 seconds, and 7T ultra-high field magnetic resonance imaging can even capture the microscopic direction of nerve fibers in the hippocampus. But when these three-dimensional matrices containing tens of millions of voxels are presented to doctors, the task of accurately segmenting organs, lesions, and vascular networks is still highly dependent on manual layer-by-layer outlining. Studies have shown that liver segmentation for a typical set of abdominal CT images takes 45-90 minutes, and radiotherapy planning annotation involving multi-organ linkage may last more than 8 hours.The boundary error rate caused by professionals' visual fatigue can reach 12%.
This dilemma has spawned the most active innovation track in the field of medical image analysis. From the early grayscale threshold-based region growing algorithm, to the U-Net three-dimensional variant V-Net that integrates deep learning, to the TransUNet hybrid architecture that introduces the visual Transformer, algorithm engineers are constantly trying to build intelligent navigation systems in the pixel maze. The latest breakthroughs at the 2024 MICCAI conference showed that some models have achieved inter-group consistency comparable to that of senior radiologists in prostate segmentation tasks, but their performance in rare anatomical variation cases still fluctuates greatly. This reveals a deeper technical philosophical proposition: When AI tries to understand the human body, how much prior knowledge does it need to be injected, and how much anatomical insight beyond human cognition can it produce?
Recently, an interdisciplinary team consisting of NVIDIA, the University of Arkansas for Medical Sciences, the National Institutes of Health, and the University of Oxford published a breakthrough research result: the VISTA3D multimodal medical image segmentation model.This model pioneered a 3D supervoxel feature extraction method.Through a unified architecture, it realizes the collaborative optimization of 3D automatic segmentation (covering 127 anatomical structures) and interactive segmentation. In a comprehensive benchmark test containing 14 datasets, it achieves the most advanced 3D hintable automatic segmentation and interactive editing, and improves the zero-sample performance by 50%.
The relevant research results are titled "VISTA3D: A Unified Seamentation Foundation Model For 3D Medical Imaging" and have been published as a preprint on arXiv.
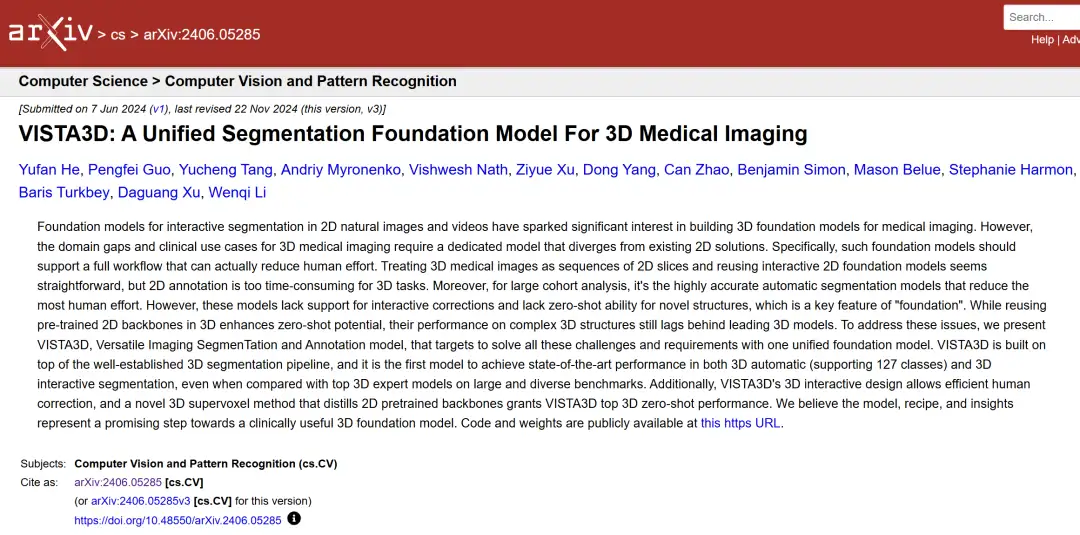
Paper address:
https://doi.org/10.48550/arxiv.2406.05285
Paradigm shifts and challenges in 3D medical imaging technology
In the digital wave of medical image analysis, 3D automatic segmentation technology is undergoing a paradigm shift from "specialists" to "general practitioners". Traditional methods build dedicated network architectures and customized training strategies to create independent expert models for each anatomical structure or pathology type. Although this model performs well in specific tasks, it is like asking radiologists to repeatedly undergo single-organ diagnosis training.When faced with a full-body CT scan containing 127 anatomical structures, the system needs to run dozens of models in parallel, and its computing resource consumption and result integration complexity grow exponentially.
More importantly, in clinical practice, what really troubles doctors are often those rare cases that break through the standard anatomical atlas: it may be the newly discovered nanoscale calcification foci in the liver of experimental mice, or the unconventional blood vessel shape formed by anatomical variations in transplant patients. These scenarios expose the fundamental flaws of the existing system.Over-reliance on preset categories and closed training makes it difficult for the model to perform zero-shot learning and open domain adaptation.
The dawn of a breakthrough in this dilemma comes from the inspiration of the field of natural image processing. When large language models showed amazing generalization capabilities across tasks, the medical imaging community began to explore the construction of "conversational" intelligent systems. SAM (Segment Anything Model) proposed by Meta realized the revolutionary interaction of "click to segment" in two-dimensional images, and its zero-sample performance even surpassed some professional models. However, when migrating this paradigm to the field of three-dimensional medicine, simple dimensional expansion encountered fundamental challenges: the topological complexity of human organs in continuous tomography is far from comparable to moving vehicles in videos.
Taking liver segmentation as an example, portal vein bifurcations, tumor infiltration, and surgical clip metal artifacts may coexist between adjacent slices, which requires the model to have true three-dimensional spatial reasoning capabilities rather than simple time series tracking. Previously, researchers have tried to three-dimensionalize the SAM architecture to form the SAM2 and SAM3D systems. Although progress has been made in tasks such as vascular tracking,However, its Dice coefficient is still 9-15 percentage points lower than that of the professional model.The error rate increases dramatically especially when dealing with overlapping areas of multiple organs.
The deeper contradiction lies in the knowledge-dependent nature of medical data. When natural image segmentation can rely on pixel-level statistical features,Medical image analysis must integrate anatomical prior knowledge.For example, pancreas segmentation requires not only identifying grayscale features, but also understanding its anatomical proximity to the duodenum. This has spawned a new paradigm based on contextual learning: guiding the model to adapt to new categories by inputting example images or text descriptions.
However, the problems exposed by the existing system during testing are quite ironic: requiring clinicians to provide high-quality example annotations itself violates the original intention of automated segmentation; and the text-guided semantic alignment bias may lead to the misjudgment of hilar cholangiocarcinoma as normal vascular structure. This paradox of the technical path reflects the fundamental proposition in the development of medical AI:How to establish a dynamic balance between open domain adaptation and clinical safety may be more practical than simply pursuing algorithm performance.
VISTA3D: A Unified Segmentation Base Model for 3D Medical Imaging
In order to break through the paradigm limitations of 3D medical image analysis,NVIDIA's research team built an innovative architecture that combines the advantages of two-dimensional pre-training with three-dimensional anatomical characteristics - the VISTA3D model.As shown in the figure below, if the segmentation task X belongs to the 127 supported categories (green circle on the left), VISTA3D will perform highly accurate automatic segmentation (Auto-seg). The doctor can review and efficiently edit the results using VISTA3D when needed. If X is a new category (blue circle on the right), VISTA3D will perform 3D interactive zero-shot segmentation (Zeroshot interactive).
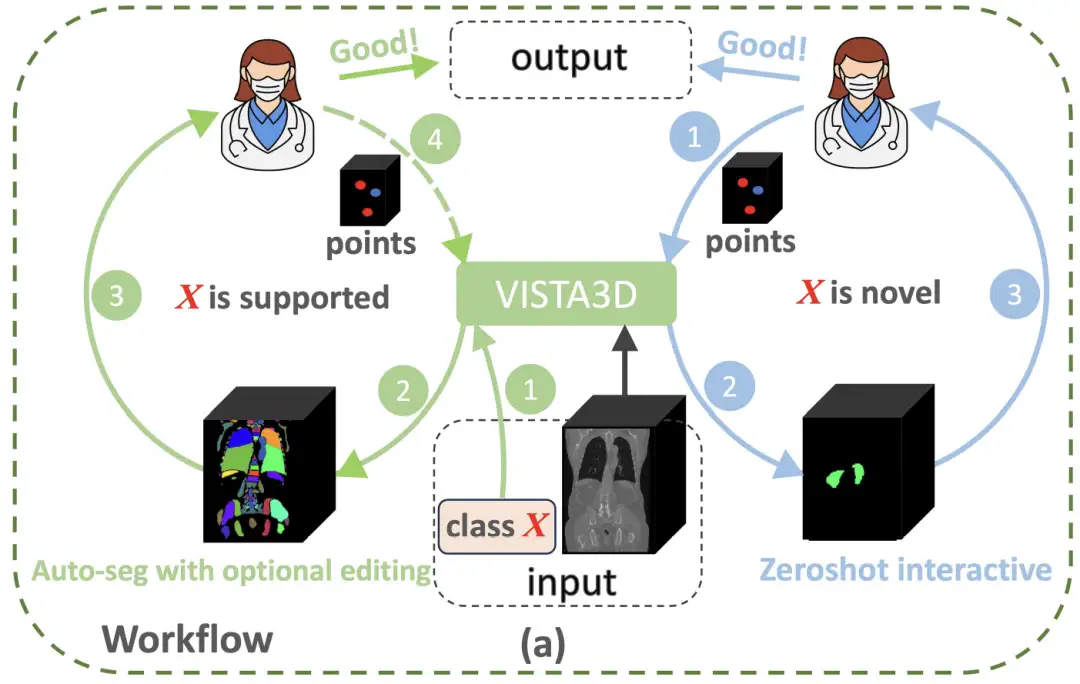
Specifically,The VISTA3D model architecture adopts a modular design concept and builds a 3D segmentation core based on SegResNet, which has been widely verified in the field of medical imaging.This U-shaped network architecture has demonstrated excellent performance in international authoritative segmentation challenges such as BraTS 2023. As shown in the figure below, if the user provides a class prompt that belongs to the 127 supported categories, the top auto-branch will activate the out-of-the-box automatic segmentation function. If the user provides a 3D click point prompt, the bottom interactive branch will activate the interactive segmentation function. If both branches are activated, the algorithm-based merging module will use the interactive results to edit the automatic results.
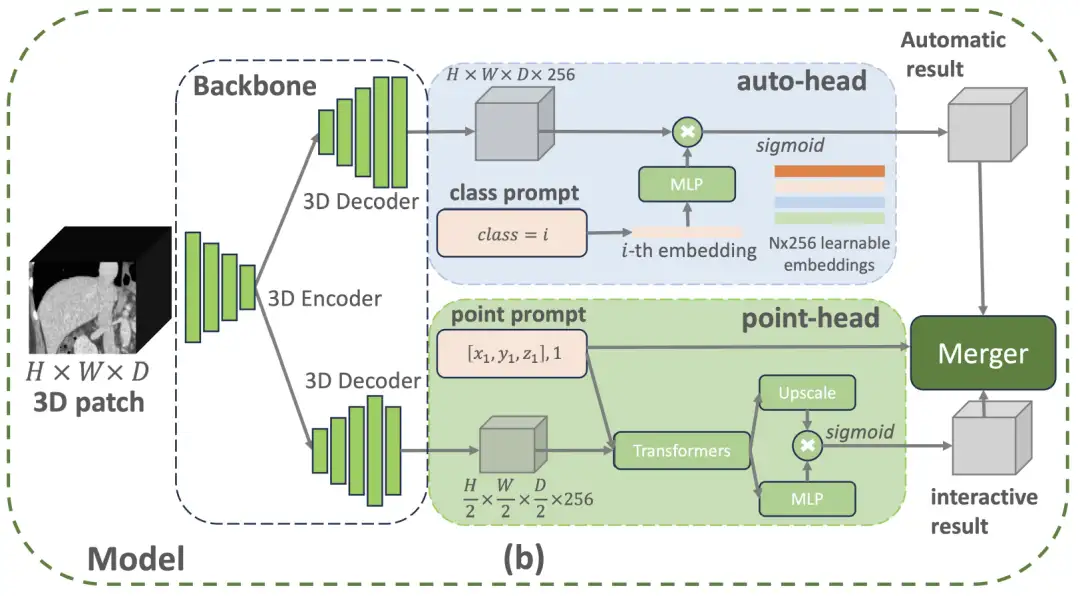
The automatic branch uses intelligent coding technology to manage 127 human structures. When a specific part needs to be located, the system will accurately match the feature information in the scanned image and generate segmentation results through intelligent conversion.This design saves 60% memory resources compared to traditional methods and can also avoid learning bias caused by incomplete annotations.The manual correction module uses three-dimensional click positioning technology: first restore the image details and then optimize the processing speed. The location clicked by the doctor will be converted into spatial coordinates and intelligently associated with the scanning features. When encountering easily confusing structures such as the pancreas and tumors, the system will automatically add distinguishing marks.
The two modules achieve precise adjustments through intelligent collaboration. The correction operation only affects the local area connected to the click position, just like using a precise scalpel to modify a specific part without destroying the overall segmentation result.This three-dimensional optimization solution improves doctors' correction efficiency by 40%.During the model training phase, the research team also integrated 11,454 CT scan data, adopted a pseudo-label generation mechanism under a semi-supervised learning framework, and combined a four-stage progressive training strategy. First, pre-training was performed on a mixed data set (including pseudo-labels and supervoxel annotations), and then fine-tuning was performed on automatic segmentation and interactive correction tasks, and finally functional integration was achieved through joint training. In the end, the VISTA3D model achieved multiple technological leaps through core innovation.
First, the model was systematically validated on 14 international public datasets, covering 127 types of anatomical structures and pathological features.Its 3D automatic segmentation accuracy (Dice coefficient 0.91±0.05) is 8.3% higher than the traditional baseline model.It also supports click-to-interactive correction, reducing the time consumption of manual correction to 1/3 of the traditional method. Secondly, the first three-dimensional super-voxel feature transfer technology, by decoupling the spatial features of the two-dimensional pre-trained backbone network, achieves a 50% mIoU improvement in zero-sample tasks such as pancreas segmentation.The labeling efficiency is 2.7 times higher than that of supervised learning.In addition, the research team also constructed a cross-institutional multimodal dataset.While maintaining the annotation accuracy of 97.2%, the data annotation cost is compressed to 15% of full manual annotation.
Research progress on the integration of 3D medical imaging and AI in China
In recent years, with the widespread application of AI technology in the medical field, the combination of three-dimensional medical imaging technology and artificial intelligence has gradually become a research hotspot and has made significant progress in China, bringing new opportunities for medical diagnosis and treatment.
In 2023, the application of AI in medical imaging will mainly focus on auxiliary diagnosis. AI can quickly screen large data sets of images and patient information to improve diagnostic efficiency. For example, some AI-integrated imaging systems can detect tiny abnormalities that are difficult to identify with the naked eye, thereby improving the accuracy of diagnosis. In addition, AI can also retrieve previous imaging scans from the patient's electronic medical record and compare them with the latest scan results to provide doctors with more comprehensive diagnostic information. For example,Shanghai Jiao Tong University proposed a new working model PnPNet for 3D medical image segmentation.The problem of inter-class boundary confusion is solved by modeling the interaction dynamics between the intersecting boundary regions and their adjacent regions. The performance is SOTA, outperforming networks such as MedNeXt, Swin UNETR and nnUNet.
* Paper address:
https://arxiv.org/abs/2312.08323
Entering 2024, the integration of 3D medical imaging technology and AI will become closer, and the research directions will become more diversified. On the one hand, the application of AI technology in 3D reconstruction of medical images has gradually matured, and it can automatically perform 3D image segmentation and reconstruction, improving the accuracy and efficiency of image reconstruction. On the other hand, AI's ability in image analysis has also been further improved, which can assist doctors in disease diagnosis and treatment plan formulation. In addition, AI technology is also used in image post-processing, such as denoising, enhancement, and rendering, to improve the readability and aesthetics of the image. For example,West China Hospital of Sichuan University has innovatively developed the data-driven Chinese Lung Nodule Reporting and Data System (C-Lung-RADS) based on the Chinese population lung cancer screening cohort and lung nodule clinical cohort.Accurate grading and personalized management of the malignancy risk of lung nodules have been achieved.
* Paper address:
https://www.nature.com/articles/s41591-024-03211-3
By 2025, AI technology will be used more extensively and deeply in 3D medical imaging. For example,A research team from Peking University recently launched a "Renal Imaging Group Project" internationally.It is planned to take the lead in constructing a digital atlas of the entire kidney through multimodal imaging technology and artificial intelligence algorithms. This "digital kidney" can make the mechanism of kidney disease more clearly visible, and provide a new direction for accurate diagnosis, new drug development, and accurate treatment of kidney disease.
at the same time,A team from China University of Geosciences and Baidu jointly proposed a general framework called ConDSeg for contrast-driven medical image segmentation.This framework innovatively introduces a consistency reinforcement training strategy, a semantic information decoupling module, a contrast-driven feature aggregation module, and a size-aware decoder, thereby further improving the accuracy of the medical image segmentation model.
* Paper address:
https://arxiv.org/abs/2412.08345
In addition, Kunming University of Science and Technology and Ocean University of China proposed a bidirectional stepwise feature alignment (BSFA) method for unaligned medical image fusion. Compared with traditional methods, this study uses a single-stage method within a unified processing framework to simultaneously align and fuse unaligned multimodal medical images, which not only achieves the coordination of dual tasks, but also effectively reduces the problem of model complexity caused by the introduction of multiple independent feature encoders.
* Paper address:
https://doi.org/10.48550/arXiv.2412.08050
However, the research on combining 3D medical imaging technology with AI also faces some challenges. Issues such as data privacy, algorithm transparency, model generalization ability, and regulatory supervision are still key issues that need to be addressed. In the future, with the continuous advancement of technology and the improvement of regulations, these issues may be gradually resolved, thereby promoting the wider application of AI technology in the field of medical imaging.