DeepMind and Google Research Work Together to Create a "hexagonal Warrior" for AI Weather Forecasting
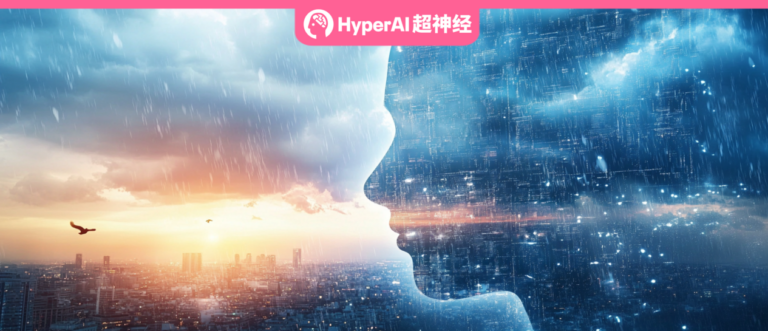
On December 10, Nature announced the top ten people of 2024. Although the gold content is not as impressive as the Nobel Prize, this top academic journal with a history of more than 100 years can be said to be a witness to scientific progress, so these ten people also represent the important scientific events of this year to a certain extent.Google DeepMind researcher Rémi Lam is on the list.
Nature reported that:Lam has been a pioneer in using machine learning to improve weather forecasting.The field has advanced by leaps and bounds over the past few years, and Lam and his team have been at the forefront, even as companies such as Microsoft, Nvidia, Huawei, and the European Centre for Medium-Range Weather Forecasts (ECMWF) are racing to develop AI-assisted weather forecasting systems. “But for most of this year, the leader in accuracy has been a project led by Lam called GraphCast.”
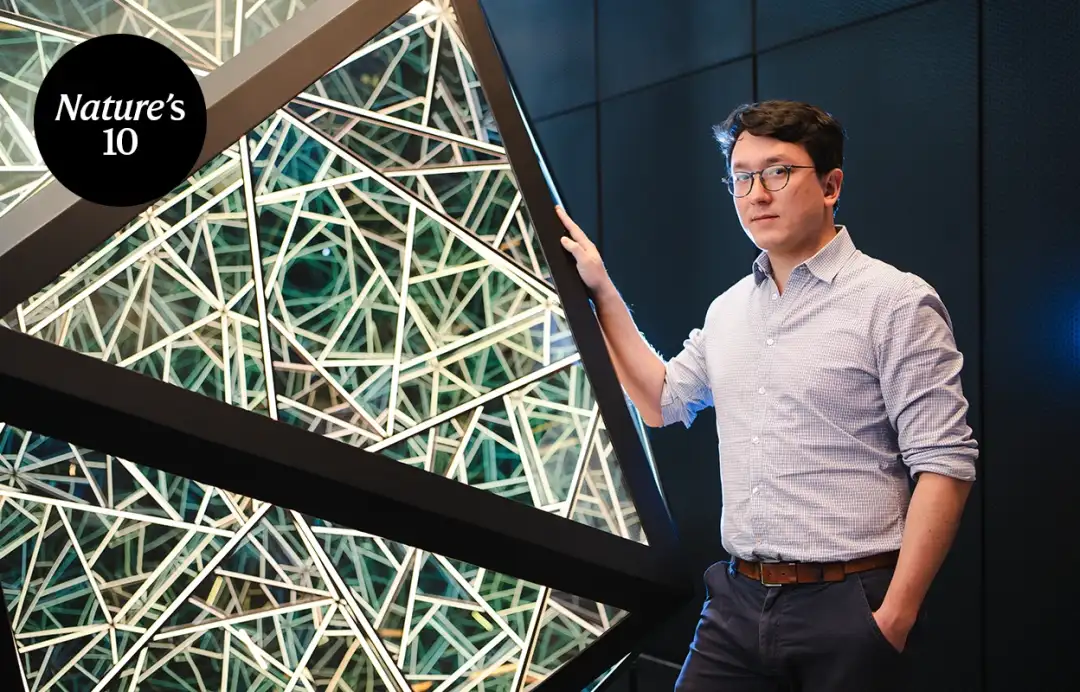
It is worth noting that shortly before the list was announced, Lam's team proposed a new weather forecast model, GenCast, which set a new record.A random set of 15-day global forecasts can be generated in 8 minutes.Covers more than 80 surface and atmospheric variables.
Some readers may wonder why DeepMind or Google did not choose AlphaFold's development route for weather forecasting, that is, to continuously iterate the same model and create a "phenomenal" product? The author boldly speculates that on the one hand, this is closely related to the forecast cycle. Short-term, medium-term, and long-term weather forecasts are applicable to different scenarios and have different accuracy requirements, so it is difficult to be consistent. On the other hand, it is also related to the current technical route exploration in the field of weather forecasting - whether AI will dominate the world, or whether traditional numerical forecasts and AI forecasts will coexist.
Taking into account short-term/medium-term/long-term forecasts, integrating traditional methods with AI
Short-term forecast,Usually refers to the weather conditions in the next 1-3 days, providing relatively detailed weather change information, such as temperature, precipitation, wind speed and direction, etc., mainly to provide guidance for people's daily life and to take timely precautions in case of heavy rain;Medium-term forecast,It refers to the weather forecast for the next 3-10 days. The trend forecast has a wider range and is mainly used for agricultural planning, reservoir scheduling, flood/drought warning, and logistics supply chain planning.Long-term forecast,It refers to the weather forecast for more than 10 days in the future, providing changing trends and climate patterns, which can be used for planning large-scale projects such as infrastructure, as well as for public health departments to take precautions in advance against medical resource pressures caused by extreme weather such as cold waves and high temperatures.
Focusing on the technical level,Short-term forecasts require higher temporal resolution and refined models, such as time series models based on deep learning. Medium-term forecasts need to consider the dynamic processes of the atmosphere and ocean over a longer time frame. Long-term forecasts tend to be based on pattern recognition and statistics rather than high-resolution numerical simulations.
Generally speaking, it is difficult for a single model to simultaneously meet the complexity of short-term, medium-term, and long-term forecasts. For example, short-term forecasts require high resolution, while long-term forecasts require wide coverage; short-term weather forecasts are more accurate, while long-term weather forecasts have larger errors…These differences require the model to make trade-offs between different tasks, making model optimization more difficult.
Therefore, most of the current research results are targeted, striving for high accuracy and short time consumption in single tasks. Google also adopted this strategy initially.
In 2023, Lam was in charge of GraphCast, which was launched by DeepMind. It is an autoregressive model based on graph neural networks for medium-term weather forecasting. It can be trained directly from reanalysis data and predict hundreds of weather variables for the next 10 days around the world at a resolution of 0.25° within 1 minute. Among the 1,380 validation targets, GraphCast significantly outperformed the most accurate deterministic business system on 90% targets.
In terms of prediction speed, a single Google TPU v4 is needed to complete a 10-day weather forecast in 60 seconds.
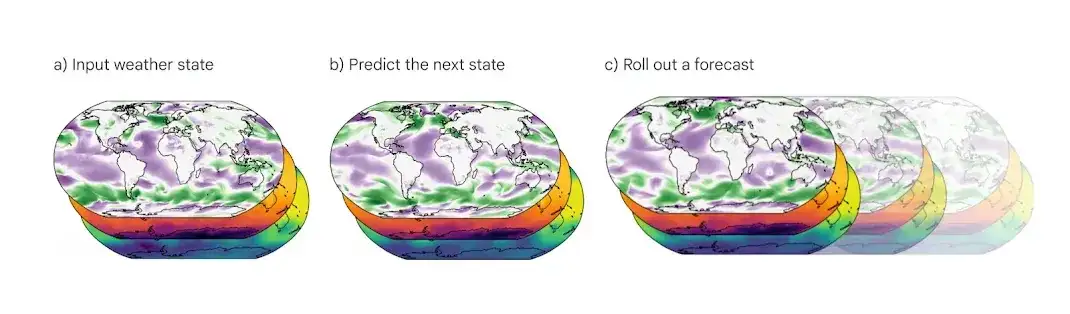
Simply input the weather conditions 6 hours ago and the current weather conditions, and GraphCast will be able to predict the weather conditions for the next 6 hours. This process can be rolled forward in 6-hour units to provide the latest forecast up to 10 days in advance.
In 2024, Google Research released NeuralGCM, a long-term weather forecasting tool whose name clearly shows the research team's choice of technology route. Neural is a neural network, and GCM stands for General Circulation Models, a physics-based weather simulator.NeuralGCM combines a differentiable solver for atmospheric dynamics with machine learning.The combination of traditional physics methods and AI is precisely the general trend in the current field of weather forecasting research.
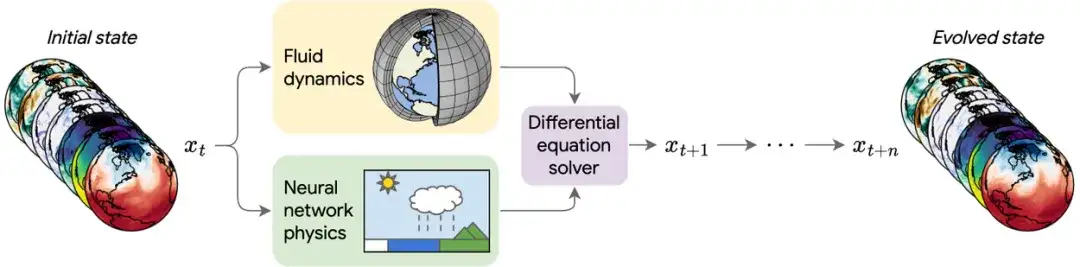
Before AI revolutionized meteorological research, numerical forecasting had been the mainstream technology in this field for nearly a hundred years. It is based on the principles of physics and meteorological theory, and predicts the changing trend of atmospheric conditions by solving the fluid dynamics and thermodynamic equations that describe atmospheric motion. After the emergence of technologies such as machine learning, there is no shortage of discussions in the industry: Will AI completely replace numerical forecasting?
Now it seems that the combination of the two may be the best solution. Taking NeuralGCM as an example, while relying on traditional computing to simulate large-scale physical processes, AI technology can also handle small-scale phenomena (such as cloud formation and regional microclimate) and correct the accumulated errors on a small scale.
Based on this, NeuralGCM has achieved a breakthrough in performance. From 1-15 days weather forecast to 10-year climate forecast, its accuracy is comparable to the best models of its kind at various time scales.
Specifically, its deterministic model NeuralCGM-0.7° at a resolution of 0.7° outperforms pure machine learning models in terms of weather forecast accuracy; in 1-15 day forecasts, it performs on par with the European Centre for Medium-Range Weather Forecasts (ECMWF). At the same time, in terms of long-term climate forecasts, NeuralGCM's 40-year climate forecast simulation results are consistent with the global warming trend shown by ECMWF data.
DeepMind's new achievements in weather forecasting
On December 4, DeepMind published a research paper titled "Probabilistic weather forecasting with machine learning" in Nature.A new machine learning weather forecasting method GenCast is proposed.Rémi Lam is one of the corresponding authors. This method has added another piece of the puzzle to Google's weather forecasting, making it more complete in terms of technical route and prediction accuracy.
Paper address:
https://www.nature.com/articles/s41586-024-08252-9
In terms of technical route,Although both are machine learning methods, GenCast is different from GraphCast in that it is based on a graph neural network. Instead, it is implemented based on a diffusion model and uses a Transformer to complete the denoising process. At the same time, in terms of prediction accuracy, GenCast has significantly improved spatial resolution compared to NeuralGCM, and can more clearly describe small-scale weather phenomena.
Specifically, GenCast is trained on decades of reanalysis data.A 15-day global weather forecast containing a random collection can be generated in 8 minutes.Output is generated every 12 hours with a spatial resolution of 0.25° (latitude-longitude) and covers more than 80 surface and atmospheric variables. The researchers evaluated 1,320 forecast targets and found that GenCast outperformed ENS (Ensemble Numerical Weather Prediction System) on 97.2% targets, especially in extreme weather, tropical cyclone path prediction and wind power generation prediction.
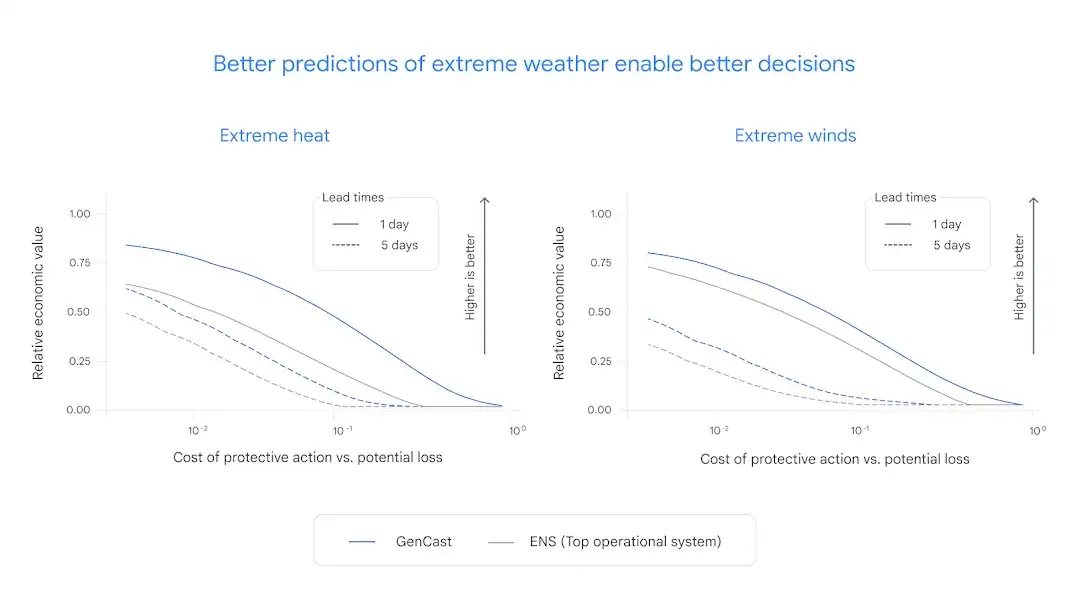
In summary, DeepMind and Google Research have worked together to explore the topic of weather forecasting, achieving more comprehensive coverage while exploring technical routes - including deterministic medium- and short-term weather forecasts, as well as long-term climate forecasts; pure machine learning methods with excellent performance, and "hexagonal warriors" that integrate numerical forecasting and AI.
A hundred schools of thought contend, building a weather forecast model based on pain points
Admittedly, Google is not the only company dominating the field of weather forecasting. Huawei, Microsoft, Nvidia, and others have all released related results. Although they are not as "wide-ranging" as Google, they all have their own strengths.
For example, Huawei's Pangu weather forecast model is the first AI method with a forecast accuracy that exceeds that of traditional numerical forecasting models, and its forecast speed can be increased by more than 10,000 times.A 3D Earth-Specific Transformer is proposed.A hierarchical time-domain aggregation strategy is used to reduce the number of forecast iterations and thus reduce the iteration error.
Microsoft's first large-scale atmospheric base model, Aurora,It is capable of operating at a high spatial resolution of 0.1°, thus capturing the intricate details of atmospheric motion.High-resolution weather forecasts for 10 days can be achieved in less than 1 minute.
NVIDIA has even pioneered a full-stack open climate digital twin cloud platform, EARTH-2, which integrates all the results of climate scientists for sharing and use by scientists around the world.
There are countless achievements in the academic world.FengWu-GHR, the first AI meteorological model to improve forecast resolution to 0.09°,"Fuxi" which can be used for sub-seasonal climate forecasts, etc.
There is no doubt that the current weather forecast research is booming with a trend of a hundred schools of thought, and multiple technical routes are running in parallel, while improving the spatial resolution, and satisfying the medium- and short-term deterministic forecasts and climate forecasts as comprehensively as possible. In the future, with the continuous iteration of AI technology, whether the mainstream technology that dominates the world will emerge is still unknown, but in the short term, the combination of AI and traditional numerical forecasting is still a better solution, and how to break through the spatial resolution on this basis still needs to be explored.
References:
1.https://www.nature.com/articles/d41586-024-03898-x
2.https://mp.weixin.qq.com/s/boOoF782fm1s65hCQ-smpg
3.https://mp.weixin.qq.com/s/0krO