AI Subverts Materials Chemistry, Summarizing the Most Noteworthy Scientific Research Results in 2024
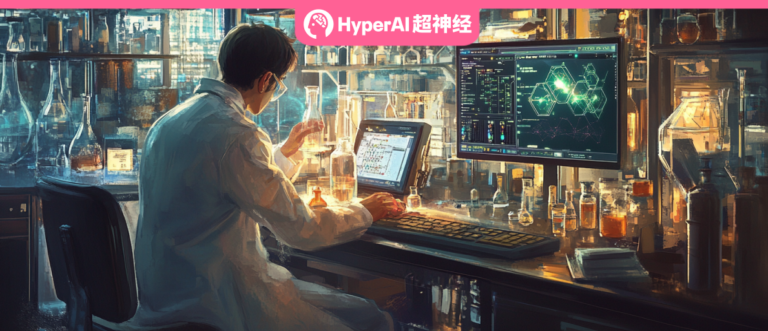
AI for Science is the "fifth paradigm" of scientific discovery.A new scientific research revolution is being initiated, and this change is particularly evident in the field of materials chemistry.
Say goodbye to the traditional "relying on experience + trial and error" model. In intelligence-driven material research and development, AI provides important means in scientific simulation, model prediction, high-throughput experiments, automated characterization, etc., which can effectively reduce the cost of new material research and development and improve research and development efficiency.
Looking back at the year 2024, which is about to end, the field of materials chemistry has made frequent good news with the support of AI, and more and more high-value research results have emerged. As one of the earliest communities focusing on AI for Science, HyperAI is committed to promoting the universalization of AI for Science through multiple methods such as interpreting cutting-edge papers and organizing online/offline academic sharing from time to time.
At this time of bidding farewell to the old and welcoming the new, we have selected and classified the cutting-edge papers interpreted during 2023-2024. This article focuses on the research of AI in the field of materials chemistry. Click on the paper title or Chinese interpretation below to jump to the paper interpretation page. I hope it will be helpful to you.
01 、Paper title:Machine-Learning-Assisted Compositional Design of Refractory High-Entropy Alloys with Optimal Strength and Ductility

Chinese interpretation:Breaking the 1200°C high temperature performance limit! Beijing University of Science and Technology uses machine learning to synthesize 24 refractory high entropy alloys with excellent room temperature ductility
Research content:The team from the University of Science and Technology Beijing integrated a multi-objective optimization framework of machine learning, genetic search, cluster analysis, and experimental feedback to find refractory high-entropy alloys with optimal high-temperature strength and room-temperature ductility.
Published journal:Engineering, 2024.09
02 、Paper title:Open Materials 2024 (0Mat24) Inorganic Materials Dataset and Models

Chinese interpretation:Meta releases open source OMat24 dataset, including 110 million DFT calculation results
Research content:Meta released the open source dataset OMat24 and the pre-trained model EquiformerV2. The OMat24 dataset contains more than 110 million DFT calculation results focusing on structural and compositional diversity.
Published journal:arXiv, 2024.10
03 、Paper title:Universal Ensemble-Embedding Graph Neural Network for Direct Prediction of Optical Spectra from Crystal Structures

Chinese interpretation:Based on 944 material data, Tohoku University and MIT jointly released the GNNOpt model, which successfully identified hundreds of solar cell and quantum candidate materials.
Research content:Researchers from Tohoku University in Japan and MIT launched a GNNOpt model based on graph neural networks, which successfully identified 246 materials with solar energy conversion efficiency exceeding 32% and 296 quantum materials with high quantum weights.
Published journal:Advanced Materials, 2024.06
04 、Paper title:Semi-supervised learning for explainable few-shot battery lifetime prediction

Chinese interpretation:The accuracy of lithium battery life prediction has been improved by 20%! The team from Shanghai Jiaotong University released the semi-supervised learning method PBCT to extract hidden information from unlabeled data
Research content:The team from Shanghai Jiao Tong University used semi-supervised learning technology to predict battery life, and the prediction accuracy was improved by 20%.
Published journal:Joule, 2024.03
05 、Paper title:ChemLLM: A Chemical Large Language Model

Chinese interpretation:Covering 7 million question-and-answer data, Shanghai AI Lab released ChemLLM, with professional capabilities comparable to GPT-4
Research content:The Shanghai Artificial Intelligence Laboratory released the ChemLLM, a large chemical language model that can perform various tasks in the chemical discipline through dialogue interactions. Its performance on core tasks is comparable to that of GPT-4. Researchers have incorporated structured chemical knowledge into the dialogue system, setting a new standard for the development of LLMs in various scientific fields.
Published journal:arXiv, 2024.02
06 、Paper title:Interpreting Chemisorption Strength with AutoML-based Feature Deletion Experiments

Chinese interpretation:Accelerating catalyst design, He Yulian's research group at Shanghai Jiaotong University automatically extracts knowledge based on AutoML
Research content:Based on automatic machine learning (AutoML), a team from the Joint Institute of Shanghai Jiao Tong University explored which factor dominates the chemical adsorption energy of reactants on the catalyst surface, which is of great significance for catalyst design optimization.
Published journal:Proceedings of the National Academy of Sciences, 2024.03
07 、Paper title:Multilevel design and construction in nanomembrane rolling for three-dimensional angle-sensitive photodetection

Chinese interpretation:Microelectronics is accelerating towards the post-Moore era! Mei Yongfeng's research group at Fudan University integrates DNN and nanofilm technology to accurately analyze the angle of incident light
Research content:The Fudan University team combined deep neural networks and nano-thin film assembly to develop a series of three-dimensional structured photodetectors that can achieve high-precision prediction of the angle of incident light and have great potential in areas such as wearable devices, smart furniture, and intelligent driving systems.
Published journal:Nature Communications, 2024.04
08 、Paper title:Neural-network density functional theory based on variational energy minimization

Chinese interpretation:A new era of material exploration! Tsinghua University's Xu Yong and Duan Wenhui team released a neural network density functional framework to open the black box of material electronic structure prediction!
Research content:The Tsinghua University team proposed a neural network density functional theory framework to overcome the shortcomings of traditional DFT calculations of material structures, which are time-consuming and complex.
Published journal:Physical Review Letters, 2024.08
09 、Paper title:Retrosynthesis prediction with an interpretable deep learning framework based on molecular assembly tasks

Chinese interpretation:Shandong University develops an interpretable deep learning algorithm RetroExplainer to identify the retrosynthetic route of organic compounds in 4 steps
Research content:Shandong University and the University of Electronic Science and Technology of China jointly developed an explainable deep learning algorithm, RetroExplainer, which can identify the retrosynthetic routes of organic compounds in 4 steps and give readily available reactants, providing a powerful tool for retrosynthetic research in organic chemistry.
Published journal:Nature Communications, 2023.10
10.Paper title:Water-dispersible X-ray scintillators enabling coating and blending with polymer materials for multiple applications

Chinese interpretation:New breakthrough in flexible composite materials! Hebei University research team uses innovative X-ray scintillators to develop three new materials
Research content:Hebei University, in collaboration with a team from Ghent University, has developed a scintillator that has good water dispersibility and is highly sensitive to X-rays, and has developed three materials using the water-dispersible scintillator.
Published journal:Nature Communications, 2024.03
11.Paper title:A comprehensive transformer-based approach for high-accuracy gas adsorption predictions in metal-organic frameworks

Chinese interpretation:Tsinghua University leads the release of the Uni-MOF model, which effectively identifies 630,000 three-dimensional spatial configurations and predicts the adsorption capacity of MOFs
Research content:Teams from Tsinghua University, University of California, Riverside, Beijing Institute of Scientific Intelligence, and others proposed a machine learning model, Uni-MOF, for predicting the adsorption behavior of three-dimensional metal-organic framework materials. The model is used to predict the adsorption performance of nanoporous materials for various gases under various working conditions.
Published journal:Nature Communications, 2024.03
12.Paper title:Universal materials model of deep-learning density functional theory Hamiltonian

Chinese interpretation:Neural network replaces density functional theory! Tsinghua research group releases universal material model DeepH, achieving ultra-accurate prediction
Research content:The Tsinghua University team proposed the DeepH universal material model, which can be used to predict material structure and properties, demonstrating the feasibility of building a "big material model".
Published journal:Science Bulletin, 2024.06

Chinese interpretation:Argonne National Laboratory releases generative AI framework to accelerate MOFs innovation
Research content:Argonne National Laboratory in the United States released a generative AI framework GHP-MOFsassemble, which can randomly generate and assemble new MOFs structures, screen out highly stable MOFs structures, and test their ability to adsorb carbon dioxide.
Published journal:Communications Chemistry, 2024.02
14.Paper title:Machine-Learning Assisted Screening Proton Conducting Co/Febased Oxide for the Air Electrode of Protonic Solid Oxide Cell

Chinese interpretation:AI screening battery materials, Academician Ye Siyu of Guangzhou University developed a machine learning algorithm model that can be used for P-SOC material prediction
Research content:Researchers from Guangzhou University established a machine learning model based on the extreme gradient boosting algorithm, which can be used for the screening of P-SOC air electrodes.
Published journal:Advanced Functional Materials, 2023.12
15.Paper title:Material symmetry recognition and property prediction accomplished by crystal capsule representation

Chinese interpretation:Li Huashan and Wang Biao's research group at Sun Yat-sen University developed the SEN machine learning model to predict material properties with high accuracy
Research content:The Sun Yat-sen University team developed a machine learning model called SEN that can accurately perceive the interactions between inherent crystal symmetries and material structure clusters.
Published journal:Nature Communications, 2023.08
16.Paper title:Machine learning-assisted prediction of water adsorption isotherms and cooling performance

Chinese interpretation:Li Song's research group at Huazhong University of Science and Technology used machine learning to predict water adsorption isotherms of porous materials
Research content:The team from Huazhong University of Science and Technology established a two-step machine learning model to train AI to predict water adsorption isotherm parameters and subsequent application performance based on the structural parameters of the material.
Published journal:Journal of Materials Chemistry A, 2023.09
17.Paper title:FlowLLM: Flow Matching for Material Generation with Large Language Models as Base Distributions

Chinese interpretation:The efficiency of stable material generation has been improved by 300%! Meta FAIR released the material generation model FlowLLM, with a data set covering more than 45,000 materials
Research content:Meta FAIR Laboratory and the University of Amsterdam jointly released the material generation model FlowLLM, which increased the efficiency of generating stable materials by more than 300% and the efficiency of generating SUN materials by about 50%.
Published journal:NeurIPS 2024, 2024.10
18.Paper title:Scaling deep learning for materials discovery

Chinese interpretation:800 years ahead of humans? DeepMind releases GNoME, using deep learning to predict 2.2 million new crystals
Research content:Google DeepMind released the deep learning tool GNoME, which discovered 2.2 million new crystals in a short period of time, of which 380,000 new crystals have stable structures and can become potential materials for research and development.
Published journal:Nature, 2023.11
19.Paper title:CMOS-Compatible Reconstructive Spectrometers with Self-Referencing Integrated Fabry-Perot Resonators

Chinese interpretation:The cover article of the Proceedings of the National Academy of Sciences of the United States! A Chinese team released an AI-adaptive micro-spectrometer that can be produced at the wafer level
Research content:The Fudan University team proposed a new design of a miniaturized reconstruction spectrometer that can be manufactured at the wafer level using mature integrated circuit processes and has a millimeter-scale size, which is sufficient to meet most miniaturized spectral testing needs.
Published journal:Proceedings of the National Academy of Sciences, 2024.08
20.Paper title:Fully forward mode training for optical neural networks

Chinese interpretation:Major breakthrough in domestic optical chips! Tsinghua team uses neural network to create the first full-forward intelligent optical computing training architecture
Research content:A team from Tsinghua University has developed a fully forward mode (FFM) learning method that is expected to promote the development of fields such as deep learning neural networks, ultra-sensitive perception, and topological photonics.
Published journal:Nature, 2024.08
twenty one ,Paper title:Superstrength permanent magnets with iron-based superconductors by data- and researcher-driven process design

Chinese interpretation:The strongest iron-based superconducting magnet is born! Scientists design a new research system based on machine learning, and the magnetic field strength exceeds the previous record by 2.7 times
Research content:British and Japanese scientists used AI technology to successfully create the world's strongest known iron-based superconducting magnet.
Published journal:NPG Asia Materials, 2024.06
twenty two ,Paper title:A simplified electrochemical model for lithium-ion batteries based on ensemble learning

Chinese interpretation:Reshaping the performance boundaries of lithium batteries, Kang Jianqiang's team from Wuhan University of Technology proposed a simplified electrochemical model based on ensemble learning
Research content:The team from Wuhan University of Technology proposed a simplified electrochemical model that can accurately predict the changes in lithium ion concentration on the surface of electrode particles and thus predict the battery voltage.
Published journal:iScience, 2024.05
twenty three ,Paper title:Fill in the Blank: Transferrable Deep Learning Approaches to Recover Missing Physical Field Information

Chinese interpretation:Fill in the blanks in the material space: MIT uses deep learning to solve nondestructive testing problems
Research content:MIT scientists have used deep learning to develop a technique that can recover missing parts of a material using limited information and further observe the surface to determine the material's internal structure.
Published journal:Advanced Materials, 2023.03
twenty four ,Paper title:Enhancing corrosion-resistant alloy design through natural language processing and deep learning

Chinese interpretation:AI "anti-corruption", Germany's Max Planck Institute combines NLP and DNN to develop corrosion-resistant alloys
Research content:The Max Planck Institute for Iron Research in Germany combined deep neural networks (DNN) and natural language processing (NLP) to develop a process-aware DNN to explore the effects of different elements on the corrosion resistance of alloys.
Published journal:Science Advances, 2023.08
25.Paper title:A comprehensive machine learning strategy for designing high-performance photoanode catalysts

Chinese interpretation:Tsinghua University uses interpretable machine learning to optimize photoanode catalysts to help photolysis of water to produce hydrogen
Research content:A team from Tsinghua University used machine learning to optimize the co-catalyst of BiVO(4) photoanode.
Published journal:Journal of Materials Chemistry A, 2023.10
26.Original paper:Universal machine learning for the response of atomic systems to external fields

Chinese interpretation:Jiang Bin's research group at USTC developed FIREANN to analyze the response of atoms to external fields
Research content:The interaction between chemical systems and external fields is crucial. A team from the University of Science and Technology of China has developed a field-induced recursive embedded atomic neural network (FIREANN), which can accurately describe the changing trend of system energy when the external field intensity and direction change, and can also predict the system response of any order.
Published journal:Nature Communications, 2023.10
The above are the cutting-edge papers on AI+materials chemistry summarized in this issue. For more papers on AI+biomedicine, medical health, meteorology and oceanography, see you next time.
Finally, let me share a technical salon! The 6th Meet AI Compiler will be held in Shanghai on December 28. Click on the picture to learn about the lecturer's sharing topics and scan the QR code to sign up directly.