AlphaFold Application New Milestone! Cambridge University Team Proposed AlphaFold-Metainference, Accurately Predicting Disordered Protein Structure Collections
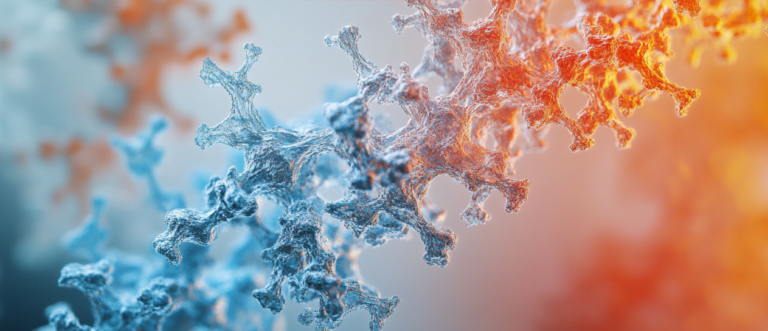
Since AlphaFold was launched at the end of 2018, the field of protein structure prediction has undergone tremendous changes with the help of AI. Today, AlphaFold is not only impressive in terms of prediction accuracy, but also gradually broadens the prediction range in the latest iteration. No wonder Shi Yigong, an academician of the Chinese Academy of Sciences, once commented on it in front of the media, "In my opinion, this is the greatest contribution of artificial intelligence to the field of science, and it is also one of the most important scientific breakthroughs made by mankind in the 21st century. It is a very remarkable historical achievement in mankind's scientific exploration of the natural world."
Although the protein structure prediction revolution led by AlphaFold is so rapid, there are still some unresolved issues ahead. Among them, the study of disordered proteins has always been a difficult problem in the field of life sciences. These proteins play a key role in cell signaling, regulatory processes, and a variety of diseases.However, due to their special heterogeneity and dynamics in structure, they cannot be represented by a single structure.Therefore, its research has not made as much progress as the prediction of ordered protein structures. However, the success of AlphaFold has pointed out new ways of solving problems for scientists.
Recently, a research team from the University of Cambridge published a new study and proposed a method called AlphaFold-Metainference.This method uses the correlation between the predicted aligned error (PAE) map predicted by AlphaFold and the distance change matrix in the molecular dynamics (MD) simulation to construct structural ensembles of disordered proteins and proteins containing disordered regions.It provides new ideas for the prediction of disordered protein structures based on deep learning methods, and also further broadens the scope of application of AlphaFold.
Currently, the relevant research results have been published in the international academic journal Nature Communications under the title "AlphaFold prediction of structural ensembles of disordered proteins".
Research highlights:
* Breaking through the prediction limitations and achieving high-precision prediction. The study confirmed that AlphaFold can accurately predict the distance between residues even if it is not trained on disordered protein data.
* Innovative prediction method to construct a structural collection. This method uses the distance predicted by AlphaFold as a structural constraint, combined with a meta-reasoning framework and molecular dynamics simulation to construct a structural collection of disordered proteins and proteins containing disordered regions.
* Deepen the deep learning method and expand the application boundaries. This method performs well in dealing with highly disordered and partially disordered proteins. The generated structure set is significantly more consistent with experimental data than a single AlphaFold structure, effectively solving the problem of disordered protein structure prediction.
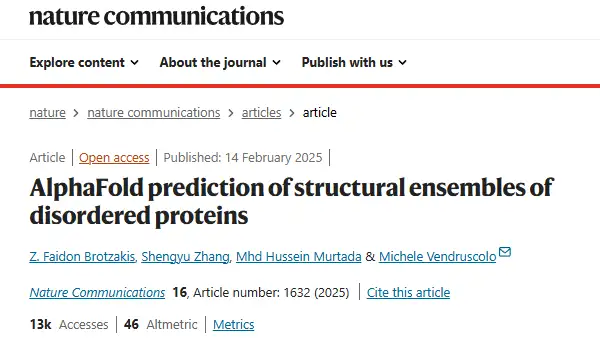
Paper address:
https://www.nature.com/articles/s41467-025-56572-9
The open source project "awesome-ai4s" brings together more than 200 AI4S paper interpretations and provides massive data sets and tools:
https://github.com/hyperai/awesome-ai4s
Dataset: Rigorous verification of multi-source data
In terms of training deep learning models, since the structural collections of disordered proteins are very low in number and accuracy, but disordered proteins can be predicted based on the available information of ordered proteins, the researchers used a large number of high-resolution folded protein structures in the Protein Data Bank (PDB) to train deep learning models.
In terms of experimental data comparison, it is challenging to obtain experimental information on the distances between residues in disordered proteins, and the data labels themselves may affect the properties of the conformational ensemble.To do this, the researchers used small-angle X-ray scattering (SAXS) data and nuclear magnetic resonance (NMR) diffusion measurements.It provides label-free information on the distance distribution between disordered protein residues for research, which is used to compare and verify the prediction results.
In addition, in further verification,The researchers also analyzed the structural ensemble data of Aβ and α-synuclein obtained through all-atom molecular dynamics simulations and coarse-grained simulations using CALVADOS-2 (C2).This further verifies the accuracy of AlphaFold's predicted distance.
Model architecture: innovative fusion meta-reasoning method
The AlphaFold-Metainference method described in this study is used to generate a collection of structures representing the native states of disordered proteins and proteins containing disordered regions.
The core of this method is based on the observation that the inter-residue distances predicted by AlphaFold are relatively accurate even for disordered proteins and can therefore be used as structural constraints in molecular dynamics simulations within a meta-inference framework. In simple terms, to generate a set of structures, AlphaFold-Metainference uses the predicted distances as structural constraints in molecular dynamics simulations.Convert AlphaFold distance maps (distograms) to structure sets.
First, AlphaFold predicts the distance. The researchers used AlphaFold's distance map to predict the average distance between residues, and calculated the predicted distance and standard deviation through a specific formula. Then, multiple sequence alignment was performed based on MMseqs2, and the AlphaFold 1.1.1 model with default settings was used for prediction, without using a structural template. AlphaFold outputs the distances between residues distributed into 64 bins of equal width, ranging from 2.15625 to 21.84375 Å, and the last bin also includes distances exceeding 21.84375 Å.
Then, we combine the meta-reasoning method. The so-called meta-reasoning is a Bayesian reasoning method that can determine the structure set by combining prior information and experimental data based on the maximum entropy principle.The researchers used the distance graph predicted by AlphaFold as pseudo-experimental data and applied the Bayesian meta-inference method.Determine the structural ensemble by separating structural heterogeneity from systematic errors, such as inaccuracies in the force field or forward model, random errors in the data, and errors due to limited sample size in the ensemble.
In molecular dynamics simulations, calculations are performed based on the meta-inference energy function, and error parameters are determined through multiple replica simulations and Gibbs sampling.Finally, the CALVADOS-2 force field was used to perform a coarse-grained simulation.Implement AlphaFold-Metainference.
The last step is distance constraint selection. In this stage, the distance predicted by AlphaFold is filtered based on the distance probability and the predicted alignment error.The selection criteria were determined by combining protein hydrophilicity and predicted local distance difference test (pLDDT) scores.It is worth noting that the experimental use of pLDDT scores to select residue distances in structured regions does not exclude their use as distance constraints to optimize the generation of structure ensembles.
All molecular dynamics simulations started from the structure predicted by AlphaFold and were performed under the NVT ensemble. Six replicas were set up for each simulation, and each replica ran for 1 million steps, starting the simulation from different initial positions obtained in the energy minimization step.The simulation uses a Langevin integrator.The time step is 5 fs, the friction coefficient is 0.01 ps⁻¹, and a Cα-based model with CALVADOS-2 parameters and functional form is used.
Among them, for highly disordered and partially disordered proteins, PULCHRA was used to convert all structures in the coarse-grained collection into all-atom representations, and then GROMACS was used for energy minimization to obtain more accurate structures.
In summary, the results presented by the researchers illustrate how to use deep learning methods originally developed for predicting the native state of folded proteins to generate a collection of structures representing the native state of disordered proteins. This method greatly expands the scope of protein structure prediction based on deep learning and provides a new idea for predicting the structure of disordered proteins.
Experimental results: fully verify its rationality
In terms of AlphaFold prediction accuracy
The researchers compared a set of 11 proteins for which both SAXS and NMR diffusion measurements were available, and found good agreement between the distance distributions predicted by AlphaFold and the SAXS-derived distance distributions. The researchers also added a folded protein as a control, as shown in the figure below.
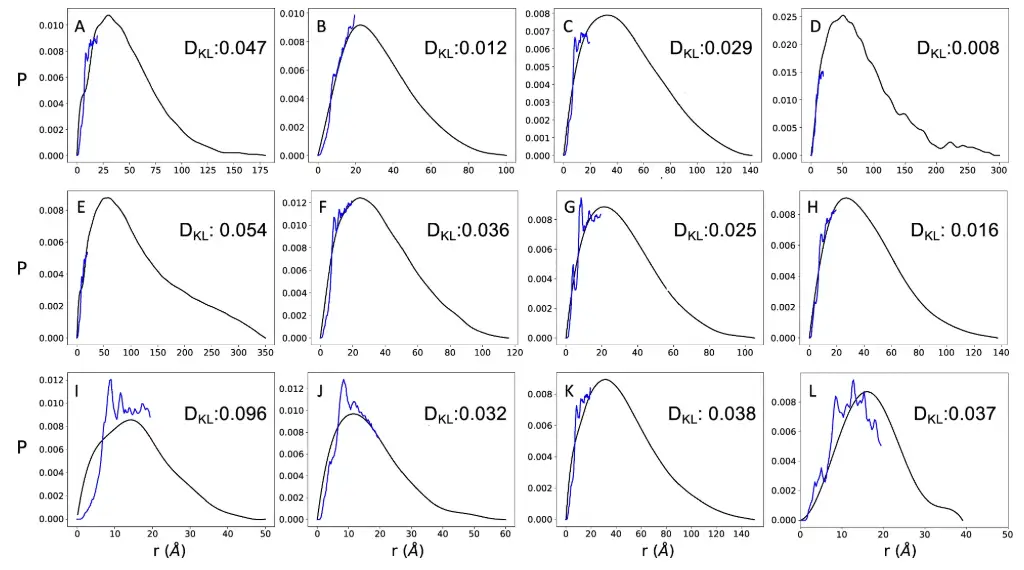
It is worth mentioning that the distance distribution predicted by AlphaFold does not cover the entire SAXA-derived distribution, since the maximum distance predicted by AlphaFold is about 22 Å. The results showed that the DKL value of the added control group was 0.037, which is comparable to the DKL values of 11 highly disordered proteins (DKL range of 0.008-0.096).This further demonstrates that AlphaFold has comparable accuracy in predicting inter-residue distances for disordered and ordered proteins.
In addition, the distances predicted by AlphaFold also have good agreement with the distances back-calculated from the MD ensembles of Aβ and α-synuclein and from the CALVADOS-2 ensemble.
In the verification of highly disordered structure collections
The researchers compared the experimentally obtained distance distributions, which can be calculated using small-angle X-ray scattering measurements, with those obtained from a collection of structures determined by AlphaFold-Metainference simulations for the same 11 highly disordered proteins.
For further comparison, the researchers also showed the distance distribution obtained using CALVADOS-2 and the AlphaFold-derived distance distribution generated directly from a single AlphaFold structure. To provide a quantitative comparison, the researchers found that the ensemble of structures provided by AlphaFold-Metainference and CALVADOS-2 was more consistent with the SAXS data than the single AlphaFold-derived structure.
The researchers further compared the structural ensembles using NMR chemical shifts, which were back-calculated at each time step using CamShift.The results show that in some cases AlphaFold-Metainference's predictions are more accurate.As shown in the figure below.
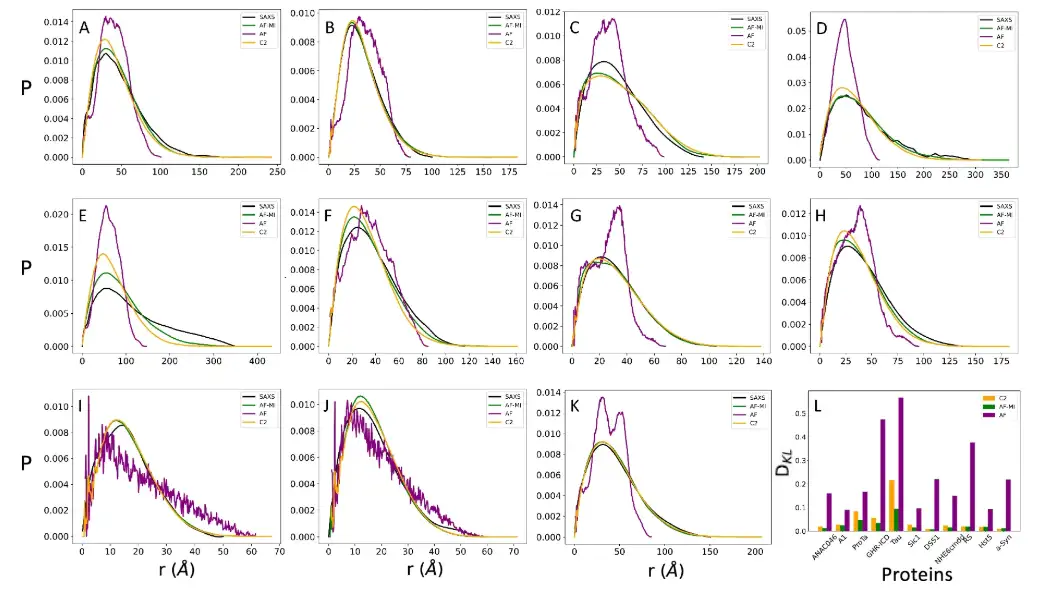
* The distribution of experimental pairwise distances obtained by SAXS is shown as a black line
* AlphaFold single structure prediction is represented by purple line
* AlphaFold-Metainference structure ensemble predictions are represented by green lines
* The pairwise distance distribution obtained by CALVADOS-2 is shown as an orange line
In the verification of partially disordered structured collections
The researchers prepared a set of six proteins with both ordered and disordered domains, with different sequence lengths and for which SAXS data were available for verification.
First is TDP-43, a multifunctional RNA-binding protein with a modular structure that participates in a variety of cellular processes, including transcription, pre-mRNA splicing, and mRNA stability regulation, which has been implicated in ALS and other neurodegenerative diseases.
The experimental results found that when applying the researchers’ filtering criteria to select the distances predicted by AlphaFold, and then applying AlphaFold-Metainference with these distance constraints,The obtained structural ensemble is in significantly better agreement with the SAXS data.The DKL value is only 0.018.This is better than the DKL value of 0.582 when using the AlphaFold predicted structure directly with SAXS data.As shown in the figure below.
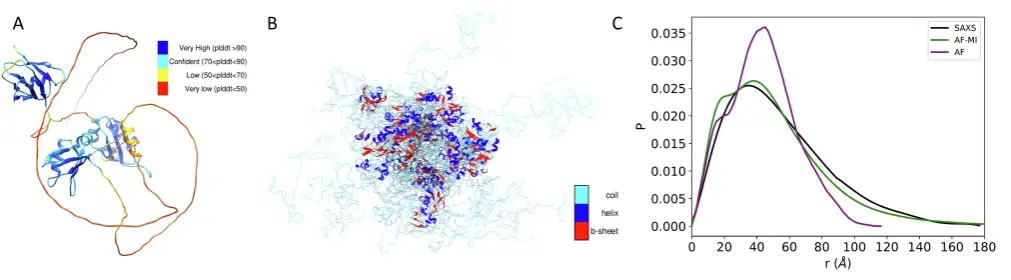
The researchers then went on to analyze ataxin-3 and human prion protein. For the former, similar results were obtained as for TDP-43 above. The predicted structure obtained using AlphaFold directly from the AlphaFold protein structure database was poorly consistent with the SAXS data, with a DKL value of 0.653. However, when a filtering criterion was applied to select the AlphaFold predicted distances for the AlphaFold-Metainference simulation,A set of structures that are more consistent with the SAXS data was obtained.The DKL value is only 0.020. As shown in the figure below.
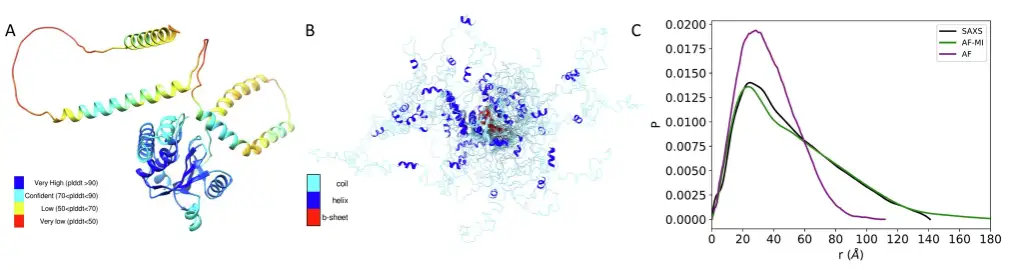
For the latter, the predicted structure obtained directly from the AlphaFold protein structure database using AlphaFold is poorly consistent with the SAXS data, with a DKL value of 0.1,When filtering criteria were applied, a set of structures was obtained that was more consistent with the SAXS data.The DKL value is only 0.053. As shown in the figure below.
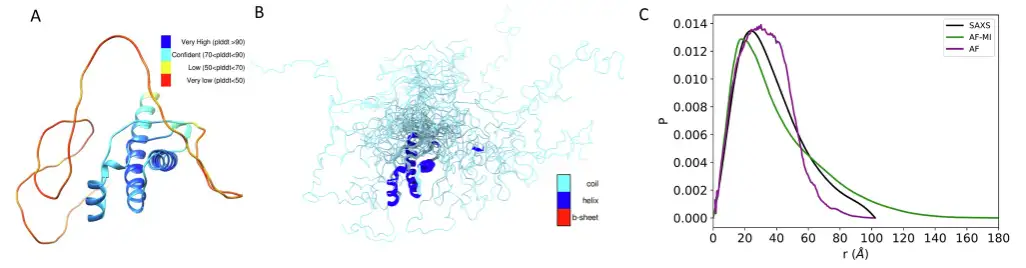
In addition, the researchers also studied three other proteins, CbpD, H16 and PC, and the results showed thatIn all cases, the agreement between the experimental and back-calculated inter-residue distance distributions is very good.And it is a significant improvement over the AlphaFold single structure obtained directly from the AlphaFold protein structure database, as shown in Figure D below.
Finally, in comparison with the CALVADOS-2 method, AlphaFold-Metainference performed better in four of the six proteins (ataxin-3, CbpD, H16, and PC), and produced comparable structural ensembles in the remaining two (TDP-43 and human prion protein), as shown in the figure below.
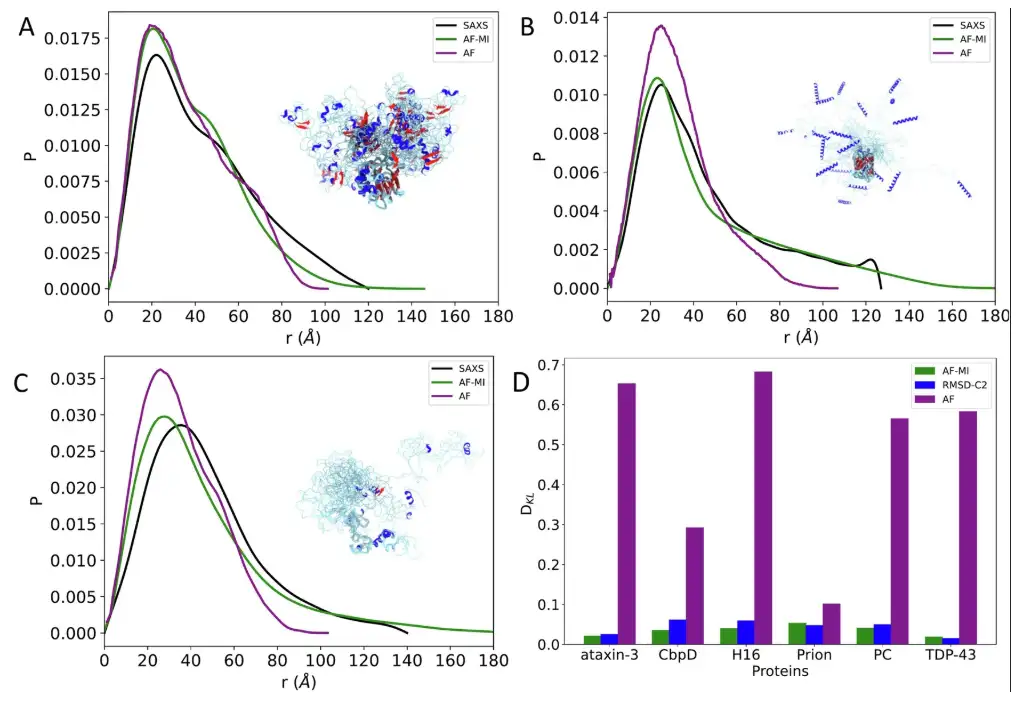
Progress in prediction of disordered proteins based on deep learning
In the past few years, AlphaFold has been mainly used to predict the static structure of folded proteins, which has also caused it to be criticized by the scientific research community. This study undoubtedly confirms that it also has potential application advantages in the prediction of disordered protein structures, and also provides a new research direction for the prediction of disordered protein structures.
In fact, with the close integration of AI and life sciences,There have been many discussions on the prediction of disordered protein structures.Using AI to reveal the mysteries of life has also become a mainstream method in the field of modern life sciences.
For example, an article previously published in Current Opinion in Structural Biology discussed the application progress of deep learning in the research of intrinsically disordered proteins (IDPs), and explained its role in promoting disordered protein prediction and conformational ensemble characterization.
The related research was published under the title “Deep learning for intrinsically disordered proteins: From improved predictions to deciphering conformational ensembles”.
* Paper address:
https://www.sciencedirect.com/science/article/pii/S0959440X24001775
Coincidentally, a research team from the University of Copenhagen in Denmark published an article on disordered protein research in Nature titled "Conformational ensembles of the human intrinsically disordered proteome". The article discussed the use of various deep learning methods to predict disordered regions, conformational ensembles and related properties of IDPs, including deep learning methods such as AlphaFold mentioned above, as well as protein language models, generative adversarial networks, etc.
*Paper address:
https://www.nature.com/articles/s41586-023-07004-5
There is no doubt that the rapid development of AI is accelerating our understanding of the true meaning of life. It took British scientist John Kendrew 12 years to explore the first protein structure using X-ray crystallography. Now AlphaFold only needs a few years to crack the mystery of the folding of hundreds of millions of proteins. In the future, who can assert that we cannot master the prediction of disordered protein structures?