Global Warming Is Difficult to Reverse. Stanford Team Uses AI to Predict the Highest Temperature Changes, With a Record-breaking Probability of 90%
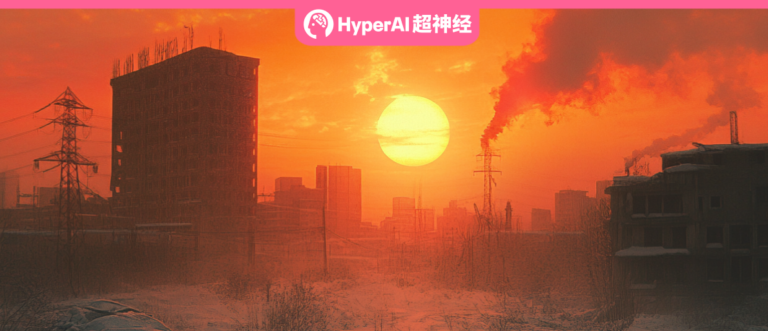
In recent years, against the backdrop of continued global warming, we have witnessed a series of rare extreme climate events that seem to silently announce the severe challenges facing our times. 2024, the year that is about to end, also presents us with amazing scenes: the Sahara Desert has suffered the worst floods in decades, the "Hurricane of the Century" in the United States has become the hurricane that has claimed the most lives in the past 20 years, heavy rains in Europe have caused the worst floods in decades, and Latin America has suffered a historic drought.
There is a famous line in The Wandering Earth: "At first, no one cared about this disaster. It was just a wildfire, a drought, the extinction of a species, the disappearance of a city. Until this disaster was closely related to everyone." With the continued warming of the global climate and the frequent occurrence of extreme weather events, a question that cannot be ignored has emerged before us: What is the reason behind all these problems? The general consensus points to human industrialization activities, especially since the Industrial Revolution, the surge in carbon emissions caused by human activities is considered to be the main driver of global warming. However, how big is the specific impact of industrialization on global warming?If the current trend continues, how will the Earth's temperature continue to change? These questions are gradually being revealed with the continuous advancement of artificial intelligence technology.
Recently, a joint research team from Stanford University, Colorado State University and ETH Zurich published two papers in the journals Geophysical Research Letters and Environmental Research Letters. They trained an advanced artificial intelligence convolutional neural network system and used a large number of climate models to simulate temperature and greenhouse gas data, and obtained a series of prediction results:Even if we can achieve rapid emissions reductions, the global average temperature may be 0.5 °C higher than the hottest year so far, 2023, with a probability of 90%. More worryingly, the model also predicts that if carbon emissions continue to grow, by 2060, the temperature in most parts of the world is expected to be 1.5 degrees Celsius higher than in 2023.
First paper:
Paper title: Data-Driven Predictions of Peak Warming Under Rapid Decarbonization
Paper link:
https://agupubs.onlinelibrary.wiley.com/doi/full/10.1029/2024GL111832
Second paper:
Thesis title: Combining climate models and observations to predict the time remaining until regional warming thresholds are reached
Paper link:
https://iopscience.iop.org/article/10.1088/1748-9326/ad91ca
The open source project "awesome-ai4s" brings together more than 100 AI4S paper interpretations and provides massive data sets and tools:
https://github.com/hyperai/awesome-ai4s
Based on different warming scenarios of the CMIP6 dataset, we train diverse CNN models for specific tasks.
Although both papers are based on multiple global climate models (GCMs) and convolutional neural network (CNN) models of CMIP6 and use similar datasets, they differ in the choice of datasets and the architecture of CNN models according to different research objectives.
The goal of the first paper was to predict the peak of global warming if decarbonization targets were achieved. To do this, the researchers trained multiple CNN models and selected the best one. The results show that during rapid decarbonization, the risk of a global hot year leading to extreme local climate conditions is considerable.
The second paper aims to predict global warming in different regions of the world in the 21st century under current emission trends. Given the complexity of the reality, the researchers selected GCMs under different conditions and used transfer learning to combine a series of climate model predictions with observational data. This method can ultimately make more accurate predictions of future temperature changes based on current climate conditions.
Specifically, in terms of data set constructionIn order to train CNN to identify multiple decarbonization paths, in the first paper, the researchers brought together data from multiple global climate models (GCMs) under decarbonization scenarios from the International Coupled Model Intercomparison Project (CMIP6), such as SSP1-1.9, SSP1-2.6, and SSP2-4.5. To ensure accurate capture of abnormal climate, the research team included at least 5 implementation versions of GCMs to balance the impact of different GCMs.
The second paper focuses on simulating the annual mean temperature anomaly more accurately, selecting GCMs with at least 10 implementations in CMIP6 under the SSP3-7.0 scenario. The research team selected 7 for training CNN, 2 for validation, and 1 for testing. They also re-gridded the annual mean temperature anomaly to a 2.5°×2.5° grid and calculated the climate mean anomaly for each grid point relative to 1951-1980.
The two papers also show significant differences in CNN structure and training methods.The first paper processes annual mean near-surface temperature maps and residual cumulative carbon dioxide emissions through CNNs, predicts a SHASH-distributed residual warming for each input, and trains more than 15 CNNs with different random seeds, from which the best ones are selected to predict peak warming until 2100.
In contrast, the second paper trained a CNN independently for each region. The researchers applied transfer learning on the Berkeley observational data to fine-tune the CNN trained on the climate model data (base-CNN) to produce a new CNN (transferred-CNN) that was more consistent with the observational data. Once the base-CNN and transferred-CNN were successfully trained, the research team made predictions for the global map based on the Berkeley observations and input the 2023 Berkeley annual average temperature anomaly and the expected threshold into the CNN.
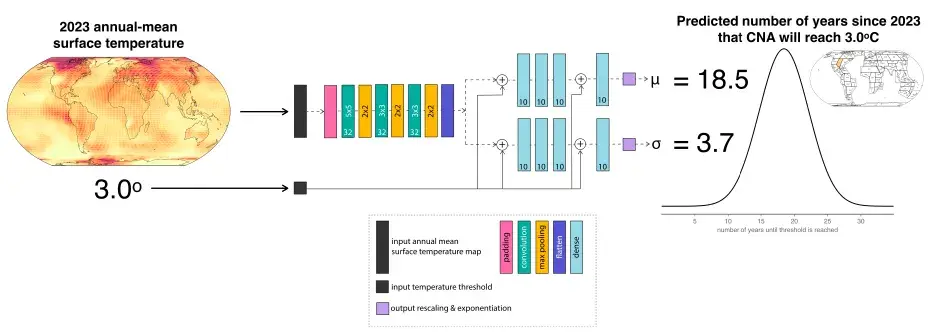
The world is facing a global warming challenge, and rapid decarbonization cannot reverse the warming trend
In terms of research details, the first paper first verified the accuracy of the prediction framework for different historical periods. The researchers trained CNN on CMIP6 historical simulation data and compared it with data from NASA and Berkeley Earth. As shown in Figure EF, the peak warming time series predicted by CNN showed high robustness to the initialization year, and the uncertainty of the CNN prediction results based on observational data was much lower than that of the CNN based on GCM simulation data.
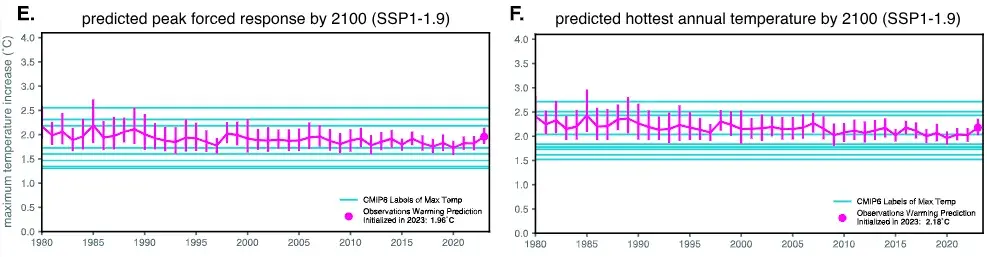
The projections further suggest that even with rapid decarbonization, the impacts of climate change could be more severe than what humans and ecosystems have experienced so far. By the end of 2023, climate change caused by human activities may have already caused global warming of about 1.5°C. Even under the most ambitious decarbonization scenario, global average annual temperatures will "almost certainly" exceed the 2023 data, and there is a 50% chance of rising by 2°C.Even if net zero CO2 emissions are reached by mid-century, it is very likely that individual years will experience global temperatures at least 0.5°C above the record-breaking 2023 anomaly.
The second paper predicts the continued increase in carbon emissions. The results reveal a grim fact: even with the most radical emission reduction measures, the trend of global warming is still difficult to completely reverse, and the intensification of extreme climate events such as heat waves, heavy rainfall and drought cannot be completely avoided. In addition,If current carbon emission levels do not change, most regions of the world will very likely face regional warming exceeding 2.0°C by 2040.This forecast highlights the urgency of the global warming problem, indicating that even under the most optimistic emission reduction scenarios, the impact of global warming remains inevitable, and the continued increase in carbon emissions will make the situation more severe.
As shown in the figure below, the study found that for the 1.5°C threshold, all major projected regions will reach it by 2040 or earlier. For the 2.0°C threshold, the vast majority of regions will reach it before 2040, and all regions of the world will reach it no later than 2060. For the 3.0°C threshold, all regions of the world will reach it no later than 2070, except for one region.
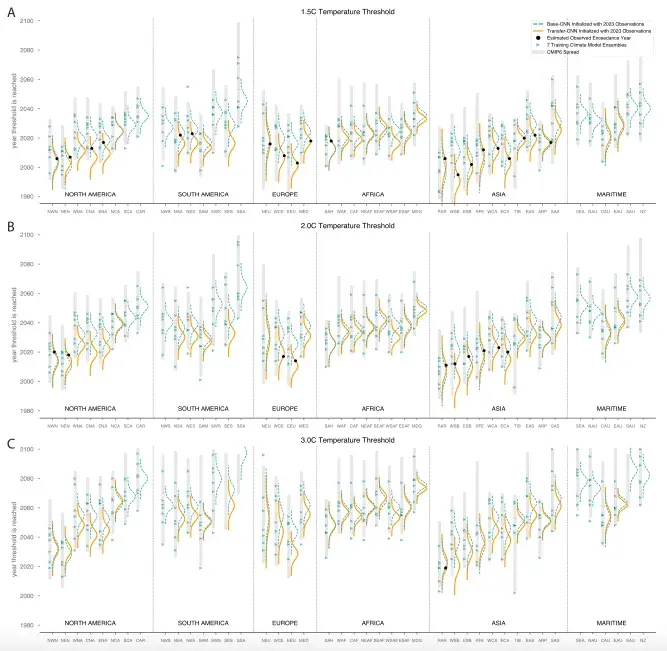
Focus on sustainable development and earth science research
It is worth mentioning that one of the key figures in this article, Professor Noah Diffenbaugh, has a prominent background in the Doerr School of Sustainability. In May 2022, John Doerr, a billionaire investor in Silicon Valley, donated $1.1 billion to Stanford University to establish the Doerr School of Sustainability. As an investor in Silicon Valley technology giants, Doerr is also a staunch supporter of the development of sustainable technologies. He has invested in zero-emission technologies and given speeches to encourage companies to adopt clean energy technologies to address global warming.
In just two years, the Durr Institute for Sustainability has already produced a number of research results. In addition to the two results discussed in this article, the institute has also launched the Mineral-X affiliate program, which aims to use artificial intelligence technology to locate key mineral deposits and build a resilient mineral supply chain to support the development of clean renewable energy. In addition,The college has also used AI technology to carry out a number of studies, including carbon dioxide zero emission monitoring, heat wave changes and global warming, and the impact of climate change on human diseases.
Professor Noah Diffenbaugh, author of two studies in this article, is a scientist who has long been concerned about global climate issues. Before the rise of the AI technology wave, Professor Diffenbaugh published a striking research result in the Proceedings of the National Academy of Sciences on April 22, 2019, pointing out:"In a study of the impact of global warming on social inequality, it was found that most poor countries have become poorer, while most rich countries have become richer, compared to before global warming."
With the revolutionary impact of AI technology, Professor Diffenbaugh also keeps up with the application of AI in the field of AI4S. In the January 2023 issue of the Proceedings of the National Academy of Sciences, he pointed out that global temperatures have risen by 1.1 or 1.2 degrees Celsius since the pre-industrial era or the mid-19th century, and artificial intelligence predicts that the earth will exceed the threshold of 1.5 degrees Celsius warming between 2033 and 2035. However, data from the United Nations show that global temperatures in 2023 have exceeded 1.5 degrees Celsius, and the global temperature rise has exceeded expectations.
In addition to Professor Diffenbaugh's work, the application of AI in the field of predicting global warming has become very extensive. For example, in July this year, the artificial intelligence model NeuralGCM developed by the Google Research team took weather forecasting and climate simulation to a new level. NeuralGCM performs well in long-term climate forecasting. Its 40-year climate forecast simulation results are consistent with the global warming trend shown from ECMWF data.
With the continuous advancement of AI technology, we have reason to believe that the speed and accuracy of weather forecasts will be further accelerated.