The Tactile Revolution of Embodied Intelligence in the Future! TactEdge Sensors Enable Robots to Have Fine Tactile Perception, Enabling Fabric Defect Detection and Dexterous Operation Control
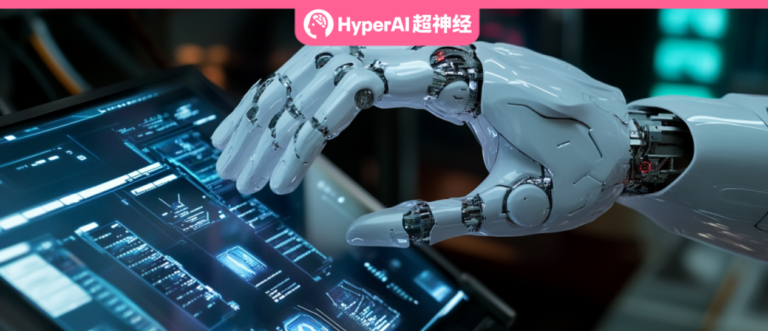
In our imagination, a perfect robot should have the same vision, touch, hearing, smell and taste as humans.
Perceiving and understanding the physical world through the above “five senses” and using a powerful AI brain to achieve accurate response to the environment is the core of building intelligent robots, and technology giant Tesla also agrees with this.
For example, their second-generation humanoid robot Optimus has enhanced tactile perception and can perform the dexterous operation of pinching an egg with two fingers.This accurate perception of objects and precise control of force are due to the tactile sensors on the tips of its ten fingers.
Humans use touch to assess the size and shape of objects, and so do robots. With the help of tactile sensors, robots can better understand the interaction behavior of objects in the real world and obtain tactile information such as the texture, temperature, hardness and deformation of the target object, so as to accurately locate the object and perform various operations (such as grasping) tasks.In short, operation is inseparable from the sense of touch, and tactile sensing has great application potential in the field of robotics.
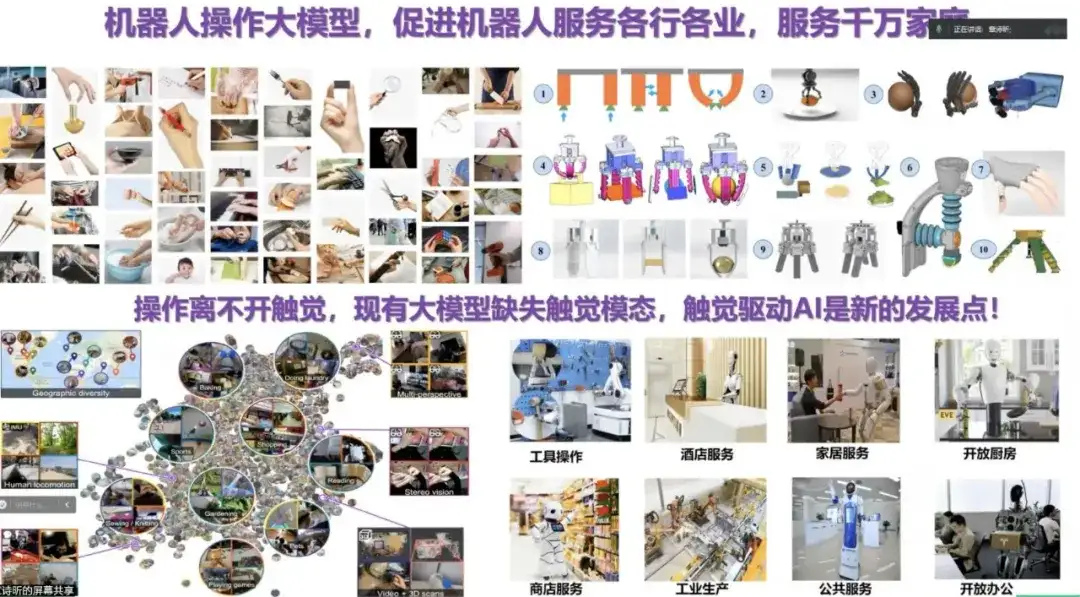
On December 13, the third online sharing event "Newcomers on the Frontier" hosted by the Embodied Haptic Community and co-organized by HyperAI officially opened.Zhang Shixin, a fourth-year doctoral student at China University of Geosciences (Beijing), was invited to this event. His speech was titled "Design, Preparation and Robotic Perception Operation of TactEdge Sensors".We gave a detailed introduction to the iteration history, hardware optimization, visual and tactile simulation, and robot perception operations of the visual and tactile sensor TactEdge.
HyperAI has compiled and summarized Dr. Zhang Shixin’s in-depth sharing without violating the original intention.
TactEdge visual tactile sensor upgrade history
In the past, people used electronic tactile sensors to count tactile information, but the sensing units of these tactile sensors are sparsely distributed, and the resolution is relatively low when mapping tactile information. In order to improve the quality of tactile information, researchers introduced a new sensing mechanism:Tactile sensors based on visual recognition principles (also known as visual tactile sensors) use images as sensing media and significantly improve the tactile quality, especially in terms of spatial resolution.The evolution of visual and tactile sensors is shown in the following figure:
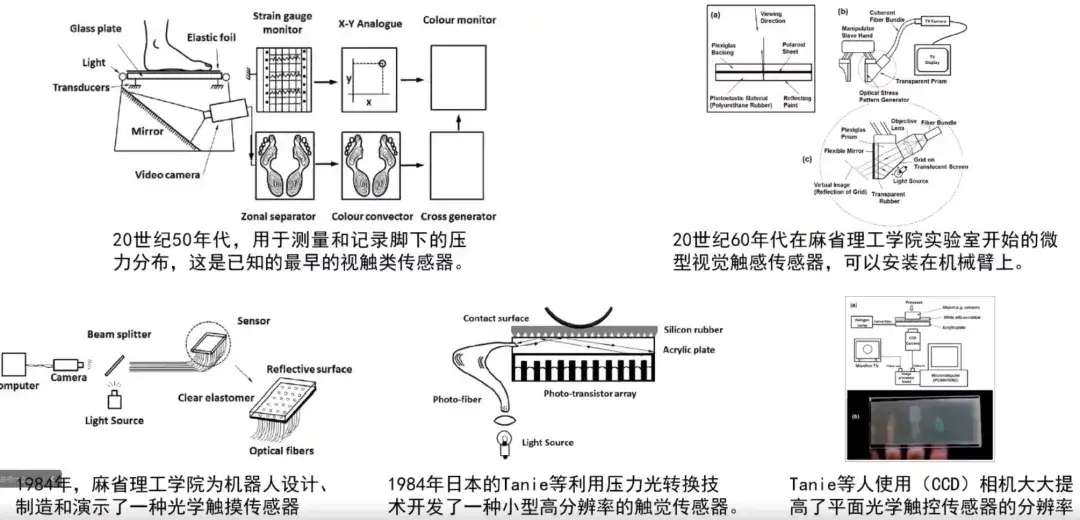
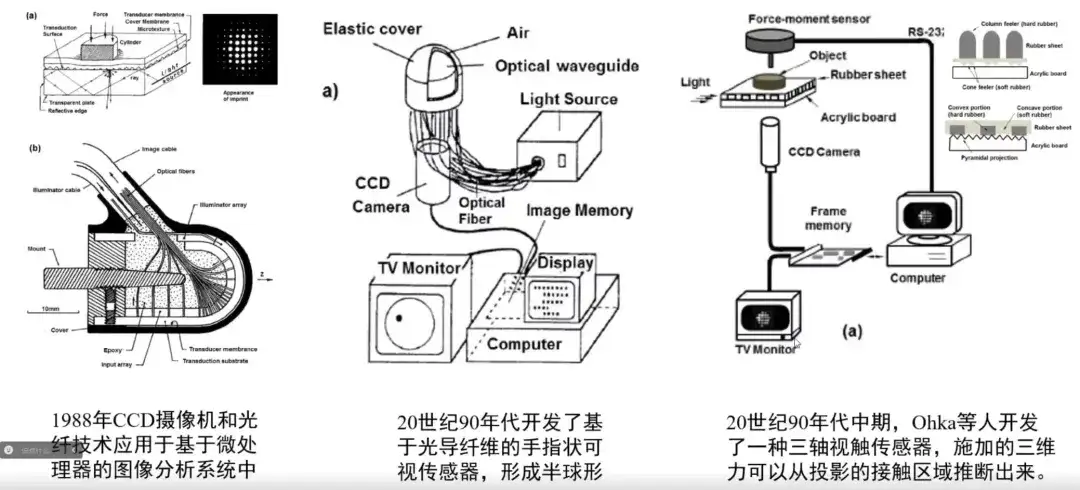
After the 20th century, researchers proposed a new visual-tactile sensing method. Their work can be summarized into three main modules: contact module, camera module, and lighting module, which are used to standardize the visual-tactile sensing mechanism.Among them, the contact module includes a marking layer, a coating, and a functional layer for transmitting tactile information (such as a temperature-sensitive layer), and then the tactile information is visualized through inverse imaging technology.
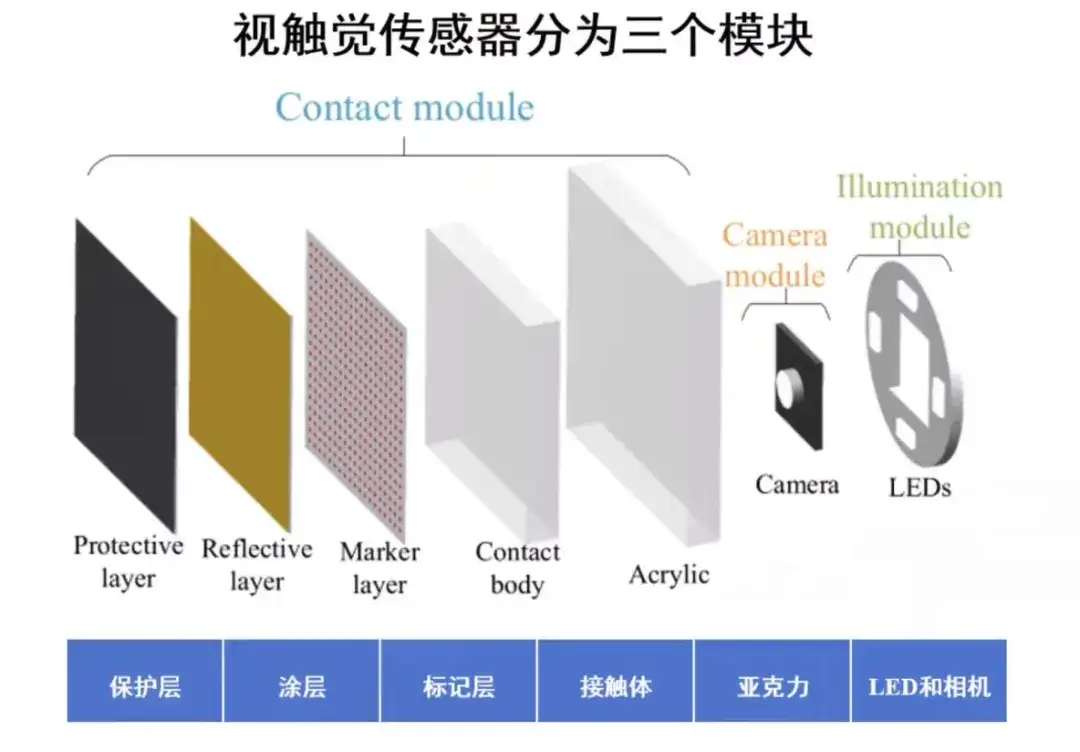
Our team has been researching visual tactile sensors since 2014, and it has been 10 years since then.We have explored and developed multiple generations of sensor technology and call it a cutting-edge tactile technology, TactEdge.As shown in the following figure:
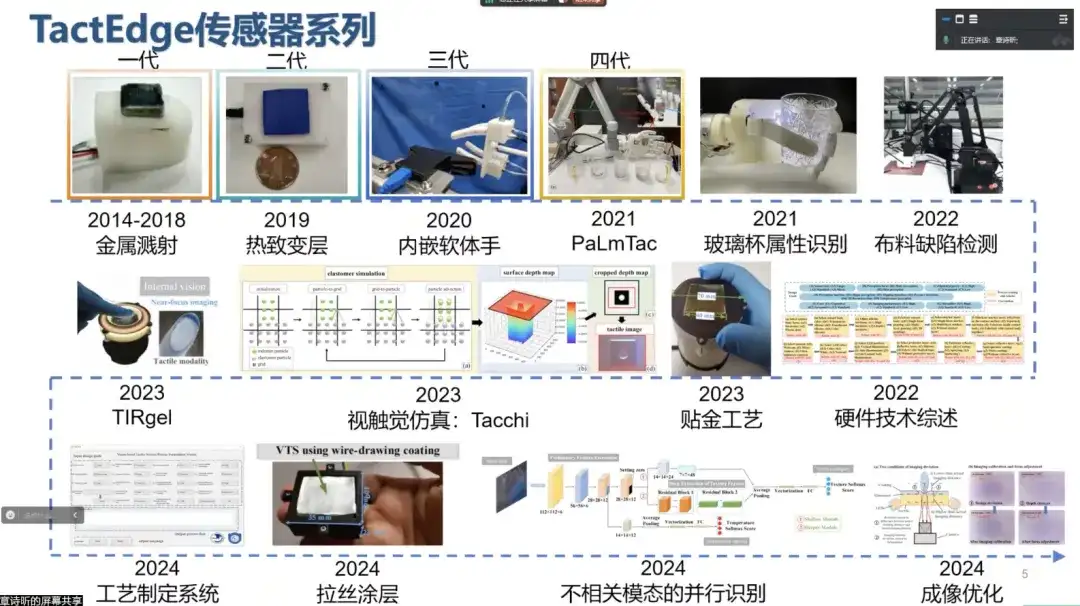
* The first generation of TactEdge adopts a coating design.Through metal sputtering and masking processes, a thin metal coating and a standard marking array were prepared, which have dual-modal tactile sensing capabilities.
* The second generation of TactEdge adds thermotropic materials to the coating or marking material.Because each material has a different temperature threshold, you can see the color change from light to dark when the temperature rises or falls, thereby gaining local temperature perception.
* The third generation TactEdge is a software hand with integrated visual and tactile sensors.The bending posture is tracked by using embedded vision to monitor the deformation state of the inner cavity.
* Fourth Generation TactEdge:The coating is used for texture mapping, the marking layer realizes force tracking, and the temperature sensing layer is responsible for temperature perception. In order to achieve multimodal perception, we combine these functional layers. The fourth-generation TactEdge combines the thermotropic layer with the coating to achieve multimodal perception of texture and temperature.
* The fifth-generation TactEdge has made great improvements in radial dimension optimization of the sensor and robustness of tactile imaging.In addition, the marking layer is combined with the coating to achieve dual-modal perception of force and texture.
* The sixth generation TactEdge uses a new visual tactile sensing mechanism TIRgel,By implementing total internal reflection inside the elastomer, photometric information is created to represent the tactile information, and a focus-adjustable camera is introduced to convert the internal and external vision.
Hardware Optimization
In the sensor development process in recent years, we have focused on hardware optimization.For example, in 2021, we summarized the current mainstream sensor preparation technologies (such as elastomer preparation process, marking layer preparation process, coating preparation process, and support structure form), and carried out process coding on this basis, and summarized 7 design goals, as shown in the figure below. These goals depend on the application requirements of visual and tactile sensors.
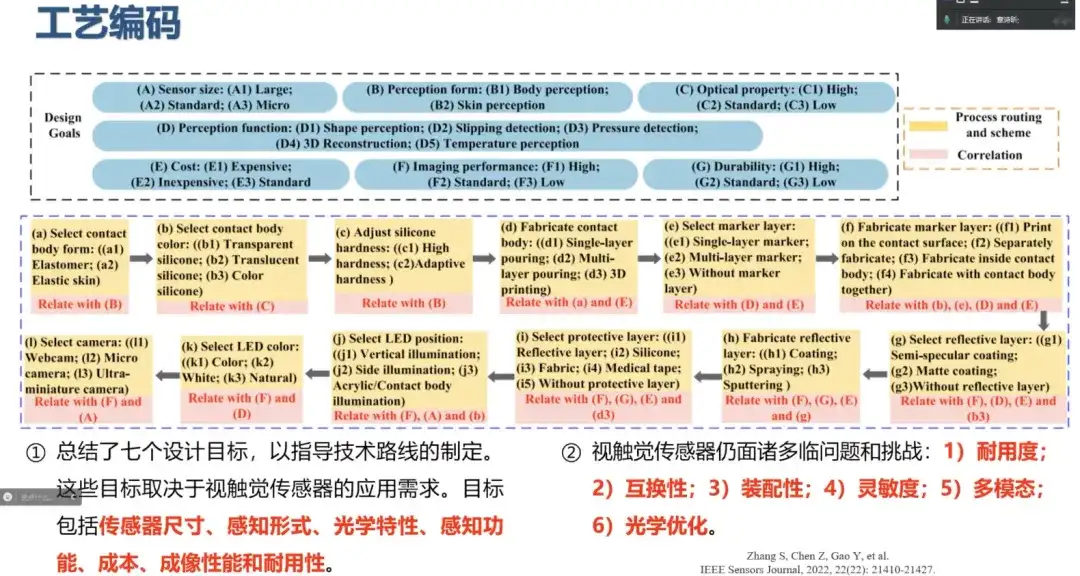
According to these sensing requirements, we added various constraints and constructed the intrinsic relationship and logical framework between the process and the design goals. Based on this, we established the entire process formulation system and developed a process formulation system visualization interface based on QT. As shown in the figure below, users can enter the design goals in the green box, and the system background will match the reasonable preparation process according to these goals to assist the team in developing sensors. However, only recommended preparation process assistance is provided here. Some requirements in special scenarios still require specific local optimization of the process.
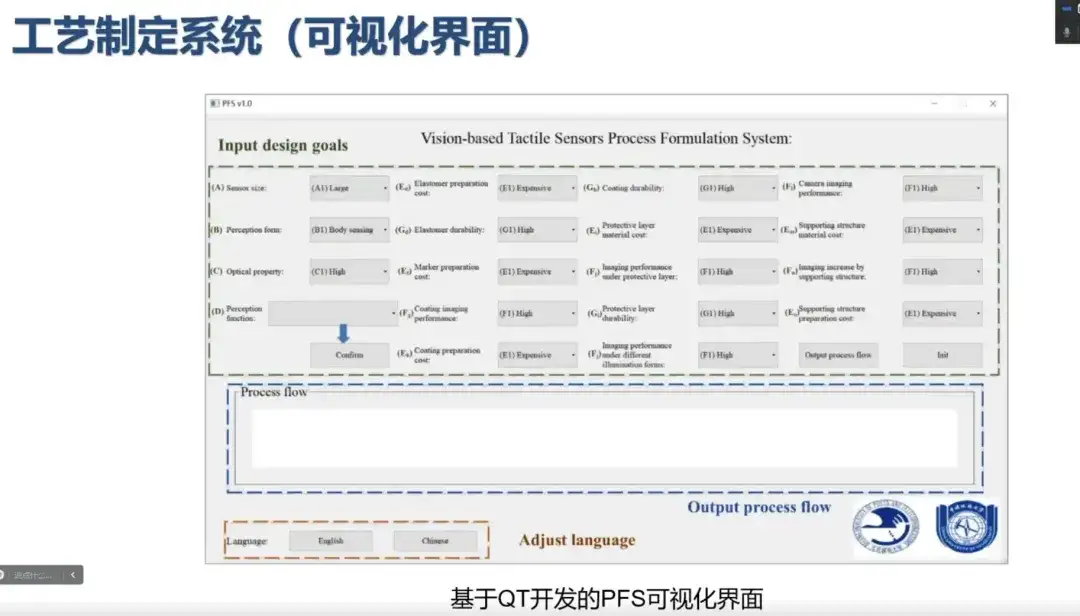
Elastomer process optimization: simplified preparation process + lower cost
For example, the common preparation process of elastomers includes mold setting, silicone mixing, casting, vacuuming, heating and demolding, followed by various coating processes. This is a common elastomer preparation process. In mass production, considering the long heating time, a self-curing special silicone material can be used. This material does not require additional heating after curing and vacuuming. In addition, in order to reduce the cost of preparing sensor elastomers, our team also proposed a lamination process.
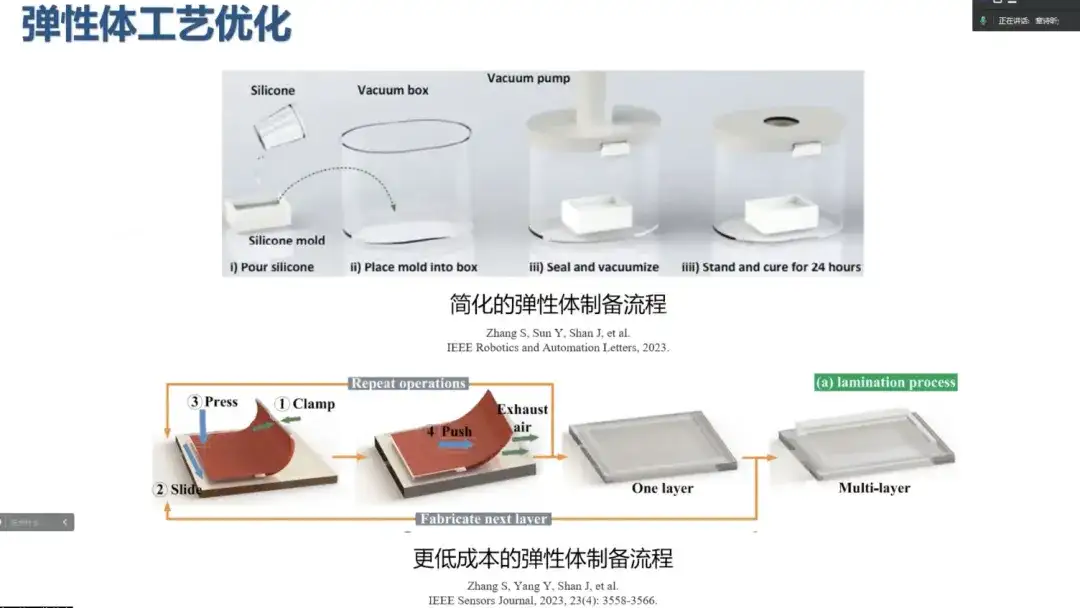
Coating process optimization: wear resistance and ductility
At present, the coating preparation schemes for sensors are mainly divided into spraying process and metal sputtering process. Metal coatings will completely fall off and wear out after frequent touch. The wear resistance of spray coatings is slightly better, but local damage will occur when encountering sharp objects. Therefore, coating quality and wear resistance are issues that cannot be ignored. In the past, coating preparation was more inclined to mechanical attachment. A few years ago, someone proposed the concept of chemical attachment, which is to attach coating materials to the surface of uncured elastomers to form chemical bonds before curing to enhance wear resistance and adhesion.
Gold plating process: improve wear resistance
In this regard, we proposed a new coating optimization solution - gold plating process to improve its wear resistance. The main thing is to attach the metal foil to the elastomer to form a thin coating. This solution achieves a double optimization of adhesion strength, that is, the peroxide on the surface of the metal foil and the acrylic tape (methyl methacrylate) trigger a chain polymerization reaction to form a chemical bond. In addition, the elastomer and the metal foil form van der Waals force.
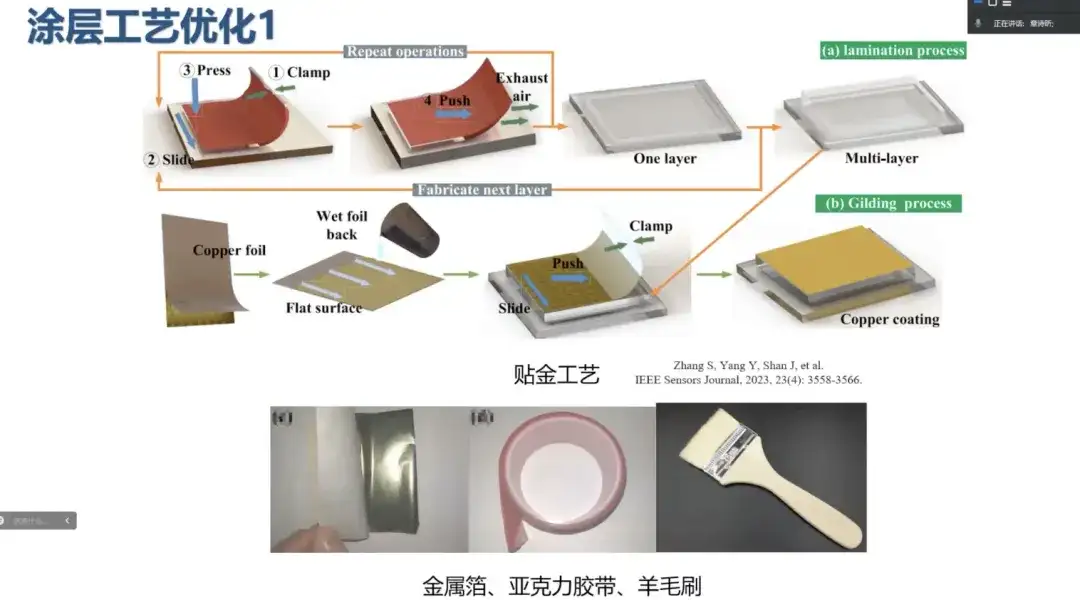
Compared with traditional spraying and metal sputtering processes, the gold plating process has significant advantages in hardware cost, preparation cost and time loss. For example, the spraying process and the trouble of cleaning the spray gun are greatly simplified, shortening the preparation cycle of the sensor. In addition, the new process not only improves the wear resistance of the functional layer, but also, under skilled operation, the preparation time of the entire contact module may be shortened to 5-10 minutes. Another significant advantage of the gold plating process is that it is easy to maintain. The coating surface is usually attached with a protective layer, such as fabric or medical tape. The thickness of these protective layers will affect the tactile sensitivity and fine texture mapping, but the gold plating process allows secondary operations on damaged coatings, which is conducive to maintenance.
Wire drawing process: improving ductility
However, in actual application, we found that since the metal foil itself is a continuous coating, it is easy to crack during the pressing process. We believe that the continuous accumulation of metal foil particles can form a continuous coating on a macro scale, thereby improving the ductility of the coating. Therefore, we improved the gold plating process and proposed the wire drawing process.
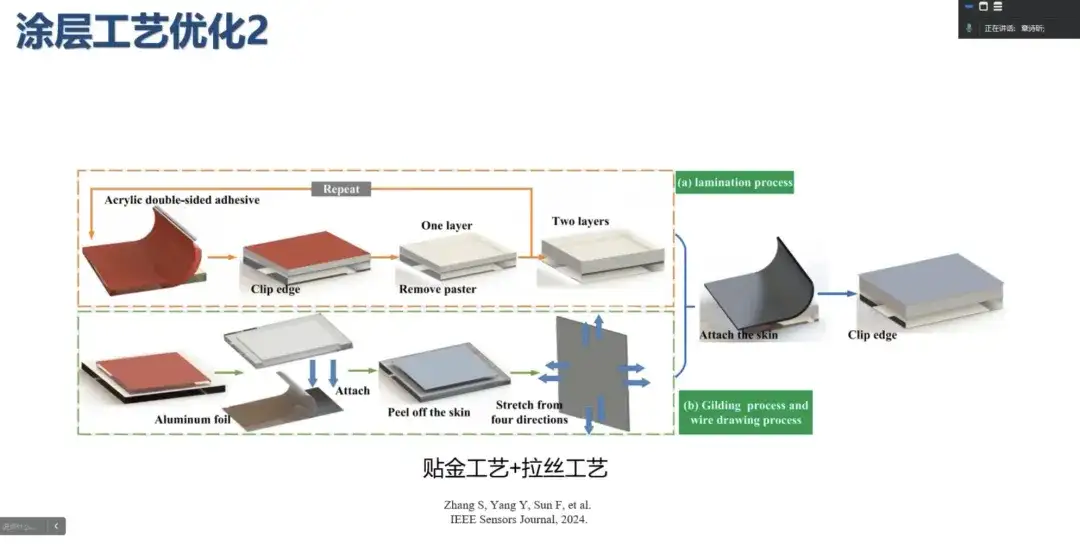
We stretched the metal foil. Because it has a rich slip system, fine particles can be formed during plastic deformation. The higher the tensile strength, the finer the particles. Different metals have different slip systems and different particle sizes. Therefore, we used copper foil, aluminum foil and silver foil for experiments. The microstructure under an electron microscope shows:
* Copper foil: Since the slip system is not as rich as that of aluminum foil and silver foil, the particles are relatively coarse during the stretching process, which affects the continuity of tactile imaging.
* Aluminum foil and silver foil: The particles after stretching are very fine and have nanoscale particle distribution, which significantly improves the spatial resolution of tactile imaging.
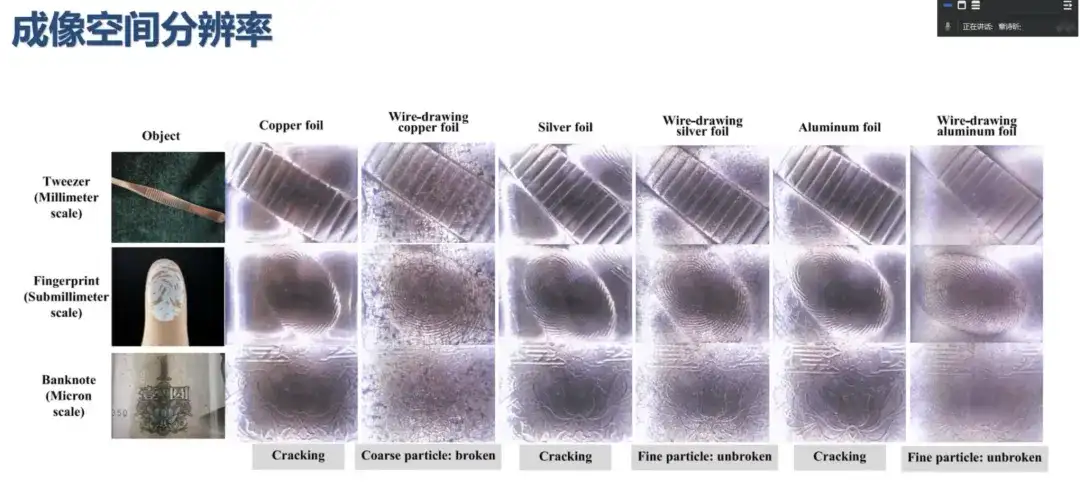
We compared the brushed coating with the current mainstream spraying process and metal sputtering. As shown in the figure below, our process is the best in terms of texture mapping effect.
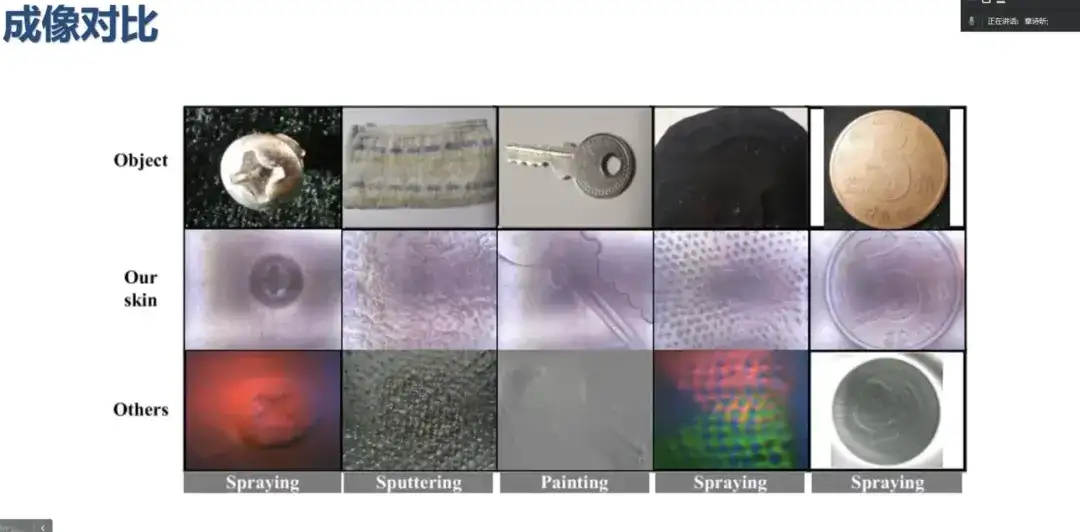
As shown in the figure below, the thin coating is easy to break during the pressing process, and the speed and degree of rupture are faster when pressed by a sharp object. However, the granulated brushed coating shows stronger resistance to irregular deformation, which indicates that the granulation process improves the ductility of the thin coating.
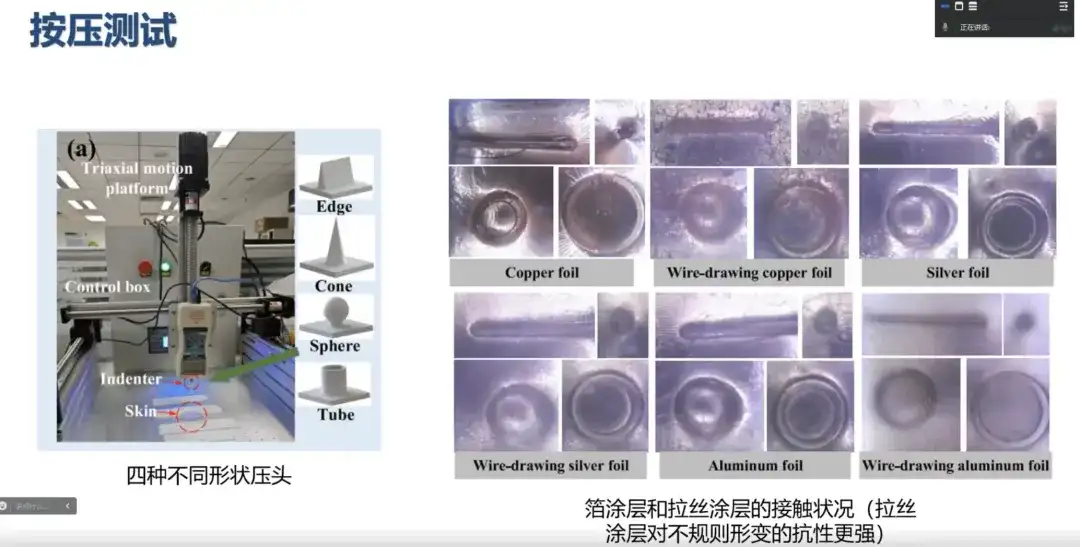
In order to evaluate the wear resistance of the coating after double adhesion strengthening, we conducted wear resistance tests and recorded the changes in the coating microstructure. The results showed that the particles of the brushed coating became finer during the wear process, and the overall wear was uniform; while the sprayed coating had weak adhesion, and localized shedding occurred during the wear process and accumulated in the surrounding area, forming pits.
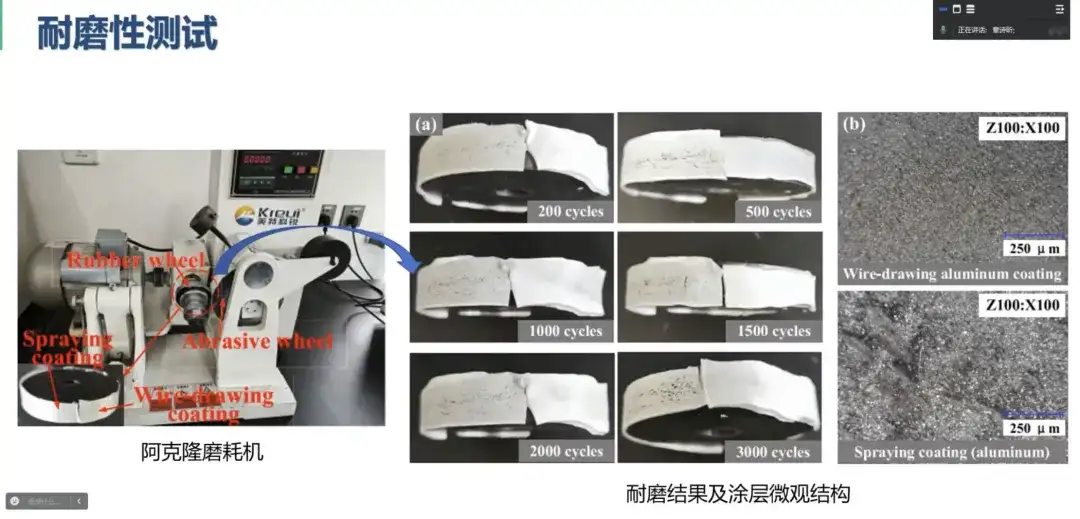
Visual tactile imaging optimization: Improving the robustness of visual tactile imaging
In addition, the improved coating also enhances the robustness of visual-tactile imaging to a certain extent.
In visual tactile sensing, the imaging distance is very small, and most of them are macro imaging. In this case, each press will change the imaging distance, resulting in imaging deviation. In addition, there may be a difference between the preset imaging distance when the sensor is designed and the imaging distance in actual use, both of which will lead to insufficient imaging clarity. To solve these problems, we have developed an imaging adjustment system, which includes a calibration module and a focusing module.
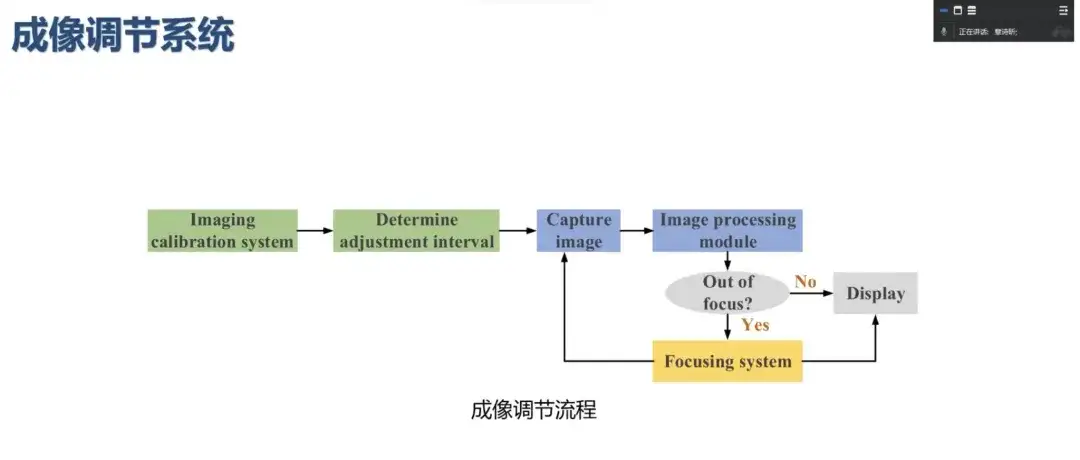
In the imaging adjustment system, the imaging calibration module uses a global search strategy to advance the adjustment interval and shorten the focusing distance, while helping to determine the reasonable adjustment value as a label. The focusing module uses two methods: the focus depth method based on three-point fitting and the defocus depth method based on deep learning. The former has high accuracy but slow speed, while the latter is faster but slightly lower in accuracy. It is an end-to-end adjustment method.
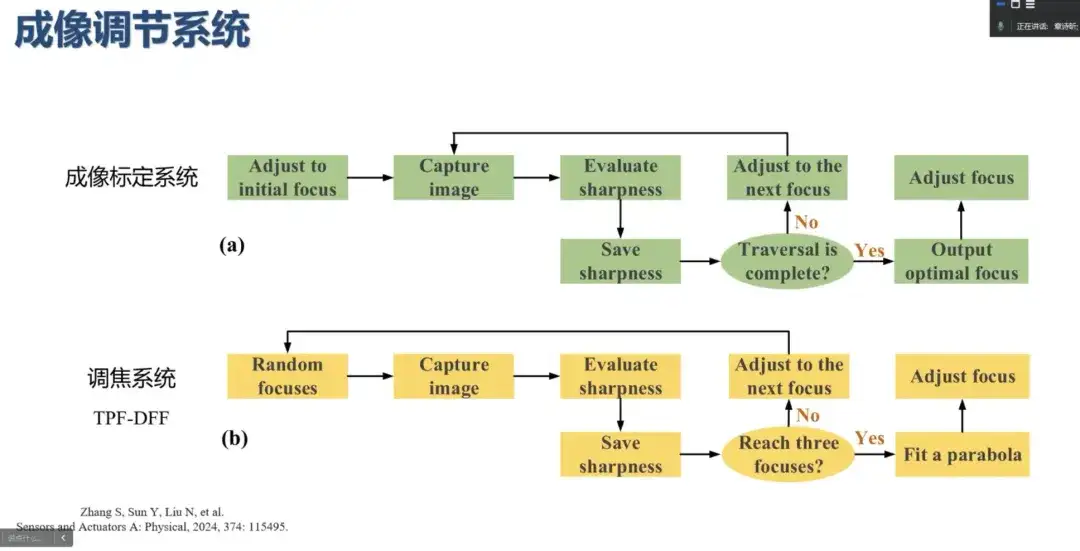
Given that visual tactile sensing relies on image mechanisms, we mainly use image clarity as the standard for focusing. As shown in the figure below, the ideal imaging clarity evaluation curve should be unbiased and unimodal (red curve). However, in the actual focusing environment, the light is often uneven (blue curve) and will fall into a local focusing peak.
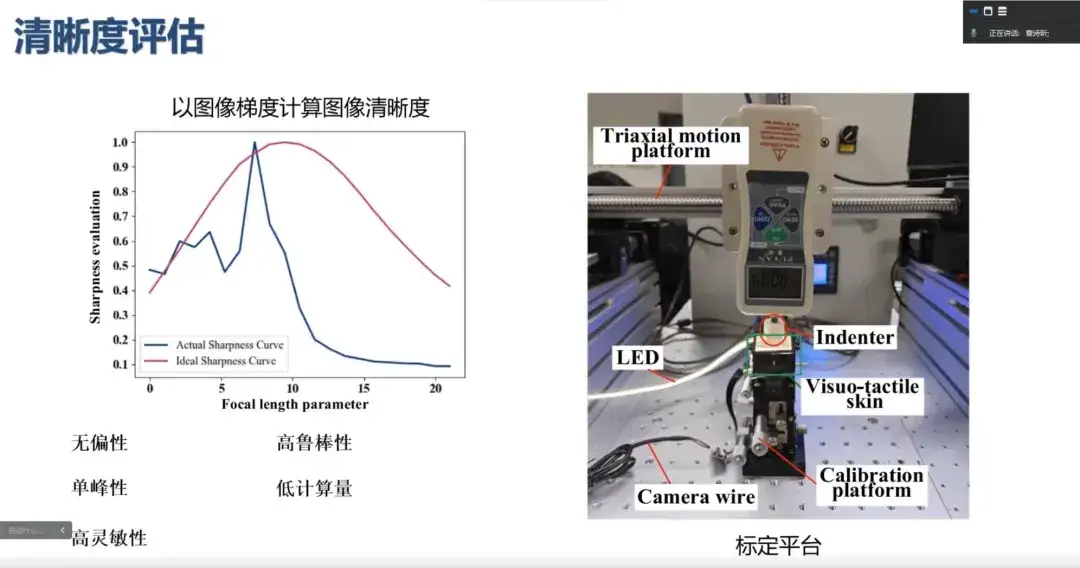
Visual tactile sensing used in a closed environment can reduce external interference factors and make the imaging curve closer to the ideal state. To this end, we built a calibration platform and tested the imaging curve of visual tactile sensing to explore the image gradient calculation method suitable for this sensor. The results show that the Tenengrad gradient calculation method is more suitable for the sensor matching degree, and the fitting curve has the five key characteristics proposed, especially unbiased and unimodal, which is crucial for the subsequent focus evaluation.
Normally, we randomly set an initial focus value, and after a slight press, calibrate the imaging to obtain a clear image, and the imaging is still within an acceptable range.
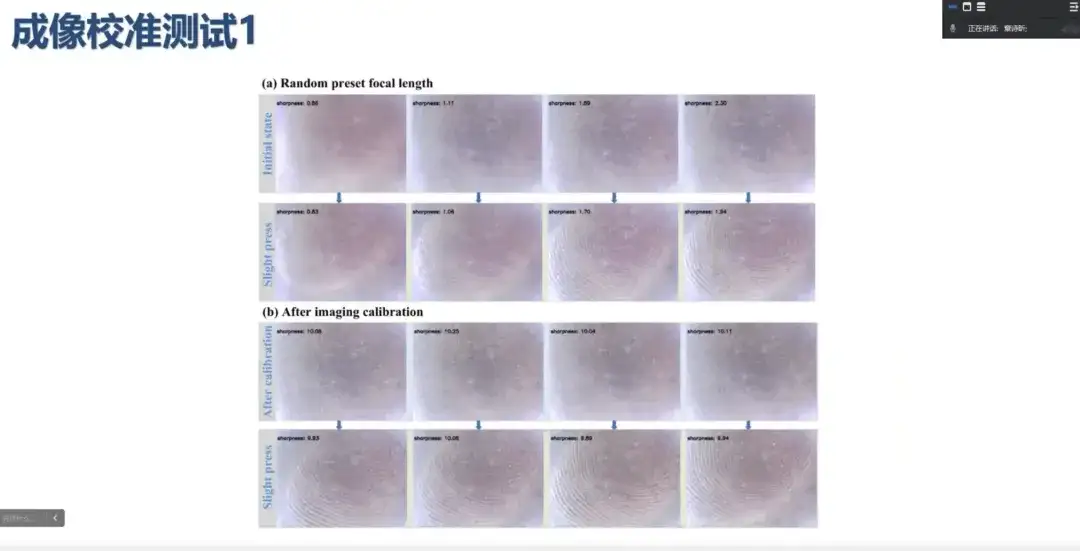
In addition, the dynamically changing sensing domain needs to be considered during the sensor design phase so that the imaging distance can be expanded or reduced according to the specific scenario. As the imaging distance increases, the sensing domain will also expand, and the overall design can be simplified by dynamically responding to imaging focus. As shown in the following figure:
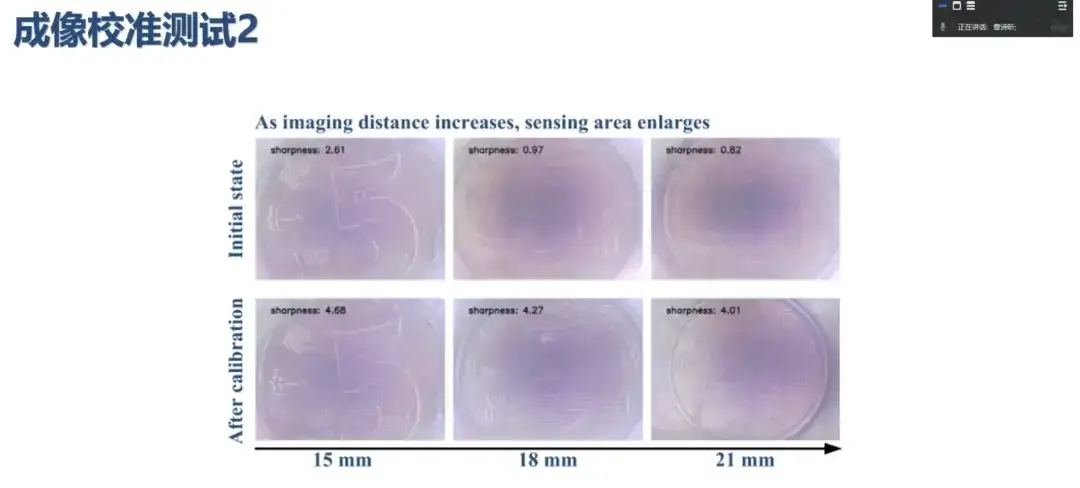
To test the focusing accuracy, we conducted experiments using objects with three different density textures. The results showed that both calibration methods were close to the expected results, with an imaging adjustment accuracy of more than 99.5%, and real-time focusing in dynamic recognition tasks.
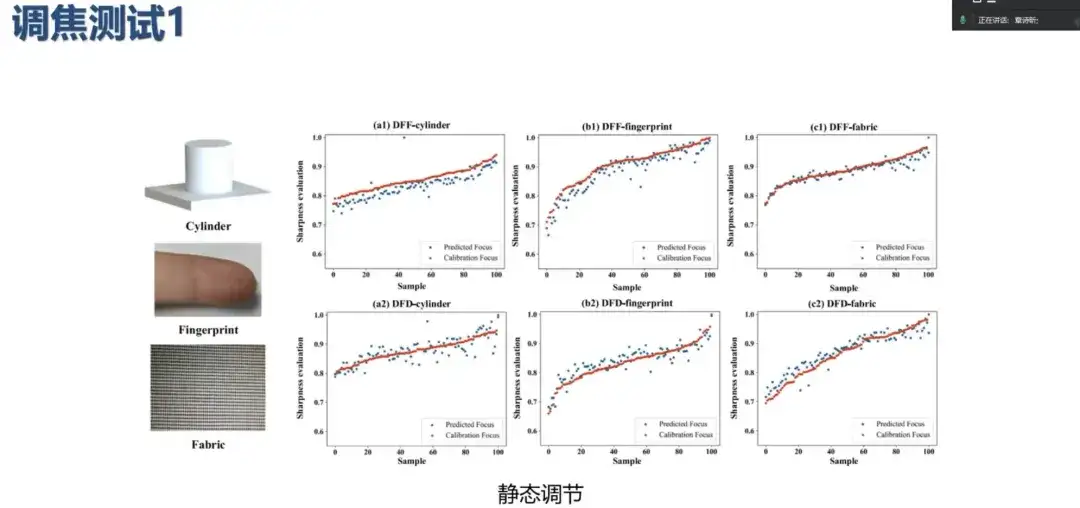
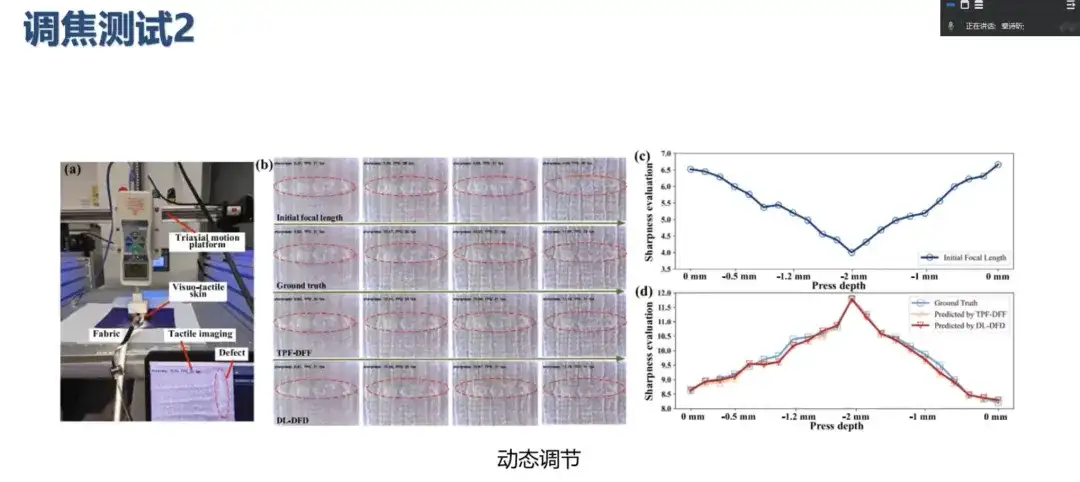
Temperature Sensing Layer Optimization: Distributed Modal Design
In terms of the optimization design of the temperature-sensing layer, there is relatively little research at present. The mainstream method is to add temperature-sensitive materials to the functional layer of the sensor, for example, thermotropic powder and thermotropic oil. From the preliminary imaging results, the color information of this method is fused with the texture information. However, there is no direct correlation between temperature and texture. It is unreasonable to fuse these unrelated features in the image without proper feature extraction or separation, and then input them into the model for recognition.
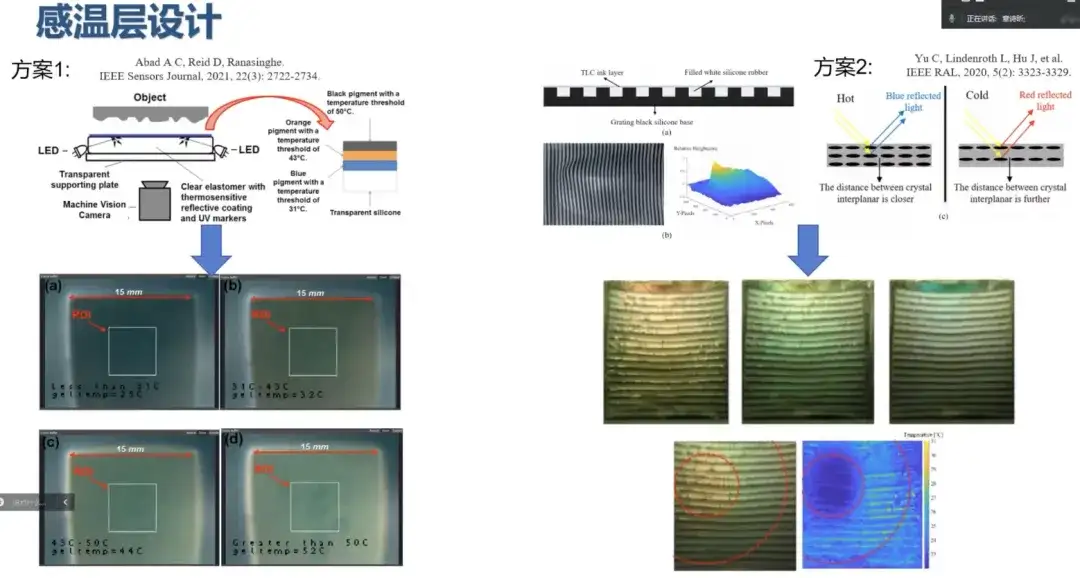
To solve this problem, we performed a distributed mode design for the temperature-sensitive layer and the coating, and the sizes of the two regions match the feature extraction mechanism. We will explain this in detail later.
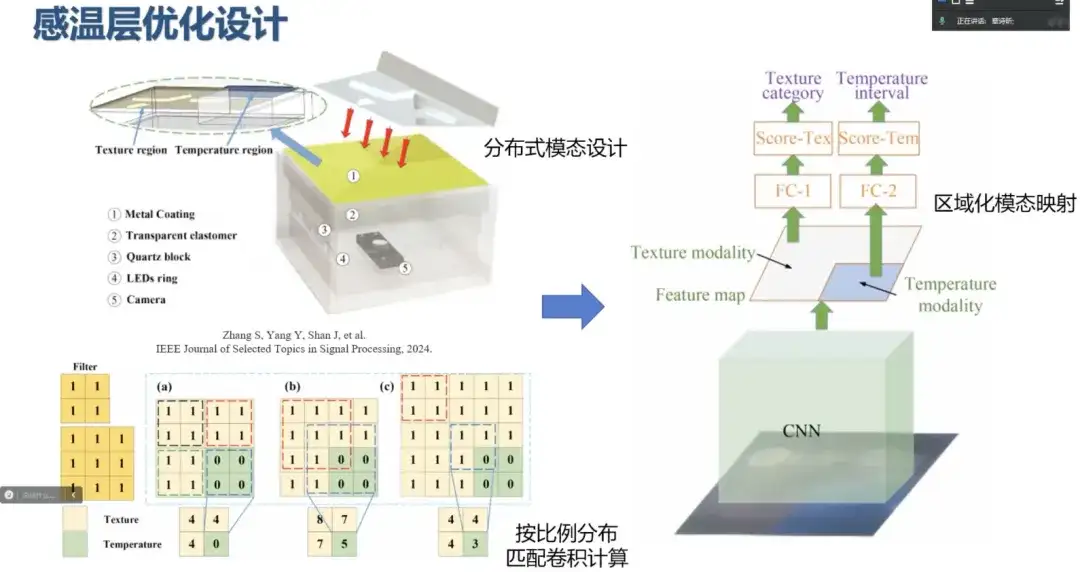
Internal structure optimization: miniaturization improves sensor integration
Considering the need for miniaturization of visual tactile sensors, we hope to reduce their size to improve the integration of sensors. Thanks to the development of microscopic imaging technology, we have effectively reduced the image size and modularized the internal structure of the sensor. By integrating components that are not often equipped, it is easy to assemble and disassemble, and the maximum use of space is achieved. Combined with different connectors, micro visual tactile sensors can be integrated into various manipulators to achieve high integration and high compatibility.
Visual and tactile simulation
Although our method improves the wear resistance of the visual tactile sensor coating, it still wears out after thousands of uses. In this case, we need to collect large-scale data, which is not realistic to rely solely on manual work. Therefore, we carried out research on visual tactile simulation, mainly based on Taichi language and MLS-MPM method to simulate the deformation of elastic bodies.
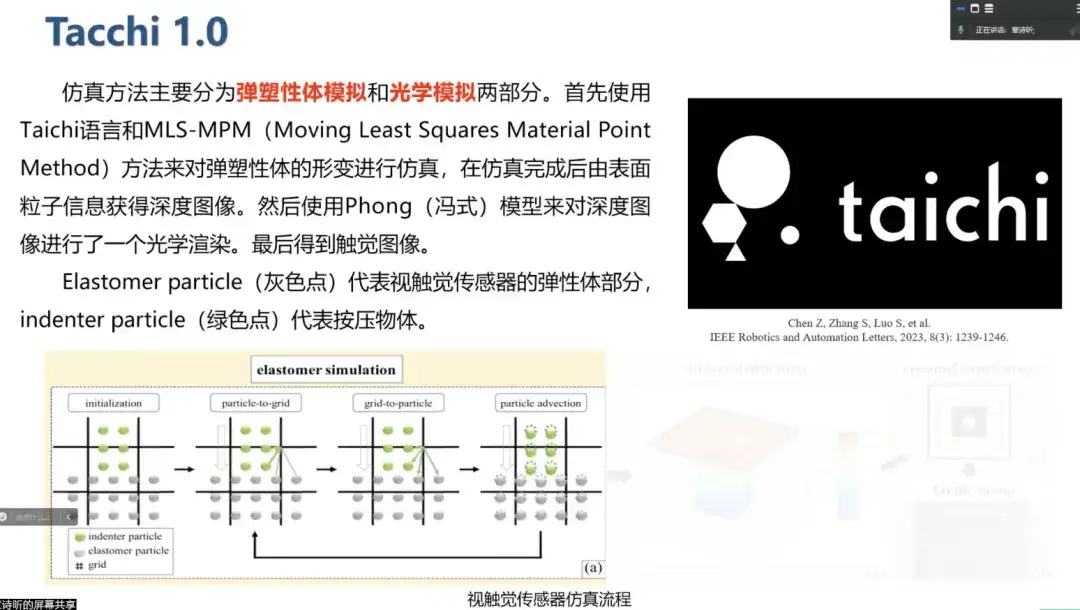
Elastic simulation mainly uses granular particles to represent elastic bodies and indenters, and transfers the physical properties of particles (such as momentum and mass) to the grid during each simulation step. The state of the particles is then updated using the previous state of the grid nodes and particles. After the simulation is completed, depth information can be obtained. Tacchi can also be connected to other robots for joint simulation. During the pressing process, the particles will change frame by frame, and finally a particle depth map will be formed and rendered. Furthermore, in Touch 2.0, we have added a mutual information transmission mechanism between particles and grids, improved the simulation effect of sliding objects, and used ray tracing to enhance the realism of rendering, making the new version more detailed than Touch 1.0.
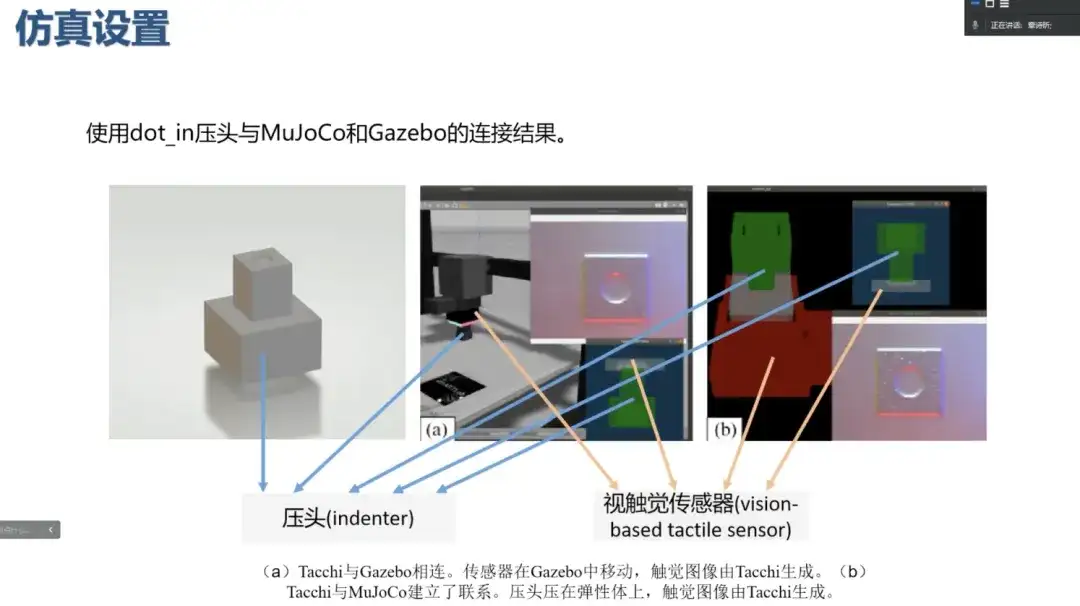
Robotic Perception Operation
Based on hardware and simulation, visual and tactile sensors are expanding into different perception fields. Our TactEdge machine is used to solve recognition problems in different fields. We are also making some interesting attempts in robot operations.
Fabric recognition: can be used for fabric defect detection
Because fabrics have fine textures and distinct geometric features, they were used to verify the texture mapping effect of visual tactile sensors in the early days. However, coated visual tactile sensors filter out color information when pressing fabrics. Although geometric information is indirectly extracted, color is equally important for fabrics. To this end, we compared the performance of coated sensors and sixth-generation visual tactile sensors on samples with the same texture but different colors. As shown in the figure below, the accuracy of coated sensors after integrating visual and tactile sensing is significantly improved, and both visual and tactile information can be obtained.
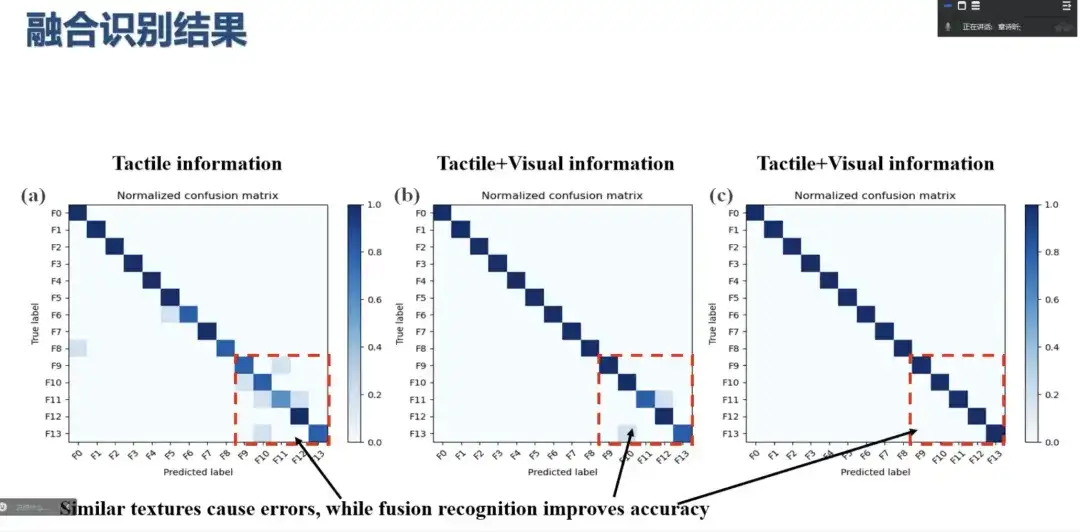
Fabric defect detection is crucial, and its defects will lead to a reduction in value by 45%-65%. Fabric dyeing patterns will seriously affect the versatility and complexity of the algorithm. As one of the important perceptual abilities of humans, touch is not affected by the surface color of objects and can assist vision to help us perceive objects. Therefore, we introduced touch into fabric defect detection and used 9 fabric samples for cross-validation. The results showed that the recognition accuracy in the tactile mode was higher than that in the visual mode, especially for the recognition of some special textures.
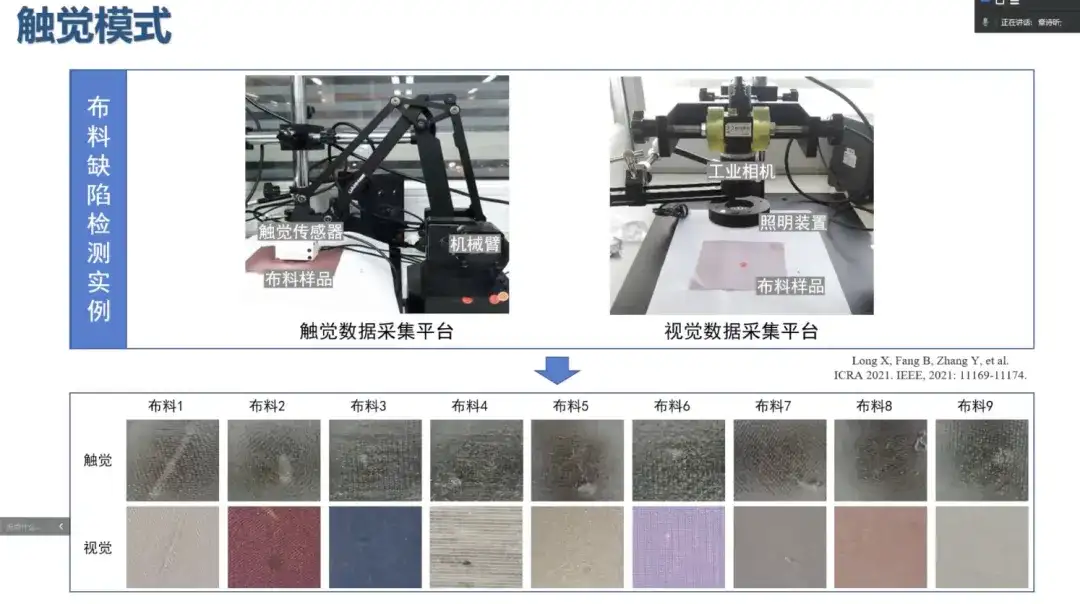
Since the texture information of the fabric is dominant and affects the determination of defective parts, we introduced an attention mechanism to focus on key defective areas and enhance the recognition accuracy of defective parts.
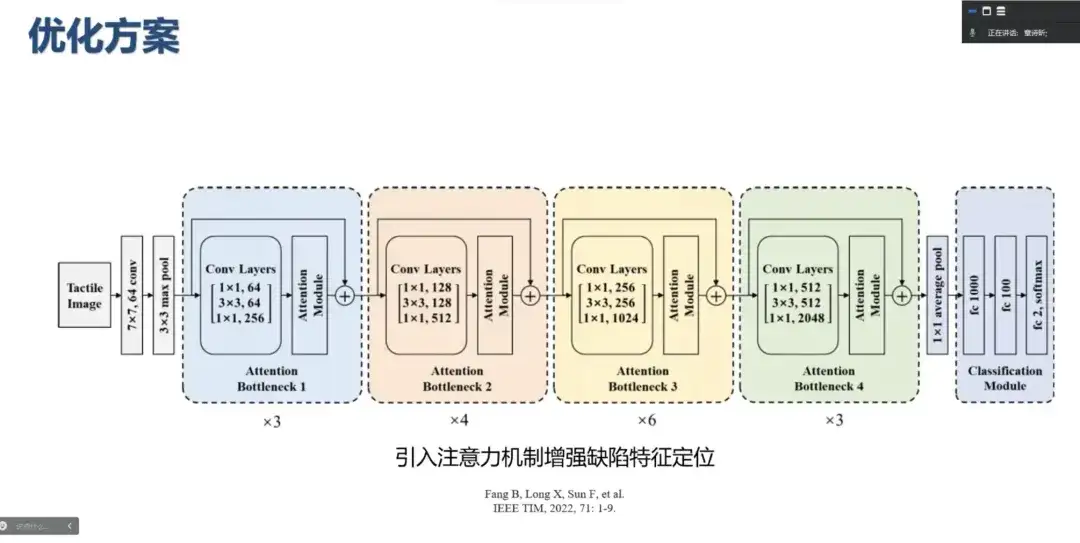
Transparent object recognition: glass recognition accuracy reaches 99% or above
In addition to fabrics, texture recognition of transparent objects is quite difficult. Due to their transparent properties and the influence of reflected light, there will be misrecognition in visual situations. Therefore, we introduce a tactile mechanism, collect tactile data, and establish a tactile platform. In the collected glass cups, considering the influence of their own temperature properties, we adjust the temperature by adding hot water or ice cubes, collect tactile images, and process them into the required tactile form.
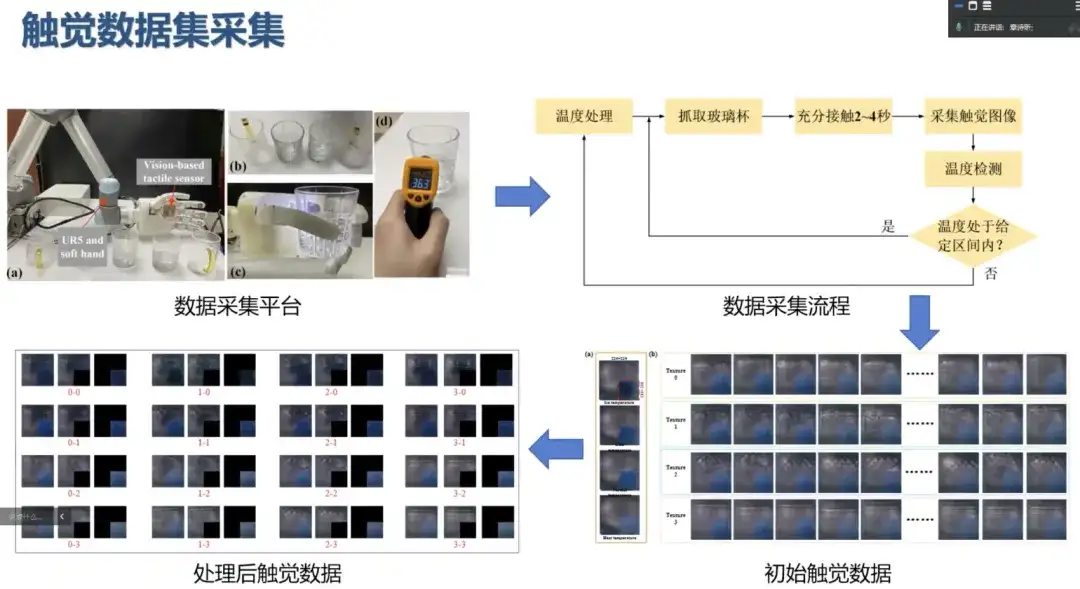
As mentioned earlier, tactile recognition usually includes temperature and texture information. Separately identifying these two features and fusing different single models to enhance the extraction and separation of the two features is a common recognition method, but it may result in irregular fusion.
Therefore, we proposed a hierarchical regional feature extraction mechanism. This mechanism ensures that the regional distribution of the temperature layer and the temperature layer is distributed according to a specific size, matches this specific size with the convolution size, and realizes independent feature extraction in each region during the convolution sliding process, which can avoid mixed fusion extraction. In addition, while extracting regional features, each modal region in the subsequent feature map will form a modal mapping with the initial region, and combined with vectorization processing, it can realize distributed parallel processing of unrelated modal features.
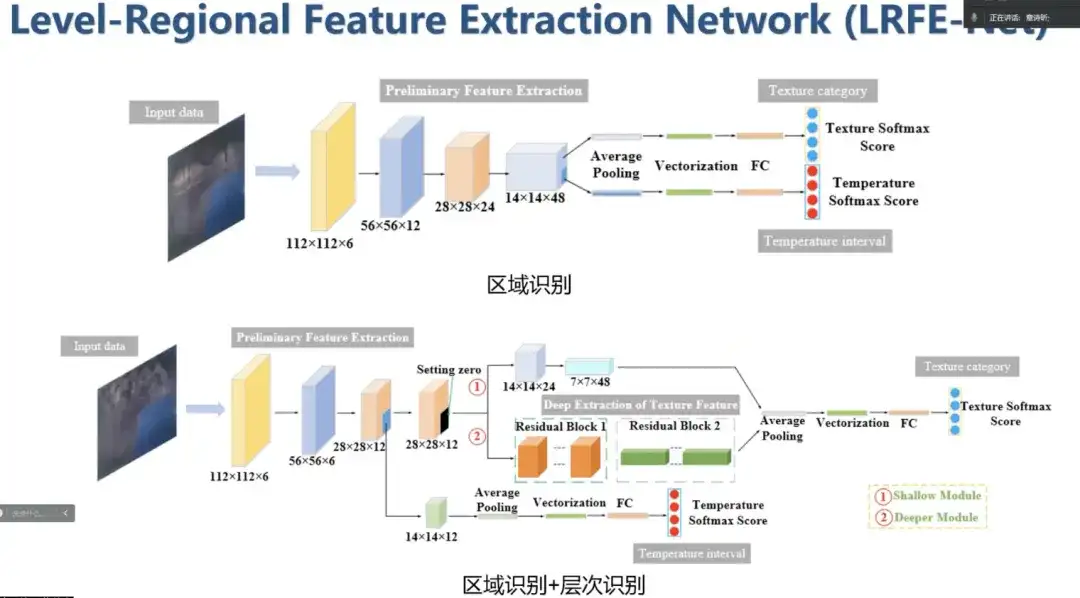
Considering the different learning difficulties of texture features and temperature features, we also integrated a hierarchical recognition mechanism to assign different deep network modules to deepen information processing. This hierarchical mechanism can effectively learn complex features.
As shown in the figure below, the traditional single model fails to effectively process texture and temperature information. Therefore, in individual recognition, the temperature recognition accuracy is low. In overall recognition, because the texture recognition of the image is dominant, the model forcibly couples and maps irrelevant features, and the temperature recognition is improved. However, our mechanism (LRFE-Net) maintains the same continuous accuracy in overall recognition and individual recognition. In addition, compared with multi-model recognition, our method has also improved time efficiency.
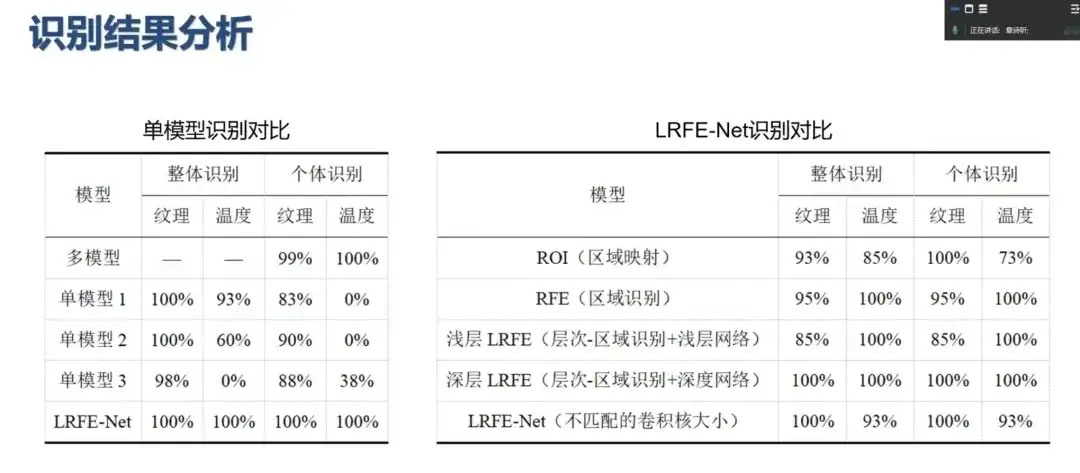
In summary, we obtain the appearance attributes of transparent objects in the visual mode and their texture or temperature attributes in the tactile mode. Next, we can use decision fusion to fuse the attributes obtained from these two modes and work together on transparent object recognition. For example, our method has achieved a visual and tactile recognition accuracy of more than 99% for glass cups.
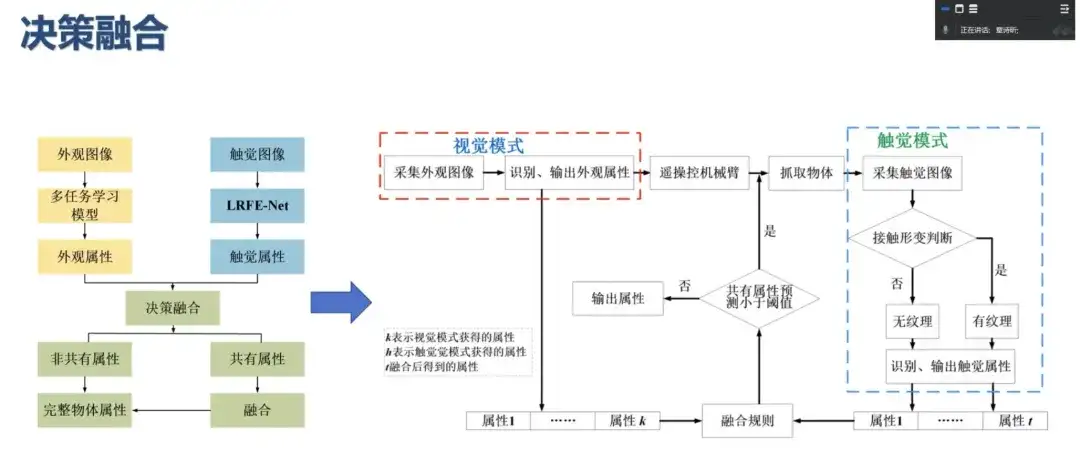
Agricultural applications: fruit quality testing
In the agricultural field, we have also extended visual-tactile sensing to fruit quality testing, using deformation differences to assess the softness and hardness of fruits and the degree of local decay.
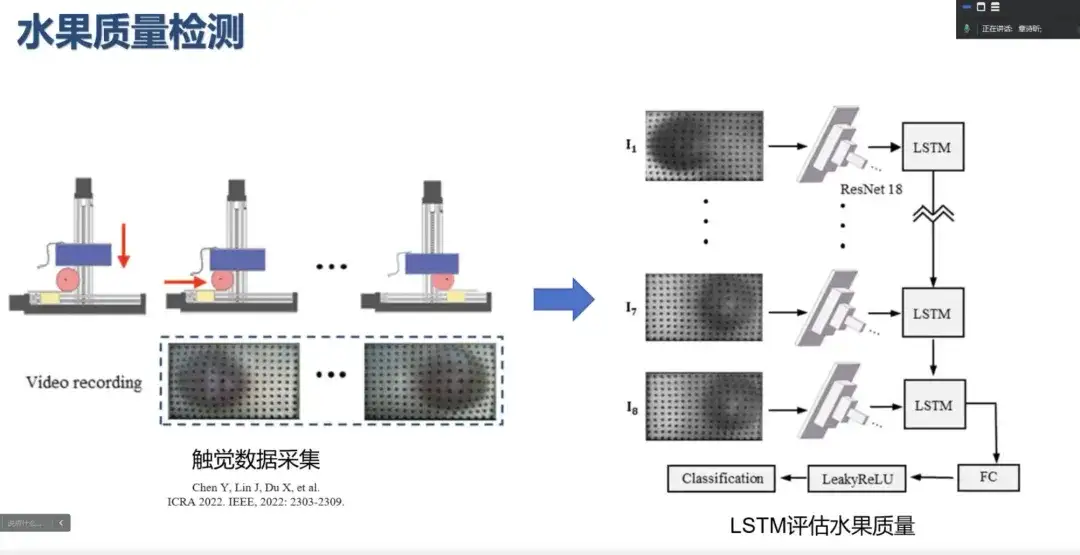
When pressure is applied, objects of different hardness will produce different deformations. Similarly, there is a relationship between the mark and the force. To fully express this tactile contact mechanism may require a very large data set and a complex model. However, in actual operation tasks, it is not necessary to accurately measure the force value. Understanding the deformation trend of the contact point is sufficient to meet the needs of certain tasks. Therefore, we propose a two-stage adaptive grasping strategy, as shown in the figure below. The strategy is mainly divided into two stages:
* Pre-grasping stage: Using tactile exploration to make the visual tactile sensor and the object reach a stable micro-contact state, which can build a preliminary understanding of the object's properties
* Stability adjustment stage: This stage determines the grasping stability based on the dynamic detection of micro-deformation, so that the visual tactile sensor and the object can reach a dynamic relative stability state, which can strengthen the cognition of the object's properties.
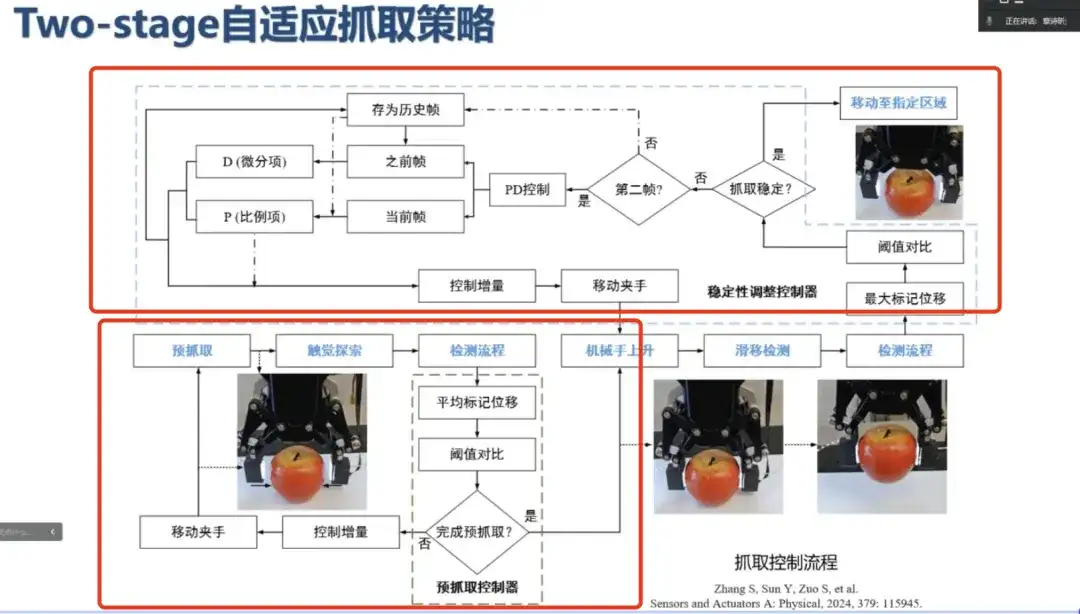
This strategy can adapt to different grasping needs, such as grasping apples, eggs, sponges, cans, etc. As shown in the figure below, in the pre-grasping stage of the egg, the manipulator moves slowly to establish a preliminary recognition of the object's attributes. Then, in the stability adjustment stage, due to the light weight of the egg, the system only needs slight adjustments to complete the grasping operation without much intervention.
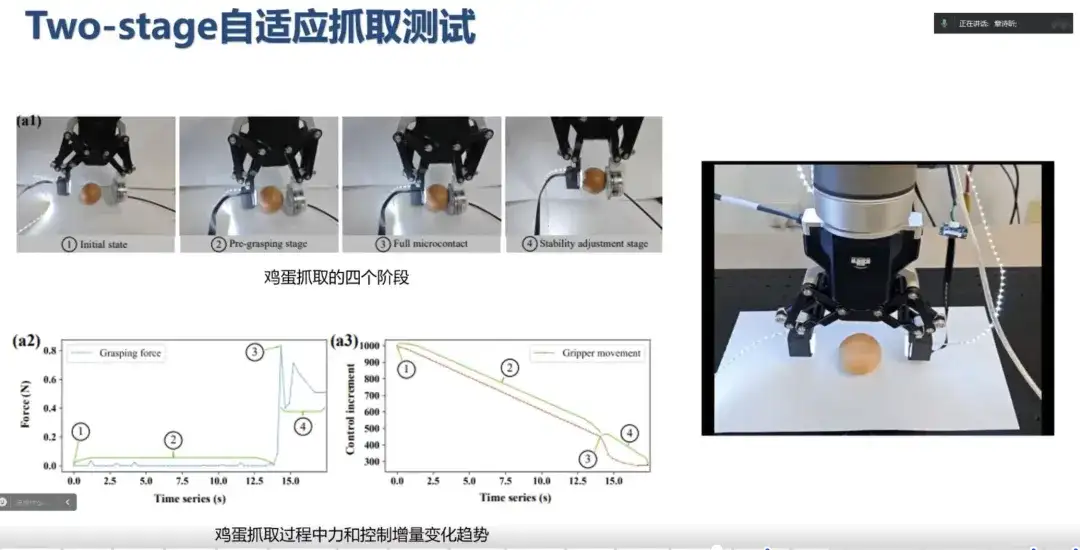
For materials such as sponges, a relatively stable contact state can be formed in the pre-grasping stage, so the system does not need to be adjusted further. In contrast, cans, as heavy objects, cannot reach a sufficiently stable state during the initial grasping. In the stability adjustment stage, the cans tend to slip, and the PD controller will fine-tune them until the stability requirements are met, and the entire adjustment process ends.
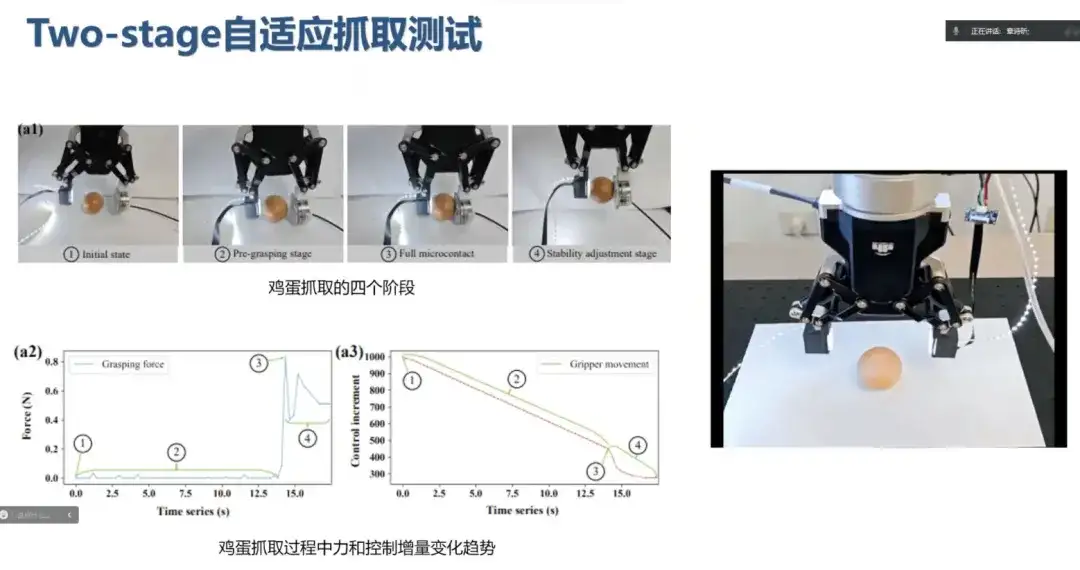
As shown in the figure below, the grasping tests of different objects show that our proposed two-stage adaptive grasping strategy is highly robust and can achieve stable and reliable grasping operations on various multi-attribute objects.
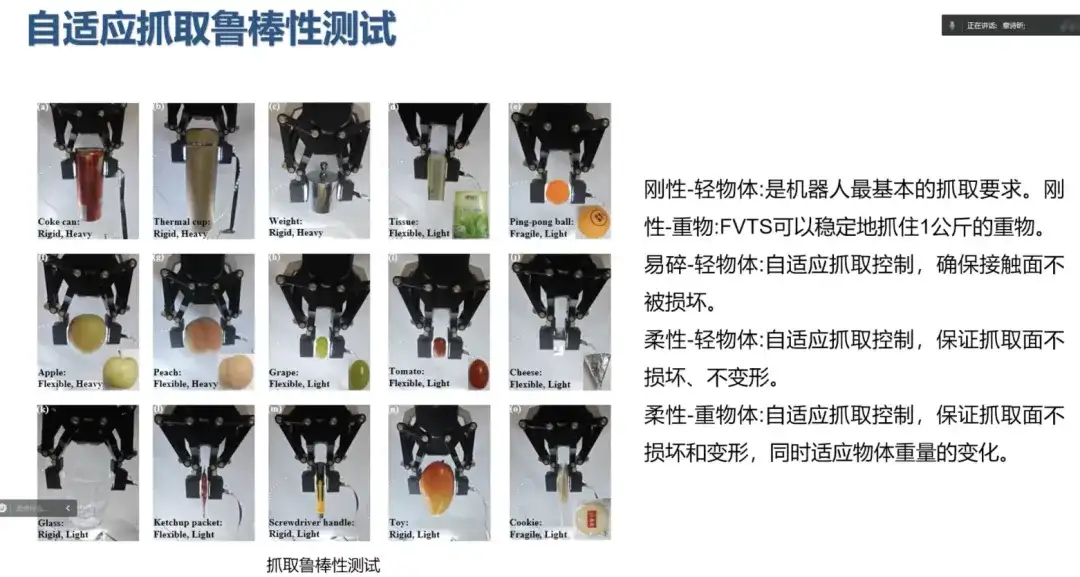
In the future, we will continue to explore the integration of touch and dexterous manipulation, and strive to achieve anthropomorphic and precise manipulation of robots.
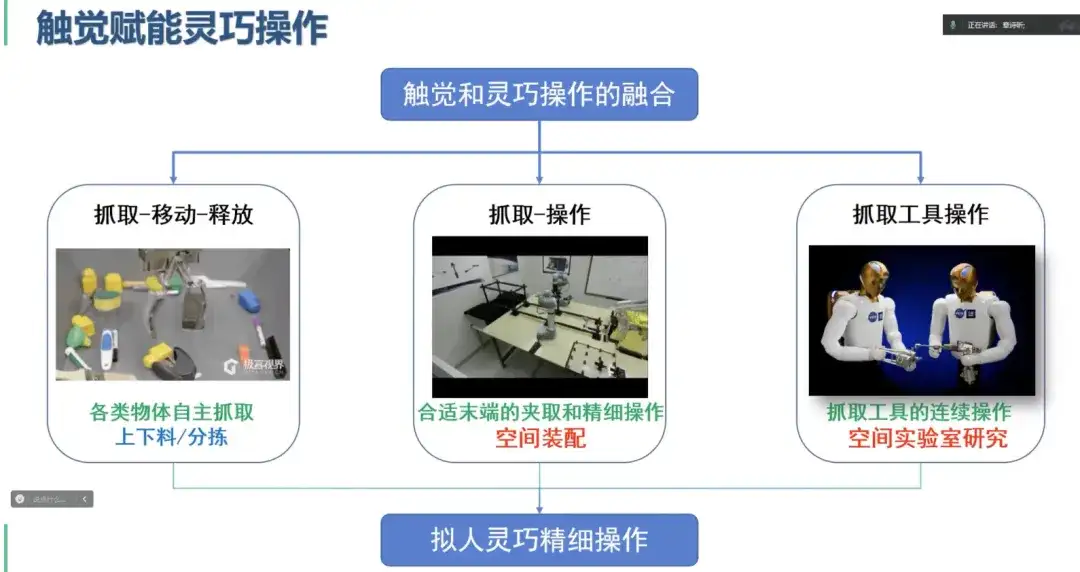
In the future, HyperAI will also assist the embodied touch community to continue to hold online sharing activities, inviting experts and scholars from home and abroad to share cutting-edge results and insights. Stay tuned!