Selected for AAAI 2025! BSAFusion Can Realize Multi-modal Medical Image Alignment and Fusion. Two Major Domestic Universities Jointly Proposed
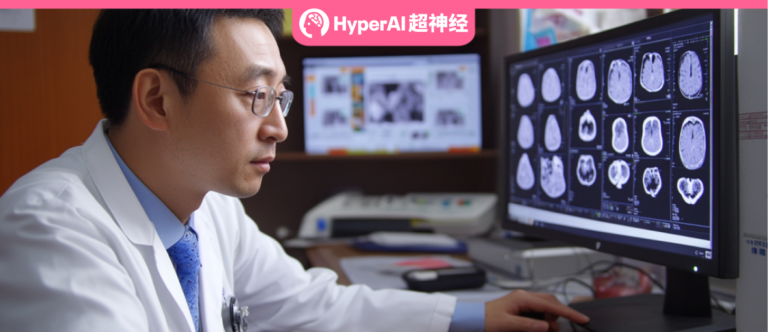
At the end of 2024, the 39th Annual AAAI Conference on Artificial Intelligence (AAAI 2025), the top international artificial intelligence conference, announced the results of the paper acceptance of this conference. In the end, out of the 12,957 submissions received, a total of 3,032 papers stood out and were included, with an acceptance rate of only 23.4%.
in,A research project jointly researched by Li Huafeng, Zhang Yafei, Su Dayong from the School of Information Engineering and Automation of Kunming University of Science and Technology and Cai Qing from the School of Computer Science and Technology of the Department of Information Science and Engineering of Ocean University of China——"BSAFusion: A Bidirectional Stepwise Feature Alignment Network for Unaligned Medical Image Fusion", attracted the attention of AI for Science researchers.This topic focuses on the field of medical image processing, which has been unprecedentedly hot in recent years, and proposes a bidirectional stepwise feature alignment (BSFA) unaligned medical image fusion method.
Compared with traditional methods, this study achieves simultaneous alignment and fusion of unaligned multimodal medical images in a unified processing framework through a single-stage approach. It not only achieves the coordination of dual tasks, but also effectively reduces the problem of model complexity caused by the introduction of multiple independent feature encoders.

Follow the official account and reply "Multimodal Medical Images" to get the complete PDF
The open source project "awesome-ai4s" brings together more than 100 AI4S paper interpretations and provides massive data sets and tools:
https://github.com/hyperai/awesome-ai4s
Medical Focus——Multimodal Medical Image Fusion
The so-called multimodal medical image fusion (MMIF)It is to fuse medical image data from different imaging methods, such as CT, MRI, PET, etc., to generate new images containing more comprehensive and accurate lesion information. The value of research in this direction in modern medicine and clinical applications is extremely important.
The reason is simple. After decades of technological development and accumulation, medical imaging has not only become more diverse in form, but also more and more common in use. For example, when people fall heavily, the first thing they think of is to go to the hospital to take a "film" to determine whether there is a fracture. "Film" usually refers to medical imaging examinations such as X-rays, CT or MRI.
However, it is obviously not enough to extract enough information from a single medical image to ensure the accuracy of clinical diagnosis in clinical medicine, especially when facing difficult and complicated diseases, such as tumors and cancer cells. Multimodal medical image fusion has become one of the important trends in the development of modern medical imaging. Multimodal medical image fusion integrates images from different times and sources in a coordinate system for registration, which not only greatly improves the efficiency of doctors' diagnosis, but also generates more valuable information, which can help doctors conduct more professional disease monitoring and provide effective treatment plans.
Before the application of medical images, many researchers have noticed the problem of image fusion and further explored methods to integrate multi-source image registration and fusion into a unified framework, such as the famous MURF. This is the first method to discuss and solve image registration and fusion in one dimension. Its core modules include shared information extraction module, multi-scale coarse registration module and fine registration and fusion module.
However, as mentioned above, firstly, these methods are not designed for multimodal medical image fusion, and they do not show the expected advantages in the field of medical imaging; secondly, these methods cannot solve the most critical challenges encountered in multimodal medical image fusion:The problem of incompatibility between the features used for fusion and the features used for alignment.
Specifically, feature alignment requires the corresponding features to be consistent, while feature fusion requires the corresponding features to be complementary.
This is actually not difficult to understand. Feature alignment is to achieve matching and correspondence of different modal data at the feature level through various technical means; while feature fusion is to be able to make full use of the complementarity between different modalities, so as to integrate the information extracted from different modalities into a stable multimodal model.
Therefore, the difficulty for MMIF can be imagined. This gap not only needs to be filled by someone, but also needs to be able to make multimodal medical image fusion more efficient and convenient based on the predecessors. In the paper,Both Professor Li Huafeng's team and Associate Professor Cai Qing's team expressed this original intention and put it into practice through research experiments.
From a technical point of view, this method proposes several designs with innovative value:
* First, by sharing the feature encoder, this method solves the problem of increased model complexity caused by introducing additional encoders for alignment, and successfully designs a unified and effective framework that integrates feature cross-modal alignment and fusion, achieving seamless alignment and fusion.
* Secondly, the Modal Discrepancy-Free Feature Representation (MDF-FR) method is integrated to achieve global feature integration by attaching a Modality Feature Representation Head (MFRH) to each input image, which significantly reduces the impact of modality differences and multimodal information inconsistency on feature alignment.
* Finally, a bidirectional step-by-step deformation field prediction strategy based on the independence of the vector displacement path between two points is proposed, which can effectively solve the problems of large span and inaccurate deformation field prediction encountered in the traditional single-stage alignment method.
BSAFusion pioneers new technology direction for medical image fusion
The single-stage multimodal medical image registration and fusion framework proposed by the research team,It mainly consists of three core components, namely MDF-FR, BSFA and MMFF (Multi-Modal Feature Fusion).The details are shown in the figure below.

It is not difficult to see that in MDF-FR,The Restormer and Transformer layers form the encoder of the network, extracting features from unaligned image pairs. Restormer and Transformer each have two layers. After two feature alignments and fusions, they are input into the subsequent MLP to obtain the prediction results.
Here, since the two modalities are quite different, cross-modal matching and deformation field prediction of these features will also face great challenges. Therefore, by generating modality-specific feature representation heads, we can reduce the impact of modality differences on deformation field prediction and prevent the loss of non-shared information due to the extraction of shared information.
Later, the team continued to use Transfer A and Transfer B to eliminate the differences between modes. Each Transfer block consists of two Transformer layers, and no parameters are shared between them, in order to further extract the features needed to predict the deformation site.
Arriving at BSFA,The research team designed a deformation field that predicts input image features from two directions - a bidirectional stepwise feature alignment method. A five-layer deformation field prediction operation was designed for both forward and reverse predictions, corresponding to the five intermediate nodes inserted between the two input source images. This method enhances the overall robustness of the alignment process. The forward registration layer is FRL, and the reverse registration layer is RRL.
Finally, in the MMFF module,The predicted deformation field is applied to align the features, and then multiple FusionBLK modules are used to fuse the features. Finally, the fused image is obtained through the reconstruction layer, and various loss functions are used to optimize the network parameters.
Of course, in order to ensure the effectiveness and rigor of the experiment, the research team made careful arrangements in the experimental details. In the experiment based on this model, the research team followed the protocol of the existing method.CT-MRI, PET-MRI, and SPECT-MRI datasets from Harvard were used for model training.These datasets consist of 144, 194, and 261 strictly registered image pairs, respectively, and the size of each object pair is 256 x 256.
In order to simulate the misaligned image pairs collected in real scenes, this experiment specifically designated MRI images as references and mixed rigid and non-rigid deformations on non-MRI images to create the required training set. In addition, the research team also applied the same deformations to 20, 55, and 77 pairs of strictly registered images to construct an unaligned test set.
The training process adopts an end-to-end approach, training 3,000 epochs on each dataset with a batch size of 32. At the same time, the Adam optimizer is used to update the model parameters with an initial learning rate of 5 x 10⁻⁵. The cosine annealing learning rate (LR) is used, which decreases to 5 x 10⁻⁷ over time.
The experiments used the PyTorch framework and were trained on a single NVIDIA GeForce RTX 4090 GPU.
Based on the research team's precise experimental details and standard data sets for training, this method also demonstrated excellent results in experimental experiments.
The experimental comparison objects are the five most advanced joint registration methods, including UMF-CMGR, superFusion, MURF, IMF and PAMRFuse. Except for the last group, the first four are not specially designed for multimodal medical image fusion, but are currently the best image fusion methods and are suitable for MMIF. As shown in the following figure:


The results are obvious. The method proposed by the research team shows stronger superiority in terms of feature alignment, contrast preservation and detail retention, and has the best average performance among all indicators.
Teams work together to safeguard medical clinical applications
One of the corresponding authors of this research topic is Cai Qing, an associate professor from the School of Computer Science and Technology, Faculty of Information Science and Engineering, Ocean University of China. In addition to working at Ocean University of China, he also holds important positions in several important academic institutions such as the China Computer Federation (CCF).
Professor Cai Qing's main research areas are deep learning, computer vision, and medical image processing.Multimodal medical image fusion, as a sub-field of medical image processing, has a strong professional knowledge barrier, and Cai Qing's many years of experience can provide guidance and assistance for this project.
It is worth mentioning that after Associate Professor Cai Qing was selected as the first author of a paper in AAAI 2024 last year, this year he was again the co-first author and corresponding author, and a total of 3 research projects were included in AAAI 2025. This includes another study on medical image processing, titled "SGTC: Semantic-Guided Triplet Co-training for Sparsely Annotated Semi-Supervised Medical Image Segmentation". In this paper, researchers proposed a new semantic-guided triplet co-training framework, which can achieve reliable medical image segmentation by only annotating 3 orthogonal slices of a small number of volume samples, solving the problem of time-consuming and labor-intensive image annotation process.
Paper address:
https://arxiv.org/abs/2412.15526
The other team for this project is Professor Li Huafeng and Zhang Yafei's team from the School of Information Engineering and Automation at Kunming University of Science and Technology.Among them, Professor Li Huafeng was selected into the latest list of the top 21 TP3T scientists in the world in 2021. He is mainly engaged in research on computer vision and image processing. Another corresponding author of this article, Associate Professor Zhang Yafei, mainly focuses on image processing and pattern recognition. He has presided over the National Natural Science Foundation of China regional projects and Yunnan Provincial Natural Science Foundation general projects many times.
Professor Li Huafeng, one of the important academic leaders in this project, has published research on medical image processing many times, such as a study entitled "Medical Image Fusion Based on Sparse Representation" as early as 2017, and a study entitled "Feature dynamic alignment and refinement for infrared–visible image fusion: Translation robust fusion" in 2023.
Paper address:
https://liip.kust.edu.cn/servletphoto?path=lw/00000311.pdf
Paper address:
https://www.sciencedirect.com/science/article/abs/pii/S1566253523000519
In addition, Li Huafeng has partnered with Professor Zhang Yafei many times to jointly publish related research, such as the research titled "Medical Image Fusion with Multi-Scale Feature Learning and Edge Enhancement" published in 2022. In this study, the team proposed a medical image fusion model based on multi-scale feature learning and edge enhancement, which can alleviate the problem of blurred boundaries between different organs in medical image fusion. The results obtained by the proposed method are better than the comparative method in terms of both subjective visual effects and objective quantitative evaluation.
Paper address:
https://researching.cn/ArticlePdf/m00002/2022/59/6/0617029.pdf
As the saying goes, a strong alliance is impeccable. The professional academic capabilities of Professor Li Huafeng, Professor Zhang Yafei's team and Associate Professor Cai Qing in the field of medical image processing are undoubtedly the key to the success of this project. We look forward to the continued cooperation between the two parties and to continue to publish cutting-edge results in the field of AI for Science in the future.
Hybrid multimodal medical image fusion becomes a trend
As multimodal medical image fusion plays an increasingly important role, its technological development is bound to move towards the direction of integration and intelligence.
As mentioned in this topic, in the study of fusion methods based on deep learning, researchers noticed that the CNN-based method and the Transformer-based method have complementary advantages. Therefore, some researchers proposed DesTrans, DFENet, and MRSC-Fusion. These studies use a hybrid approach to make the advantages of the two technologies complementary, thereby improving the efficiency of the fusion method.
In addition to deep learning-based fusion methods, multimodal medical image fusion methods also include traditional fusion methods, such as multi-scale transformation, sparse representation, subspace-based, salient feature-based, hybrid models, etc. Similarly, hybrid methods based on deep learning + traditional methods have also emerged.
The trends of the above studies can be seen.In the future, the method of multimodal medical image fusion will inevitably show a development trend based on deep learning as the mainstream, while mixing a variety of technical assistance.
