Open Source 176 Billion Parameter Universal Medical Language Model! BUPT/PKU/CTSU Proposed MedFound, Whose Reasoning Ability Is Close to That of Expert Physicians
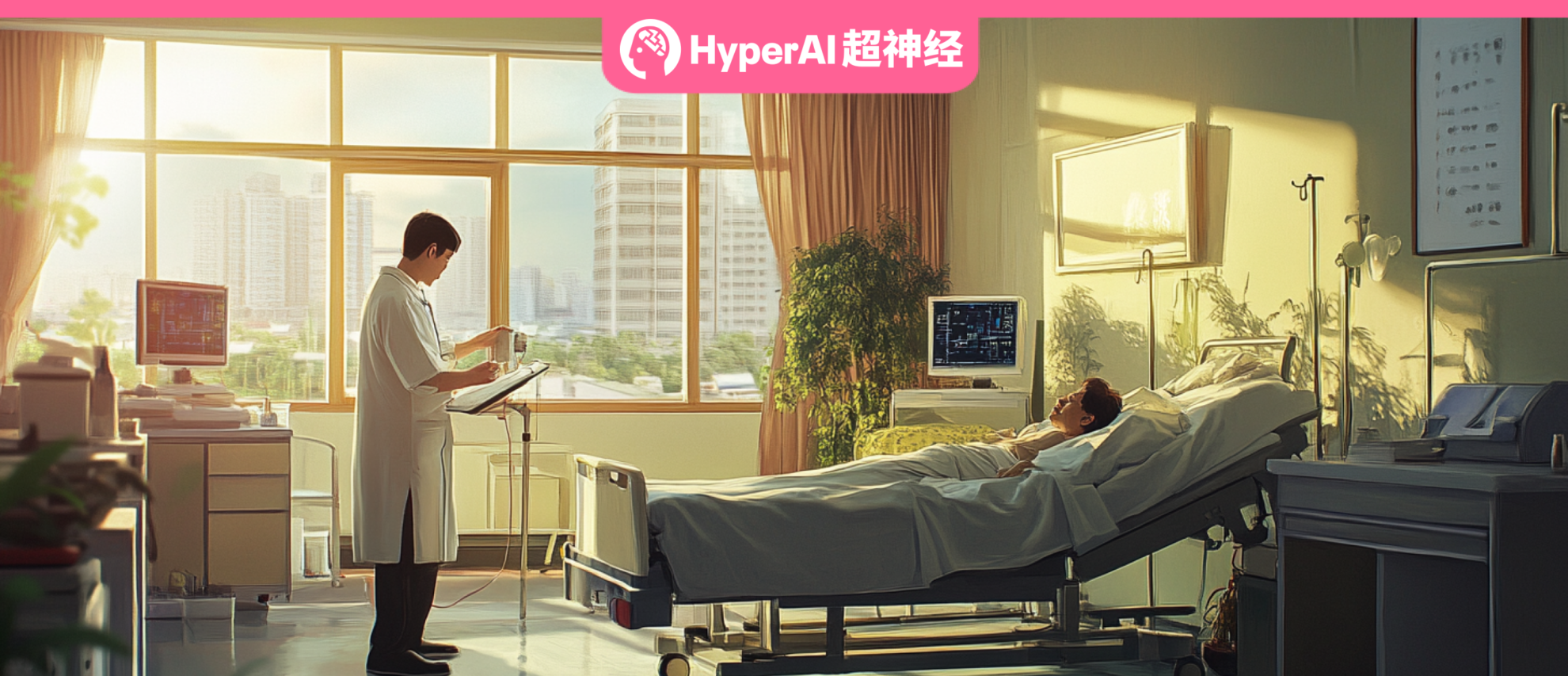
As the old saying goes, "No one is perfect, no one is without fault." However, in the medical field, "mistakes" such as misdiagnosis can have disastrous consequences. On the one hand, for patients, it can be a false alarm at best, or even a delay in the treatment of the disease. In either case, it can cause the patient to suffer mental, financial, or even life losses. On the other hand, for doctors, wrong judgments can damage the image of doctors who save the world at best, or even affect the credibility of the entire medical system. However, contrary to expectations, misdiagnosis is still a high-frequency event both at home and abroad.
Chen Xiaohong, who was the editor-in-chief of the journal "Clinical Misdiagnosis and Mistreatment" and one of the authors of the medical monograph "Misdiagnosis", mentioned in an interview that the misdiagnosis rate mentioned in the sample size of domestic and foreign literature is generally around 20% to 40%. In addition, there are relevant statistics in his book "Misdiagnosis", such as the misdiagnosis rate of 48 % in the 200 clinical pathology discussion materials reported by several representative domestic medical journals from 1973 to 1980. It can be said that misdiagnosis has almost become one of the main stumbling blocks on the road to the advancement of human medicine.
In order to solve the problem of misdiagnosis, in ancient times, medical works such as "Medical Records of Chinese and Western Medicine", "Mistakes of Doctors", and "Corrections of Medical Errors" all tried to compile the lessons of misdiagnosis in medical records as a warning to future generations; in modern times, with the assistance of modern medical methods such as B-ultrasound, CT, and magnetic resonance imaging, the means of clinical diagnosis have become increasingly rich and advanced. However, as a practical science and exploratory discipline, medicine cannot avoid the occurrence of misdiagnosis 100% anyway. Therefore, only by further reducing the misdiagnosis rate and improving the accuracy and accessibility of disease diagnosis can it be possible to clear the way for the further development of the medical cause.
AI for Science is a new paradigm that provides new ideas for solving the above problems.A medical-engineering cross-disciplinary team consisting of Professor Wang Guangyu from Beijing University of Posts and Telecommunications, Professor Song Chunli from Peking University Third Hospital, and Professor Yang Jian from China Three Gorges University introduced and verified MedFound (176B), the biomedical language model with the largest number of parameters.We have further created MedFound-DX-PA, a large language model for generalist medical diagnosis, which has knowledge and reasoning capabilities close to those of experts and can provide efficient and accurate diagnostic support across medical scenarios.
The related results were published in Nature Medicine under the title "A generalist medical language model for disease diagnosis assistance".

Paper address:
https://www.nature.com/articles/s41591-024-03416-6
Follow the official account and reply "MedFound" to get the complete PDF
The open source project "awesome-ai4s" brings together more than 200 AI4S paper interpretations and provides massive data sets and tools:
https://github.com/hyperai/awesome-ai4s
What is the innovation of MedFound?
The largest open source biomedical language model with the largest number of parameters
The research team said that the lack of well-designed, publicly available LLMs that are specifically tailored for real-world clinical settings is the key reason why LLMs are still in their infancy in biomedical applications. MedFound is pre-trained based on the general field large language model BLOOM-176B, which is a general medical large language model with a parameter scale of 176 billion.
In order to ensure that the model can obtain comprehensive general medical knowledge, the research team specially constructed a medical corpus dataset MedCorpus that integrates massive medical knowledge and clinical practice. It consists of a total of 6.3 billion text tags in 4 datasets, including MedText, PubMed Central Case Report (PMC-CR), MIMIC-III-Note and MedDX-Note. These datasets cover Chinese and English medical literature, professional books, and 8.7 million real electronic medical records, which are an important basis for the model to be applicable to diagnosis in various disciplines.
It is worth mentioning that according to the research team, MedFound is now open source and can provide underlying basic large model services to researchers, clinicians and medical institutions around the world.
Project address:
https://github.com/medfound/medfound?tab=readme-ov-file
Innovative clinical diagnostic reasoning capabilities make it a "living doctor"
In addition, an important difference between machines and humans is that human doctors can make reasonable inferences about the patient's true condition based on their own experience and knowledge reserves, and thus provide differentiated treatments. The research team introduced that some current studies only incorporate clinical knowledge into LLM for medical Q&A or dialogue, but do not reflect the ability of clinical diagnostic reasoning.
For example, Sainan Zhang and Jisung Song published a result in Nature. They developed a conversational interface named Chat Ella after transfer learning and fine-tuning based on GPT-2. The system can accurately predict chronic diseases based on the symptoms described by the user. However, at the end of the paper, the researchers also mentioned the shortcomings of the research, pointing out some limitations of the result in the reasoning process, such as the inability to explain the reasoning process. The paper is titled "A chatbot based question and answer system for the auxiliary diagnosis of chronic diseases based on large language model".
Paper address:
https://www.nature.com/articles/s41598-024-67429-4
Therefore, in order to achieve rigorous disease diagnosis, it is not enough for the big model to have extensive interdisciplinary medical knowledge, but it also needs to be able to perform complex reasoning.Based on the MedFound model, the research team further created MedFound-DX, a large language model for generalist medical diagnosis with knowledge and reasoning capabilities close to those of experts, through two-stage training optimization.As shown in the following figure:

Specifically, in the first phase, the research team used a self-guided strategy-based Chain of Thought (CoT) approach to enable the large model to automatically generate diagnostic evidence and reasoning processes like medical experts. However, generative LLMs may produce "hallucinations" or fabricate false facts, and if these diagnoses are adopted, the consequences will be disastrous.
Therefore, in the second phase, the research team also introduced a unified preference alignment framework to align LLM with the knowledge system of professional fields and clinical diagnostic preferences to ensure that the model can be scientific and reasonable when making diagnoses, and at the same time conform to the logic and values of medical experts in clinical practice. This framework integrates "diagnostic hierarchy preference" and "helpfulness preference", both of which use the direct preference optimization algorithm (DPO) - a simple algorithm that does not require reinforcement learning. On the one hand, it can guide the model to improve the fine-grained accuracy of disease identification, and on the other hand, it can also improve the effectiveness and credibility of model reasoning and reduce the risk of misleading and incorrect information.
It is worth mentioning that in the fine-tuning and alignment of this part, the research team also specially built a dataset called MedDX-FT, which contains demonstrations of reasoning processes manually written by doctors based on real medical records for training and fine-tuning. The dataset covers a seed set based on manual demonstrations and 109,364 EHR notes.
Amazing demonstration results show its potential application capabilities
During the evaluation phase, the research team also constructed a dataset MedDX-Bench, which includes three clinical datasets: MedDX-Test, MedDX-OOD and MedDX-Rare.
* The MedDX-Test dataset is used to evaluate the diagnostic performance of MedFound-DX-PA in various fields and contains 11,662 medical records with the same distribution as the training dataset.
* MedDX-OOD and MedDX-Rare are external validation sets, the former contains 23,917 records of common diseases, and the latter contains 20,257 records of 2,105 rare diseases, which have a long-tail distribution.
The evaluation experiment mainly consists of three stages, namely in-distribution (ID) evaluation, out-of-distribution (OOD) evaluation and long-tail disease distribution evaluation. The comparison objects include leading open source and closed source LLMs such as MEDITRON-70B, Clinical Camel-70B, Llama 3-70B and GPT-4o.
The results show that its performance is better than other leading LLMs.For example, in the diagnostic performance of common diseases, the average Top-3 accuracy of MedFound-DX-PA is 84.2% (under ID setting), in comparison, the diagnostic accuracy of GPT-4o is only 62%; in the diagnostic performance of rare diseases, the average Top-3 accuracy of MedFound-DX-PA in 8 specialties is 80.7%, and GPT-4o ranks second with an average of 59.1%.
It is worth mentioning that in the comparison between MedFound-DX-PA and endocrinologists and pulmonologists, the diagnostic accuracy rates were 74.7% and 72.6% respectively, which was much better than that of doctors with lower and middle years of experience, and comparable to that of doctors with higher years of experience. In terms of auxiliary diagnosis, it can help doctors in these two departments improve their diagnostic accuracy by 11.9% and 4.4% respectively. The figure below is an intuitive model diagnosis case.
As shown in the figure below, the doctor's initial diagnosis was acute bronchitis. The MedFound model highlighted the patient's history of recurrent bronchitis. With the model's prompt, the doctor revised the diagnosis to acute exacerbation of chronic bronchitis.

As shown in the figure below, the doctor initially diagnosed the patient with subclinical hypothyroidism. The MedFound model suggested the possibility of underlying autoimmune thyroid disease, and the doctor revised the result to autoimmune thyroiditis.

It can be seen that MedFound not only has the potential to improve diagnostic efficiency and accuracy, but also has the potential to become a diagnostic assistant for clinical workers.This provides strong support for the future development of intelligent clinical diagnosis and treatment and personalized medicine.
AI4S continues to make progress, and the era of implementation has arrived
Wang Guangyu's team keeps moving forward
In this collaborative effort, each team did their best to contribute to this achievement using their expertise. It is worth mentioning that Professor Wang Guangyu from Beijing University of Posts and Telecommunications is one of the corresponding authors of this study.
In fact, this is not the first time that Professor Wang Guangyu’s team has integrated AI with biomedicine.As the first post-90s winner of the Science Exploration Award, Wang Guangyu has long been famous and has published a series of internationally cutting-edge academic achievements.His works have been included in top international academic journals such as Cell, Nature Medicine, and Nature Biomedical Engineering.

For example, in 2020, Professor Wang Guangyu, as the first corresponding author, published a study titled "Clinically Applicable AI System for Accurate Diagnosis and Prognosis of COVID-19 Pneumonia Using Computed Tomography" in the top international journal Cell. The study focused on the then-raging COVID-19 pneumonia, and used a total of more than 530,000 CT images to build an AI diagnostic model based on lesion segmentation, with a diagnostic accuracy rate of up to 92.49%.
Paper address:
https://www.cell.com/pb-assets/products/coronavirus/CELL_CELL-D-20-00656.pdf

In 2023, Wang Guangyu's team once again published two research papers in Nature Medicine. One paper, titled "Deep-learning-enabled protein–protein interaction analysis for prediction of SARS-CoV-2 infectivity and variant evolution", proposed an artificial intelligence framework called UniBild, which can effectively and scalably predict the impact of SARS-CoV-2 spike protein variants on humans.
Paper address:
https://www.nature.com/articles/s41591-023-02483-5

Another paper, titled "Optimized glycemic control of type 2 diabetes with reinforcement learning: a proof-of-concept trial", proposes a model-based reinforcement learning framework RL-DITR, including a patient model that tracks individual blood sugar status and a policy model for multi-step planning of long-term care, which can help doctors and patients specify dynamic and flexible insulin treatment plans.
Paper address:
https://www.nature.com/articles/s41591-023-02552-9
As Wang Guangyu said, "We have expectations for this. For myself, I hope to develop more powerful AI methods and use them to solve many important biomedical problems, such as conquering sudden epidemics or cancer."
The integration of AI and biomedicine is accelerating
In fact, the integration of AI and biomedicine has long been a focus of major laboratories. Due to the particularity of the medical field, AI has more opportunities to play a role in this field, and more teams are willing to delve deeper into this area.
For example, in 2024, a team from the Chinese University of Hong Kong also developed a multi-round consultation virtual doctor system based on LLM, called DrHouse, which can improve the accuracy and reliability of diagnosis with the help of smart devices, and provide intelligent and reliable medical assessments with an ultra-long professional life through a constantly updated medical knowledge base and advanced diagnostic algorithms. The relevant paper is titled "DrHouse: An LLM-empowered Diagnostic Reasoning System through Harnessing Outcomes from Sensor Data and Expert Knowledge".
Paper address:
https://arxiv.org/abs/2405.12541
In addition, the team of Wang Yanfeng and Xie Weidi from Shanghai Jiaotong University also released relevant results in 2024. The study mentioned that the team built a multilingual medical corpus containing about 25.5 billion tokens and covering 6 major languages, MMedC, and also proposed a multilingual medical multiple-choice question benchmark, MMedBench. The final model of the research team, MMed-Llama 3, has only 8 billion parameters, but its level on MMedBench and English benchmarks is comparable to GPT-4.
It can be seen that the storm of integration of AI and biomedicine has intensified. With its powerful computing power, novel algorithms and the ability to more easily absorb massive data, AI is making traditional scientific research more efficient and intelligent. What is even more exciting is that these gradually advancing results will eventually make the application come to the ground faster. An era where implementation is king seems to have quietly arrived.
References:
1.https://mp.weixin.qq.com/s/9mhp6luTzQeNhqpEKw9CWQ
2.https://mp.weixin.qq.com/s/WlamJ7N9YKrOJljvEvE9cA
3.https://mp.weixin.qq.com/s/r-S9qkVU645K-ZdaLGYhBA
4.https://mp.weixin.qq.com/s/BfByFCWC9VN6iABnPq1iDw
