Diffusion Model × Music Generation, DiffRhythm Completes Song Creation in Minutes! Low Threshold Deployment of Large Language Models, MiniMind Data Set Is Open Source
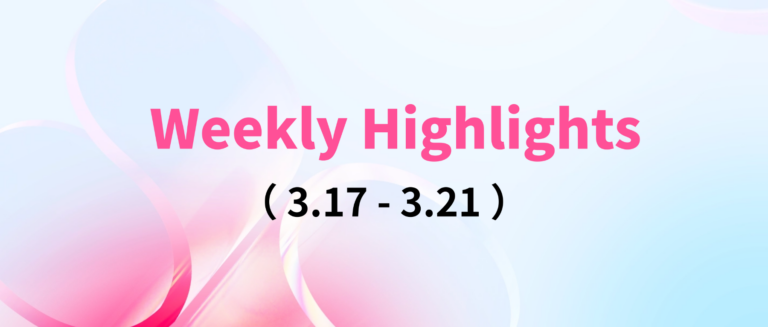
The field of music generation has made significant progress in recent years, but existing models still have many limitations in practical applications. Most models can only generate vocal or accompaniment tracks independently, resulting in an incoherent music experience. To address these challenges, the Audio, Speech and Language Processing Laboratory of Northwestern Polytechnical University and the Chinese University of Hong Kong jointly developed a model called DiffRhythm.
As the first open source complete song generation model based on diffusion technologyDiffRhythm not only maintains a high level of music generation and comprehensibility, but also ensures its scalability through a concise and effective model architecture and data processing flow. In terms of user experience, its non-autoregressive structure ensures a fast generation speed.Generate a complete music in just 1 minute.
At present, HyperAI has launched the "DiffRhythm: Generate a complete music demo in 1 minute" tutorial. Come and try it~
Online use:https://go.hyper.ai/sHdPu
From March 17 to March 21, hyper.ai official website updates:
* High-quality public datasets: 10
* Selected high-quality tutorials: 2
* Community Article Selection: 6 articles
* Popular encyclopedia entries: 5
* Top conferences with deadline in March: 1
Visit the official website:hyper.ai
Selected public datasets
1. KodCode-V1 coding synthesis dataset
This dataset is currently the largest fully synthetic open source dataset, providing verifiable solutions and tests for coding tasks. It contains 12 different subsets, covering various fields (from algorithms to software package-specific knowledge) and difficulty levels (from basic coding exercises to interviews and competitive programming challenges), and is designed for supervised fine-tuning (SFT) and RL tuning.
Direct use:https://go.hyper.ai/CfZCm
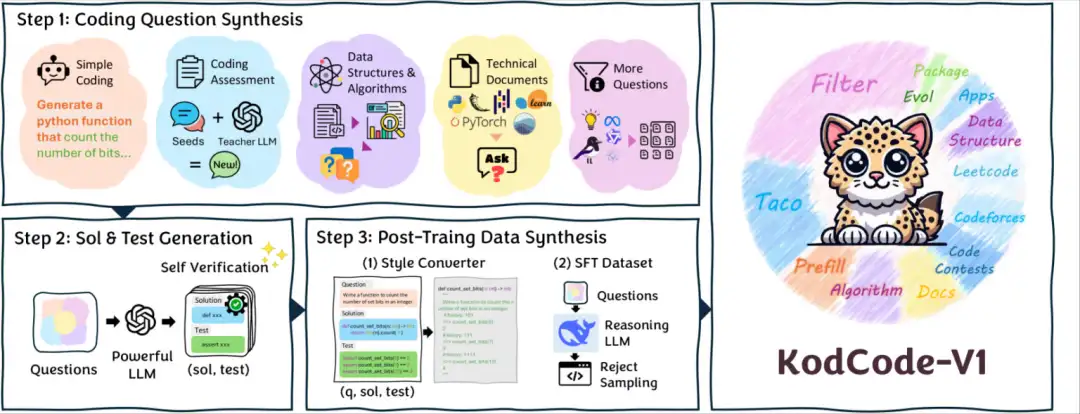
2. Road Hazards Road Hazard Dataset
This dataset contains 2.7k images and is mainly used to detect potholes, cracks, and open manholes on the road.
Direct use:https://go.hyper.ai/XPJNQ
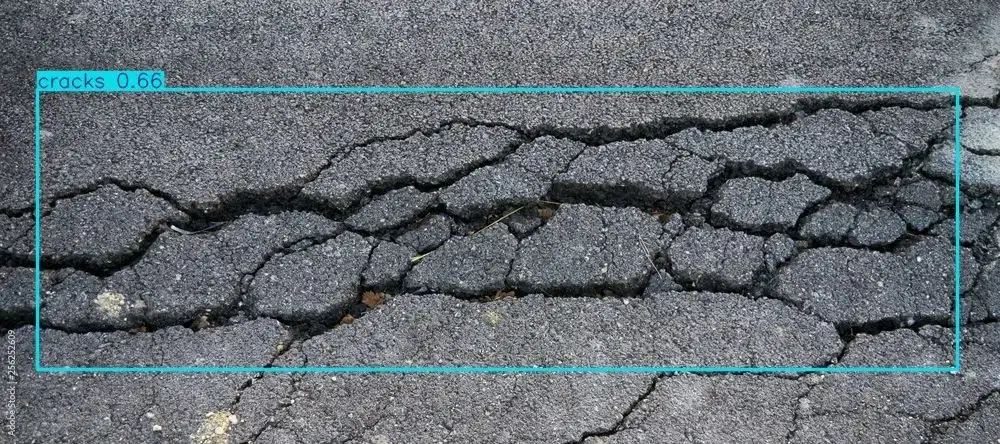
3. DexGraspVLA Robot Grasping Dataset
This is a small dataset containing 51 human demonstration data samples, which is used to understand the data and format, as well as run the code to experience the training process. Its research background stems from the need for high success rate of dexterous grasping in cluttered scenes, especially achieving a success rate of more than 90% under unseen objects, lighting and background combinations.
Direct use:https://go.hyper.ai/pJ44Y
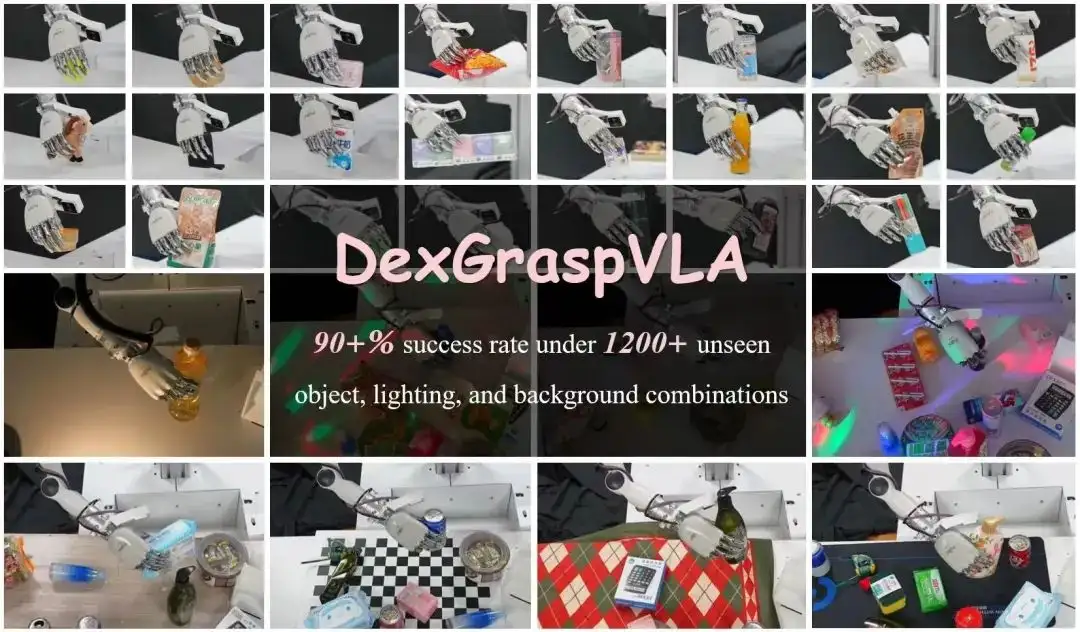
4. IllusionAnimals Visual Illusion VQA Dataset
The IllusionAnimals dataset is a FiftyOne dataset containing 2k samples. The dataset contains 10 animal categories and one no-illusion category. The image resolution is 512×512 pixels. It is used to evaluate the ability of multimodal models in identifying and explaining animal-based visual illusions.
Direct use:https://go.hyper.ai/Ebl40
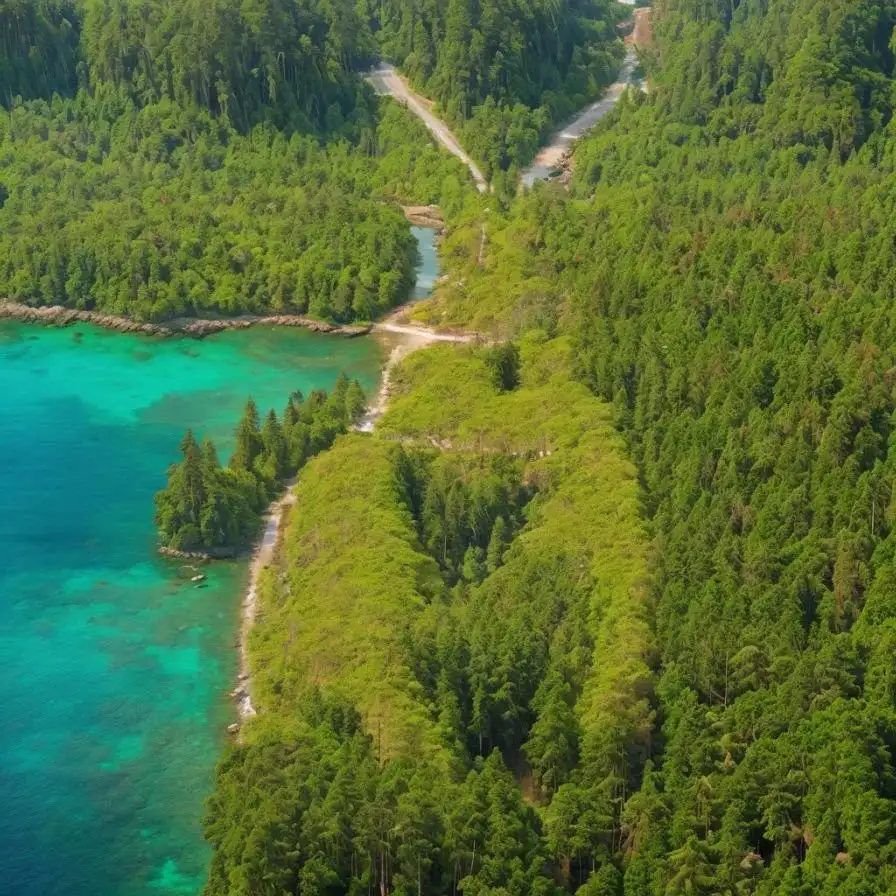
5. m-WildVision Multi-language Multi-modal Large Model Evaluation Dataset
The dataset contains 500 challenging user query examples in 23 languages, each of which is derived from the WildVision-Arena platform. The structure of the dataset includes question ID, language type, instruction text, and image data, and is designed to evaluate the generalization and robustness of the model in different languages.
Direct use:https://go.hyper.ai/Im6mN
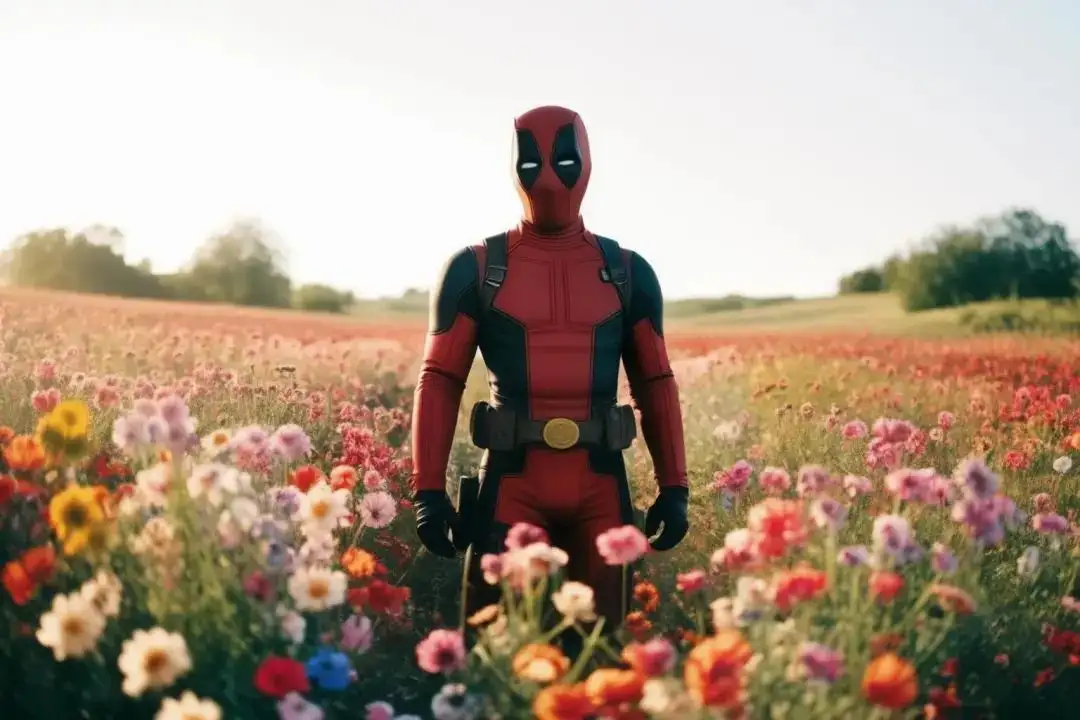
6. MiniMind large model training and fine-tuning dataset
MiniMind is an open source lightweight large language model project that aims to lower the threshold for using large language models (LLM) and enable individual users to quickly train and infer on ordinary devices.
Direct use:https://go.hyper.ai/gCz2y
7. Seaclear Marine Debris Detection and Segmentation Dataset
The dataset contains 8,610 marine debris images annotated for object detection and instance segmentation tasks, covering 40 object categories, including not only debris but also observed animals, plants, and robot parts. The annotations are provided as COCO format (.json) files, and the images are arranged in folders, each dedicated to a unique site-camera pair. All images are 1920×1080 resolution.
Direct use:https://go.hyper.ai/JFofd
8. Text and Audio Captchas Text and Audio Captchas Dataset
The dataset contains 100k CAPTCHA samples, each labeled with its corresponding alphanumeric string, which makes it ideal for training OCR models, speech recognition, and AI-based CAPTCHA solvers.
Direct use:https://go.hyper.ai/vFmTJ
9. Garbage Classification Dataset
The dataset contains images and YOLO-format annotations for classification and detection of different types of waste: plastic, paper and cardboard, glass/metal, organic, waste, textiles, and electronics (e-waste).
Direct use:https://go.hyper.ai/NwEF7
10. Mars surface image (Curiosity rover) Mars surface image dataset
The dataset consists of 6,691 images collected by three instruments of the Mars Science Laboratory (MSL, Mars Science Laboratory) (right eye Mastcam, left eye Mastcam and MAHLI), covering 24 categories. These images are "browsed" versions of each raw data product, not full resolution, each image is approximately 256×256 pixels.
Direct use:https://go.hyper.ai/B1T0l
Selected Public Tutorials
1. Deploy Gemma-3-27B-IT using vLLM
The Gemma series is a series of large models open sourced by Google, built on the same research and technology as the Gemini model. Gemma 3 is a multimodal large model that can process text and image inputs and generate text outputs. The model is suitable for a variety of text generation and image understanding tasks, including question answering, summarization, and reasoning. Their relatively small size enables them to be deployed in resource-limited environments, such as laptops, desktops, or cloud infrastructure.
The relevant models and dependencies of this project have been deployed. After starting the container, click the API address to enter the Web interface.
Run online:https://go.hyper.ai/JxVbA
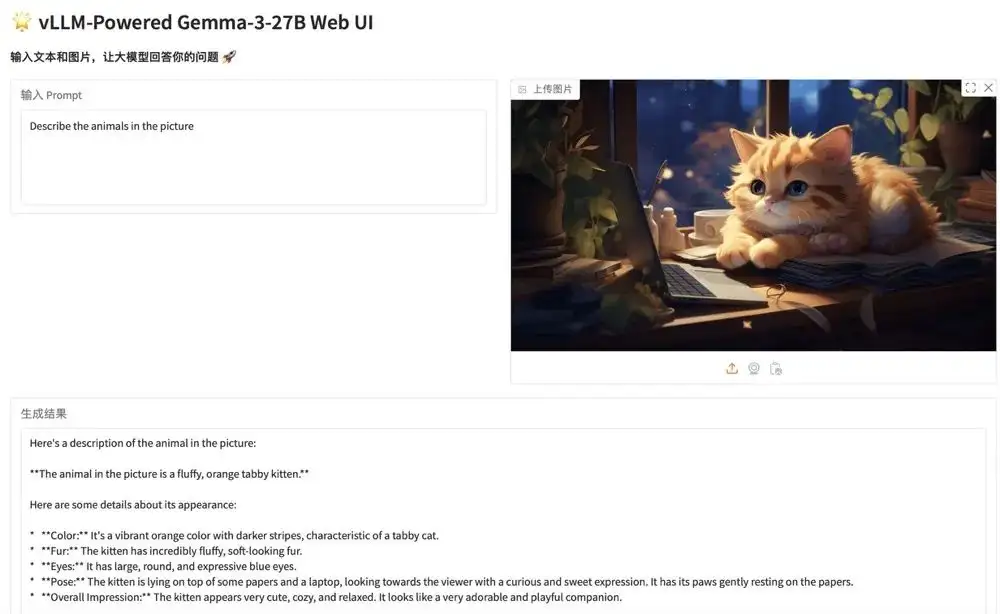
2. DiffRhythm: Generate a complete music demo in 1 minute
DiffRhythm is the first diffusion-based song generation model that can create complete songs. It can generate a complete song up to 4 minutes and 45 seconds long, including vocals and accompaniment, in a short time. Users only need to provide lyrics and style hints, and DiffRhythm can automatically generate melodies and accompaniment that match the lyrics, supporting multi-language input.
The relevant models and dependencies of this project have been deployed. After starting the container, click the API address to enter the Web interface.
Run online:https://go.hyper.ai/sHdPu
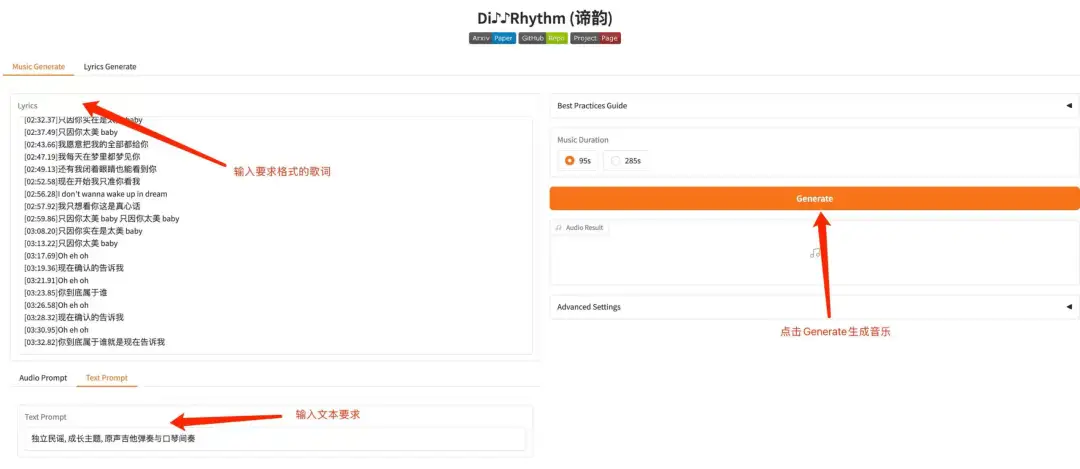
Community Articles
Academician Wu Lixin's team deeply integrated physical oceanography with AI, used ocean dynamics theory to drive the neural network architecture, and built a large global high-resolution ocean environment intelligent forecasting model "Wenhai", which better reflects the state of the real ocean and greatly saves computing time and energy consumption. This article is a detailed interpretation and sharing of the research.
View the full report:https://go.hyper.ai/s7YMj
A research team from the University of Cambridge proposed a virtual tissue model called Celcomen, which can not only estimate the impact of the environment on individual cells, but also infer the impact of individual cells on their surroundings and the overall tissue. The researchers verified the Celcomen model's identifiability in causal structure learning and unraveling causal relationships through self-consistent synthetic data and real-world data experiments, as well as its ability to unravel and recover gene-gene interactions in real and self-simulated spatial transcriptomics data. Related results have been selected for ICLR 2025. This article is a detailed interpretation and sharing of the research.
View the full report:https://go.hyper.ai/ylKOr
In the seventh Meet AI4S live broadcast, HyperAI invited Associate Professor Huang Hong from Huazhong University of Science and Technology, Dr. Zhou Dongzhan from Shanghai AI Lab, and Dr. Zhou Bingxin from Shanghai Jiaotong University Research Institute to discuss the cutting-edge development of AI in social sciences, physical chemistry, life sciences, and other fields with three scholars, and shared their insights on choosing research directions and their experience in submitting papers to top AI conferences. This article is a summary of the sharing of the three teachers.
View the full report:https://go.hyper.ai/klU6m
Nvidia CEO Huang Renxun gave a keynote speech at the GTC 2025 conference, the annual global AI event, focusing on the latest developments in the frontier field of AI. Not only did he showcase the Blackwell new generation of nuclear-grade AI chips, but he also launched a series of new achievements including the Physical AI dataset, GR00T N1 model, Newton physics engine, and Cosmos world model. This article is a summary of Huang Renxun's speech and new achievements.
View the full report:https://go.hyper.ai/Q6wdO
NVIDIA, in collaboration with MIT and others, has developed a new type of large-scale streaming protein backbone generator, Proteina. Proteina has five times the number of parameters of the RFdiffusion model, and has expanded the training data to 21 million synthetic protein structures. It has achieved SOTA performance in de novo protein backbone design, and has generated diverse and designable proteins with an unprecedented length of up to 800 residues. The results have been selected for ICLR 2025 Oral. This article is a detailed interpretation and sharing of the research.
View the full report:https://go.hyper.ai/w7jlU
Shanghai Jiao Tong University, in collaboration with a number of top institutions, has built an authoritative evaluation system to conduct systematic testing on 10 mainstream LLMs at home and abroad, including ChatGPT and DeepSeek, providing real-world evidence of AI-assisted primary care physician training for the first time, and providing key support for AI-enabled primary care. This article is a detailed interpretation and sharing of the research.
View the full report:https://go.hyper.ai/DH8hf
Popular Encyclopedia Articles
1. DALL-E
2. Reciprocal sorting fusion RRF
3. Pareto Front
4. Large-scale Multi-task Language Understanding (MMLU)
5. Contrastive Learning
Here are hundreds of AI-related terms compiled to help you understand "artificial intelligence" here:
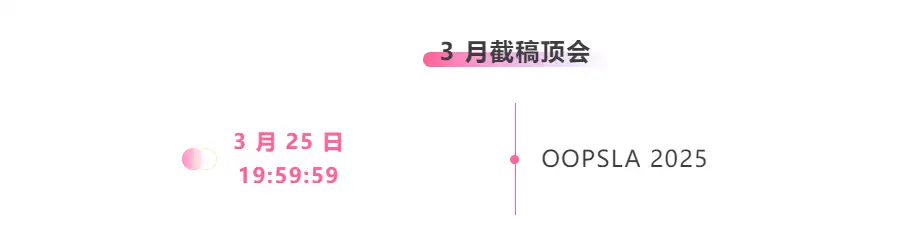
One-stop tracking of top AI academic conferences:https://go.hyper.ai/event
The above is all the content of this week’s editor’s selection. If you have resources that you want to include on the hyper.ai official website, you are also welcome to leave a message or submit an article to tell us!
See you next week!
About HyperAI
HyperAI (hyper.ai) is the leading artificial intelligence and high-performance computing community in China.We are committed to becoming the infrastructure in the field of data science in China and providing rich and high-quality public resources for domestic developers. So far, we have:
* Provide domestic accelerated download nodes for 1700+ public data sets
* Includes 500+ classic and popular online tutorials
* Interpretation of 200+ AI4Science paper cases
* Supports 600+ related terms search
* Hosting the first complete Apache TVM Chinese documentation in China
Visit the official website to start your learning journey: