4 Major Pain Points, 5 Studies, 7 Companies, One Article Explains AI-led Battery R&D Innovation
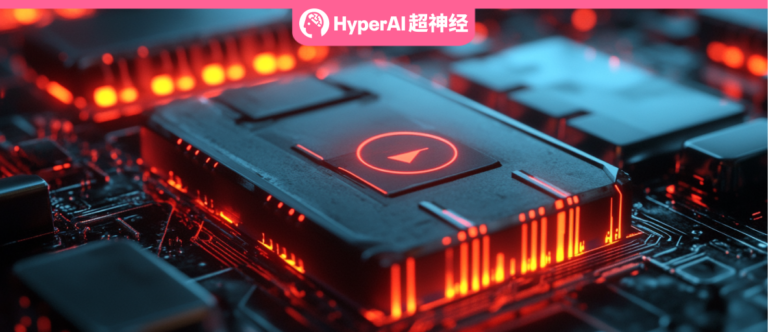
"In July, the domestic retail penetration rate of new energy vehicles reached 51.1%, 11 years ahead of schedule."This is the data released by the Passenger Car Market Information Joint Branch of the China Automobile Dealers Association in August this year. While highlighting the strong growth momentum of the new energy vehicle market, it is also an important milestone in the transformation of the energy structure in this field.
The reason why new energy vehicles have been able to occupy the consumer market so quickly, in addition to policy-driven and increased market acceptance, is mainly due to two key factors.On the one hand, the fierce price war initiated by Tesla has led to a wave of price cuts in the industry, and an endless stream of preferential policies have continued to stimulate sales; on the other hand, the battery technology equipped in new energy vehicles has been continuously innovated, effectively alleviating consumers' mileage anxiety about long charging times and uneven distribution of charging stations, further boosting consumers' purchasing confidence.
As the "heart" of new energy vehicles, power batteries are crucial to improving vehicle performance, cost and user experience.For example, the launch of BYD's blade battery not only greatly improves the space utilization and safety of the battery pack, but also has the potential to extend the range of pure electric vehicles to over 1,000 kilometers, making it the top endurance performance. Therefore, one netizen commented vividly: "Half the life of new energy vehicle companies is given by power batteries." This comment not only reveals the core position of batteries in the new energy vehicle industry chain, but also further illustrates the importance of technological innovation to market competitiveness.
It is worth mentioning that the importance of battery technology is not limited to the field of new energy vehicles. Whether it is consumer electronics or large-scale energy storage systems, batteries are an indispensable key technical support.The "Strategic Research on Building a New Material Powerhouse by 2035" released by the Chinese Academy of Engineering clearly lists battery materials as a key development direction in the field of new energy materials.This not only demonstrates the strategic position of battery technology in the future energy structure, but also provides important guidance for the comprehensive upgrading of the new energy industry.
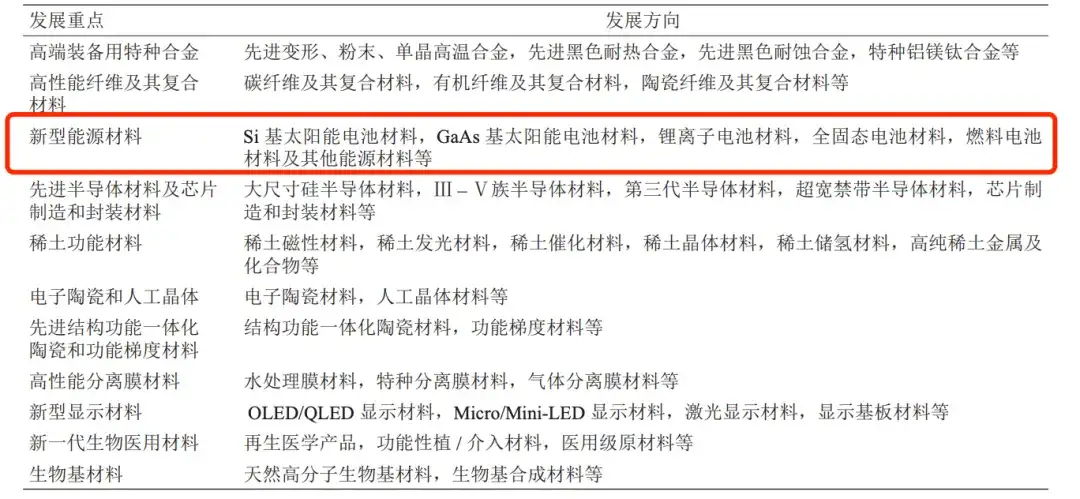
At the same time, the rapid development of artificial intelligence has injected new impetus into the research and development of highly difficult new batteries.Ouyang Minggao, an academician of the Chinese Academy of Sciences and a professor at Tsinghua University, said at the 2024 Science and Intelligence Summit held recently:"In terms of battery materials, all material research and development used to be trial-and-error, which consumed a lot of manpower, had a long cycle, and was inefficient. Now with artificial intelligence, we can change the previous research and development paradigm. Currently, we have achieved full-process automatic material design, such as automated experiments, characterization, simulation, and preparation, and achieved full-process intelligence, which has greatly improved the research and development efficiency of high-difficulty new batteries."
After years of hard work, the pain points and difficulties of traditional battery research and development
Battery R&D is a complex and systematic project, including battery material screening, synthesis preparation, characterization testing and process optimization.Traditional battery R&D mainly adopts the method of "trial and error". The entire R&D cycle spans several years and requires a large amount of capital investment. In this process, each stage faces unique pain points and difficulties.
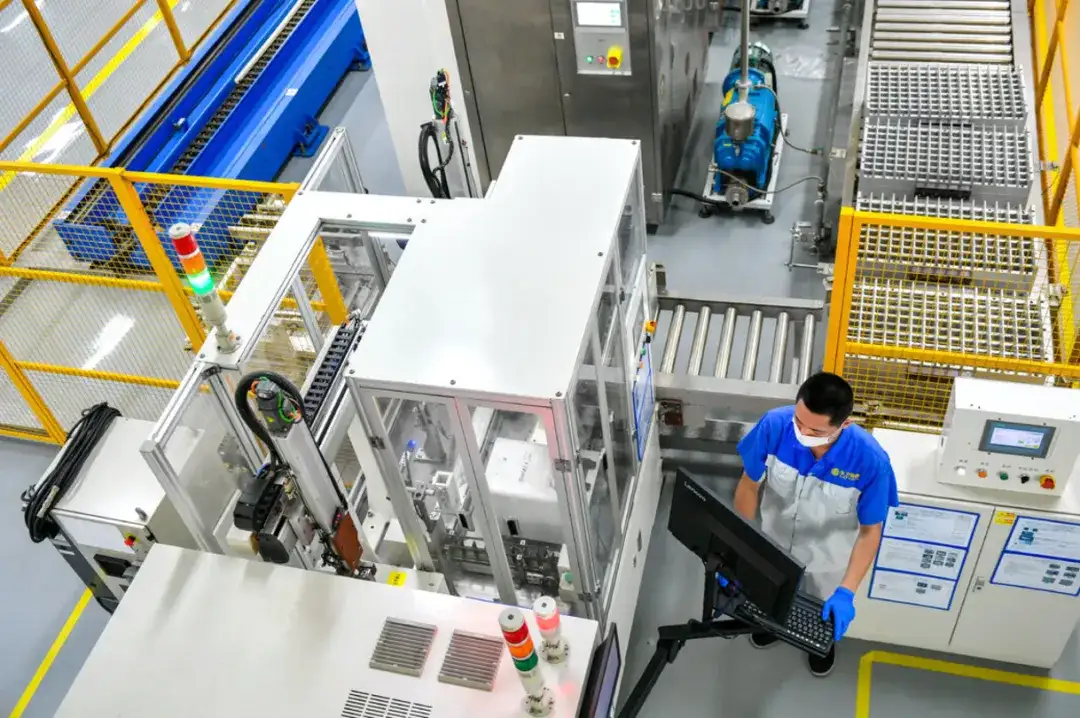
Specifically,In the process of battery material screening,Researchers need to find the best electrode material and electrolyte formula based on experimental feasibility, cost-effectiveness, and safety. However, there are many potential materials to choose from for battery components such as the positive electrode, negative electrode, electrolyte, and separator. Traditional screening methods rely on experiments to verify them one by one, which consumes a lot of time and resources and has high trial and error costs.
During the battery synthesis process,Researchers need to precisely control the reaction conditions of the synthesis to obtain the ideal material properties. These reaction conditions include temperature, pressure, time, environment, etc. For example, solid-state batteries still face major challenges in synthesis, such as the poor air stability of sulfides and the chemical and electrochemical stability of the electrode/electrolyte interface. These challenges limit their large-scale application in solid-state batteries and place higher requirements on the synthesis and preparation process.
Paper address:
https://wulixb.iphy.ac.cn/article/doi/10.7498/aps.69.20201581
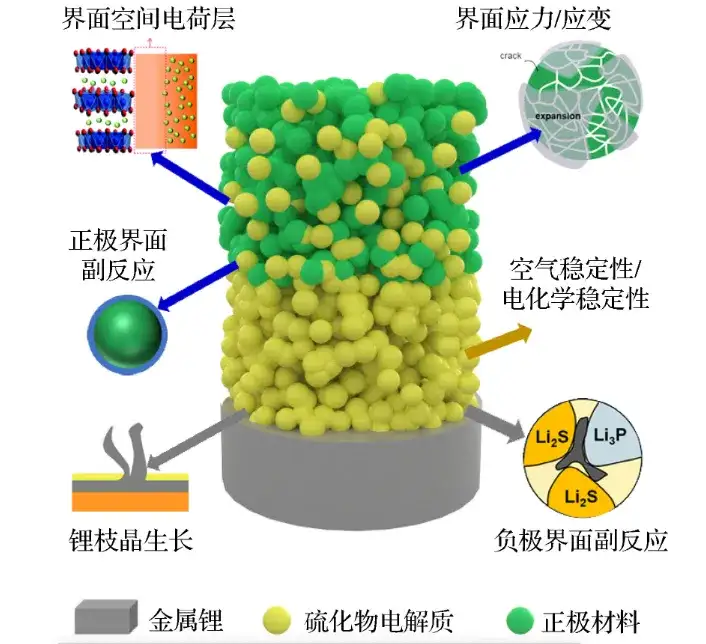
During the characterization test,Researchers need to test and analyze key properties of battery materials, such as crystal structure, electrochemical performance, and thermal stability. However, core indicators of battery performance (such as cycle life and energy density) usually require long-term testing to evaluate, and this testing cycle significantly delays the progress of research and development.
During the process optimization phase,The optimization of multiple parameters such as coating, drying, and compaction is a highly complex multivariable problem. The ideal performance obtained in small-scale laboratory research and development is often difficult to reproduce in industrial production.
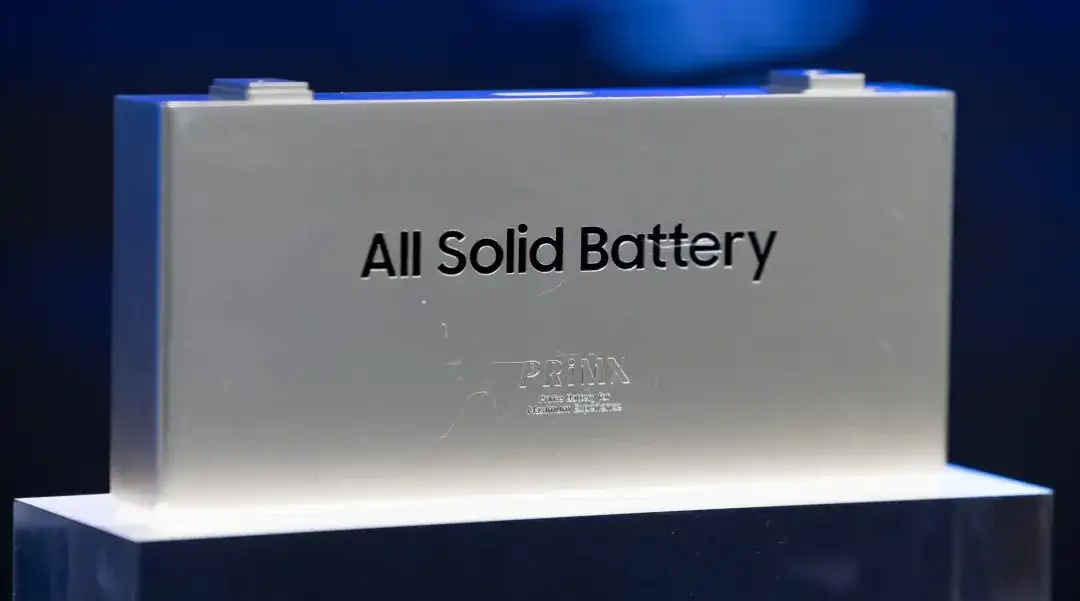
In summary, in traditional battery R&D methods, from material screening to process optimization, each stage faces complex challenges. Future battery R&D needs to introduce more data-driven design methods, high-throughput synthesis and testing technologies, and intelligent manufacturing methods to fundamentally break the bottleneck of traditional R&D. In this process, AI will play a vital role.
AI for Science unlocks new ideas for battery R&D
Although the development of new battery technologies represented by solid-state batteries still faces many challenges, with the development of the AI for Science (AI4S) paradigm, more and more universities and research institutes have begun to explore the implementation of AI-related technologies around battery research and development.
Specifically,First, AI can accelerate the screening and discovery of battery materials.The research and development of battery materials involves tens of thousands of chemical combinations, but experimental verification has limited time and resources. The application of AI in high-throughput computing and machine learning enables researchers to quickly screen potential high-performance materials through simulation and prediction. For example, Microsoft and PNNL used AI technology to screen 32 million potential battery materials and narrowed the list to 23 in 80 hours, 5 of which were known materials. The team said that if traditional methods were used to obtain these materials, the process would take more than 20 years.
The related research was published on the preprint website arXiv under the title "Accelerating computational materials discovery with artificial intelligence and cloud high-performance computing: from large-scale screening to experimental validation".
Paper address:
https://arxiv.org/pdf/2401.04070
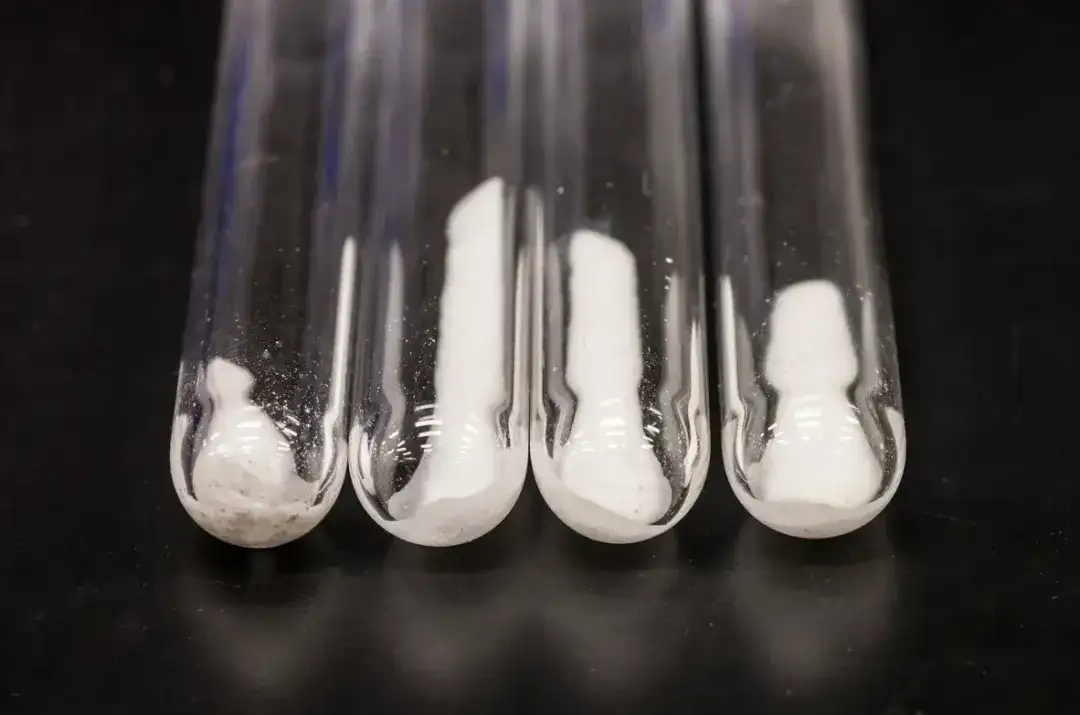
Secondly, AI also performs well in the synthesis and preparation process of batteries.Specifically, interface issues are the key bottleneck of battery performance. For example, the interface stability between the lithium metal anode and the electrolyte directly determines the safety and life of the battery. Traditional experiments make it difficult to fully understand the complex reactions at the interface, while AI models can combine molecular dynamics simulations and experimental data to predict interface reaction paths and design better electrolyte materials. For example, researchers at South China University of Technology used AI models to model the interface reactions of lithium-ion batteries, focusing on optimizing battery components, and providing direction for the development of more stable electrolyte materials.
The related research was published in the Journal of Materials Chemistry under the title “Insights into the interface reaction between electrolyte and Li(2)MnO(3) from ab initio molecular dynamics simulations”.
Paper address:
https://pubs.rsc.org/en/content/articlelanding/2024/ta/d4ta04598j
During battery characterization testing, AI also performed well in predicting battery life.For example, researchers from MIT, Stanford University, and Toyota Research Institute (TRI) use AI to predict battery life. The AI algorithm developed by the team can determine the battery life based on the battery's five charge and discharge cycles, with an accuracy rate of up to 95%, and the error between the predicted value and the actual battery life value is within 9%. It is worth mentioning that this dataset has been open sourced and is the largest of its kind.
The related research was published in Nature under the title "Data-driven prediction of battery cycle life before capacity degradation".
Paper address:
https://www.nature.com/articles/s41560-019-0356-8
Not long ago, the Dalian Institute of Chemical Physics of the Chinese Academy of Sciences and Xi'an Jiaotong University made new progress in the field of battery health management. The researchers developed a new deep learning model that effectively solved the traditional method's reliance on a large amount of charging test data, provided new ideas for real-time battery life estimation, and realized end-to-end evaluation of lithium battery life. At the same time, the model is also an important part of the core model of the first-generation battery digital brain PBSRD Digit, providing a solution for intelligent battery management.
The related research was published in IEEE Transactions on Transportation Electrification under the title “Deep learning powered lifetime prediction for lithium-ion batteries based on small amounts of charging cycles”.
Paper address:
https://ieeexplore.ieee.org/document/10613834
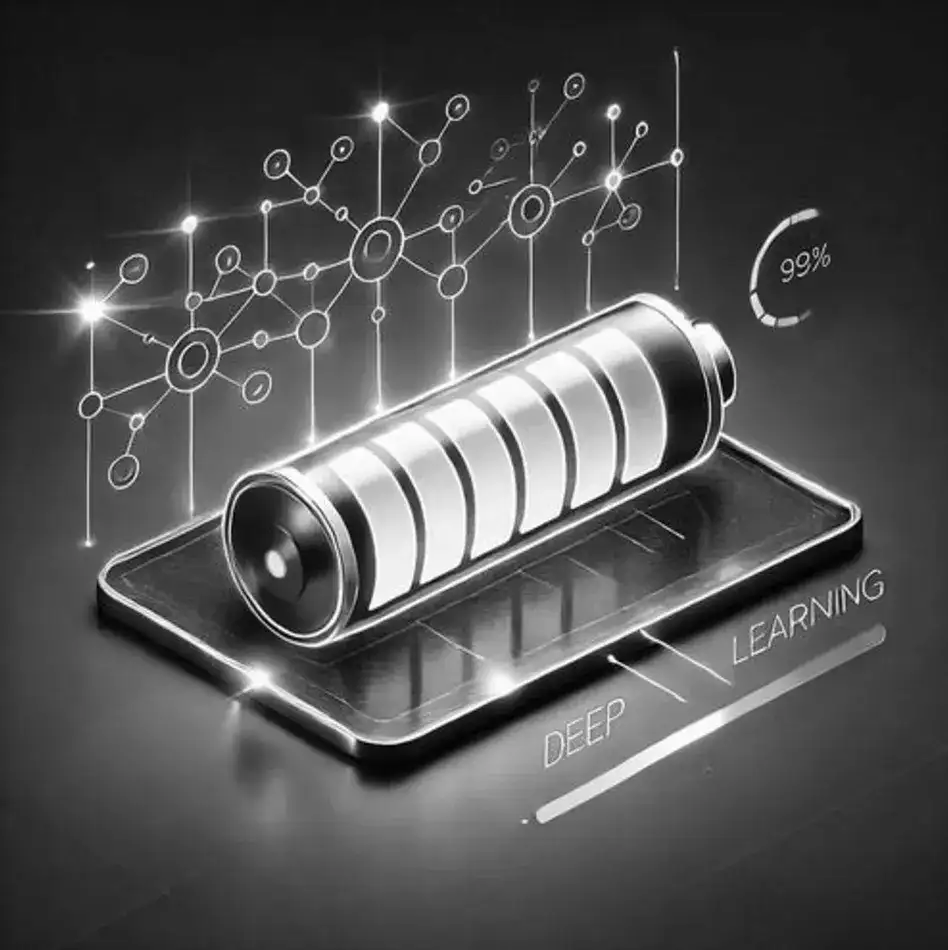
In addition, AI also shows great potential in optimizing the production process of battery materials.Taking solid-state batteries as an example, their manufacturing has strict requirements on the microstructure of the electrolyte. AI technology can analyze parameters in the material preparation process, such as temperature and pressure, through computer vision and optimization algorithms, thereby improving production consistency and reducing manufacturing costs. For example, a study by Jules Verne University of Picardy in France and other institutions demonstrated how to monitor and optimize the electrode manufacturing process through machine learning technology. This method can adjust battery manufacturing parameters in real time, thereby significantly reducing waste and improving product consistency.
The related research was published in Science under the title "Toward High-Performance Energy and Power Battery Cells with Machine Learning-based Optimization of Electrode Manufacturing".
Paper address:
https://www.sciencedirect.com/science/article/pii/S037877532301050
It is foreseeable that, driven by the AI for Science paradigm, the field of battery materials is on the threshold of a new technological revolution. The application of AI not only brings new ideas and tools to the research and development of battery materials, but is also reshaping the development path of the entire battery technology.
AI accelerates the pace of industrialization of new batteries
The battery industry is at the top of a wave of technological innovation, and AI is undoubtedly the core driving force leading this technological renaissance. The in-depth application of AI technology has not only spawned cutting-edge theories of battery science in the field of academic research, but also demonstrated strong practical value in the industry, providing new impetus for the commercialization, large-scale production and performance optimization of battery technology.
In the international market, many companies have taken the lead in deploying AI-driven battery research and development.Tesla uses AI to optimize its battery management system (BMS), uses deep learning and machine learning techniques to predict battery health status and lifespan, and uses data-driven methods to improve supercharging and energy management.
South Korean battery manufacturer LG Energy Solution has developed an AI platform that focuses on predicting battery aging, failure modes, and energy management optimization, while providing dynamic prediction and optimization capabilities for energy storage systems (ESS).
Lithium metal battery company SES AI also announced that it will join forces with technology companies NVIDIA, Crusoe and Supermicro to accelerate the research and development of new battery materials. It plans to use high-performance supercomputers optimized for AI to draw a small molecule database, thereby enhancing the understanding of battery chemical systems and accelerating the development of energy storage solutions.
In addition, NVIDIA recently announced that the ALCHEMI NIM project is accelerating the development of sustainable energy materials such as electric vehicle batteries and solar panels through AI technology. These projects can efficiently simulate and predict the electrochemical properties of materials, which not only shortens the development cycle of new materials, but also significantly reduces costs, providing technical support for global energy transformation.
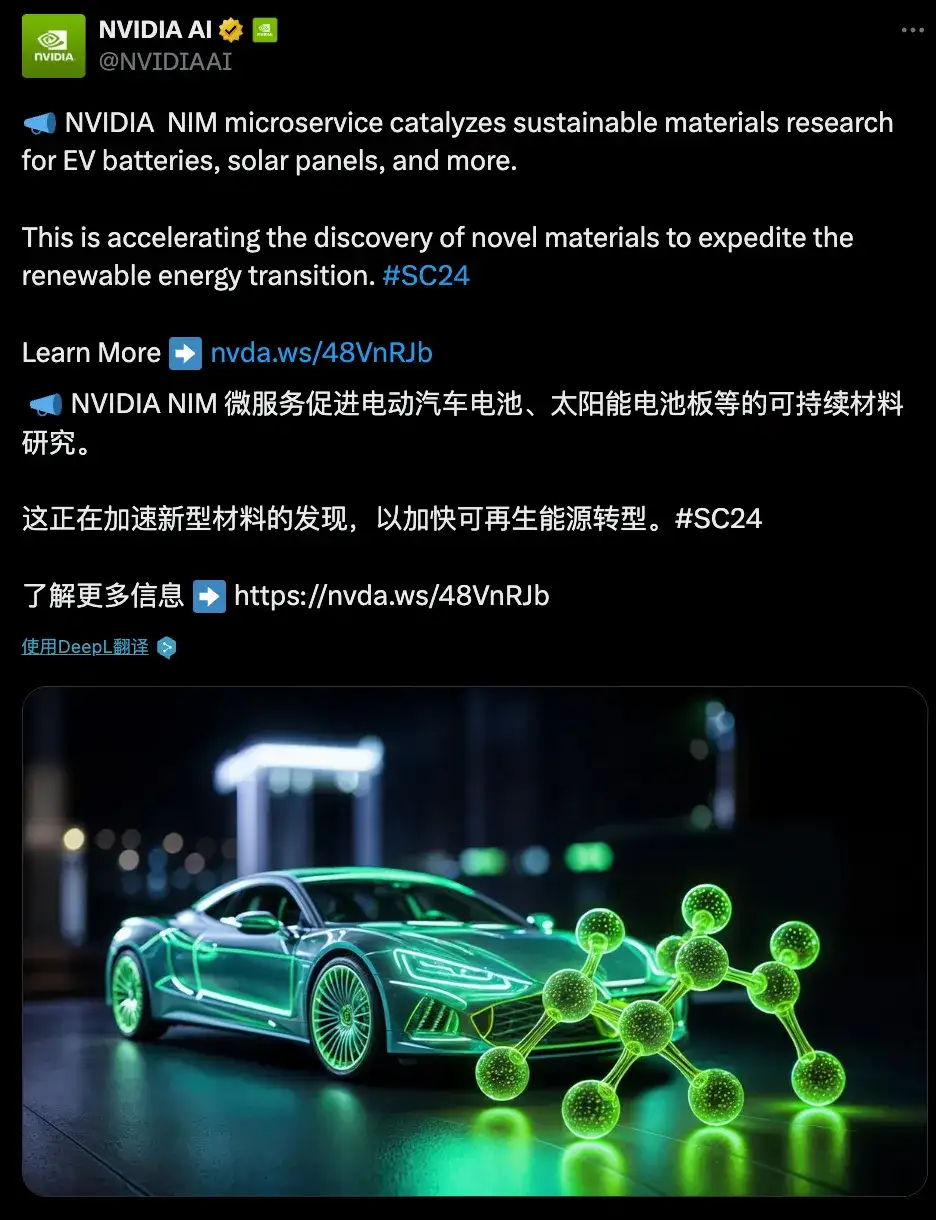
Returning to the domestic market, battery research and development and technological innovation among various companies are also showing a trend of a hundred schools of thought contending.As a leader in the global power battery industry, CATL actively applies AI technology to the modeling and optimization of battery chemistry and material performance, focusing on the research and development of high-energy-density batteries. In December 2023, CATL announced that it would set up an international R&D center in Hong Kong to focus on AI for Science. Zeng Yuqun, chairman of CATL, has also mentioned in public many times in the past year the acceleration of the introduction of AI, especially in the innovation of battery material systems.
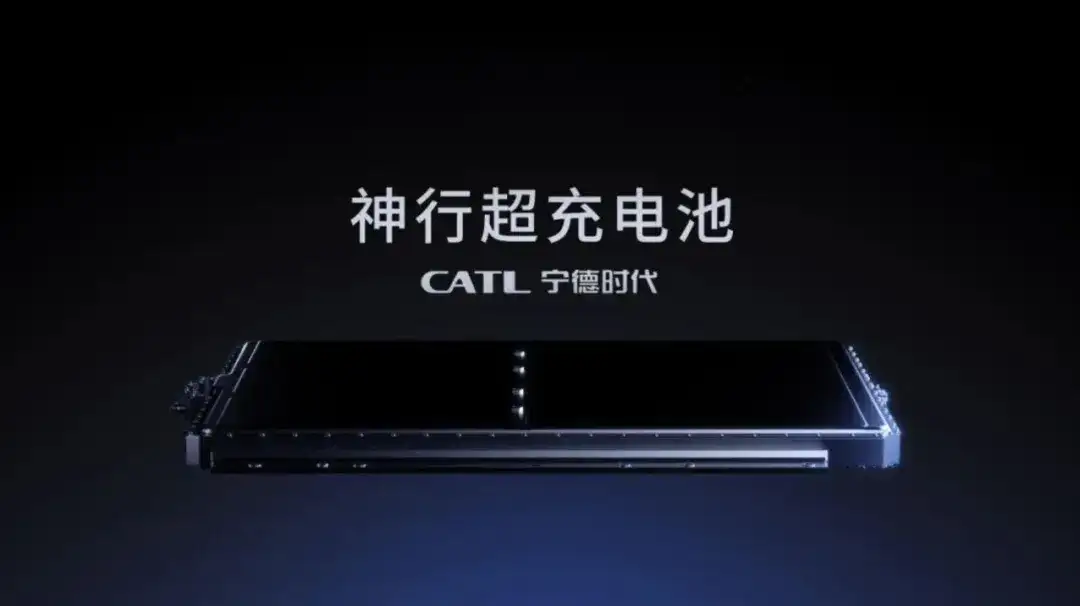
In addition, Honeycomb Energy (SVOLT) took the lead in building the industry's first automotive-grade AI smart power battery factory in Jintan, Jiangsu, using AI to manage the entire battery process and launched a series of high-performance battery products, greatly accelerating the large-scale application of new energy batteries.
At the same time, a number of AI battery material start-ups have sprung up in the foreign market, such as QuantumScape, Inobat Auto, Mitra Chem, Aionics, etc., aiming to introduce artificial intelligence into the field of battery development. Among them, Mitra Chem is described by some big names in the battery technology industry as "a battery material innovator driven by artificial intelligence technology located in Silicon Valley."
A number of new energy AI companies have also emerged in the Chinese market, such as Suntech Energy, a company incubated by the team of Academician Ouyang Minggao, which released the world's first battery AI large model PERB2.0. This model can process and analyze massive amounts of battery data, playing a key role in battery design, performance optimization and intelligent decision-making.
To sum up, whether it is the international market or domestic enterprises, whether it is leading enterprises or start-ups, they are actively embracing AI in the field of battery research and development.
Final Thoughts
At present, from material discovery to manufacturing optimization, from performance prediction to full life cycle management, AI technology is fully empowering every link of battery research and development, injecting strong momentum into the new energy industry. By deeply integrating scientific research results with industrial practice, AI not only accelerates technology iteration, but also promotes the large-scale application of battery technology and reduces costs.
However, the development of everything is a zigzag process, and the deep integration of AI and battery research and development cannot be achieved overnight.As Zeng Yuqun, chairman of CATL, said, "AI4S (for battery material research and development) currently does not have particularly good models, structures, or algorithms, and there is still a long way to go."
References:
1.http://finance.people.com.cn/n1/2024/0812/c1004-40297368.html
2.https://www.auto-made.com/news/show-16443.html
3.https://www.engineering.org.cn/sscae/CN/article/downloadArticleFile.do?attachType=PDF&id=28528
4.https://esst.cip.com.cn/CN/10.19799/j.cnki.2095-4239.2024.0698
5.http://www.xinhuanet.com/science/20241121/6c8a64232e464ee886b8dc4c732f81fd/c.html
6.https://developer.nvidia.com/zh-cn/blog/revolutionizing-ai-driven-material-discovery-using-nvidia-alchemi/
7.http://www.dicp.cas.cn/xwdt/mtcf/202410/t20241022_7405757.html
8.https://www.cas.cn/syky/202411/t20241120_5040077.shtml