Selected for ICLR 2025! Zhejiang University Shen Chunhua Et Al. Proposed Boltzmann Alignment Technology, Protein Binding Free Energy Prediction Reached SOTA
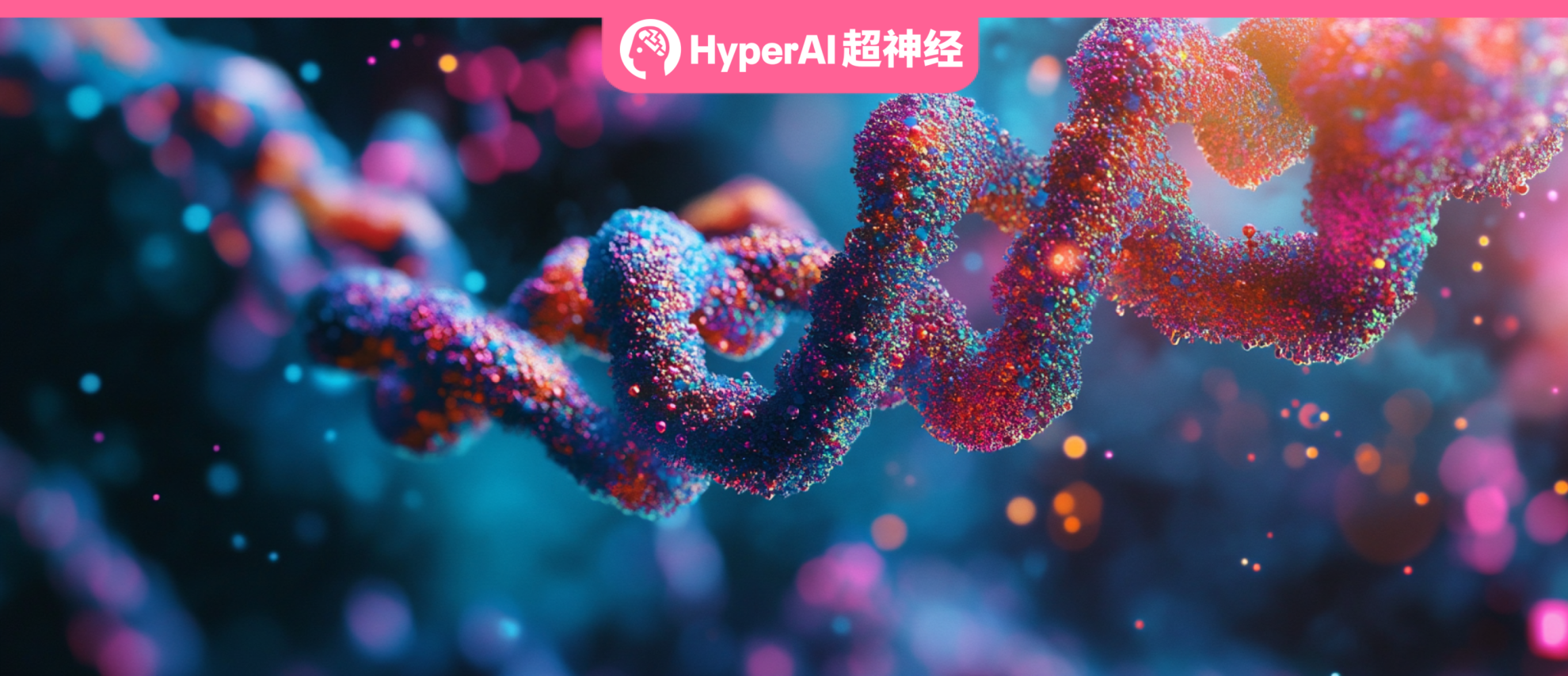
Protein-protein interactions (PPIs) are the basis for all organisms to perform various biological functions, mainly through the interaction and influence between different protein molecules. Accurately identifying and understanding the interactions between proteins is extremely important for deciphering protein functions, revealing life activities, exploring disease mechanisms, developing targeted drugs, and innovating biological applications.
With the development of computers and artificial intelligence, the research on PPIs has made great progress in recent years with the support of deep learning. In particular, AlphaFold 3 released by DeepMind in 2024,The success rate of predicting the structure of general protein complexes has been raised to nearly 80%.This also effectively solves the problem of high-fidelity computational modeling of protein interactions that has plagued the scientific research community for decades.
However, the interaction between proteins is a dynamic process that includes binding and dissociation. It is difficult to fully capture the interaction between biological molecules by studying static structures alone.Parameters such as binding free energy (∆G, the difference in Gibbs free energy between bound and unbound states) can quantitatively characterize the dynamics of protein-protein interactions.However, how to accurately predict the change in binding free energy (∆∆G, also known as mutation effect) has become one of the prerequisites for the scientific community to understand or regulate protein-protein interactions.
Based on this, Professor Shen Chunhua's team from the School of Computer Science and Technology at Zhejiang University, together with teams from the University of Adelaide in Australia and Northeastern University in the United States,We jointly propose a technique called Boltzmann alignment to transfer knowledge from a pre-trained inverse folding model to the predictions of ∆∆G.The study first analyzed the thermodynamic definition of ∆∆G and introduced the Boltzmann distribution to link energy and protein conformation distribution, thus highlighting the potential of pre-trained probabilistic models. The team then used Bayes' theorem to circumvent direct estimation and used the log-likelihood provided by the protein reverse folding model to estimate ∆∆G. This derivation provides a rational explanation for the high correlation between the binding energy and log-likelihood of the reverse folding model observed in other previous experiments.
Compared with the previous inverse folding-based method, the experimental results of this method on the SKEMPI v2 dataset show a superior level.Its Spearman coefficient in supervised and unsupervised states reached 0.5134 and 0.3201 respectively.Significantly higher than the previous SOTA methods of 0.4324 and 0.2632.
The achievement was titled "Boltzmann-Aligned Inverse Folding Model as a Predictor of Mutational Effects on Protein-Protein Interactions" and was included in ICLR 2025, the top international academic conference in the field of artificial intelligence. It is worth mentioning that this year's ICLR received a total of 11,565 submissions, and only 32.08% of manuscripts were accepted.
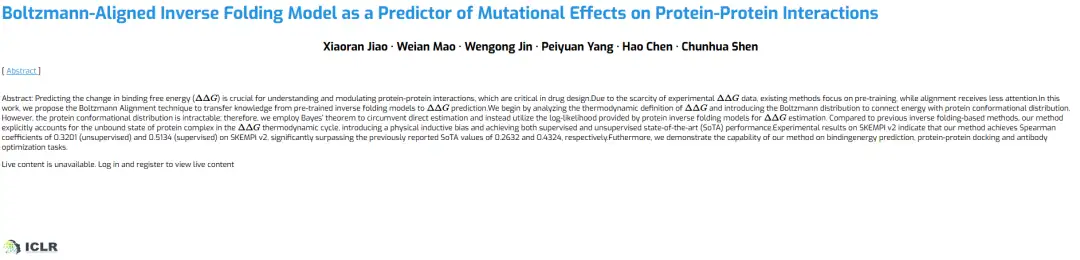
Paper address:
https://arxiv.org/abs/2410.09543
Recommend an academic sharing event. The latest Meet AI4S live broadcast invitation is at 12:00 noon on March 7.Huang Hong, associate professor at Huazhong University of Science and Technology, Zhou Dongzhan, young researcher at AI for Science Center of Shanghai Artificial Intelligence Laboratory, and Zhou Bingxin, assistant researcher at the Institute of Natural Sciences of Shanghai Jiao Tong University,Introduce personal achievements and share scientific research experience.
Deep learning accelerates paradigm shift in computation of mutation effects
The scientific community has been studying the prediction of ∆∆G for a long time.Traditional methods can be divided into two categories: biophysical methods and statistical methods.Among them, biophysical methods mainly simulate how proteins interact at the atomic level through energy calculations; statistical methods rely on feature engineering, mainly using descriptors to capture the geometric, physical, and evolutionary characteristics of proteins.
There is no doubt that no matter which traditional method is used, it needs to rely heavily on human expertise, which is not only time-consuming and laborious, but also unable to accurately capture the complex interactions between proteins. In addition, both methods have their own disadvantages. For example, biophysical methods often face challenges in balancing speed and accuracy. The deep learning-based method not only shows extremely high "talent" in protein modeling, but also accelerates the transformation of the ∆∆G prediction paradigm.
More and more cases are supporting this. For example, a team from the Chinese Academy of Sciences proposed a method based on representation learning, called SidechainDiff.This method uses the Riemann diffusion model to learn the generation process of side chain conformations and can also give a structural background representation of mutations at the protein-protein interface.Using the learned representations, the method achieves state-of-the-art performance in predicting the effects of mutations on protein-protein binding.
This result is titled "Predicting mutational effects on protein-protein binding via a side-chain diffusion probabilistic model" and is included in NeurIPS 2023.
* Paper address:
Although deep learning-based methods have achieved considerable results, they are not perfect.This paper also mentions that "experimental data on the annotation of binding energy is lacking".This is generally considered to be a major challenge based on deep learning methods, which has directly led to more teams tending to pre-train on a large number of unlabeled datasets before improving the ability to predict mutations. This involves a variety of pre-training agent tasks, such as protein inverse folding, mask modeling, and side chain modeling in the above example.
Fortunately, these "alternative" methods have achieved their goals, but unfortunately, they have also shown their weaknesses without exception. Most pre-training-based methods only use supervised fine-tuning (SFT).However, they ignore the importance of data alignment, which may cause supervised fine-tuning to cause the model to forget the general knowledge previously acquired during unsupervised pre-training, resulting in the risk of overfitting.Looking back, these "alternative" methods undoubtedly highlight the urgency of transferring the acquired knowledge for accurate mutation prediction.
Innovative development of Boltzmann alignment to surpass SOTA models
Specifically, the research team first based on the Boltzmann distribution and thermodynamic cycle principles,The change in binding free energy when a protein mutates is linked to the possibility of the protein's amino acid sequence.The Boltzmann alignment was proposed (as shown on the right side of the figure below). Subsequently, the research team proposed a method called BA-Cycle, which integrates the inverse folding model into the Boltzmann alignment and uses the inverse folding model to evaluate mutations by predicting the likelihood of protein sequences (as shown on the left side of the figure below).
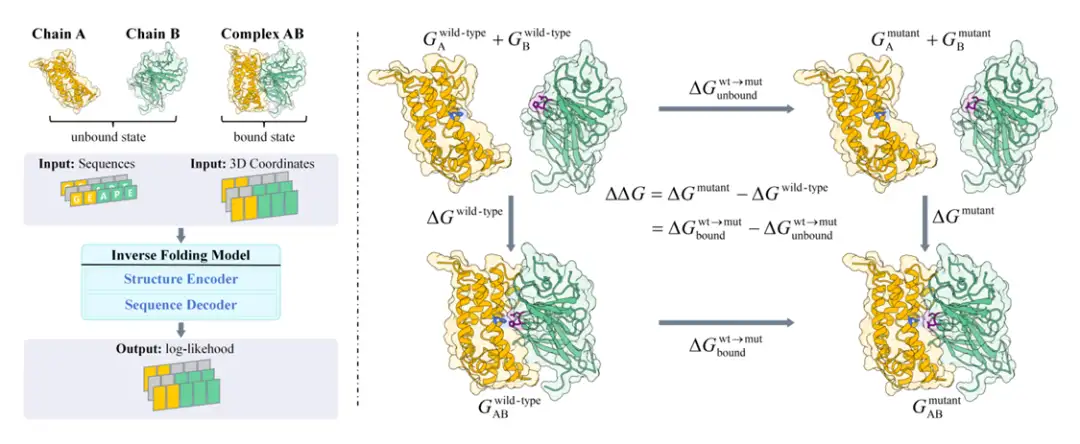
It is worth mentioning that in order to establish the connection between protein binding free energy and protein sequence conditional probability, and to solve the two major difficulties faced in directly estimating the probability p(X|S) of protein structure under a given sequence:The limitations of existing protein structure prediction models and the shortcomings of probabilistic models,The research team substituted Bayes' theorem into the calculation formula for binding freedom, i.e. p(X|S) = p(S|X) ・ p(X)/p(S), and successfully linked the binding free energy to the conditional probability p (X|S) of the protein sequence, avoiding the difficulty of directly estimating p (X|S). This laid the foundation for further analysis of the relationship between changes in binding free energy and the conditional probability of protein sequences.
In addition, since it is assumed that the protein structure remains unchanged before and after mutation,The research team used the reverse folding model to evaluate the sequence probabilities of the bound and unbound states.The backbone structure of the bound state is usually known, and the model can directly calculate its probability; the backbone structure of the unbound state is not explicitly given, and the probability can be estimated by evaluating the two chains in the complex separately.
Based on this,The research team proposed a method called BA-Cycle for unsupervised estimation of ∆∆G.Using the pre-trained reverse folding model ProteinMPNN, an unsupervised estimation of ∆∆G was achieved. This is in stark contrast to previous studies that did not explicitly consider the probability of unbound states in the thermodynamic cycle.
at last,The research team also proposed a method called BA-DDG.BA-Cycle is fine-tuned by Boltzmann alignment using labeled data with binding free energy changes. BA-DDG uses the same forward process as BA-Cycle. The goal of BA-DDG is to minimize the gap between the true binding free energy change and the predicted binding free energy change while maintaining the distribution of the original pre-trained model.
The research team conducted a series of experimental verifications on the SKEMPI v2 dataset.Among them, the SKEMPI v2 dataset is an annotated mutation dataset containing 348 protein complexes, including 7,085 amino acid mutations as well as changes in thermodynamic parameters and kinetic rate constants.
There are a total of 7 evaluation indicators, including 5 overall indicators, namely Pearson correlation coefficient, Spearman's rank correlation coefficient, minimum root mean square error (RMSE), minimum mean absolute error (MAE) and AUROC. In addition, the research team grouped the mutations according to their structural characteristics and calculated the Pearson correlation coefficient and Spearman correlation coefficient for each group as 2 additional indicators.
The research team first compared BA-Cyale and BA-DDG with SOTA unsupervised and supervised methods,There are three types of unsupervised methods, including traditional empirical energy functions such as Rosetta Cartesian ∆∆G and FoldX; sequence/evolution-based methods such as ESM-1v, Position-Specific Scoring Matrix (PSSM), MSA Transformer and Tranception; and pre-trained methods based on structural information that are not trained on ∆∆G labels, such as ESM-1F, MIF-∆logits, RDE-Linear and B-factor.
Supervised methods are divided into two categories, including end-to-end learning models such as DDGPred and End-to-End; and pre-training methods based on structural information, fine-tuned on ∆∆G, including MIF-Network, RDE-Network, DiffAffinity, Prompt-DDG, ProMIM and Surface-VQMAE.
The results show thatBA-DDG outperforms all baselines in all evaluation metrics.The Pearson correlation coefficient and Spearman correlation coefficient under the supervised mode reached 0.5453 and 0.5134 respectively. Its significant improvement in the correlation of each structure highlights its higher reliability in practical applications;BA-Cycle achieves comparable performance to the empirical energy function and outperforms all unsupervised learning baselines.As shown in the following figure:
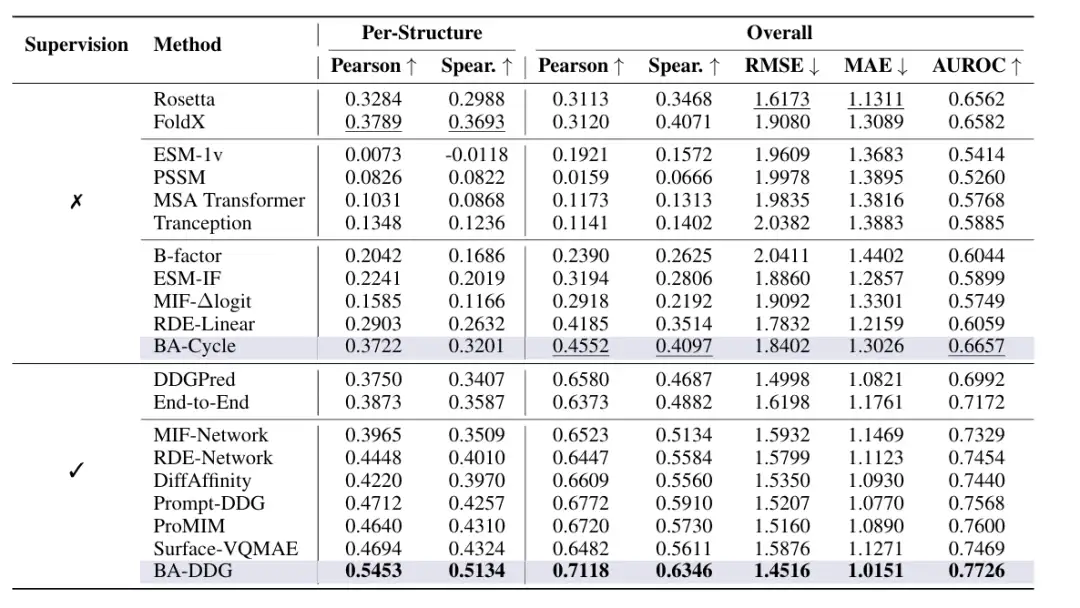
In addition, it is clearly seen in the relevant visual analysis thatBA-DDG outperforms other methods in both qualitative visualization and quantitative metrics.As shown in the following figure:
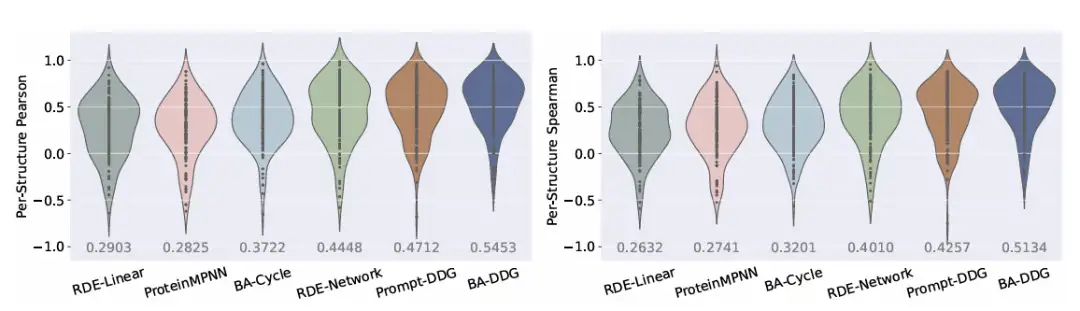
In addition, the researchers also conducted experiments on binding energy prediction, protein-protein docking and antibody optimization, and the results showed that it has a wide range of applicability. These positive effects will play an extremely important role in drug design and virtual screening, laying a theoretical foundation for future real-world applications.
Deeply cultivate machine learning and machine vision to realize AI universalization
In this study, the researchers used cross-disciplinary theories to provide a new perspective for protein sequence analysis, and at the same time formed a systematic research framework through innovative model integration and model optimization. This step-by-step in-depth research method not only helps to fully and deeply understand the relationship between protein sequence and free energy changes, but also provides a new idea for subsequent research.
It is worth mentioning thatProfessor Shen Chunhua, one of the main participants in this research, has been committed to the research of machine learning and computer vision for a long time.More than 150 papers have been published so far, including on internationally renowned academic platforms such as TPAMI and IJCV. Only two months into 2025, the team led by Professor Shen Chunhua has already produced significant results, publishing three papers on the preprint platform arXiv.
In the first paper, Professor Shen Chunhua's research group developed a DNA-based model based on the CNN network, named ConvNova. The model is simple in design but has significant performance.In the related histone task, the average score exceeded the second place method 5.8%, achieving faster calculations with fewer parameters.At the same time, this method also verifies that the method based on CNN network architecture has strong competitive potential compared with the method based on Transformer network and SSM network. The related research was published under the title "Revisiting Convolution Architecture in the Realm of DNA Foundation Models".
* Paper address:
https://arxiv.org/abs/2502.18538
In the second article, Professor Shen Chunhua’s research group and the Shanghai AI Laboratory jointly developed a general vision model DICEPTION.The pre-trained diffusion model is used to solve multi-task visual perception problems, which requires less training data and has strong adaptability to tasks.Using only 0.06% of SAM data, the model achieved a level comparable to that of SOTA models in tasks such as segmentation, and unified task outputs through color coding, significantly reducing training costs. The related research was published under the title "DICEPTION: A Generalist Diffusion Model for Visual Perceptual Tasks".
* Paper address:
https://arxiv.org/pdf/2502.17157
In the third article, Professor Shen Chunhua's team and Alibaba jointly proposed a benchmark called PhyCoBench, which is used to evaluate the ability of video generation models to generate videos that conform to physical laws. At the same time, the study also introduced the automatic evaluation model PhyCoPredictor, which is a diffusion model that generates optical flow and video frames in a cascade manner. By comparing the consistency evaluation of automatic and manual sorting,Experimental results show that PhyCoPredictor has the ability closest to human evaluation.The related research was published under the title “A Physical Coherence Benchmark for Evaluating Video Generation Models via Optical Flow-guided Frame Prediction”.
* Paper address:
https://arxiv.org/pdf/2502.05503
Professor Shen Chunhua's team has not only achieved fruitful results, but also has a significant personal influence. The papers published by Professor Shen Chunhua have always been an important source of citations in the scientific research community. He was also selected into the "2023 Highly Cited Chinese Researchers" list released by Elsevier, a global information analysis company.
Today, Professor Shen Chunhua has served as the Qiushi Chair Professor and deputy director of the National Key Laboratory of Computer-Aided Design and Image Systems at Zhejiang University for three years. Not only has he achieved fruitful research results, but his teaching results are also impressive, and he has trained many master's and doctoral students. In addition, the National Key Laboratory of Computer-Aided Design and Image Systems, where he is located, as an interface connecting "industry-university-research", has also achieved multi-faceted development in recent years, cooperating with many companies including Ant, and has become an innovation base for scientific research, a talent training base, and an innovation incubation base.