New Discovery of High Entropy Alloys! Multiple Teams Work Together to Achieve High-precision Prediction of Oxidation Resistance. Increasing the Content of Aluminum/chromium/silicon Can Effectively Improve
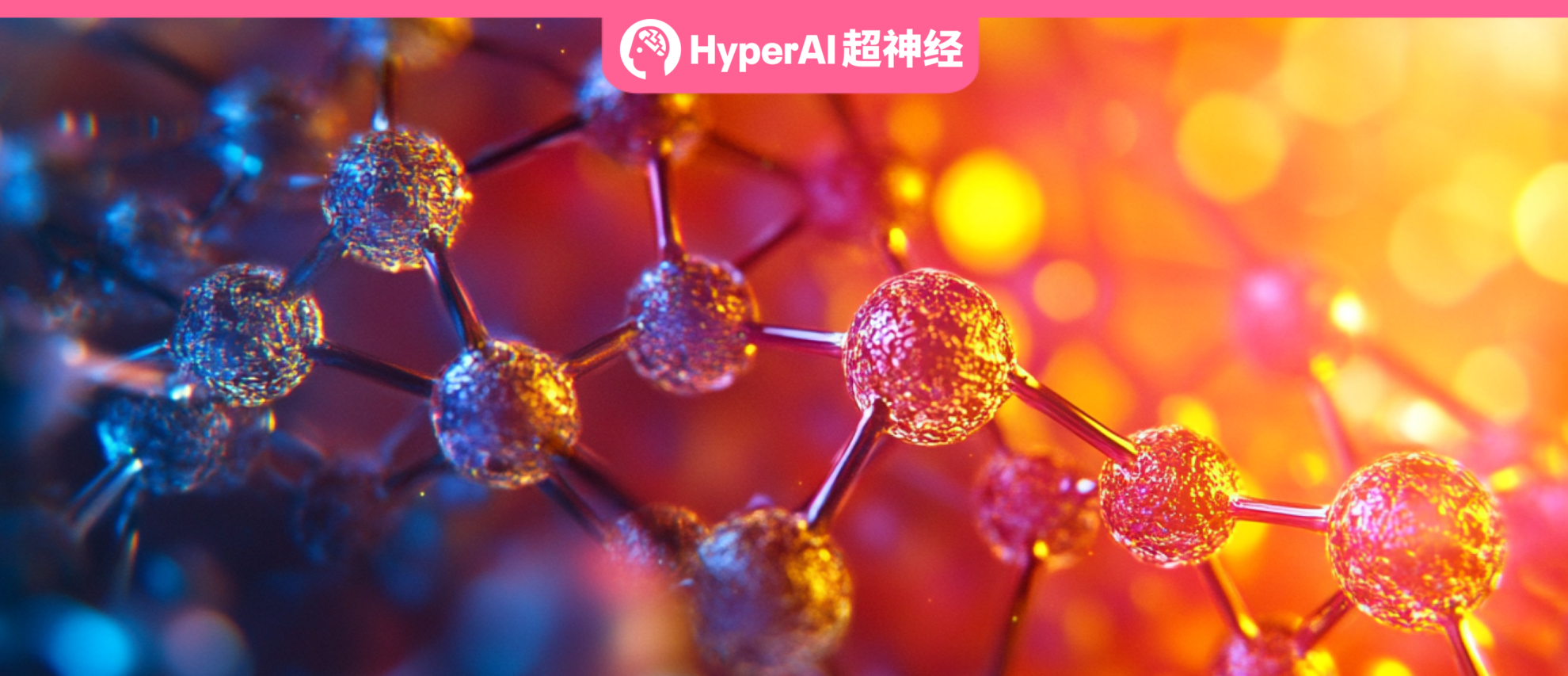
As one of the most important components in an aircraft engine, turbine blades need to work continuously at temperatures exceeding 1000°C while bearing enormous mechanical stress. In 2018, a Southwest Airlines commercial passenger plane suddenly suffered an engine failure during flight, resulting in an emergency landing. Subsequent investigations found that the root cause of the failure was oxidation and corrosion of the engine turbine blades in a high temperature environment, which eventually led to structural failure.
This incident not only caused huge losses to the airlines, but also made scientists realize:Traditional high-temperature materials have reached their performance limits, and a more powerful material is urgently needed to meet future challenges.
Nickel-based superalloys have long been the preferred material for manufacturing turbine blades, but as the performance of aircraft engines continues to improve, the performance of nickel-based superalloys is gradually approaching its limit. Scientists began to look for new materials that can work stably at higher temperatures and in harsher environments.Refractory high entropy alloys (RHEAs) and refractory composite high entropy alloys (RCCAs) came into being.
These new materials, due to their unique composition and structure, show amazing high-temperature performance and are hailed as "the hope of the next generation of high-temperature materials." But the question also arises: How to quickly and accurately predict the antioxidant properties of these new materials in high-temperature environments?
Traditional methods are not only time-consuming and laborious, but also difficult to cope with the diversity of complex alloy systems. Recently, a joint research team from the University of Bordeaux in France, the National Institute for Materials Science in Japan, the National Tsing Hua University in Taiwan, the University of Leuven in Belgium, and the WEL Institute in Belgium,The high-precision prediction of the antioxidant properties of RHEAs and RCCAs was successfully achieved through the gradient boosting decision tree (GBDT) technology.It brought a revolutionary breakthrough in the field of materials science.
The related results were published in Scripta Materialia, a journal in the field of materials science, under the title "Advancing refractory high entropy alloy development with AI-predictive models for high temperature oxidation resistance".
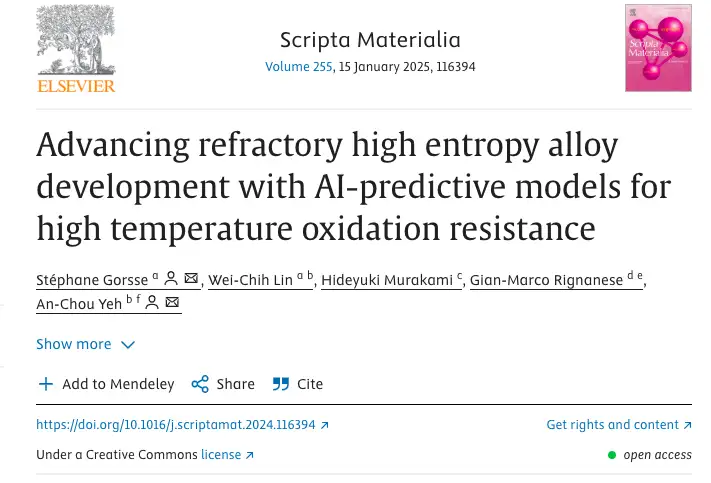
Paper link:
https://doi.org/10.1016/j.scriptamat.2024.116394
The open source project "awesome-ai4s" brings together more than 200 AI4S paper interpretations and provides massive data sets and tools:
https://github.com/hyperai/awesome-ai4s
AI is expected to solve the oxidation prediction problem in the search for a perfect replacement for nickel-based superalloys
The rapid development of modern industry is inseparable from the continuous innovation of materials science. From aerospace to energy development, from electronic equipment to medical equipment, every technological leap is accompanied by the birth of new materials. Among them, high-temperature alloys have always played a core role with their excellent performance.
High-temperature alloy is a high-performance material that can maintain high strength, oxidation resistance, thermal corrosion resistance, fatigue resistance, fracture toughness and stable internal structure in extremely high temperature environment. It is mainly composed of elements such as iron, nickel, cobalt, and supplemented by elements such as titanium, aluminum, chromium, molybdenum, and tungsten.Depending on the matrix elements, high-temperature alloys can be divided into three categories: iron-based, nickel-based and cobalt-based.
Among them, iron-based high-temperature alloys are often used in parts of the engine with lower operating temperatures; nickel-based high-temperature alloys are widely used in the hottest parts of aircraft engines and industrial gas turbines due to their excellent high-temperature strength, accounting for about 80% of the total demand for high-temperature alloys; cobalt-based high-temperature alloys are the ideal choice for guide blade materials due to their excellent castability and weldability.
Among the four major high-temperature alloy systems in the world, nickel-based alloys occupy a very core and important position. In recent years, refractory high-entropy alloys (RHEAs) and refractory composite high-entropy alloys (RCCAs) have become important candidate materials for high-temperature applications. These alloys are characterized by a mixture of multiple main refractory elements (such as Zr, Hf, V, Nb, Ta, Cr, Mo, W and Re), and a small amount of Al, Si or Ti is added.They generally exhibit superior mechanical properties and higher melting points than traditional materials and can withstand temperatures exceeding 1000°C.However, the development of RHEAs and RCCAs also faces major challenges. They are easily oxidized, which is particularly prominent in many high-temperature environments and may seriously weaken their mechanical properties.
Although high-temperature oxidation processes are controlled by complex thermodynamic and kinetic factors, involving the formation, growth, dissolution and exfoliation of oxide layers, researchers have long relied on empirical observations and complex physical models to predict the oxidation behavior of alloys, but these traditional methods have significant limitations.
With the continuous development of artificial intelligence technology, high-throughput computing methods and advanced characterization techniques have provided new ideas for the study of RHEAs and RCCAs.
XGBoost’s “Highlight Moment”: Increasing the content of Al, Cr, and Si can effectively improve the oxidation resistance of the alloy
The performance of AI models is highly dependent on the quality of training data. In order to predict the oxidation resistance of alloys, researchers need to build an accurate dataset that links the chemical composition of the alloy, oxidation conditions (such as temperature and time) and oxidation resistance indicators (such as mass gain). However, the oxidation behavior of alloys involves complex physical and chemical processes, including element diffusion, microstructural changes, oxide stability, and environmental interactions.Currently, there is no large database readily available for direct use in the scientific community.
To this end, the researchers of this study "mined" from published literature and extracted a large amount of experimental data.A comprehensive dataset containing 886 observations was constructed.The data covers traditional refractory alloys and RHEAs/RCCAs composed of 11 elements (Al, Cr, Hf, Mo, Nb, Si, Ta, Ti, V, W, and Zr), with detailed records of alloy composition, oxidation test temperature, and exposure time. These descriptors are controllable during the alloy manufacturing process and lay the foundation for subsequent machine learning modeling.
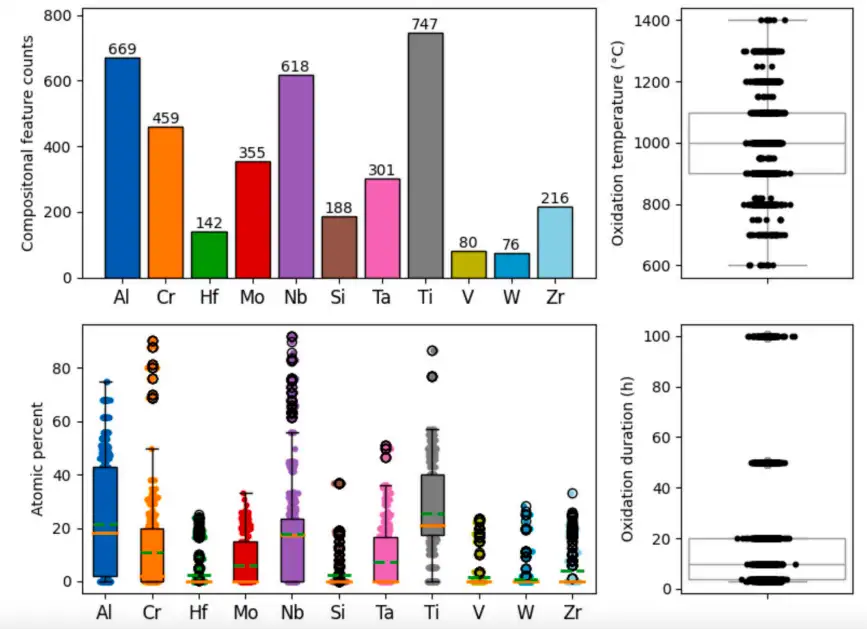
In order to more intuitively understand the relationship between different alloy components in the data set, the researchers visualized the data, using 163 nodes to represent different alloys, and using the color of each node to represent the element with the highest molar percentage. The results show thatThree main high-concentration areas were formed in the data set:As shown in the figure below: the blue area is dominated by Al, the orange area is dominated by Cr, and the purple area is dominated by Nb. These areas reflect the importance of different elements in alloy design.
To further enrich the dataset,The researchers randomly extracted nine components from these high-concentration areas and synthesized nine "random" alloys using arc melting technology.The addition of these new alloys not only expands the diversity of the dataset, but also provides more samples for model training and validation.
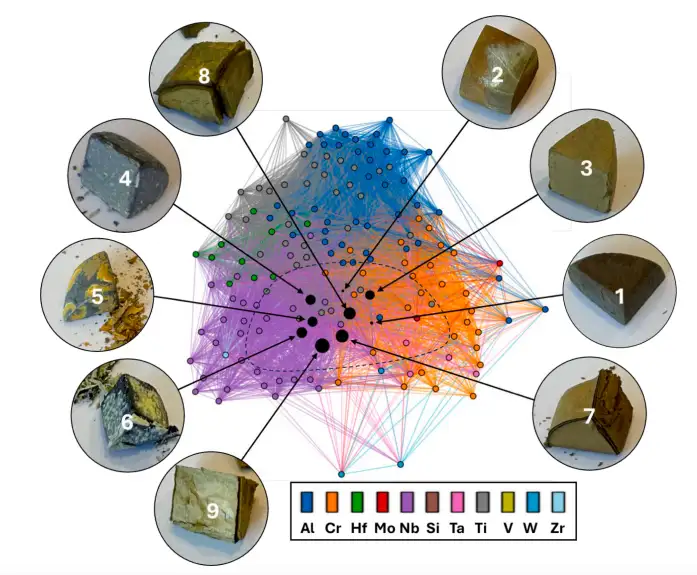
Since the dataset was relatively small and antioxidant activity involved complex nonlinear relationships, the researchers chose the XGBoost (Extreme Gradient Boosting) algorithm, an efficient gradient boosted decision tree (GBDT) model. XGBoost is known for its ability to handle nonlinear relationships and feature interactions.Very suitable for solving this type of problem.
After training the model on the entire dataset of 886 observations, the results show that XGBoost performs well on multiple performance metrics.Compared with the traditional multivariate linear regression model (MLR), XGBoost has achieved significant improvements in the coefficient of determination (R²), root mean square error (RMSE), and mean absolute error (MAE), which means that XGBoost can more accurately predict the oxidation resistance of the alloy.
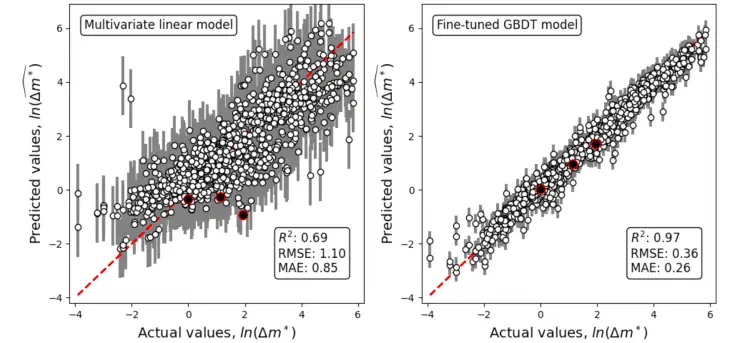
To further understand which factors have the greatest impact on antioxidant activity, the researchers calculated SHAP values (Shapley Additive Explanations), a method for explaining the output of machine learning models. The results showed:
* Oxidation temperature and time are key factors affecting oxidation resistance. The higher the temperature and the longer the time, the greater the mass gain of the alloy and the worse the oxidation resistance.
* The concentrations of Nb, Zr, V, Ti, W, and Hf were positively correlated with the mass gain, indicating that these elements have an adverse effect on the oxidation resistance.
* On the contrary, increasing concentrations of Al, Mo, Cr, Ta, and Si reduce the mass gain and thus enhance the oxidation resistance.
These findings not only verify known scientific laws, but also provide important guidance for future alloy design.Increasing the content of Al (aluminum), Cr (chromium) and Si (silicon) can effectively improve the oxidation resistance of the alloy.
The rise of China's high-temperature alloy industry and the support of artificial intelligence
In the field of global materials science, high-temperature alloys have been regarded as strategic materials because of their irreplaceable role in key fields such as aerospace and energy development. However, due to its complex production technology, long R&D cycle and large capital investment, the high-temperature alloy industry has long been monopolized by a few international giants, forming an obvious oligopoly. Developed countries represented by the United States have taken advantage of their first-mover advantage.Industry giants such as Pratt & Whitney (PCC), Carpenter and Haynes International have been formed.These companies not only master core technologies and patents, but also firmly control the global market through vertical integration and global layout.
In contrast, China's high-temperature alloy industry started late but has developed rapidly.China has made the leap from "following" to "running side by side" in the field of high-temperature alloys, and has even become a "leader" in some areas.
At present, domestic institutions engaged in the research and development and preparation of high-temperature alloys include research institutes such as the Central Iron and Steel Research Institute, Beijing Institute of Aeronautical Materials, Institute of Metal Research, Chinese Academy of Sciences, University of Science and Technology Beijing, and high-quality enterprises such as China Iron and Steel Research Institute, Western Superconducting, AVIC Shanghai University, and Tunan Co., Ltd. Through years of technological accumulation and innovation, these institutions have achieved a breakthrough from "nothing" to "something", and have reached international advanced levels in some areas.
However, the performance optimization of high-temperature alloys is a complex multi-objective problem, which requires finding the best balance between multiple indicators such as high-temperature strength, room-temperature ductility, and oxidation resistance. In recent years, the introduction of artificial intelligence technology has brought revolutionary breakthroughs in the design of high-temperature alloys. With the continuous integration of AI for Science and the field of materials science, domestic researchers have begun to focus on new breakthroughs in AI technology in the field of high-temperature alloys, and have made many advances in the past 2024.
For example, Su Yanjing's team at the University of Science and Technology Beijing proposed a multi-objective optimization (MOO) framework that combines machine learning, genetic search, cluster analysis, and experimental feedback.Used to design refractory high entropy alloys (RHEAs) with optimal high-temperature strength and room-temperature ductility.The team synthesized 24 RHEAs and experimentally verified that the ZrNbMoHfTa alloy exhibited excellent performance at high temperatures.
Prior to this, the research team of the University of Science and Technology of China and the Institute of Metal Research of the Chinese Academy of Sciences focused on the design of high-temperature alloys for additive manufacturing, using machine learning technology.The key issue of "balancing crack sensitivity and high temperature performance" is solved.The study details the basic methods of machine learning-assisted high-temperature alloy design, including data collection and preprocessing, model building and training, and alloy performance prediction. Through machine learning, the research team was able to quickly screen out high-temperature alloy components suitable for additive manufacturing, significantly shortening the research and development cycle.
The related results were published in "Smart Security" under the title "Design of Nickel-based Superalloys for Additive Manufacturing Based on Machine Learning: Research Status and Future Trends".
Paper link:
10.12407/j.issn.2097-2075.2024.02.096
Although China has made significant progress in the field of high-temperature alloys, there is still a certain gap compared with international giants. For example, the stability and consistency of high-end products still need to be further improved. In addition, the industrial chain of high-temperature alloys has not yet been fully self-controlled, and some key raw materials and equipment still rely on imports.
However, with the widespread application of emerging technologies such as artificial intelligence and big data, China's high-temperature alloy industry is welcoming new development opportunities. Through AI technology, researchers can design new materials and optimize production processes more efficiently, thereby accelerating technological breakthroughs. In the future, China is expected to achieve a leap from "running side by side" to "leading" in the field of high-temperature alloys, contributing more "Chinese wisdom" to global industrial development.
References:
1.http://znaq.ijournals.cn/znaq/article/abstract/20240124001?st=article_issue
2.https://www.sohu.com/a/739946600_120113054
3.https://www.chyxx.com/industry/1194170.html
4.https://baijiahao.baidu.com/s?