Directly Design Target Property Materials! Microsoft MatterGen Model Is Open Sourced, Redefining the New Paradigm of Material Reverse Design With Generative AI
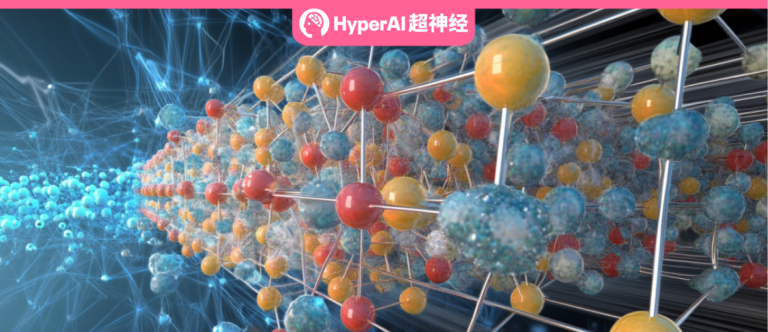
In December 2023, Google DeepMind published its deep learning model GNoME in the field of materials chemistry in Nature, claiming to have discovered 2.2 million new crystal structures of inorganic materials. Less than a week after this breakthrough, Microsoft announced that it would launch MatterGen, a generative AI model for reverse design of materials, and told everyone,In the future, it will be possible to directly design the structure of new materials based on the required properties.

If Google's GNoME model shows us the potential of AI to quickly discover new materials in a vast chemical space, then Microsoft's MatterGen further proves the ability of generative AI to accurately meet specific needs through reverse design. The two show different entry points of AI in the field of materials chemistry, and also mark the new technological leap from large-scale discovery to "on-demand design". On January 16, the MatterGen results were finally officially published in Nature under the title "A generative model for inorganic materials design". What's more exciting is that the model is now open source.HyperAI has launched the tutorial "MatterGen Inorganic Material Design Model Demo" on its official website. It can be deployed and run with one click. Everyone is welcome to test the model performance.
Tutorial address:https://go.hyper.ai/5mWaL

Professor Wang Jinlan of Southeast University once pointed out in the article "Inverse design with deep generative models: next step in materials discovery" that in traditional machine learning-assisted material design research, most of them predict the properties of candidate materials in the entire chemical space and conduct large-scale screening to find potential materials with target performance, but inverse design can directly generate qualified compounds along the optimal path. She believes that generative models are an effective strategy for reverse design of materials, which coincides with Microsoft's research.
MatterGen is based on a diffusion model and can generate structures according to target space groups. For example, when designing multi-attribute magnetic materials, a structure with both high magnetic density and low supply-chain risk chemical composition is proposed. At the same time, the model is also equipped with multiple adjustable adaptation modules, which can be fine-tuned according to constraints such as chemical properties, symmetry, and material properties to generate materials that meet specific magnetic, electronic or mechanical properties, and verified by DFT. It can be seen that "customizing" new materials based on a certain scenario may become a reality in the near future.
In addition to the diffusion models mentioned above, today’s mainstream generative models also include generative adversarial networks (GANs), variational autoencoders (VAEs), autoregressive models, etc. Their core principles are to generate new samples by learning data distribution.
In this article, HyperAI will introduce the value of generative models in reverse design of new materials, and explore the specific progress of this technology in battery materials, high entropy alloys, superconducting materials, etc.
Similarities between new material development and protein design
In a typical materials development problem, we want to find a new material with specific properties, which is actually a matter of finding a suitable crystal structure that matches the target properties.
In the past, the way we developed new materials mainly relied on trial and error. This "forward design" is characterized by the discovery from structure to properties. Taking the most common substitution method as an example, La-Ba-Cu-O superconductor is the earliest copper-based superconductor, but it only superconducts at 35 K, which is lower than the liquid nitrogen temperature zone. Starting from the structure, researchers replaced La with Y elements and found that the superconducting temperature of Y-Ba-Cu-O superconductor is higher than the liquid nitrogen temperature zone. However, the research and development cycle of this method is very long and highly accidental.
With the advancement of computer technology and quantum mechanics theory, material prediction methods based on density functional theory (DFT) have gradually matured. Combined with structure search algorithms and high-throughput computing, potential materials can be efficiently screened on certain databases based on certain constraints, and then sent to the laboratory for synthesis and testing. However, the chemical space of unknown materials is extremely large, and the potential combinations of different elements are even as high as millions, which makes the computational cost of large-scale screening very expensive.
AI-driven reverse design provides a new way of thinking. It breaks away from the inertial thinking of material space screening and directly generates material structures that meet the target performance, thereby achieving efficient design and optimization of materials.
In fact, AI-driven reverse design has made breakthroughs in the biomedical field. In October 2024, the Nobel Prize in Chemistry involved the AI field for the first time, and half of the prize was awarded to David Baker of the University of Washington in recognition of his outstanding contributions to protein design. In many of his studies, we can observe cases of using deep learning in reverse to generate amino acid sequences for designing functional new proteins.

There are many similarities between the development of new materials and protein design. For example, the macroscopic properties of materials are determined by their microscopic structures, and the same is true for proteins. In the field of proteins, the amino acid sequence guides the protein to fold into specific secondary, tertiary, and even quaternary structures, which in turn determine its biological function. Similarly, materials science relies on the selection and arrangement of atoms, chemical bonds, and functional groups to construct molecules or more complex material structures, which in turn determine their performance.
This similarity allows popular AI methods in protein design to provide insights into materials science research, such as optimizing material properties through inverse design, exploring new structures, or developing entirely new materials.
At the same time, other generative models, visual models, language models and other advanced technologies that have emerged in the biomedical field, such as reinforcement learning, attention mechanism, diffusion model, pre-training model, multimodal technology, model alignment mechanism, etc., also have broad application potential in materials science.
It is worth mentioning that since new materials do not need to go through the long clinical trial cycle of biomedicine and exclude the influence of factors such as ethical safety, the possibility of actual implementation may be greater.
Taking Microsoft MatterGen as an example, we explore the new paradigm of generative AI reverse design of materials
Microsoft's MatterGen model is mainly based on a diffusion architecture, which first gradually destroys the atomic type, atomic position, and periodic lattice into a random structure, and then trains a model to complete this process in reverse, allowing the model to learn how to gradually restore the original material structure from random noise. Xie Tian, the corresponding author of the paper, believes that this is very similar to the core idea of video generation.
Taking the Vincent video model Sora developed by OpenAI as an example, researchers use the technology of "video compression network" based on autoencoder to compress the input image or video into a lower dimensional data, and decompose these compressed videos into "space-time patches", which are further converted into one-dimensional data sequences for Transformer processing. Subsequently, Transformer will complete the noise removal of each space-time patch, and then restore the processed tensor data into video through the decoder.

On the other hand, based on the diffusion architecture, the researchers let the model learn the structure of known stable material data. Once the model is trained, it can unconditionally sample from the random distribution and, through the reverse process, let the model generate new material structures that meet the conditions based on its understanding of the material laws. Furthermore, the researchers add conditions to each layer of the network to fine-tune the basic model. These conditions can be specific chemical properties, symmetry, or any target properties (magnetism, density, etc.). After fine-tuning,The model can directly generate material structures according to specified conditions and verify their stability through calculation methods.
As shown below, in the case of new material generation for the strontium-vanadium-oxygen chemical system, the material structures generated by MatterGen appear very reasonable (fi), and calculations have verified that these materials are stable.

In addition to computational verification, the team also collaborated with the Shenzhen Institute of Advanced Technology of the Chinese Academy of Sciences to successfully synthesize a new material TaGr using MatterGen.2O6The experimentally measured bulk modulus is 169 GPa, which is less than the design value of 200 GPa by 20%. At the same time, the team also hopes to obtain feedback from scientists, continuously iterate and optimize the model, in order to improve its practical application value.
It is worth mentioning that since most material design problems involve finding materials with extreme properties, such as room-temperature superconductors and superionic conductors for batteries, traditional search-based methods are difficult to implement, but generative models are guided by target properties and can provide opportunities for discovering these breakthrough materials.Microsoft is using this model to explore a variety of materials, covering battery design, solar cell design and carbon capture.
More applications: Taking the development of high entropy alloys and superconducting materials as an example
We all know that new materials are not only the cornerstone for the development of high-tech fields such as aerospace, new energy, electronic information and biomedicine, but also the backbone of new technologies, new equipment and new projects. However, at present, my country's materials industry is still dominated by traditional materials, and the supply of new materials, especially high-end new materials, is limited. At the same time, due to the shortage of key technologies, we have a certain dependence on imported materials, and the problem of being constrained by people is still prominent.
Nowadays, with the development of generative AI, materials science is ushering in a new research paradigm change. If we can enter this emerging field as early as possible, it may provide a possibility to overcome shortcomings and achieve "overtaking on the curve". Next, the author will take the specific cases of generative AI in the development of high entropy alloys, superconducting materials and other applications as an example to explore how this technology can help new materials achieve leapfrog development.
High Entropy Alloy
In engineering applications such as gas turbines, nuclear reactors, and aviation propulsion systems, there is a strong demand for metal alloys with excellent high-temperature mechanical properties. Refractory high-entropy alloys (RHEAs) can maintain high strength at temperatures of 1000°C and above by adding different high-melting-point refractory elements, showing high-temperature strength comparable to that of high-temperature alloys, which has attracted widespread attention from researchers.
However, compared with other high-temperature alloys, the performance of RHEAs in certain aspects (such as room temperature ductility) is still challenging. In the past, the design of RHEAs mostly relied on the experience and intuition of researchers, which was highly uncertain. At the same time, the possible composition space of RHEAs is large, containing billions of candidate components, which severely limits our rapid discovery of potential alloys.
In this regard, Wesley Reinhart, assistant professor in the Department of Materials Science and Engineering and the Institute of Computational and Data Science at Pennsylvania State University, published a paper titled "Generative deep learning as a tool for inverse design of high entropy refractory alloys" in the Journal of Materials Informatics, and came to a preliminary conclusion that generative models are a promising new method for material design, especially in the design of high entropy alloys. This result was rated as the best paper of the year by JMI.
Paper address:
https://www.oaepublish.com/articles/jmi.2021.05
In this paper, the researchers mentioned that in the past 10 years, computational methods such as density functional theory (DFT) have basically matured and accumulated a large amount of data, which provided a basis for the application of deep learning and promoted the development of "forward models". Unfortunately, the huge design space is still a key challenge. The "reverse design" of generative modeling provides a solution for this.
Therefore, the researchers used conditional generative adversarial networks (CGAN) to provide the generator with additional conditional vectors to control its output. In other words, the conditional vector can provide information related to the target attribute (such as alloy composition or performance indicators), establish a mapping between the latent space and the desired indicators, and the generator generates samples that meet the conditions by learning the probability distribution of alloy performance data based on alloy composition. It is worth mentioning that the model has successfully designed aluminum alloys and has been verified by computational methods.

It is worth mentioning that the researchers also mentioned that in addition to using CGAN, conditional variational autoencoder (CVAE) can also be used for new material design, but because of the inherent noise injection in the training process and the predefined measurement requirements for reconstruction error, VAE is not as effective as GAN.
Superconducting materials
Superconducting materials refer to conductors with zero resistance at a certain temperature. They have a wide range of applications, covering power transmission, motors, transportation, aerospace, microelectronics, electronic computers, communications, nuclear physics, new energy, bioengineering, medical care, and military equipment. Since the discovery of the superconductivity phenomenon, this field has produced many related Nobel Prizes.
Discovering new superconductors with high critical temperature (Tc) has always been an important task in the fields of materials science and condensed matter physics. The National Institute of Standards and Technology and other researchers from Microsoft have proposed a new diffusion model for generating superconductors with unique structures and chemical compositions. The study was published in The Journal of Physical Chemistry Letters under the title "Inverse Design of Next-generation Superconductors Using Data-driven Deep Generative Models".
Paper address:
https://pubs.acs.org/doi/10.1021/acs.jpclett.3c01260
In this work, the researchers mentioned that the main challenge in applying generative models to periodic materials is to create representations that are translationally and rotationally invariant, a problem that can be solved using a crystal diffuse variational autoencoder (CDVAE).

Therefore, as shown in the figure above, the researchers trained the CDVAE model with DFT data of 1,058 superconducting materials to generate 3,000 new superconductor candidate materials. Subsequently, the pre-trained deep learning model ALIGNN was used to predict the superconducting properties of these candidate structures, and 61 candidate materials were obtained after screening. Finally, the researchers performed DFT calculations on these materials to verify the prediction results and evaluate the dynamic and thermodynamic stability of the new materials. The structures of 15 potential candidate superconducting materials are shown in the figure below. The study found that such an approach makes the reverse design of the next generation of materials possible.

Of course, in addition to the cases mentioned above, generative models have also been applied in other material designs. I have specially compiled some cases for your reference.
*Lithium battery design
Paper title: Li-ion battery design through microstructural optimization using generative AI
Paper address:
https://www.cell.com/matter/fulltext/S2590-2385(24)00446-6
*Nanocomposite material design
Paper title: Generative AI for Tailored Functionalities in Nanocomposite Materials
Paper address:
https://easychair.org/publications/preprint/sDm2
*2D material design
Thesis title: Computational Discovery of New 2D Materials Using Deep Learning Generative Models
Paper address:
https://pubs.acs.org/doi/abs/10.1021/acsami.1c01044
*Design of engineering cement-based composite materials
Paper title: Generative AI for performance-based design of engineered cementitious composite
Paper address:
https://www.sciencedirect.com/science/article/abs/pii/S1359836823004961
*Mechanical and biomimetic material design
Paper title: Enhancing mechanical and bioinspired materials through generative AI approaches
Paper address:
https://www.sciencedirect.com/science/article/pii/S2949822824001722
Final Thoughts
At present, many applications of generative AI in material design are still in the experimental stage. In order to truly implement the technology, in addition to evaluating material properties through calculations, it is also necessary to rely on real-life experimental verification. In this regard, if we want to narrow the gap between computational screening and experimental synthesis of new materials, and quickly discover materials with minimal manpower, it is particularly important to build automated laboratories and achieve closed-loop discovery.
Take the automated laboratory A-Lab at the University of California, Berkeley, for example. It can not only automatically execute experimental steps, but also make decisions based on data. In 17 days of continuous operation, it successfully synthesized 41 of 58 target materials, with a success rate of 71%. This shows that using generative AI to design materials and efficiently synthesizing and verifying them through automated laboratories is becoming an effective way to promote the rapid development of materials science.
References:
1.https://nullthought.net/?p=5222&utm_source=chatgpt.com
2.https://academic.oup.com/nsr/article/9/8/nwac111/6605930?login=false
3.https://mp.weixin.qq.com/s/UX71cMgsEo49tLPiFu3D8A
4.https://mp.weixin.qq.com/s/e1DqTa1Tgyi4OWpgwrj48Q
5.https://www.youtube.com/watch?v=Smz1go6_Spo&t=896s
6.https://www.youtube.com/watch?v=yWXPV3bsC2c&t=7s
7.https://www.youtube.com/watch?v=Uv22eVcmmXA
