Superconducting Material Search Efficiency Increased by 5 Times! University of Florida and Others Use Deep Learning to Transform Material Discovery, and the Results Are Published in Nature
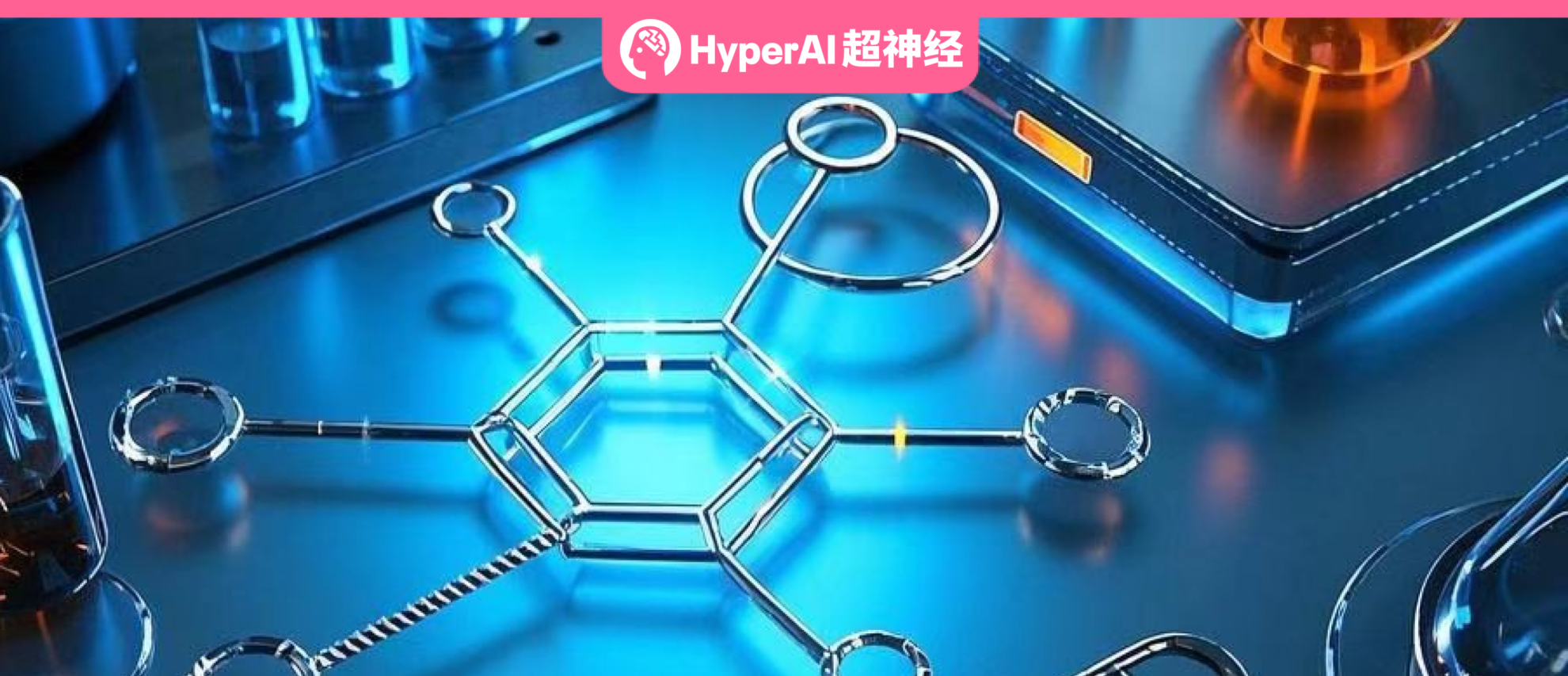
In the science fiction movie "Avatar", the Hallelujah Mountain on Pandora, which is entangled with green vines and suspended in the clouds, undoubtedly left a deep impression on the audience. The secret of its suspension lies in the room-temperature superconducting ore "Unobtanium" hidden in the mountains. In the movie, humans destroy the homes of the Na'vi in order to snatch this treasure that does not exist on Earth. Although this is just a fictional story, in reality, physicists' desire and pursuit for superconducting materials is no less than the human obsession with "Unobtanium" in the movie. Because in theory, superconducting materials can provide an inexhaustible supply of energy.
Researchers' research on superconducting materials reached a small climax in 2023. At that time, the Korean team claimed to have discovered the room-temperature superconducting material LK-99, which attracted widespread attention from the world for a time, and was even regarded by some as another major technological breakthrough after ChatGPT. Although this discovery was eventually confirmed to be a mistake, it has once again made the popularity of superconducting materials soar, and has also made people see the huge potential of this field.
With the rise of AI for Science, people began to boldly imagine: Can AI technology be used to discover room-temperature superconducting materials? Theoretically, this is entirely feasible. Although there are still challenges, some research teams have taken an important step in this regard.For example, researchers from the University of Florida and the University of Tennessee in the United States successfully predicted the electroacoustic interaction Eliashberg spectral function α²F(ω) of metals through the deep learning model BETE-NET, and increased the efficiency of searching for high-Tc superconductors by 5 times.This achievement not only provides new ideas and methods for the discovery of superconducting materials, but also sets an example for the application of AI technology in the field of materials science.
The related results were published in the academic journal npj Computational Materials under the title "Accelerating superconductor discovery through tempered deep learning of the electron-phonon spectral function".

Paper address:https://www.nature.com/articles/s41524-024-01475-4
Follow the official account and reply "BETE-NET" to get the complete PDF
Dataset download address:
The open source project "awesome-ai4s" brings together more than 200 AI4S paper interpretations and provides massive data sets and tools:
https://github.com/hyperai/awesome-ai4s
The dilemma of superconducting materials: the "dilemma" between training data sets and machine learning techniques
The effectiveness of AI models for superconductors generally depends on two key factors: the training dataset and the choice of machine learning technique.Only by solving these two key factors can we better promote the research and development of superconducting materials and lay a solid foundation for future scientific and technological innovation. However, both directions face many difficulties.
First, models that can quickly and accurately estimate the superconducting properties of metals usually require tens of thousands of data points from materials informatics databases. However, unlike other databases, it is extremely difficult to develop a corresponding large-scale α²F(ω) data set. This is not only because of its high cost, but also because of the lack of a set of standardized density functional theory parameters (such as k-point and q-point density, smoothing values, etc.) to accurately calculate α²F(ω). In view of these obstacles, scientists need a set of machine learning techniques that can effectively handle small data sets to break through the current difficulties and promote the development of superconducting property estimation models.
Secondly, in the field of superconductivity research, researchers often face a major challenge when using such models: the available data sets are often uneven and limited in number. For a long time, the study of superconducting materials has mainly relied on the well-known "SuperCon" database containing experimental Tc values to solve the problem of limited data on superconducting properties. However, the database has many problems, such as being full of duplicate entries, questionable values, and ambiguous chemical formulas. This lack of large and comprehensive data sets not only greatly limits the development of new superconducting materials, but also seriously hinders the revolutionary potential of superconducting materials in energy transmission, magnetic levitation in the field of transportation, and powerful superconducting magnets in medical imaging.
Although a number of databases of material structures and calculated α²F(ω) have emerged, such as the Superhydra database proposed by the Institute of Theoretical and Computational Physics of Graz University of Technology, which only contains high-pressure hydrides, the database launched by the Halle Institute for Physics in Germany that focuses on Heusler superconductors, the model trained by researchers from the Department of Physics of the University of Coimbra in Portugal based on 7,000 electron-phonon calculations, and the database developed by the National Institute of Standards and Technology in the United States that contains 626 dynamically stable materials and their related α²F(ω), these databases still perform poorly in predicting α²F(ω).
In order to better solve these problems,In this study, researchers from the University of Florida and the University of Tennessee successfully addressed these two key elements by creating a comprehensive Eliashberg spectral function dataset and developing a robust model using modern deep learning techniques, opening up new avenues for the research and development of superconducting materials.This achievement not only provides new methods and tools for the research of superconducting materials, but also lays a solid foundation for future scientific and technological innovation and application.
BETE-NET: Significantly Expanding the Boundaries of Computational Exploration with Limited Data
When calculating electron-phonon coupling, it is necessary to ensure that the k-point mesh used to calculate the Kohn-Sham wave function matches the q-point mesh used to calculate the phonons. To address the problem with the dataset, the study first proposed an algorithm for standardized selection of k and q meshes, which generates meshes based on user-provided k and q point densities, rather than using fixed meshes to handle materials with different unit cell volumes. With this approach, the researchers not only improved the uniformity and quality of the data, but also ensured the wide applicability of the dataset.The result is a comprehensive database of high-quality electron-phonon calculations for 818 dynamically stable materials.Next, the researchers divided the 818 dynamically stable materials into training and test sets in the ratio of 80%-20%.
Dataset download address:
https://go.hyper.ai/GjZDo
After evaluating the quality of the dataset, the researchers further designed BETE-NET to address the deep learning problem of limited database size.As shown in the figure below, the BETE-NET model converts the crystal structure into a graph and combines the atomic number, atomic mass, interatomic distance, and PhDOS information of the site projection through a series of convolution and gated-block operations, and finally generates a prediction of α²F(ω) through pooling operations. By introducing PhDOS information, the prediction performance of the model has been significantly improved. This design not only makes full use of the information of the crystal structure, but also combines the vibration characteristics of the material, making the model more accurate and reliable in predicting the α²F(ω) of superconducting materials. In the end, the study trained 3 variants:
* CSO (Crystal Structure Only) variant: the base network of the model, using only crystal structure information for predictions.
* CPD (coarse PhDOS) variant: The model introduces site-projected phonon density of states (PhDOS) information, further improving the predictive performance of the model.
* FPD (Fine PhDOS) variant: PhDOS is calculated using a finer q-mesh, further improving the model's ability to capture the material's vibrational properties.

With limited data, models tend to overfit quickly. Although traditional machine learning believes that overfitting is harmful to the generalization ability of the model, many deep learning models can still maintain good generalization error when trained to near-zero loss. This phenomenon is called "double descent" and can be regarded as a controlled overfitting. As shown in the figure below, the double descent phenomenon consists of 3 stages: Classical Rigime, Critical Regime, and Modern Regime, and the loss landscape of each stage is shown in the illustration. By drawing these loss landscapes, the researchers proposed a reasonable method to intuitively explain the bias and variance of neural networks, thereby providing a qualitative explanation for the double descent phenomenon.

This study was further validated in the screening of high Tc materials.First, the study defined all materials with Tc^DFT≥5K as high-T materials, and finally 33 materials met this criterion. Then, the study plotted the precision-recall curves for each model. The results showed that the average precision (AP) obtained by the CPD and FPD models was almost 5 times that of the random classifier. This shows that these models perform well in identifying high-Tc materials, significantly outperforming random classifiers, thus verifying the effectiveness and reliability of the models in practical applications.

In summary, the BETE-NET model is a perfect example of the perfect fusion of domain-specific knowledge and advanced deep learning technology. It can efficiently predict the electroacoustic interaction Eliashberg spectral function α²F(ω) of metals with limited data. It not only significantly expands the boundaries of computational exploration, but is also expected to bring transformative social impact by assisting the discovery of new superconductors.
Equivariant Neural Networks: Helping the Birth of AlphaFold in the Materials Industry
Interestingly, shortly after this study was published, on January 17,Microsoft CEO Satya Nadella personally announced that its MatterGen model was published in Nature magazine.This model can go beyond currently known materials and use AI to discover new materials for specific needs. This marks a new paradigm in the field of material design: from traditional database screening to directly generating new materials based on demand prompts. Some netizens exclaimed: "The AlphaFold of the materials world is here."
It is worth noting that the key to the MatterGen model lies in its unique diffusion model architecture. In this diffusion process, the MatterGen model uses an equivariant fractional network, which is also the model selected in this study when learning α²F(ω). It is mainly responsible for recovering the original crystal structure from the diffusion process, that is, the denoising process.
Equivariant neural networks add equivariant constraints to traditional neural networks. Each operation in the network is required to be equivariant, so the entire network is an equivariant mapping. In fact, equivariant neural networks have become the mainstream of AI for Science research in the field of materials.
Last September, researchers from Tohoku University in Japan and the Massachusetts Institute of Technology launched a new artificial intelligence tool called GNNOpt.By integrating equivariant neural networks, GNNOpt achieved high-quality predictions using a small dataset of 944 materials, successfully identifying 246 materials with solar energy conversion efficiencies exceeding 32% and 296 quantum materials with high quantum weights, greatly accelerating the discovery of energy and quantum materials.
Last August, the research group led by Xu Yong and Duan Wenhui from Tsinghua University proposed the neural-network DFT framework.This study uses the embedding of material structure information as input conditions for the equivariant neural network, and then outputs the Hamiltonian matrix, thereby unifying the minimization of the loss function in the neural network and the optimization of the energy functional in the density functional theory. Compared with traditional supervised learning methods, this framework has higher accuracy and efficiency, and has opened up new avenues for the development of deep learning DFT methods. Previously, the research team also proposed the xDeepH (extended DeepH) method, which uses a deep equivariant neural network framework to represent the DFT Hamiltonian of magnetic materials, thereby performing efficient electronic structure calculations.
Today, equivariant neural networks are leading the field of superconducting materials towards a new research paradigm. The research and application of superconducting materials are no longer limited to laboratories, but are gradually being integrated into real life, and their market potential is also being continuously released. It is predicted that the global superconducting materials market will continue to expand and is expected to increase to 19.2 billion euros by 2027. With the deep integration of AI technologies such as equivariant neural networks and superconducting materials, humans are touching the "transition point" of technology, ushering in a new era full of infinite possibilities.
