Computational Efficiency Increased by 3,000 Times! Laoshan Laboratory and Others Proposed a Large-scale Intelligent Forecast Model for the Ocean Environment, "Wenhai", Which Performs Better Than Numerical Ocean Forecasting
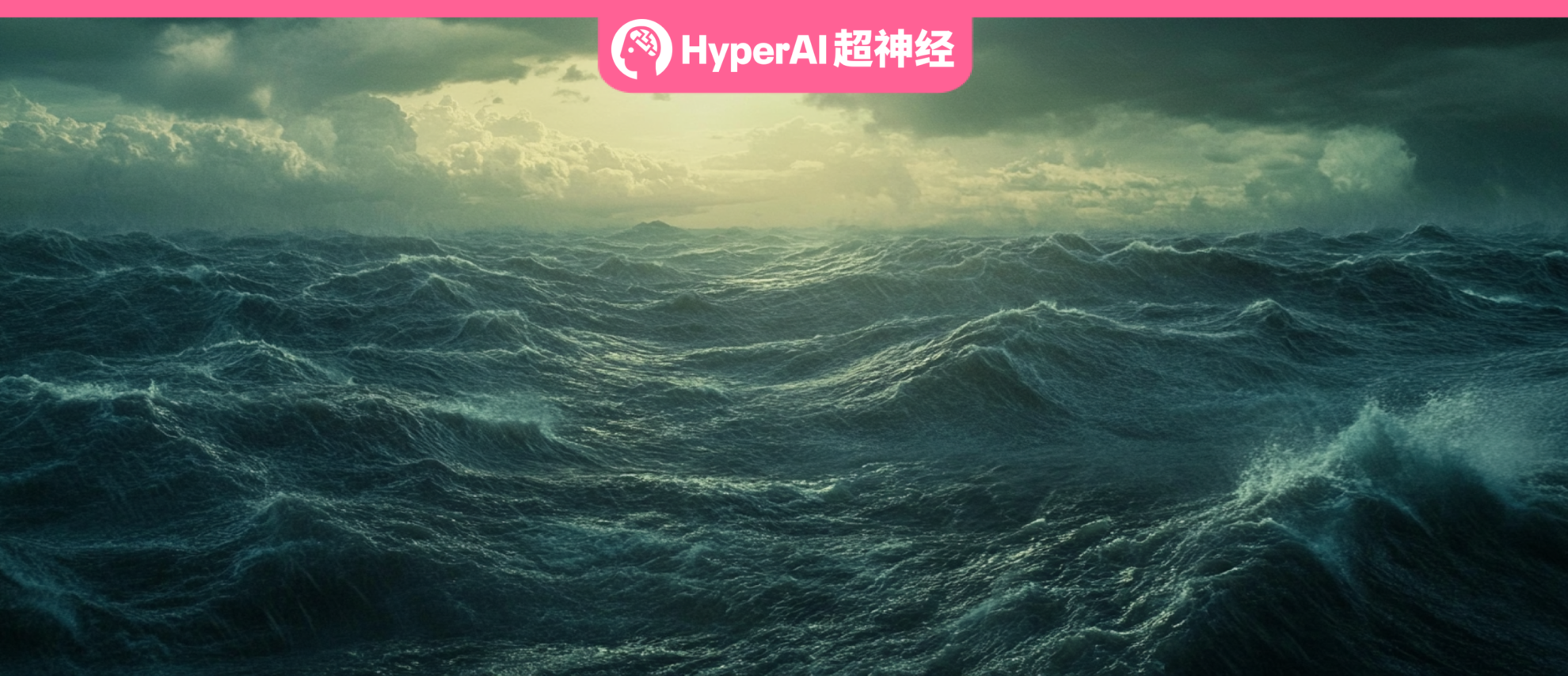
There are eddies of various time and space scales active in the ocean.Mesoscale eddies with spatial scales ranging from 10km to 100km are the main carriers of ocean kinetic energy.The mesoscale eddies dominate short-term changes in ocean velocity, temperature, and salinity, and have important impacts on atmospheric processes. Therefore, accurate prediction of mesoscale eddies is not only crucial for ocean activities and management, but also helps improve the accuracy of weather forecasts.
In the past, oceanographers mainly predicted future ocean changes by numerically solving large sets of physical equations. In the process of numerical solution, as the grid resolution increases, the computational burden increases rapidly.This results in huge computing resources being required to predict ocean mesoscale eddies.In recent years, artificial intelligence (AI) has brought new methods and powerful tools to scientific research and has made important progress in weather forecasting.The existing AI large models still have problems such as insufficient representation of sea-air interactions and fuzzy forecast results.This poses a challenge to achieving refined marine environment forecasts.
In response to this, the research team led by Academician Wu Lixin of Laoshan Laboratory, in collaboration with Ocean University of China, University of Science and Technology of China, and Qingdao Guoshi Technology Group Co., Ltd., deeply integrated physical oceanography with artificial intelligence, and used the theory of ocean dynamics to drive the design of neural network architecture.We developed "Ask the Sea" - a global high-resolution (1/12°) ocean environment intelligent forecasting model.
The relevant research results were published online in Nature Communications under the title "Forecasting the Eddying Ocean with a Deep Neural Network".
Research highlights:
* The “Ask the Sea” model uses ocean dynamics theory to drive the neural network architecture design
* The forecast performance of the "Wenhai" large model is better than the numerical forecast system released by the Mercator International Center for Oceanography in France
* The "Wenhai" large model has improved computational efficiency by 3,000 times compared to the numerical forecast model
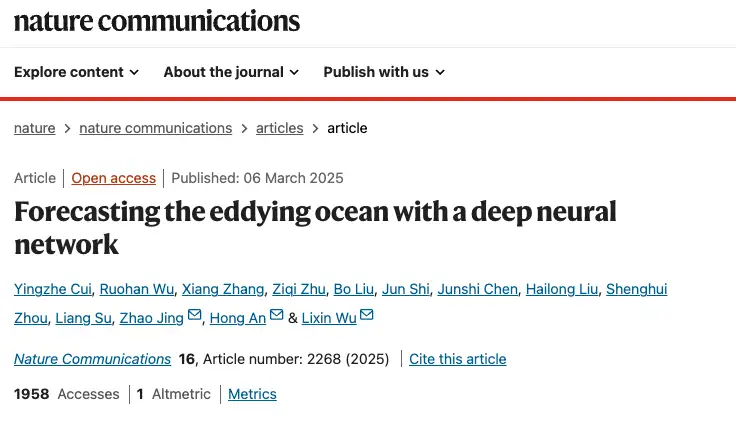
Paper address:
https://www.nature.com/articles/s41467-025-57389-2
The open source project "awesome-ai4s" brings together more than 200 AI4S paper interpretations and provides massive data sets and tools:
https://github.com/hyperai/awesome-ai4s
Dataset: Using ocean observation data as ground truth
The data used in this study mainly include ocean and atmospheric reanalysis data for training models, as well as observational data for evaluating model forecasting capabilities. The reanalysis data include the GLORYS global 1/12° ocean reanalysis data from the Mercator International Center for Oceanography in France, and the ERA5 global 1/4° atmospheric reanalysis data from the European Center for Medium-Range Weather Forecasts.
Different from the existing AI meteorological models, which basically use reanalysis data as the initial field for forecasting,This study strictly uses the initial field and forcing field consistent with the ocean numerical prediction system to drive the large model, and uses the ocean observation data as the true value.Objectively evaluate the actual forecasting capabilities of the ocean model and the ocean numerical forecasting system.
The initial ocean field comes from the GLO12v4 forecast system of the Mercator International Centre for Oceanography in France, and the atmospheric forecast field comes from the IFS HRES forecast system of the European Centre for Medium-Range Weather Forecasts. The observation data include the temperature-salinity profile measured by Argo, the sea surface height measured by satellite remote sensing, and the sea surface temperature and near-shore surface current velocity measured by drifting buoys.
Model framework: Ocean dynamics theory drives neural network architecture design
The existing AI big models have problems such as insufficient representation of sea-air interaction and fuzzy forecast results, which poses challenges to the realization of refined marine environmental forecasts. Through the deep integration of physical oceanography and artificial intelligence, the neural network architecture design is driven by ocean dynamics theory, which effectively overcomes the shortcomings of the existing AI big models.
The "Wenhai" model explicitly embeds the bulk formula into the neural network.It accurately characterizes the momentum, heat and material exchange between the sea and the air; uses the sea and land distribution mask to give the model the ability to perceive the terrain and coastal boundaries; takes the changing trend of the ocean state as the forecast target, so that the model pays more attention to fast-changing small and medium-scale processes; reduces the information loss in the downsampling process by optimizing the neural network hyperparameters, and enhances the fidelity of small and medium-scale processes in the ocean; uses fine-tuning technology to improve the cumulative error in the iterative forecasting process.
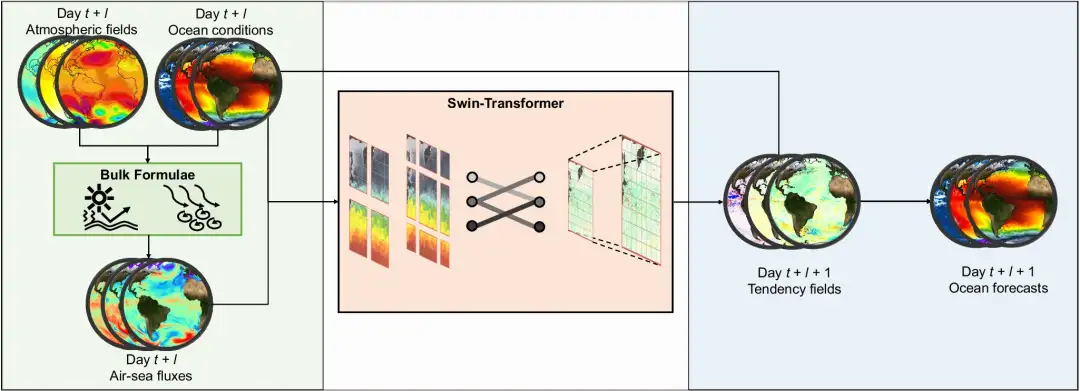
Experimental conclusion: The forecasting ability of the "Wenhai" large model is better than that of the numerical ocean forecasting system
The small-scale processes in the ocean are highly nonlinear and have low predictability. In addition, ocean observations are sparse, and the initial field given by the assimilation system deviates from the actual ocean state.It is difficult to require that the predicted small and medium-scale processes are completely consistent with the actual observations in terms of location and intensity.In particular, due to the existence of the "double penalty" problem, traditional point-to-point error indicators, such as the root mean square error (RMSE), are not suitable for evaluating the performance of high-resolution forecast systems, because such indicators will make the performance of high-resolution forecast systems "look" worse than that of low-resolution systems, even though the former can better reflect the real state of the ocean.
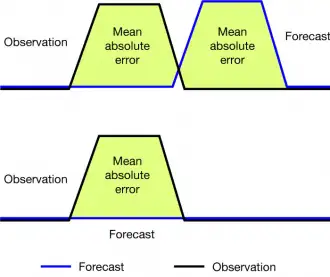
In order to fairly compare the "Wenhai" large model with the GLO12v4 numerical forecast system, this study adopted the "neighborhood evaluation" scheme for high-resolution forecast systems.The forecast values within a certain range around the observed value are taken as the ensemble forecast of the observed location, and their continuous ranked probability scores (CRPS) are evaluated. The return results from April to November 2024 show that the forecast performance of the "Ask the Sea" model for the next 10 days of temperature, salinity, current velocity, and sea level height is better than that of the GLO12v4 numerical forecast system.The "Wenhai" large model has improved its computational efficiency by 3,000 times compared to the numerical forecast model.It greatly saves computing time and energy consumption.
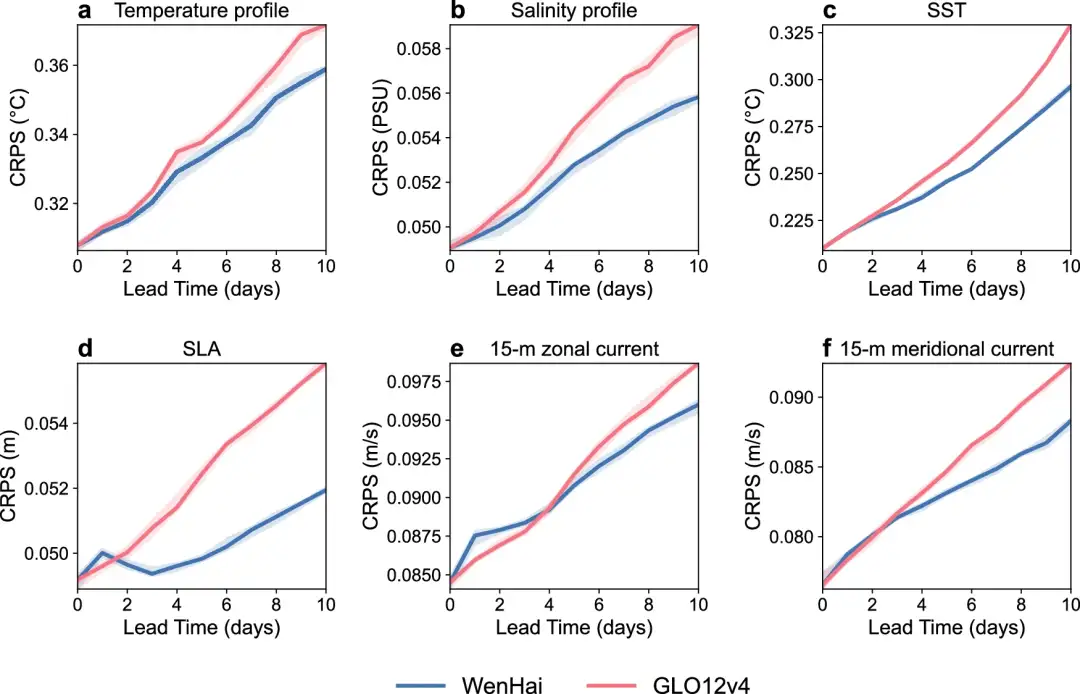
The continuous ranked probability score (CRPS, the lower the better) of the forecast results of the "Ask the Sea" large model (blue line) and the GLO12v4 numerical prediction system of the Mercator International Center for Oceanography in France (red line) from April to November 2024.
(a) Temperature profile
(b) Salinity profile
(c) Sea surface temperature
(d) Sea level anomaly
(e) 15 m zonal velocity and
(f) 15 m meridional velocity. The temperature-salinity profile shows the vertically averaged CRPS. The shading indicates the 50% confidence interval obtained using the bootstrap method.