AlphaFolding Fills the Gap in Protein Dynamic Structure Prediction! Fudan University and Others Proposed a 4D Diffusion Model, and the Results Were Selected for AAAI 2025
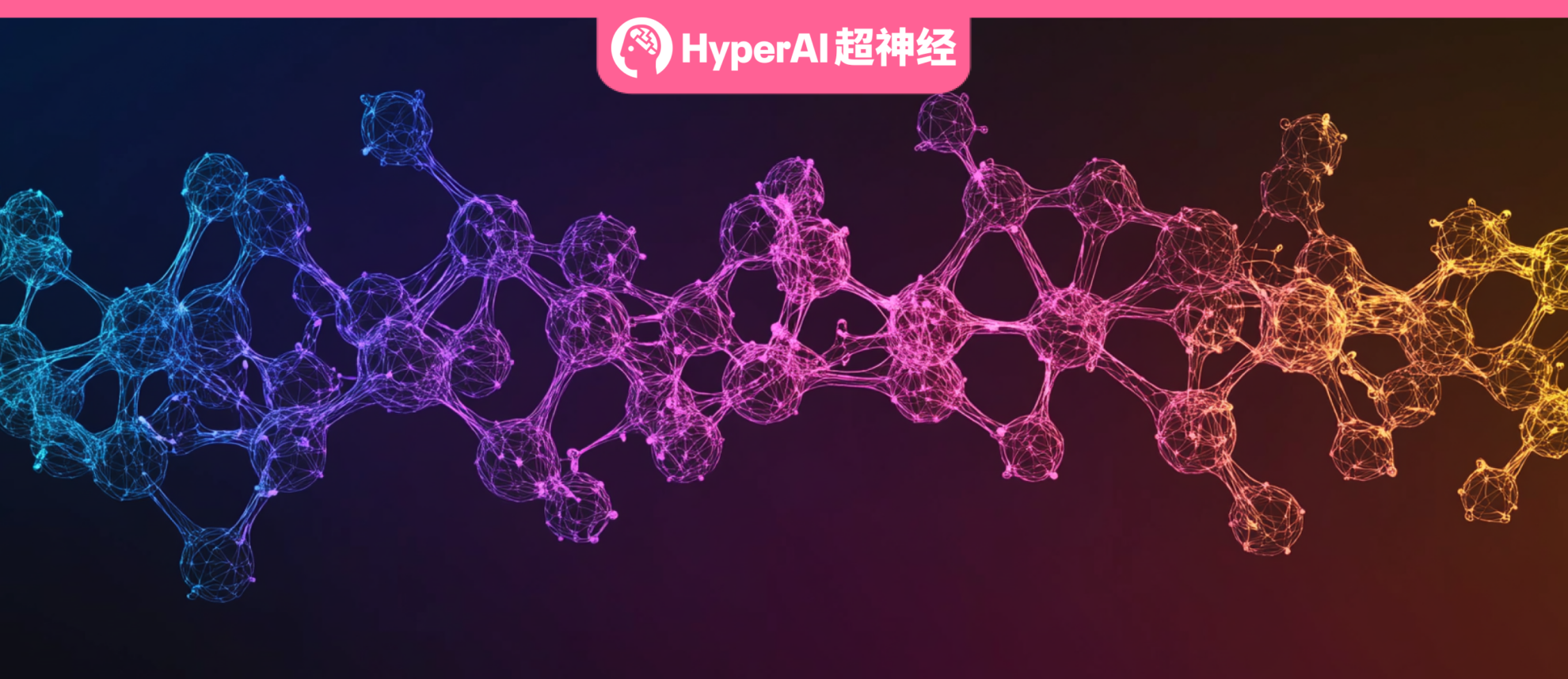
The function of a protein depends largely on its 3D structure. In the mid-19th century, the scientific community generally believed that protein structure was fixed and rigid, similar to the "lock-and-key model".That is, the binding of protein and ligand is determined by a fixed three-dimensional structure.However, traditional thinking began to be challenged when Daniel Koshland proposed the idea that enzymes undergo conformational changes when they bind to substrates.
In the 1980s, molecular dynamics simulation (MD) emerged.For the first time, the movement trajectory of proteins was revealed from a computational perspective.Since then, the functional role of protein dynamic structure has received more and more attention. For biotechnology researchers and scientists, understanding the dynamic characteristics of protein "movement" is of great significance to understanding life processes and developing new drugs.
For example, G protein-coupled receptors (GPCRs) are the main targets of many drugs, accounting for more than 30% of the current FDA-approved drugs. However, GPCRs are not rigid structures, but are highly dynamic, and different conformational states can affect drug binding patterns. If drugs are designed based solely on static crystal structures, key binding sites may be missed, resulting in insufficient drug affinity and selectivity. Dynamic structure prediction can help identify multiple conformations of GPCRs in physiological environments,This will optimize the design of small molecule drugs and improve the success rate of targeted therapy.
In this context, the team of Professors Zhu Siyu and Qi Yuan from Fudan University and Shanghai Institute of Science and Intelligence, together with Professor Yao Yao from Nanjing University,An innovative 4D diffusion model AlphaFolding is proposed.Combined with molecular dynamics simulation data to learn dynamic protein structure, this is the first diffusion-based method that can predict protein motion trajectories over multiple time steps simultaneously.
Validation results on benchmark datasets show that the new model exhibits high accuracy in predicting dynamic 3D structures containing up to 256 amino acids and spanning 32 time steps, and can effectively capture local flexibility in stable states as well as significant conformational changes.
The related results, titled "4D Diffusion for Dynamic Protein Structure Prediction with Reference and Motion Guidance", have been selected for the top international conference AAAI 2025, and the preprint has been published on arXiv.

Paper address:
https://arxiv.org/abs/2408.12419
Follow the official account and reply "4D Diffusible Proteins" to get the full PDF
The open source project "awesome-ai4s" brings together more than 200 AI4S paper interpretations and provides massive data sets and tools:
https://github.com/hyperai/awesome-ai4s
There is still a gap in the study of protein dynamic structure prediction
The AlphaFolding model can be seen as an important advance in structural biology research. Structural biology is a science that explains life phenomena based on the study of the structure, movement and interaction of biological macromolecules such as proteins. It has now developed into the mainstream of molecular biology.
In recent years, the advancement of deep learning technology, coupled with the exponential growth of experimental protein structure data in the Protein Data Bank (PDB), has led to a number of important breakthroughs in the field of protein structure prediction. Among them, the most well-known is AlphaFold2.It uses the latest artificial intelligence algorithms to achieve accurate predictions of protein structures close to experimental accuracy.The related results were rated as one of the top ten scientific breakthroughs of 2020 by Science.
Coincidentally, in July 2021, RoseTTAFold, developed by the team of biologist David Baker at the University of Washington, built a "three-track" neural network.The three-dimensional structure of a protein with a given sequence can be resolved within a dozen minutes.
In addition, the availability of large-scale data repositories has facilitated the development of protein conformation sampling studies. For example, Microsoft Research has developed a deep learning framework called Distributional Graphformer (DiG)Aims to predict the distribution of molecular structures in equilibrium.Although traditional molecular dynamics simulation and enhanced sampling methods can obtain the equilibrium distribution of molecules, these methods are computationally expensive and time-consuming, making them difficult to apply to complex practical application scenarios. DiG, on the other hand, uses deep learning technology to quickly generate realistic and diverse conformations.
Although significant breakthroughs have been made in the prediction of protein structure and its conformation, the study of dynamic structure is still relatively lagging behind. Take AlphaFold2 as an example, which can accurately predict the three-dimensional structure of proteins.However, it can only predict the static structure of a protein at a moment in time, and is not yet able to predict dynamic changes.
In May 2024, DeepMind released the upgraded AlphaFold3, which can predict the structure and interactions of all biological molecules with unprecedented "atomic precision", including the 3D structure of proteins, nucleic acids and smaller molecules, and reveal how they are combined together.However, its prediction of dynamic 3D structures of biological molecules still has great limitations.
Therefore, the innovative 4D diffusion model proposed in this study is actually to fill this research gap, focusing on the dynamic characteristics of protein structure and providing new ideas for a deeper understanding of protein function. The researchers made full use of high-quality molecular dynamics simulation (MD) data.Generate dynamic protein structures with full side-chain representations for complex proteins consisting of hundreds of amino acids.This will expand the scope of applicability of MD simulations, enabling them to predict the dynamic behavior of larger and more complex protein systems and improve our understanding of protein dynamic properties.
Demonstrates high accuracy in predicting protein motion trajectories over multiple time steps
Static protein models are relatively easy to construct, but how to represent dynamic protein models? To solve this problem,The researchers used AlphaFold2's frame-based protein structure representation method and extended it to the time dimension.To describe the structural transformation over time.
In static protein modeling, proteins are composed of a series of amino acid residues, each of which is parameterized by a backbone framework. In this study, the researchers defined dynamic proteins as systems containing N amino acid residues whose backbone frameworks transform in S time steps. These frames are transformed by special Euclidean transformations to maintain the orientation of the local frame to the global reference frame.
All additional atomic coordinates in proteins are organized into rigid groups according to their dependencies on dihedral angles to ensure chemical structural integrity. Within each rigid group, the relative positions and orientations of all atoms remain unchanged. Combined with transformation parameters, the model can reconstruct all atomic positions from idealized experimental coordinates in the time dimension.
On this basis, the figure below shows the method of building the entire research model: the diffusion model takes the reference structure and the corresponding residue sequence (amino acid residues sequence) as input, and generates a series of denoised 3D protein structures (denoised 3D structure) as output.

The researchers used 3D structure embedder and GeoFormer to embed 3D protein structure and residue sequence, respectively. Invariant Point Attention (IPA) updates node features by incorporating explicit framework information of residues.
The Reference Network and Motion Alignment modules capture the 3D protein dynamics sequence based on the reference 3D protein structure. The entire generative model is constructed as a score-based diffusion model, where the feature embeddings of nodes and edges are updated through EdgeUpdate and BackboneUpdate modules, respectively.
After building the model, the researchers conducted comparative experiments on the proposed framework with DFF and Flow-Matching in current short-term-to-long-term (S2L) tasks, using data sets including ATLAS and Fast-Folding Proteins.
The results are shown in the following table: In the S2L task on the ATLAS dataset, the proposed method reduces R32 The error was reduced from 4.60 to 2.12,Significantly improved the accuracy of long-term forecasts;In the S2L task on the Fast-Folding dataset, the proposed method converts R32 The error was reduced from 5.48 to 4.39,It also shows good long-term predictive ability.At the same time, the performance of the proposed model on the O2O task is comparable to that on the S2L task.This indicates its excellent generalization ability.


Furthermore, the method is able to handle proteins with longer simulation times, which have larger dynamical changes at each trajectory step.The experimental results further verified the effectiveness of this method in modeling protein kinetics.
Furthermore, the researchers also generated dynamic protein distributions of the first two TICs (temporal consistency components) through the visualization model and compared them with real data. As shown in the figure below,The new model effectively predicts the dynamic behavior of the protein and is highly consistent with the true distribution.

* The darker the point, the higher its frequency. The blue curve represents the kernel density distribution estimated from the MD data.
The figure below shows the reverse diffusion process at a selected time step, highlighting how the protein structure gradually becomes more consistent during the denoising process.The proposed method effectively captures the dynamics of the protein and generates reasonable trajectories.

* The pink and yellow areas indicate α-helix and β-sheet, respectively
The dynamic properties of protein structures will receive more attention
Proteins do not exist statically in the cellular environment, but are in complex dynamic changes. Although traditional static structure prediction methods have made important progress in revealing protein folding and interactions, they cannot fully capture the dynamic behavior of proteins.Dynamic protein structure prediction has become one of the frontier challenges in structural biology and computational biology.In recent years, more and more researchers have devoted themselves to this direction.
In December 2022, Li Ziqing's team from Westlake University collaborated with Xiamen University and Deruizhi Pharmaceuticals.We developed ProtMD, an AI model that can characterize protein conformational changes and predict affinity.This is the first AI method that attempts to analyze the dynamic conformation of proteins. Given a drug molecule and a target protein, ProtMD predicts the changes in protein structure after the drug molecule binds to the target protein in the body, infers the stability of the drug-target protein binding, and predicts the drug function, thereby improving the accuracy and efficiency of AI drug design and accelerating preclinical drug development.
The relevant research results were published in Advanced Science under the title "Pre-Training of Equivariant Graph Matching Networks with Conformation Flexibility for Drug Binding".
* Paper address:
https://advanced.onlinelibrary.wiley.com/doi/full/10.1002/advs.202203796
In August 2024, a new study from the University of Connecticut revealed an advanced computational model and tool thatAble to accurately predict the dynamic characteristics of proteins and their crystallization tendency,The relevant research results were published in the materials science journal Matter under the title "Protein dynamics inform protein structure: An interdisciplinary investigation of protein crystallization propensity". The research focuses on how the natural movement and fluctuation of proteins, that is, their swaying characteristics, affect their functional properties, especially the ability of proteins to form high-quality crystals.
In October 2024, the research group led by Zheng Shuangjia from Shanghai Jiao Tong University, together with Star Pharma Technology, Sun Yat-sen University School of Pharmacy and Rice University,A geometric deep generative model DynamicBind designed for protein dynamic docking is proposed.It can effectively adjust the protein conformation from the initial AlphaFold predicted state to a holo-like state, providing a new research paradigm based on deep learning and considering the dynamic changes of proteins for drug development in the post-AlphaFold era.
The related research was published in Nature Communications under the title “DynamicBind: predicting ligand-specific protein-ligand complex structure with a deep equivariant generative model”.
In summary, dynamic protein structure prediction can not only help us understand life processes, but also play an important role in drug development, disease mechanism research, industrial biotechnology and other fields. From GPCR drug design, protein-protein interaction, to enzyme catalysis and protein aggregation pathology research, dynamic structure prediction will continue to promote the frontier development of life sciences.
References:
1.https://www.forwardpathway.com/119037
2.https://www.westlake.edu.cn/news_events/westlakenews/academics/202212/t20221208_24193.shtml
3.https://www.cell.com/matter/abstract/S2590-2385(24)00196-6
