The Efficiency of Inorganic Material Retrosynthesis Has Soared. A Korean Team Launched Retrieval-Retro, and the Results Were Selected for NeurIPS 2024
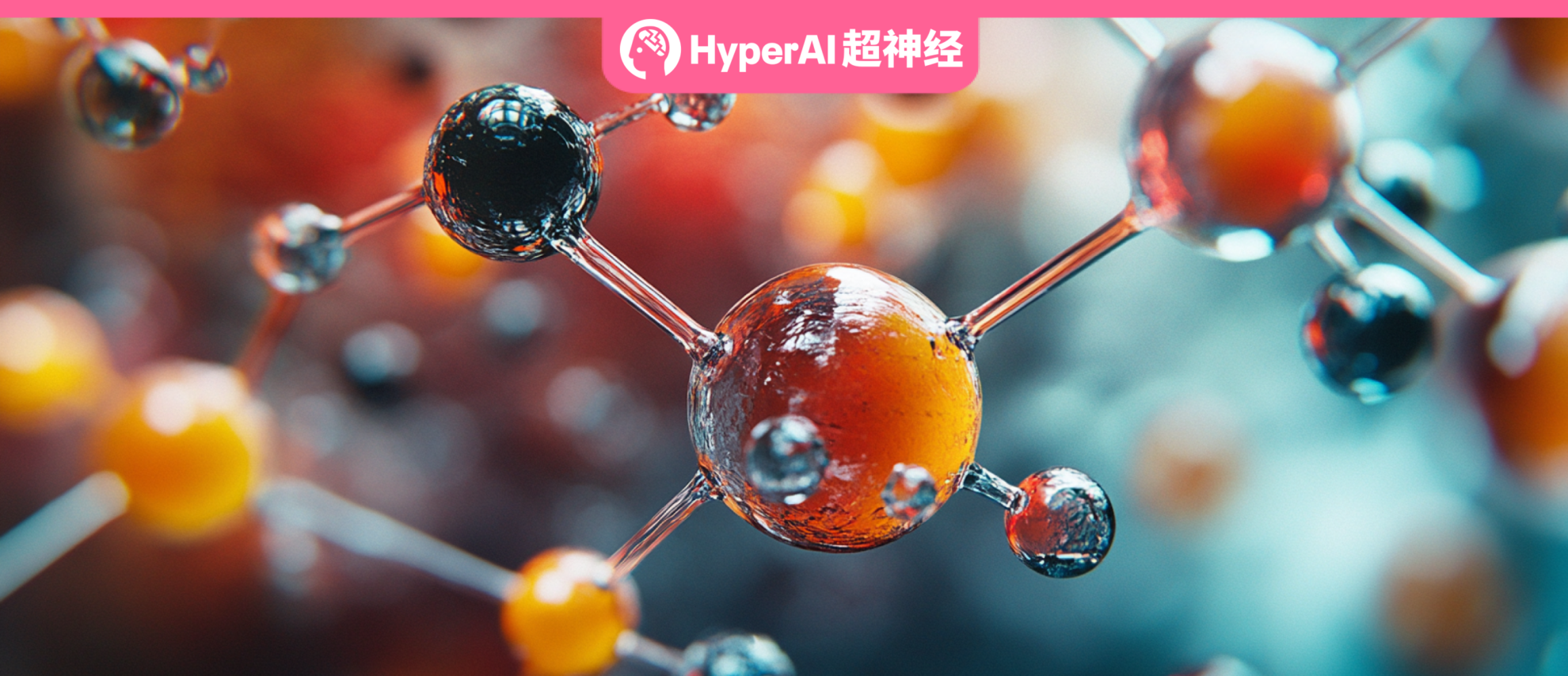
In November 2023, scientists at the Lawrence Berkeley National Laboratory in the United States gathered around a robotic arm with bated breath. This AI material synthesis platform called A-Lab had just failed to synthesize 41 new inorganic materials in a row. When the red warning light came on, the laboratory cheered. Professor Gerbrand Ceder, the project leader, explained: "This mistake is more valuable than success.It exposes AI's blind spot in understanding solvent dynamics.This is a critical moment in the co-evolution of humans and machines."
This seemingly abnormal celebration reflects a paradigm shift in the field of inorganic material synthesis that has not happened in a century. Since the organic chemist EJ Corey proposed the retrosynthetic analysis method in the 1960s, inorganic chemists have been looking for their own "holy grail" -How can we reverse-engineer complex inorganic materials into actionable synthesis steps, just like disassembling Lego bricks?
This dream took a turn for the better in 2020: the team of Academician Yu Shuhong of the University of Science and Technology of China disclosed in the journal Nature Nanotechnology that they used machine learning to predict interface energy differences and successfully "carved" magnetic materials at specific locations on semiconductor nanowires.This technology, which was once believed by the academic community to require 20 years of experimental accumulation, was successfully cracked by AI in just 3 months.
The wave of change is coming faster than expected.Google DeepMind launched the GNoME platform.In just 17 days, 380 stable inorganic crystals were screened, 52 of which were experimentally verified. Even more amazing is that when the Northwestern Polytechnical University team was developing ceramic coatings for spacecraft,AI reversely deduces the counterintuitive path of "first building a microcrack network and then filling it with healing agent"——This “loss-preventing” strategy increases the material’s temperature resistance by 300°C, just like forging a self-repairing “armor scale” for the aircraft.
Today, behind the fume hoods of laboratories around the world, a quiet dual revolution is quietly unfolding: AI is not only learning from human synthetic experience, but also exploring and creating new preparation paths that surpass human intuition.The Korea Research Institute of Chemical Technology (KRICT) and the Korea Advanced Institute of Science and Technology (KAIST) have jointly proposed an inorganic retrosynthesis planning method called Retrieval-Retro.This method successfully promotes the efficiency and accuracy of inorganic material synthesis by combining thermodynamic relationships and attention mechanisms. Its outstanding performance in identifying new synthetic formulas brings new hope to the field of material discovery and is expected to play a greater role in future research.
The related results, titled "Retrieval-Retro: Retrieval-based Inorganic Retrosynthesis with Expert Knowledge", were selected for NeurIPS 2024, the top academic conference in the field of AI.
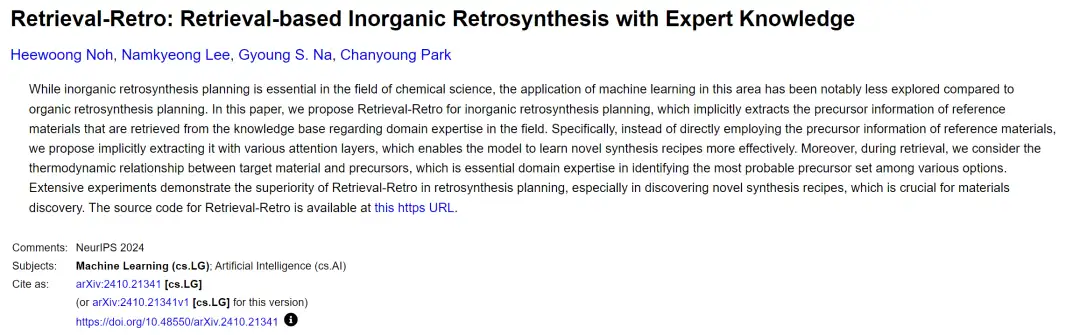
Paper link:
https://doi.org/10.48550/arXiv.2410.21341
Dataset download link:
Open source address:
https://github.com/HeewoongNoh/Retrieval-Retro
Inorganic retrosynthesis: highly dependent on experimental trial and error, AI algorithms still need to be improved
In the long history of materials science, trial and error was the only way to explore the unknown - scientists repeatedly adjusted formulas and fired samples like a blind man touching an elephant until they accidentally touched the "sweet spot" of performance. This pattern was first broken in the field of organic synthesis due to the birth of retrosynthetic analysis: in 1964, EJ Corey proposed to reversely disassemble the target molecule into synthons like a puzzle, and find the synthesis path through logical reasoning rather than blind attempts. Just like when solving the problem of benzofuran synthesis, chemists no longer need to test all possible combinations of phenol derivatives, but by identifying the breakage site of the key CO bond, they can accurately locate the iron-catalyzed coupling path of phenol and 1,3-dicarbonyl compounds. This revolution in thinking based on intelligent severing of chemical bonds has enabled organic synthesis to move from empiricism to the era of rational design.
However, when the battlefield turns to the inorganic world, things become much more complicated.first,Inorganic compounds involve more complex bonding mechanisms, and their structure-property relationships are difficult to analyze modularly through functional groups like organic molecules;Secondly,Inorganic synthesis reactions are often accompanied by multiphase interface evolution and competition with metastable states, and their kinetics are more difficult to predict than solution reactions in organic systems.Furthermore,The calculation accuracy of existing computational chemistry methods for key parameters such as inorganic crystal field stabilization energy and defect formation energy is not sufficient to support reliable reverse path deduction. As a result, inorganic retrosynthesis research is still highly dependent on experimental trial and error, and the construction of its theoretical framework is far more complicated than that of organic systems.
Today, the addition of AI technology has opened up a new path for this field. For example, generative adversarial networks break through the limitations of human experience and can design innovative structures such as perovskite lattices with special electromagnetic properties. The quantum Monte Carlo method goes deep into the microscopic world and analyzes the quantum entanglement mechanism of Cooper pairs in high-temperature superconductors. Not to be outdone, graph neural networks gradually build a quantum reaction rule system exclusive to inorganic materials by decoding the laws of atomic orbital reorganization.
With the continuous evolution of AI technology in these areas, the difficulties of inorganic retrosynthesis are also being gradually overcome. In the wave of technological iteration,Convolutional variational autoencoders are the first to achieve reverse design of materials.This brought hope to this field. Subsequently, the ElemwiseRetro model further introduced a precursor template library to optimize the prediction accuracy. However, although the existing algorithms have made great progress,However, the decision-making wisdom of chemists who "refer to similar materials" has not yet been fully replicated.In other words, AI needs to further learn how human chemists think in order to design materials more accurately.
To make up for this shortcoming, a research team from Seoul National University developed a new inorganic retrosynthesis planning method called Retrieval-Retro.It aims to accelerate the discovery and synthesis of materials by efficiently identifying and extracting precursor information through advanced retrieval techniques and attention mechanisms.Extensive experiments have shown that Retrieval-Retro has demonstrated excellent performance in various scenarios, especially in the more realistic and challenging year-division scenario. Its outstanding ability in discovering new synthetic formulas for inorganic materials fully demonstrates its great application potential in practical material discovery.
Retrieval-Retro: An innovative approach to inorganic retrosynthesis planning
The core of Retrieval-Retro is to use two complementary retrievers——Masked Precursor Completion (MPC) retriever and Neural Reaction Energy (NRE) retriever, which extract precursor information based on 33,343 inorganic material synthesis formulas extracted from 24,304 material science papers as reference materials.
Dataset download link:
The MPC retriever identifies reference materials with similar precursors to the target material by learning the dependencies between precursors. It retrieves the top K most similar materials by calculating the cosine similarity between the target material and all materials in the knowledge base.This method can effectively capture the correlation between precursors and target materials, providing important clues for subsequent synthesis planning.
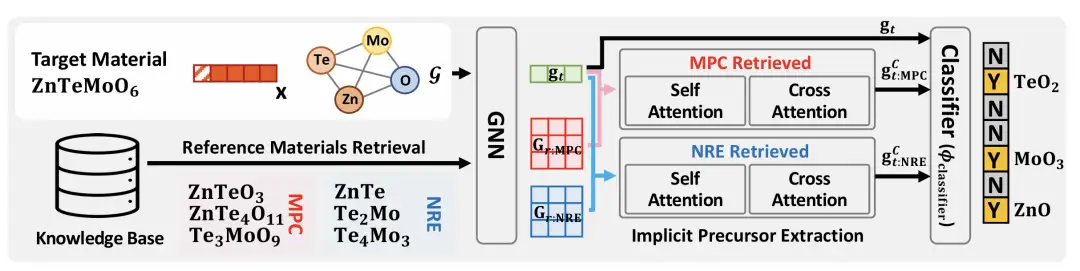
However, while the MPC searcher can identify similar precursor sets, it ignores the thermodynamic relationships between materials, which are crucial in inorganic synthesis. To this end, the NRE searcher is based on the thermodynamic driving force.The reference material is selected by considering the Gibbs free energy (∆G) between the target material and the precursor ensemble.Under constant pressure and temperature, if ∆G is negative, it indicates that the synthesis reaction can occur spontaneously, and the larger the ∆G value, the higher the possibility that the precursor set can synthesize the target material. The NRE retriever uses DFT calculated generation energy data and experimental generation energy data through pre-training and fine-tuning mechanisms to predict the generation energy of the target material and the reference material, thereby selecting the most thermodynamically favorable reference material.
In the process of extracting precursor information,Retrieval-Retro adopts self-attention and cross-attention mechanisms.By encoding the target material and the reference material through the composition graph encoder, the model can enhance the representation of the reference material through the self-attention mechanism, and merge the representation of the target material with the enhanced reference material representation through the cross-attention mechanism, thereby implicitly extracting the precursor information. This method can not only make full use of the information of the reference material, but also avoid the limitations of directly using the precursor information of the reference material, significantly improving the model's ability to learn and derive new synthesis formulas.
To verify the effectiveness of Retrieval-Retro, the researchers compared it with a variety of existing inorganic retrosynthesis methods and baseline methods. These methods include material composition-based representation learning methods (such as Roost and CrabNet) and newly proposed baseline methods (such as Composition MLP and Graph Network). The experimental results show thatRetrieval-Retro outperforms the baseline model in all test scenarios.Especially in the setting divided by year, the performance improvement is more significant, which shows that Retrieval-Retro is not only innovative in theory, but also has strong adaptability and effectiveness in practical applications.
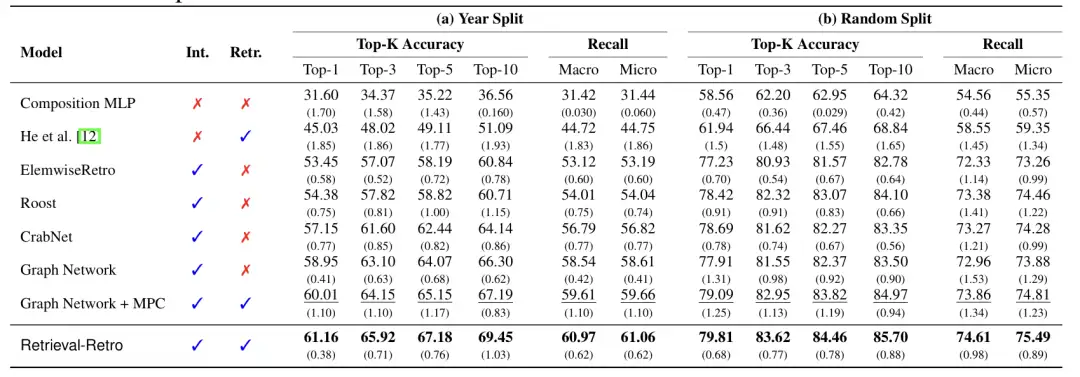
The ultimate form of material alchemy: when AI begins to question the periodic table
Against the backdrop of Seoul National University's Retrieval-Retro model breaking through the boundaries of traditional retrieval, the field of inorganic retrosynthesis is welcoming new development opportunities.As of 2024, humans have synthesized element 118, Og.Although these elements may have extremely short half-lives in the real world, applications for AI-assisted materials discovery are already emerging.
This kind of virtual-real exploration is reshaping the cognitive dimension of materials science. While traditional inorganic chemistry is still adhering to Pauling's rule and Hume-Rothery's law, AI has begun to use tensor networks to reconstruct electron correlation effects and explore the potential mechanism of high-temperature superconductors through quantum annealing algorithms. For example, A-Lab has successfully synthesized a variety of new inorganic materials by combining robotics and machine learning, demonstrating the great potential of AI in material synthesis.
This cognitive leap brings about a double revolution:On the technical level,Microsoft's quantum computing team is combining topological qubits with retrosynthetic algorithms to achieve more stable and efficient quantum computing using topological conductor materials through its latest quantum chip "Majorana 1";In terms of philosophy of science,MIT's Synthetic Intelligence Laboratory has begun to explore how AI can simulate and optimize chemical synthesis processes through virtual reactors, thereby redefining human understanding of the material world. Just like Madame Curie extracted radium from pitchblende, AI may be precipitating material forms that humans have not yet named in virtual reactors.
Standing at the intersection of the old and new paradigms, inorganic retrosynthesis is writing the most exciting chapter: it not only continues the tradition of material deconstruction inherited from the Lavoisier era, but also breeds the "post-human materials science" of human-machine collaboration. When the X-rays of the Shanghai Synchrotron Radiation Light Source and the neural network of GNoME jointly analyzed the 380th stable crystal, we saw not only technological iteration, but also the upgrading of cognitive dimensions - just like quantum mechanics subverted classical physics, AI is opening up the "Schrödinger toolbox" of multiple realities for materials science.
It is worth noting that the real revolution is not that machines replace humans, but that when AI begins to redefine chemical bonds using non-local wave functions, humans finally gain a second pair of eyes to observe the material world. Under the gaze of this pair of "mechanical eyes", inorganic material synthesis is transforming from an empirical skill to a cognitive bridge connecting classical chemistry and the quantum universe.