Professor Zheng Wei of Nankai University: AlphaFold Is Not Perfect, and the Academic Community Still Has the Opportunity to "overtake on the Curve"
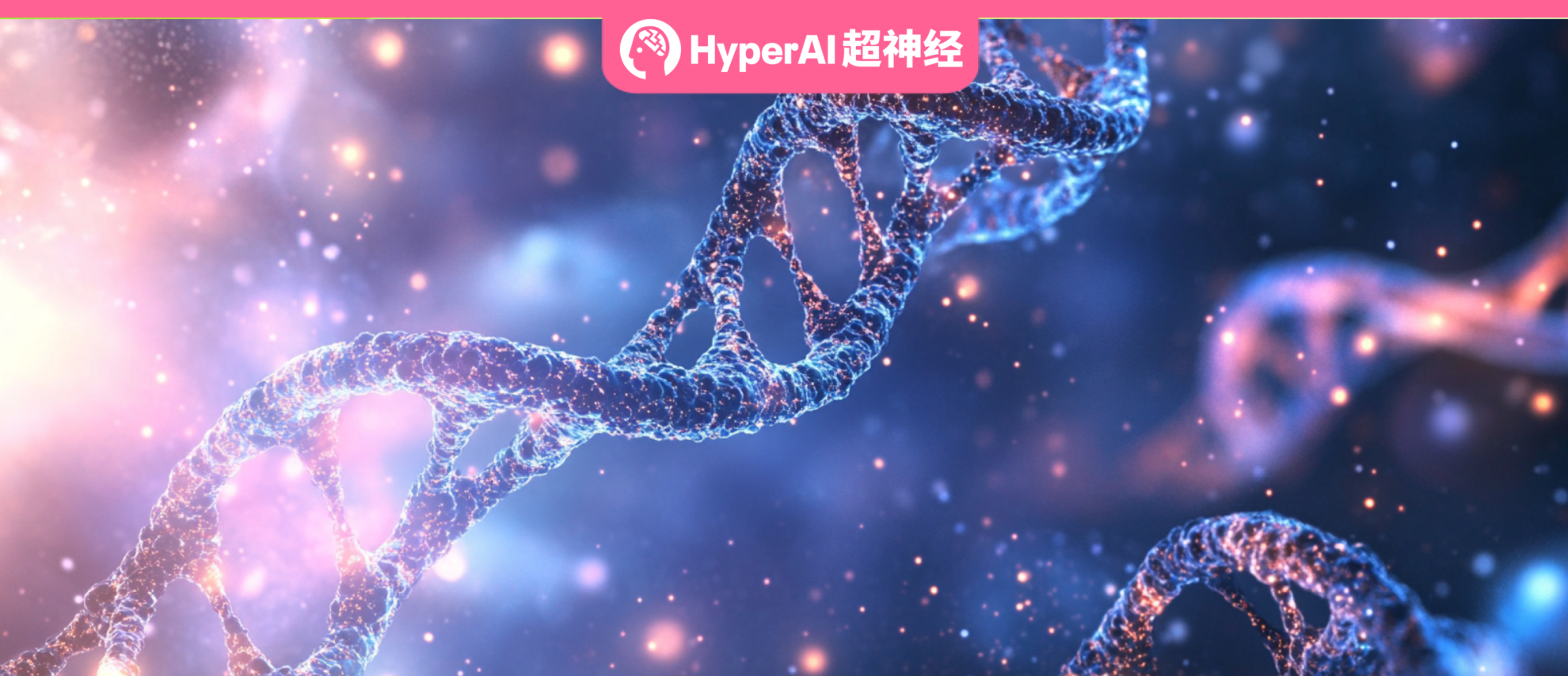
In recent years, with the assistance of AI technologies such as deep learning, the field of protein structure prediction has developed rapidly. In October 2024, DeepMind's Demis Hassabis and John M. Jumper won the 2024 Nobel Prize in Chemistry because of AlphaFold. However, this does not mean that AlphaFold is irreplaceable, and other excellent algorithms are still worth exploring.
In the sixth episode of the "Meet AI4S" live series,HyperAI is honored to have invited Professor Zheng Wei, a professor at the School of Statistics and Data Science of Nankai University,With the theme of "AlphaFold3's throne is not stable, and the academic community is overtaking it: three-dimensional structure prediction of biological macromolecules and their interactions based on deep learning", he shared with everyone the limitations of AlphaFold and future optimization directions, as well as what algorithms and research topics are worth exploring in the academic community.
* Follow the official account and reply "Meet AI4S 6th" to get the presentation PPT
HyperAI has organized and summarized the in-depth sharing without violating the original intention. The following is the transcript of the speech.
Limitations of AlphaFold
Proteins are the cornerstones of life activities, and predicting protein three-dimensional structures is crucial to understanding biological functions. Although AlphaFold 2 launched by DeepMind has taken protein structure prediction to a new level, this does not mean that AlphaFold 2's end-to-end framework has solved all protein structure prediction problems.
First of all, taking AlphaFold 2 itself as an example, it still has many limitations:
* Accuracy needs to be improved
Official reports show that AlphaFold 2 can predict structures with an accuracy of more than 90%, but the actual task cannot reach such a high level.
* Multi-domain protein structure prediction is limited
AlphaFold 2 performs well in predicting single-domain proteins, but for complex multi-domain proteins, where the domains are relatively flexible, the prediction accuracy is not good.
* Protein complex structure prediction is limited
Proteins typically need to form complexes with other proteins to function, but the initial version of AlphaFold 2 did not address this issue.
* RNA structure prediction, RNA-RNA, and protein-RNA structure prediction are limited
As above, these issues were not addressed in the initial version.
* Prediction of protein dynamics/conformational changes is limited
Experimental analysis methods can usually only capture the structural state at a certain moment, but proteins do not exist statically in their organisms, and their structures at different time points may be different. These problems have not yet been solved by AlphaFold 2.

Furthermore, although DeepMind has iterated AlphaFold 3, and we all know that it performs well in predicting protein monomer structures, its accuracy in predicting complexes, nucleic acids, and small molecules still needs to be improved.The next generation of AlphaFold may add prediction modules with other functions.For example, considering that the existing models are mainly used to deal with static structures, they will explore the dynamic process of molecules and predict the conformational changes of proteins. In addition, they may also involve the field of protein design and reverse the entire prediction process.
Therefore, even with AlphaFold, there is still a lot of work to be done throughout academia.
In addition to AlphaFold, are there any other methods worth exploring?
In the past, we mainly used X-ray, nuclear magnetic resonance (NMR), and cryo-electron microscopy to analyze the three-dimensional structure of proteins. Due to the difficulty and high cost of experimental analysis of protein structure, some teams may need to spend months to years to analyze a protein three-dimensional structure. Therefore, people began to explore a more economical and faster method, that is, to predict protein structure through algorithms.
We know that proteins are mainly composed of 20 kinds of amino acids, usually represented by English letters, and amino acid molecules also contain many atoms.Therefore, the protein structure prediction problem can be summarized as follows: input an amino acid string consisting of these letters, and use a computational algorithm to predict the three-dimensional spatial coordinates (x, y, z) of each atom in each amino acid in the protein sequence.
Throughout the development of protein structure prediction, a variety of representative algorithms have emerged at different stages, such as comparative modeling or homology modeling, molecular dynamics simulation (MD), Threading algorithm, de novo prediction, structure prediction algorithm based on deep learning prediction of contact map, etc. The main introduction is as follows:
* Comparative modeling or homology modeling
This method is based on the principles of biological evolution.It is believed that if the sequence similarity is high, the structure and function of the protein will also be relatively similar.Therefore, we can first obtain the amino acid sequence of the unknown protein, and then find the resolved protein structure template with high sequence similarity in the PDB database through sequence alignment, and predict the structure of the unknown protein through migration or alignment.
*PDB database contains the structures of proteins that have been solved in this field

* Molecular dynamics simulation
The basic idea is to randomly generate an initial three-dimensional structure based on the amino acid sequence of the protein, assign random coordinates to each atom, adjust the atomic position, and then calculate the state energy of the protein at different times based on the pre-constructed physical energy field.The structure with the lowest energy is the reasonable protein conformation.

* Threading algorithm
Similar to homology modeling, the difference is that although proteins with high sequence similarity are often similar in structure, proteins with similar structures may have low sequence similarity, and such proteins cannot find suitable template information in the PDB database. Therefore, researchers proposed the concept of profile, which uses multiple sequence alignment (MSA) based on the collected homologous sequences to align different amino acids in the same way as aligning two protein profiles.
That is, even if the two amino acid sequences are different,But their profiles are similar, so we can assume that their structures are similar.Use this to find templates.

* De novo prediction
Some proteins may not have similar structures in the database.The researchers then tried to make predictions by breaking down the entire protein sequence into shorter fragments, looking for templates of these small fragments in the database, and then assembling these small fragment templates into a complete three-dimensional structure.
Specifically, Professor David Baker of the University of Washington developed the Rosetta software, whose main principle is to decompose the protein sequence into many small fragments, randomly assemble these fragments, and then optimize them using the energy function developed in molecular dynamics simulation, and perform structure prediction through principles similar to dynamic simulation and energy minimization.

* Contact map
The main idea is to convert the three-dimensional structure of a protein into a two-dimensional graph.Using the three-dimensional structure information of proteins, that is, using the coordinate positions of all spatial points to calculate the distance between different amino acids, it is assumed that when the distance between two amino acids is less than a certain threshold, contact is formed, otherwise, no contact is formed. This definition is used to compress the three-dimensional structure into a two-dimensional graph. Furthermore, the information of this two-dimensional contact graph can reconstruct the three-dimensional structure of the protein.
Specifically, the researchers developed many deep learning-based methods. The core idea is to first construct a multiple sequence alignment (MSA) to observe the co-evolution information of the profiles in amino acids i and j, because such co-evolving amino acids are often very close in space and will form contacts. Subsequently, the co-evolution information is input as a feature into the deep learning network for training, and then the contact map of the protein is predicted and the three-dimensional structure of the protein is restored.
For example, Professor Zheng Wei's team previously developed an algorithm called CI-TASSER, which is currently a commonly used method for predicting protein structure based on contact maps.

Finally, AlphaFold integrates the basic principles of many of the above algorithms and successfully builds an end-to-end framework that can directly input protein sequences and then output structures.
Taking team achievements as an example, exploring the opportunities for academics to overtake
Protein structure prediction has a huge impact on the biomedical field, for example,The algorithms currently developed by Professor Zheng Wei's team involve predicting unknown viral protein structures (new coronavirus), assisting in the analysis of protein structures using cryo-electron microscopy, helping biologists understand protein evolutionary functions, and antibody screening.

In addition, as shown in the figure below, all protein monomer and complex structure prediction algorithms developed by the team have been converted into automatic server algorithms and published on the research group's website. Its algorithms have served more than 90,000 users in more than 100 countries around the world, and everyone is welcome to use them.
*Total project address:
https://seq2fun.dcmb.med.umich.edu/DMFold

Protein monomer structure prediction method DI-TASSER
The problem of protein monomer structure prediction has always attracted much attention. Before AlphaFold 2, Professor Zheng Wei's team had already been doing research on structure prediction based on contact maps. After the emergence of AlphaFold 2, the team began to think about whether they could integrate spatial constraints such as contact maps predicted by AlphaFold 2 into the previously developed algorithms. So based on spatial constraints, metagenomes, statistical energy functions, etc.,The team developed a protein monomer structure prediction algorithm DI-TASSER, which showed good results after optimization.
As shown in the example on the right side of the figure below, red represents the protein structure predicted by DI-TASSER, and blue represents the structure analyzed by the experiment.The structure predicted by DI-TASSER is very similar to the experimentally resolved structure.In contrast, the structure predicted by AlphaFold 2 is significantly different from the experimental structure even after alignment, and its prediction accuracy is slightly lower.

In addition, multiple protein datasets were evaluated. As shown in the right figure below, when predicting single domain and multi-domain structures,The prediction accuracy of DI-TASSER is higher than that of AlphaFold 2 and even higher than that of AlphaFold 3.

To ensure the authority of the evaluation, the team not only conducted internal evaluations, but also participated in the authoritative competition in the field - CASP.
The CASP competition is known as the Olympics in this field, and is mainly aimed at standardizing the evaluation methods for protein structure prediction. Because there are many types of protein three-dimensional structure prediction algorithms, each laboratory has also developed its own algorithm. Since the evaluation data sets and methods may be different, each research group usually claims that their method is the most accurate in the world. In order to solve this chaotic situation, the CASP competition came into being.
As of last year, CASP has been successfully held for 16 sessions and has lasted for 32 years, attracting many authoritative teams to participate, such as Professor David Baker's team and the DeepMind team.
DI-TASSER and its predecessor algorithms participated in CASP competitions many times. During CASP 13-CASP 15, this method has been in the leading position in the field of protein monomer structure prediction. In CASP 15,The DI-TASSER algorithm also participated in the multi-domain evaluation, and its overall accuracy was better than that of all participating research groups.

DMFold, a protein complex structure prediction method
The main challenge in complex structure prediction is to predict the relative torsion between two proteins, which can be analyzed using co-evolutionary information.
For example, by constructing a multiple sequence alignment (MSA) of the monomers of two proteins, merging the two MSAs into one MSA based on some connection methods, and using the co-evolutionary relationship of amino acids between the two MSAs to infer the distance between amino acids in different proteins, the co-evolutionary information can also be integrated into the deep learning framework to predict the relative torsion between two proteins.
In this regard,Professor Zheng Wei's research group developed the DeepMSA and MetaSource algorithms to construct deeper multiple sequence alignments.In addition, the team also used deep learning, metagenomics, etc. to develop a protein complex structure prediction algorithm DMFold.

As shown in the rightmost example in the figure above, the upper part is the real structure obtained through experimental analysis, the lower left part is the structure predicted by DMFold, and the right part is the result predicted by AlphaFold 2. It can be seen that the structure predicted by AlphaFold 2 is relatively chaotic and has abnormal tentacle-like extensions. In contrast, the predicted structure of DMFold is highly similar to the experimental structure.This shows that the DMFold algorithm is superior to AlphaFold 2 in complex structure prediction.
In addition, DMFold also showed high accuracy in large-scale protein-protein complexes, nanoantibody-antigen complexes, and conformational changes caused by point mutations. DMFold's overall ranking is much higher than AlphaFold 2, and in CASP 16, DMFold also outperforms AlphaFold 3.

RNA-RNA complex structure prediction method ExFold
In recent years, the team has begun to focus on the problem of RNA structure prediction. For example, they have developed the RNA/RNA complex structure prediction algorithm ExFold using deep learning models such as Transformer and RNA big language models.
As shown in the example on the right side of the figure below, the gray part is the experimental structure and the colored part is the predicted structure.Using the ExFold method, the two structures were aligned well. In contrast, the AlphaFold 3 prediction showed that there was not even any contact between the two RNA molecules, which can almost be considered completely wrong.

The team also compared the accuracy of ExFold 3 with AlphaFold 3 using a larger data set, as shown on the left side of the figure below. The Y-axis represents the prediction accuracy of ExFold.The X-axis represents the prediction accuracy of AlphaFold 3. It can be seen that ExFold has an obvious advantage.

In addition, in the CASP 16 RNA complex structure prediction competition,Although ExFold is not ranked first overall, it ranks highest among all automatic algorithms (server algorithms).
* The CASP competition is divided into an automatic group and a manual group. The automatic group is required to submit prediction results within 3 days without any manual intervention; the manual group has 3 weeks and is allowed to add expert experience and manual adjustments.
Protein-RNA complex structure prediction method DeepProtNA
Regarding the problem of protein-RNA complex structure prediction, the team used deep learning models such as Transformer and the recently popular large language model to develop a new structure prediction algorithm - DeepProtNA.
As shown in the example on the right below, in the antibody-RNA complex, the color represents the prediction result of DeepProtNA, while the gray represents the experimental structure. After alignment, it can be found thatThe predicted structure of DeepProtNA is highly consistent with the experimental structure (gray and color overlap).Especially at the interface between antibody protein and antigen RNA, the prediction accuracy is very high. In contrast, the predicted structure of AlphaFold 3 is almost incompatible with the experimental structure, and the prediction effect is poor.

also,DeepProtNA is about 7.5 percentage points more accurate than AlphaFold 3.Ranked first in the server group competition of CASP 16.

EnsembleFold: A method for predicting allosteric structures of biomacromolecules
The team also focuses on the problem of predicting allosteric structures of biological macromolecules. The input of the macromolecular multi-conformation problem is a protein sequence, and the output is multiple key frames of the protein in different states. This means that compared with static prediction algorithms, multiple different structures need to be predicted from a single amino acid sequence. These structures represent the key frames of the entire dynamic process. This is a topic that has received a lot of attention in the current field, but is difficult to predict.
By integrating previously developed methods and optimizing them for macromolecular allostery,The research team developed some clustering algorithms and finally formed an algorithm called EnsembleFold.
As shown in the example on the right side of the figure below, the conformational changes of proteins after binding to small molecules are shown. The blue color represents the experimental structure when the small molecules are not bound, and the red color represents the tilt and conformational changes after binding to the green small molecules. The team predicted two structures based on the input protein sequence, which are the black parts. It can be seen that the predicted structure of EnsembleFold is very consistent with the real structure when the small molecules are not bound. After binding to the small molecules, EnsembleFold can also fit the experimental structure well. Therefore,EnsembleFold demonstrates extremely high accuracy in predicting conformational changes in biomacromolecules.

at the same time,After comparing with AlphaFold 3, it was found that EnsembleFold's accuracy was about 12.4% higher.It ranks first among all macromolecular conformation competitions in CASP 16.

An interesting example is that the team predicted the conformational changes of phage DNA integrase in CASP. As shown in the figure below, the amino acid sequence of the phage is represented by P-P', and the genetic material sequence of the bacteria is represented by B-B'. Through the dynamic process, the phage DNA integrase integrates the genetic material P' of the phage into the genetic material B of the bacteria to form B-P', and the conformation changes.
The team used an algorithm to predict this multi-conformation change. The experimental structure is shown on the left, with the top part showing the unintegrated state (conformation 1) and the bottom part showing the integrated state (conformation 2). It can be seen that the team's predictions can accurately reflect these two different conformations.

It is worth mentioning that in the CASP 16 competition,The contestants only received sequence information and did not know the specific biological process or details of conformational changes. However, Professor Zheng Wei's team successfully restored the entire biological process through prediction.During the post-match summary, the judges also expressed surprise.
Research Group Recruitment
Professor Zheng Wei from the School of Statistics and Data Science at Nankai University has long been committed to the prediction research of the structure, function and interaction of biological macromolecules such as proteins. He has led the development of a number of protein monomer, protein complex, nucleic acid and complex, protein-nucleic acid complex structure prediction algorithms and structure evaluation algorithms with better accuracy than AlphaFold2/3. He has won the championship in many competitions of the World Protein Structure Prediction Competition (CASP) (CASP13-16), leading more than 80 academic/industrial research groups around the world.
The bioinformatics team of the School of Statistics and Data Science at Nankai University where he works is recruiting new members.If you are interested in computational structural biology, bioinformatics, or data science, whether you are a master's, doctoral, or postdoctoral fellow, you are very welcome to join Professor Zheng Wei's team.
Interested students can contact Professor Zheng Wei via the following methods:
* Email: jlspzw@nankai.edu.cn
* WeChat: 18622152765
