David Baker's Team's New Achievement! RFdiffusion Evolves Again to Achieve Atomic-level Precision Antibody De Novo Design
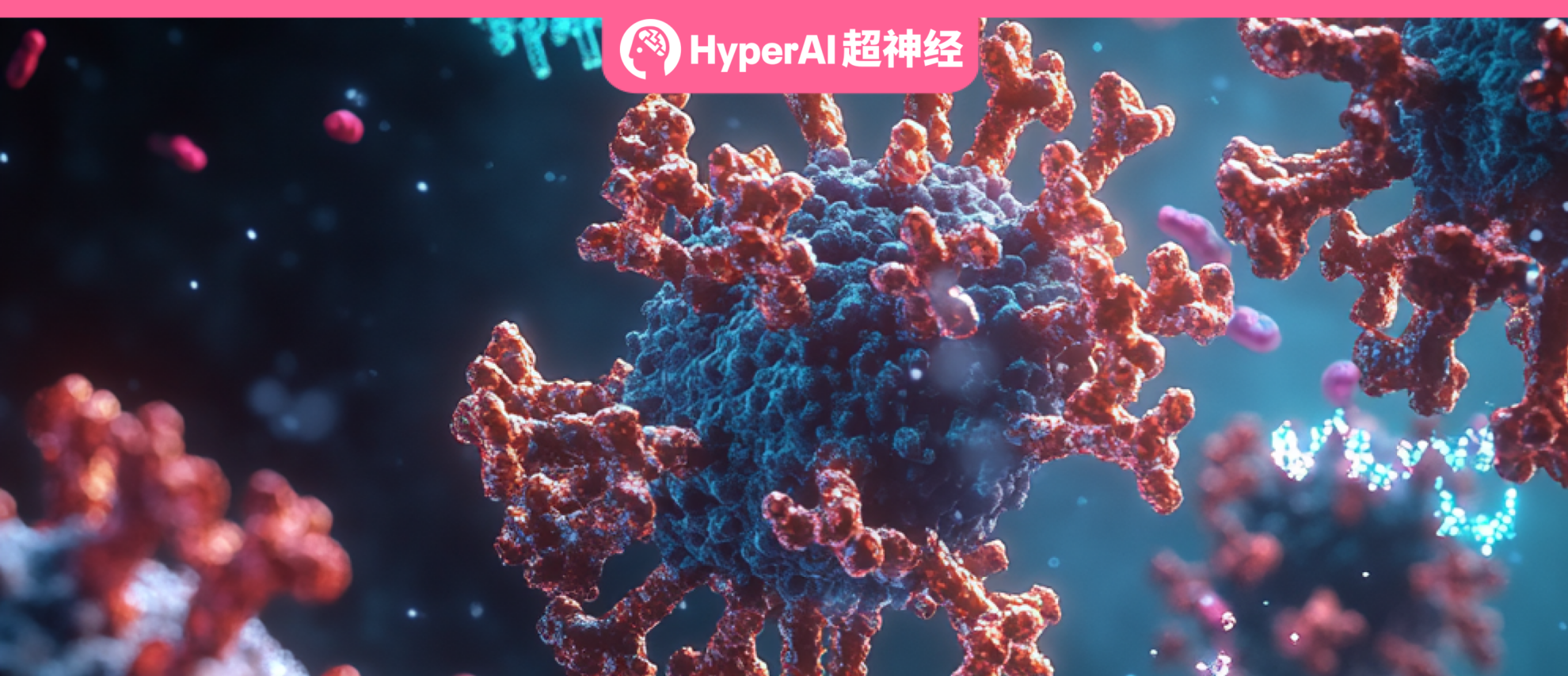
At the end of the 19th century, German bacteriologist Emil Adolf von Behring conducted in-depth research on diphtheria toxin. At that time, diphtheria was like the scythe of the god of death, ruthlessly taking the lives of many children. In the experiment, Behring injected rabbits with trace amounts of diphtheria toxin, wanting to observe their reactions and find ways to fight the toxin. A few days later, he was surprised to find that these rabbits not only did not die, but also developed resistance to subsequent injections of diphtheria toxin. Further research found that a substance that can neutralize diphtheria toxin appeared in rabbit serum.This was the first time that humans discovered the existence of antibodies.This accidental discovery opened the curtain for the application of antibody research and demonstrated the great potential of using the body's own power to fight disease.
After more than a century of in-depth exploration, scientists have continued to make efforts in the field of antibody research, but have not yet developed an efficient path to generate new antibodies targeting specific epitopes that completely relies on computer design.The discovery of therapeutic antibodies is still mainly mired in two traditional methods: animal immunization or random library screening.In the face of increasingly complex disease challenges, the limitations of these traditional methods have become increasingly prominent, and new breakthroughs are urgently needed to achieve more efficient and precise design of antibodies against specific targets, thus bringing new hope to antibody research.
To solve the problem of antibody discovery relying on traditional methods, the team of David Baker, professor of biochemistry at the University of Washington, and his collaborators combined the fine-tuned RFdiffusion network computational protein design with yeast display screening.We have successfully generated antibody variable heavy chain VHHs and single-chain variable fragment scFvs that can bind to specific epitopes with atomic-level precision.The feasibility of de novo design of antibody domains was demonstrated. This method provides a rational framework for computational design, screening, isolation, and characterization of de novo designed antibodies, achieving atomic-level precision in structure and epitope targeting.
The relevant research results have been published as a preprint on bioRxiv under the title "Atomically accurate de novo design of antibodies with RFdiffusion".
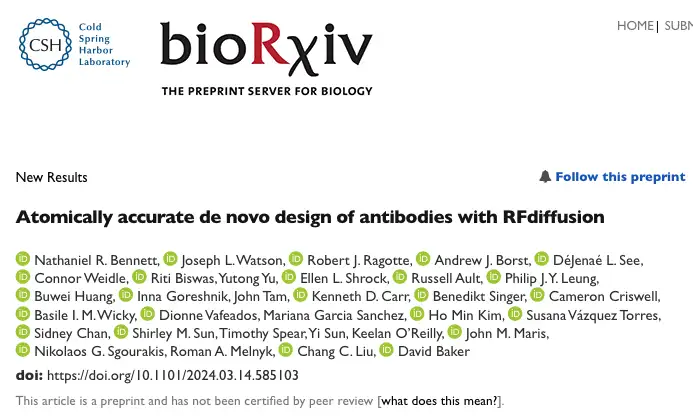
Paper address:
https://doi.org/10.1101/2024.03.14.585103
The open source project "awesome-ai4s" brings together more than 200 AI4S paper interpretations and provides massive data sets and tools:
https://github.com/hyperai/awesome-ai4s
Technological innovation in antibody research: breakthroughs and challenges of AI
As the leading category of protein therapeutic drugs, antibodies have become the core treatment for tumors, autoimmune diseases and other fields due to their high specificity and low side effects. As of 2025, more than 160 antibody drugs have been approved by regulatory agencies worldwide, and their market size has grown at an average annual rate of 15% in the past 10 years.It is expected to reach US$445 billion in the next five years.
However,Traditional antibody development technology has long relied on animal immunization and random library screening.Faced with significant bottlenecks. Animal immunization requires multiple antigen injections to stimulate the animal immune system to produce antibodies.The process usually takes 6-12 months.Furthermore, due to the differences in individual animal immune responses, it is difficult to obtain highly effective antibodies against complex antigens such as membrane proteins. Although random library screening methods (such as phage display technology) can expand the screening scope, they are difficult to stimulate immune responses to antigens, such as unfolded proteins or glycosylated epitopes, and the affinity of the screened antibodies is generally low.
In order to break through the limitations of traditional technologies, computational design and artificial intelligence have gradually become new directions in antibody research and development. Early studies successfully improved antibody performance by embedding residues into existing antibody frameworks, optimizing the conformation of the complementary determining region (CDR loop), and combining the Rosetta algorithm to transform the interaction interface. For example,A 2018 study increased the affinity of anti-PD-1 antibodies by 20 times through computational design.
In recent years, deep learning technology has further promoted the generation of antibody sequences. In 2023, a team from Stanford University used neural networks to design broad-spectrum neutralizing antibodies against new coronavirus variants, and their in vitro activity was 3 times higher than that of traditional methods.The RFdiffusion model developed by David Baker's team enables de novo design of binding proteins without the need for a pre-set backbone structure.This technology simulates the physical process of protein folding to generate a binding interface that is highly complementary to the shape of the target epitope, and has successfully designed a new inhibitor against influenza virus hemagglutinin. However, this research is only applicable to epitopes with regular secondary structures, such as α-helices and β-folds, while antibodies usually rely on complex ring structures (such as CDR-H3) to achieve binding, which leads to challenges in its direct application in antibody design.
Designing structurally precise antibodies from scratch, that is, with no homology to known antibodies, remains an unsolved problem, with core challenges including insufficient dynamic conformational simulations, lack of high-quality data, and long experimental verification cycles.
The binding process of antibodies involves conformational changes in flexible CDR loops, and existing algorithms are difficult to accurately simulate such dynamic interactions; at the same time, the scarcity of antibody-antigen complex structural data restricts the generalization ability of deep learning models. Although computational design can significantly shorten the initial R&D time, expression purification and activity testing still require several weeks, forming a bottleneck in the closed-loop technology.Future breakthroughs may rely on hybrid algorithm development to integrate physical models with generative AI tools.Single-cell sequencing and cryo-electron microscopy data are integrated based on the construction of a cross-scale database, and real-time iteration is achieved through robotic automation based on the "dry and wet closed-loop" R&D model.
From animal immunity to computational design, the innovation of antibody technology is not only a paradigm shift in the field of biomedicine, but also reflects the potential of multidisciplinary cross-border. With the development of AI and synthetic biology, the vision of designing antibodies completely from scratch may gradually be realized, opening a new chapter for precision medicine.
It is worth noting that the field of antibody computational design has recently achieved a key breakthrough. In March 2025, David Baker's team developed an AI protein generation tool RFdiffusion (called: new RFdiffusion) based on their previous development.A new version of the model specifically optimized for antibody variable regions such as CDR loops has been released.The team had achieved the generation of short-chain functional antibody fragments such as nanobodies in a study in March 2024, but due to the complexity of the antibody structure, the version at the time (called: ordinary RFdiffusion) still had limitations in designing more complex antibody structures.
After more than a year of algorithm iteration, the new RFdiffusion has been able to generate more complete single-chain variable fragment scFv that is closer to natural human antibodies by introducing antibody-antigen complex structure database training. This progress marks thatAI has achieved the coordinated design of antibody heavy and light chains with complete antigen-binding domains without the need for templates.Brings new hope to antibody design.
New RFdiffusion: De novo antibody design with atomic-level precision
In order to make RFdiffusion suitable for antibody design, the research team fine-tuned it. As shown in the figure below, during the training process, it uses the AlphaFold2/RF2 framework to represent the protein backbone, and adds noise to the protein framework through a series of "time steps (T)" until the framework becomes unrecognizable. At each time step, RFdiffusion predicts the denoised structure and optimizes it by minimizing the mean square error mse between the true structure X₀ and the predicted structure pX₀. After this unique training method, RFdiffusion is able to incrementally generate new protein structures starting from random residue distributions at inference time.
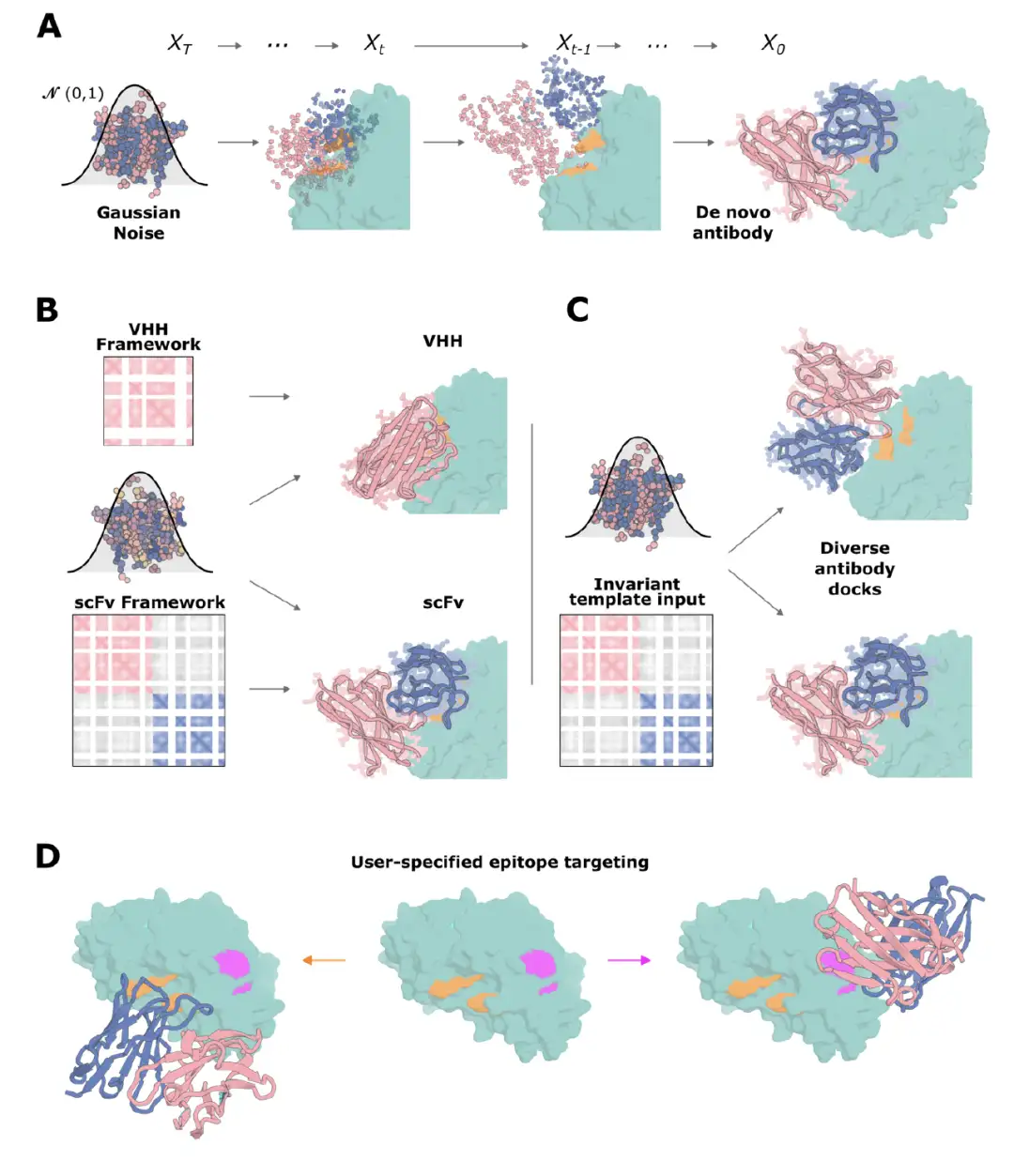
The study then applied the new RFdiffusion to design single-domain antibodies (VHHs). VHHs are designed based on the variable domains of camelid heavy chain antibodies, and their smaller size makes the genes encoding the designs easier and less expensive to assemble than single-chain variable fragments (scFv) or antigen-binding fragments (Fab).
Although VHHs have only three CDR loops, which is less than the six of traditional antibodies, the average interaction surface area of VHHs is very similar to that of traditional antibodies.This suggests that the approach for designing VHHs is also applicable to antibody design.
To design VHHs, the researchers chose a widely used chimeric VHH framework as the basis for a series of disease-related targets, including Clostridium difficile toxin B (TcdB), influenza H1 hemagglutinin HA, etc., and performed CDR loop sequence design in the target context through ProteinMPNN, and then screened using the fine-tuned RoseTTAFold2 network. In the design of influenza HA, in order to make the experimental design conditions consistent with the calculation parameters, the researchers used commercially produced monomeric HA products expressed by insect cells for affinity measurement.
The results show thatRFdiffusion enables the design of VHHs that specifically interact with target epitopes.The highest affinity binders to RSV site III, influenza HA, RBD, and TcdB are shown in the figure below, respectively. The CDR loops are significantly different from natural VHHs, indicating that the design was beyond the scope of the training dataset. For TcdB, the target epitope is the Frizzled-7 interface, and there are no antibodies or VHHs targeting this site in the PDB. In addition, the TcdB VHHs neutralized the toxicity of TcdB in CSPG4 knockout cells.
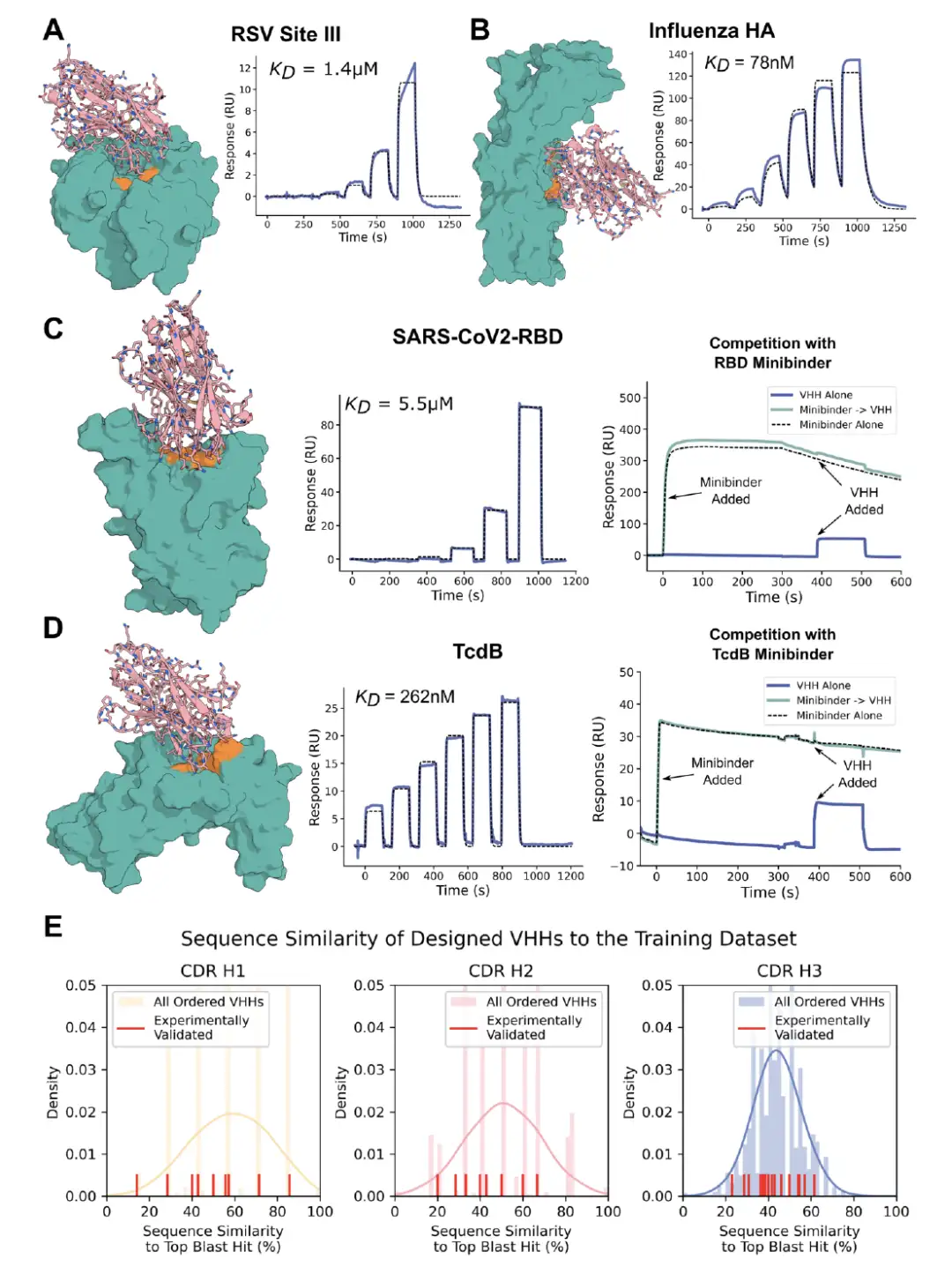
* Figure AC: Test results of VHH design targeting RSV III locus, influenza HA, RBD, and TcdB
* Figure E: Difference between VHH design and training dataset
The researchers further explored the ability of RFdiffusion to design heavy and light chains in the single-chain variable fragment scFv format. Unlike VHHs, the design of scFv is more complex, requiring the construction of all 6 CDRs and their docking patterns on the heavy and light chains. However, the gene synthesis of scFv faces challenges. On the one hand, the scFv sequence is long and difficult to assemble through conventional oligonucleotide pairs. On the other hand, the high sequence homology between scFvs makes specific pairing difficult.
To this end, the researchers developed a stepwise assembly protocol to achieve the construction of heavy and light chains.They can be paired specifically by design model or combined and mixed in subsets of designs with similar targeted binding modes.The experimental results show that new scFvs generated by combining different designs of heavy and light chain pairs can bind to the target epitope with a similar frequency as the original design. In addition, in the design collection with the same binding direction, the CDRs of the heavy and light chains interact with different regions and can be combined without losing structural accuracy, while random pairing rarely produces predicted binders.
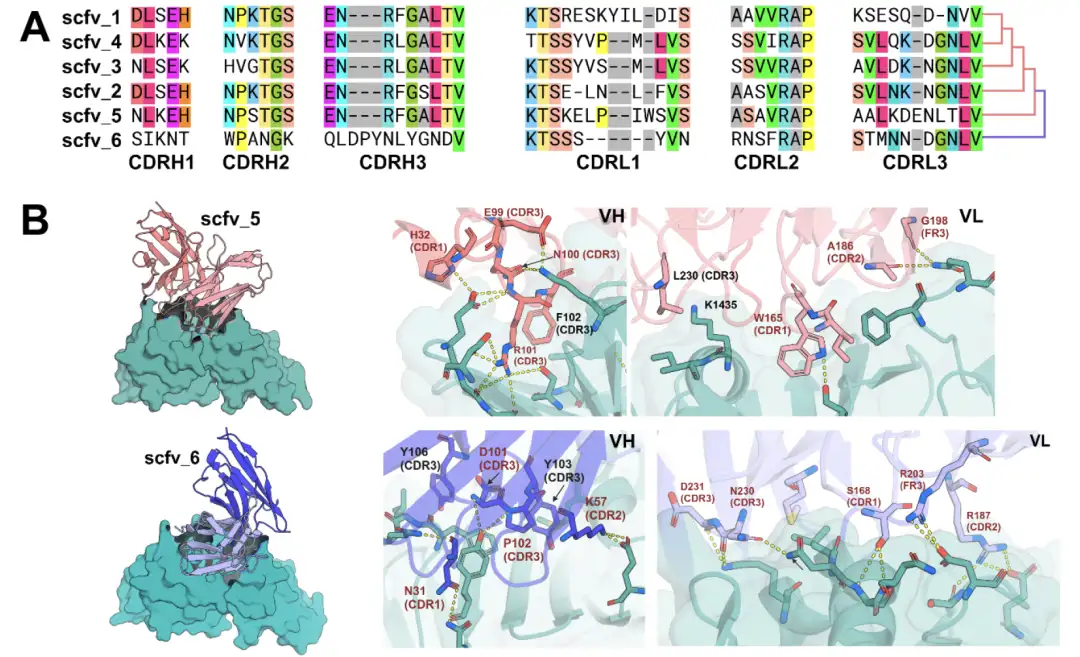
* Figure A: Multiple sequence alignment of 6 scFvs binding to TcdB
* Figure B: AlphaFold3 predicted structure of scFv5 and scFv6 in complex with TcdB receptor binding domain
David Baker: The Evolution of Antibodies and RFdiffusion
This study is just the tip of the iceberg of David Baker’s research achievements. In fact, in the frontier field of computational biology, David Baker’s team has achieved a series of breakthroughs from virus targeting to disease treatment through artificial intelligence-driven protein design.
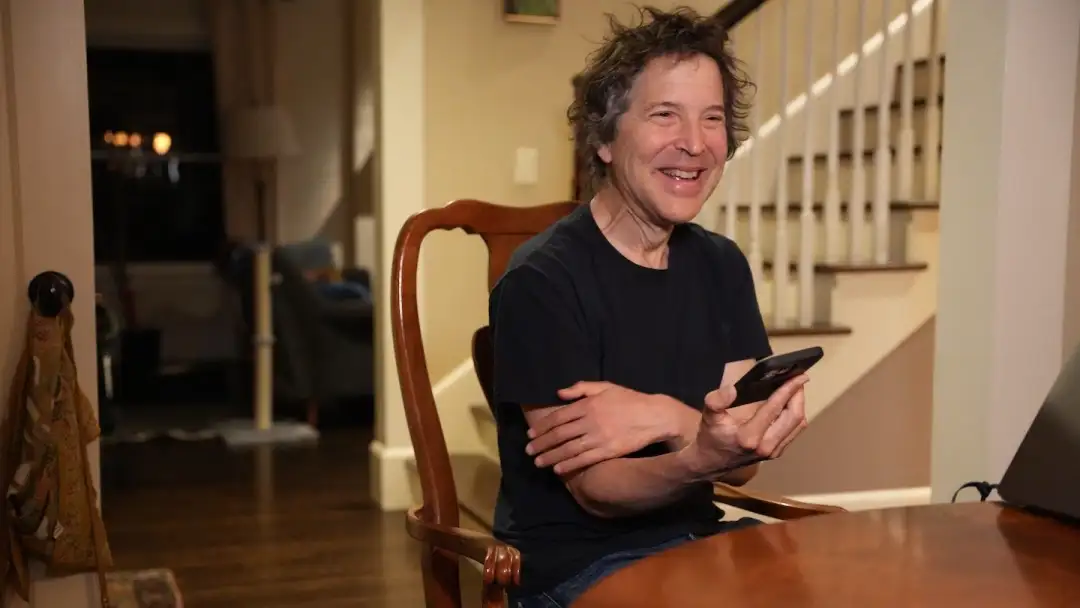
Among them, the RFdiffusion model has become an important foundation for them to change the paradigm of antibody drug development. In 2021, David Baker's team developed RosettaFold based on the AlphaFold2 framework.Its core capabilities are limited to predicting the three-dimensional structures of known proteins.
* RoseTTAFold open source address:
https://github.com/RosettaCommons/RoseTTAFold
The research team soon realized that the real revolution lies in "generation" rather than "reproduction". So they combined the diffusion model with the protein folding algorithm and launched the first generation of RFdiffusion in 2023. This model is like obtaining the key to reverse engineering: the traditional method requires inferring the structure from the amino acid sequence.RFdiffusion can reversely generate a new protein skeleton based on the target functional requirements.In early tests, it successfully designed a nanobody that binds to influenza hemagglutinin, but the CDR loop region of the antibody it generated still had conformational deviations, and cryo-electron microscopy showed a root mean square deviation of 1.2Å in the binding interface.
* Paper address:
https://www.science.org/doi/10.1126/science.abj8754
This limitation prompted the introduction of a key upgrade in 2024 - a dynamic restraint system.The research team added physical and chemical parameter constraints of the antigen-antibody binding site to the model, so that the generation process not only considers structural stability but also simulates the dynamic interactions between molecules.
The upgraded RFdiffusion performed very well in the design of the new coronavirus spike protein antibody: the flexible ring structure it constructed accurately locked the conserved epitopes on the virus surface, which was verified by experiments.Its binding affinity reaches 0.8nM, which is 15 times stronger than natural antibodies.What is even more remarkable is that this model begins to challenge "undruggable" targets: the miniature antibody designed for the IL-23 receptor contains only 58 amino acids, but can remain active in a high temperature of 80°C and a pepsin environment, realizing the oral administration of antibodies for the first time.
* Paper address:
https://www.biorxiv.org/content/10.1101/2024.03.14.585103v2
In 2025, RFdiffusion entered the multimodal fusion stage. The team integrated single-cell sequencing data and cryo-EM structure libraries, enabling the model to customize personalized antibodies directly based on the patient's immune repertoire characteristics. In the latest case, a tumor neoantigen from a patient with drug-resistant lung cancer was input into the system.RFdiffusion generated 12 candidate antibodies within 36 hours, three of which showed significant tumor-killing effects in organoid models.The model is no longer limited to antibody design: it is exploring the synthesis of cross-species protein elements, such as fusing the mechanosensitive ion channels of deep-sea barytoscopy bacteria with human antibodies to create smart drugs that can sense changes in the pH of the tumor microenvironment.
* Paper address:
https://www.nature.com/articles/s41586-024-08393-x
It can be seen that RFdiffusion is transforming from a "protein 3D printer" to a "life function architect", redefining the boundaries of synthetic biology. What's more interesting is that this evolution is far from reaching its end, and the innovation of antibody technology is pushing the biomedical field to new heights.