Published in Science! Shanghai Jiao Tong University and Shanghai AI Lab Jointly Release a Protein Mutant Design Model That Outperforms the Most Advanced Methods
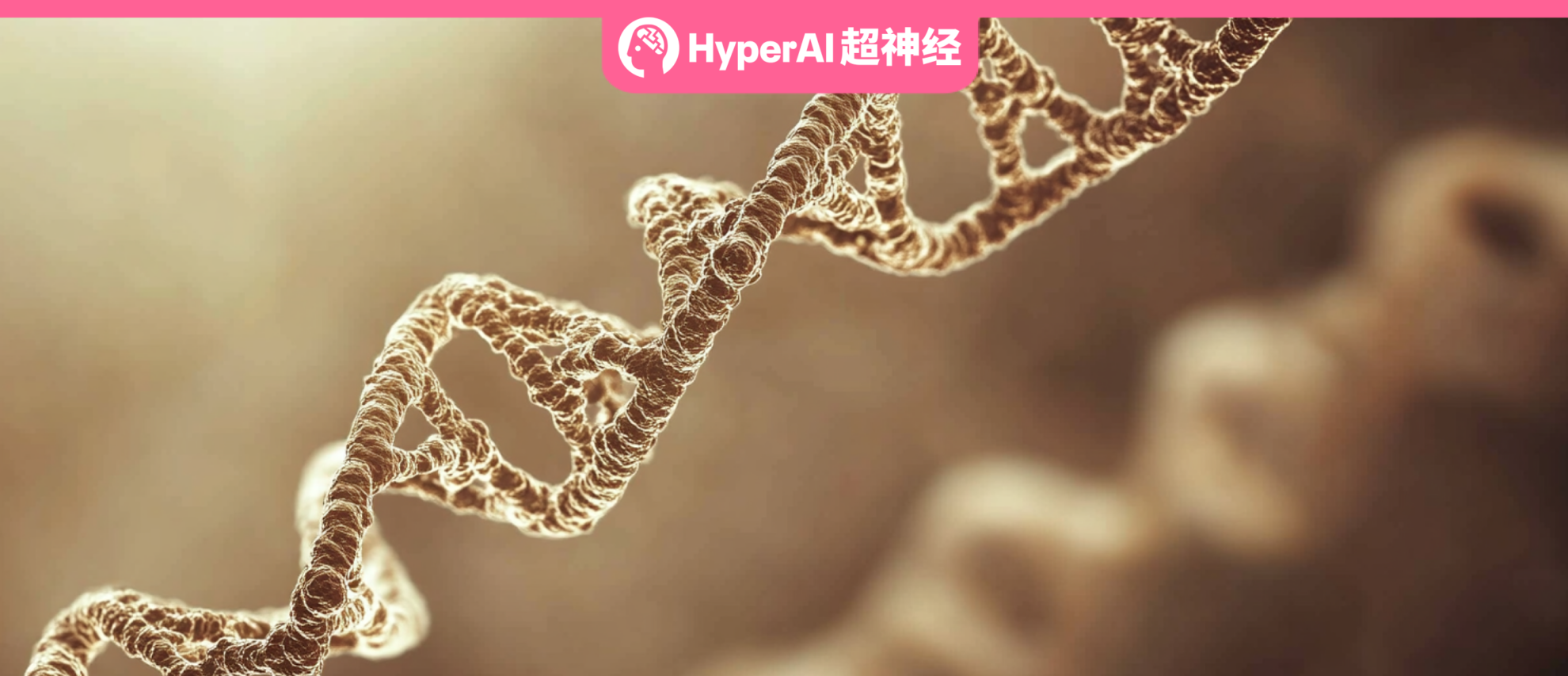
Protein is not only the executor of human life activities, but also plays an important role in many fields such as biomedicine, food processing, brewing, and chemical industries. Therefore, people have never stopped conducting research on protein structure and function to select proteins that meet the needs and have high stability for industrial application scenarios.
However, the physical and chemical conditions (such as temperature and pH value) required for the "wild-type" proteins extracted from organisms to function in industrial environments are mostly far from their native biological environment. In other words, the stability of this type of protein is difficult to adapt to the harsh industrial environment. Therefore, in order to meet the needs of different application scenarios,Mutations are often required to improve the physicochemical properties of proteins, thereby increasing their stability under extreme temperature/pH conditions or increasing enzyme activity and specificity.
It should be noted that changing the biological activity of a protein requires years of experimental research on its working mechanism, which is not only time-consuming and laborious, but also increasingly difficult to meet the rapidly changing needs of modification. In recent years, the emergence of protein language models has greatly improved the accuracy of protein fitness prediction, but it is still lacking in the accuracy of its stability prediction.
A truly meaningful protein mutation should improve stability while maintaining its biological activity, and vice versa. In response to this, Professor Hong Liang's research group at the School of Natural Sciences/School of Physics and Astronomy of Shanghai Jiao Tong University, together with Tan Pan, a young researcher at the Shanghai Artificial Intelligence Laboratory, and collaborators from ShanghaiTech University and the Chinese Academy of Sciences Hangzhou Medical College,They jointly developed a new protein sequence large language model pre-training method PRIME,At the same time, the best prediction results were achieved in protein mutation-activity and mutation-stability prediction, as well as other temperature-related representation learning.
The related research, titled "A General Temperature-Guided Language Model to Design Proteins of Enhanced Stability and Activity", has been published in Science Advances, a well-known journal under Science.
Research highlights:
* PRIME can predict the performance improvement of specific protein mutants without relying on prior experimental data
* PRIME can effectively predict multiple properties of a protein, allowing researchers to successfully design proteins in unfamiliar areas
* PRIME is trained based on a "temperature-aware" language model, which can better capture the temperature characteristics of protein sequences
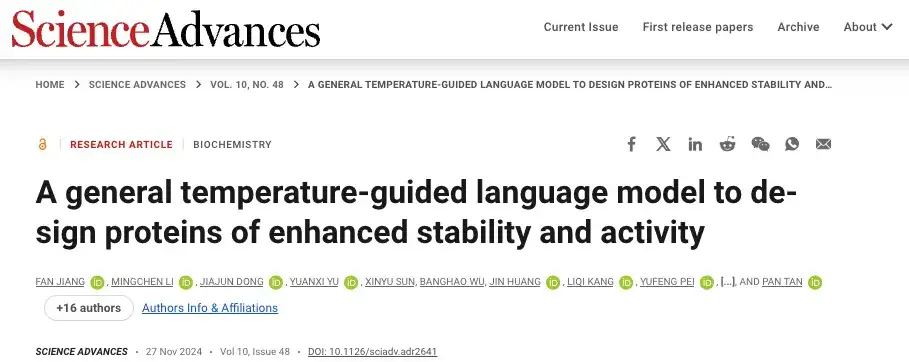
Paper address:
https://www.science.org/doi/10.1126/sciadv.adr2641
The open source project "awesome-ai4s" brings together more than 100 AI4S paper interpretations and provides massive data sets and tools:
https://github.com/hyperai/awesome-ai4s
Dataset: 96 million records, exploring the relationship between protein sequence and temperature
By integrating public data from Uniprot (Universal Protein Resource) and protein sequences obtained from environmental samples through metagenomics studies,Researchers have compiled a large database, ProteomeAtlas, containing 4.7 billion natural protein sequences.
* UniProt is a large database providing protein sequences and associated detailed annotations.
During the sequence screening process, the researchers retained only full-length sequences and processed these sequences using the biological sequence alignment tool MMseqs2, setting the sequence identity threshold to 50% to reduce redundancy, and then identifying and annotating sequences related to the optimal growth temperatures (OGT) of bacterial strains.
final,The researchers annotated 96 million protein sequences in this way.It provides a rich resource for exploring the relationship between protein sequence and temperature.
In addition, in the zero-shot predictive ability analysis of the model's thermal stability, the datasets used to study the melting temperature change (ΔTm) were derived from MPTherm, FireProtDB, and ProThermDB, and all experiments were performed under the same pH conditions.
Among them, MPTherm contains experimental data related to protein thermal stability; FireProtDB is specifically used to store mutation experimental data related to protein thermal stability and function; ProThermDB specifically collects data related to protein thermodynamic properties. At the same time, the researchers also combined deep mutation scanning (DMS) data, mainly from the protein mutation analysis database ProteinGym.
* ProteinGym protein mutation dataset
https://go.hyper.ai/YlMT5
Model architecture: Deep learning model based on "temperature perception"
The new deep learning model PRIME (Protein language model for Intelligent Masked pretraining and Environment prediction) proposed by the institute,Ability to predict performance improvements of specific protein mutants without relying on prior experimental data.
The model is trained based on a "temperature-aware" language model, relying on a dataset of 96 million protein sequences, combining the masked language modeling (MLM) task at the token level and the optimal growth temperature (OGT) prediction target at the sequence level, and introducing the correlation loss term through multi-task learning. It can screen out protein sequences with high temperature tolerance to optimize their stability and biological activity.
Specifically,PRIME consists of 3 main parts:As shown in the figure below. The first is the Encoder module, which is a Transformer encoder used to extract latent features of the sequence. The second is the MLM module, which is designed to help the encoder learn the contextual representation of amino acids. At the same time, the MLM module can also be used for mutant scoring. The third component is the OGT prediction module, which can predict the OGT of the organism in which the protein is located based on the latent representation.
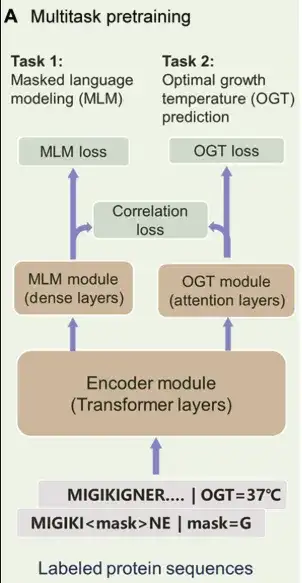
PRIME's multi-task learning in the pre-training stage includes MLM, OGT prediction and Correlation loss.
in,MLM is often used as a pre-training method for sequence data representation.In this study, noisy protein sequences were used as input, some labels were masked or represented by alternative labels, and the training goal was to reconstruct these noisy labels. This approach helps the model capture the dependencies between amino acids and the contextual information of the sequence, and can also use this reconstruction process to score mutations.
The second training task was optimized under supervised conditions, and the researchers used a dataset of 96 million protein sequences annotated with OGT to train the PRIME model. The input of this task is a protein sequence, and the temperature values generated by the OGT module range from 0° to 100°C. It is worth noting that the OGT module and the MLM module run using a shared encoder.This structure enables the model to simultaneously capture amino acid context information as well as temperature-dependent sequence features therein.
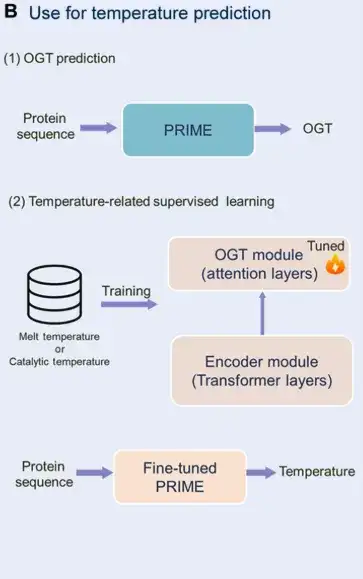
Finally, the researchers introduced Correlation loss to facilitate feedback from predicted OGT to MLM classification, aligning task information at the token and sequence levels.This enables the large model to better capture the temperature characteristics of protein sequences.
Experimental conclusion: Outperforms the most advanced methods in predicting the adaptability of mutant protein sequences
The researchers experimentally compared the zero-shot prediction capabilities of PRIME with those of the most advanced models for thermal stability, including the deep learning models ESM-1v, ESM-2, MSA-transformer, Tranception-EVE, CARP, MIF-ST, SaProt, Stability Oracle, and traditional computational methods GEMME and Rosetta.
The researchers used datasets from MPTherm, FireProtDB, and ProThermDB, which contained melting temperature changes (ΔTm) collected under the same pH environment, and ensured that each protein had at least 10 data points, for a total of 66 tests. At the same time, the study also included deep mutation scanning (DMS) detection methods, using ProteinGym as a test benchmark.
The results are shown in the figure below.PRIME outperforms all other methods in predicting both protein availability and stability.
In the ProteinGym benchmark (yellow in the figure below), PRIME scored 0.486, and the second-ranked SaProt scored 0.457. In the ΔTm dataset (dark purple in the figure below), PRIME still ranked first with a score of 0.437, and the second-ranked score was 0.412. In addition, the researchers also compared PRIME with other methods in the ProteinGym sub-dataset ProteinGym-stability (light purple in the figure below), and PRIME still outperformed all other methods.
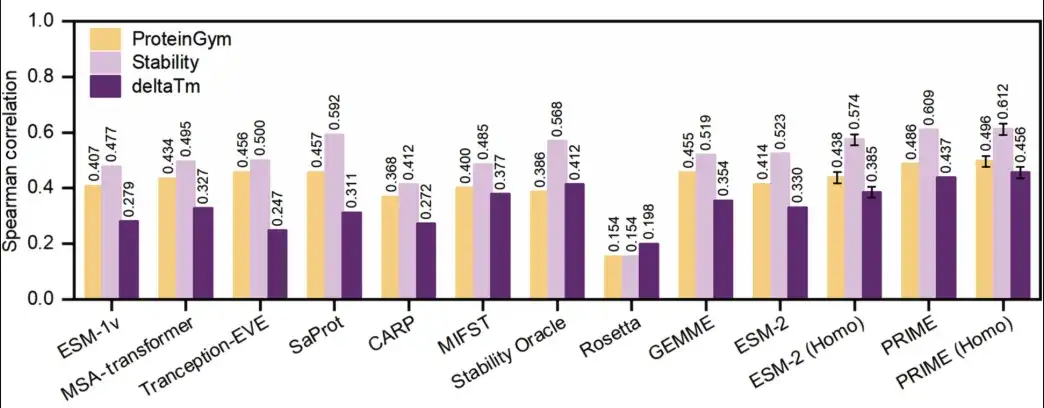
It is worth noting that in order to test the effectiveness and effect of PRIME in the practical application of protein engineering,The researchers also conducted a wet experiment and selected five proteins for verification.Includes LbCas12a, T7 RNA polymerase, creatinase, artificial nucleic acid polymerase, and the heavy chain variable region of a specific nanoantibody.
In the experimental testing of the top 30-45 single-point mutations, more than 30% of AI-recommended single-point mutants were significantly superior to wild-type proteins in key properties such as thermal stability, enzymatic activity, antigen-antibody binding affinity, non-natural nucleic acid polymerization ability, or tolerance under extreme alkaline conditions, and the positive rate of individual proteins exceeded 50%.
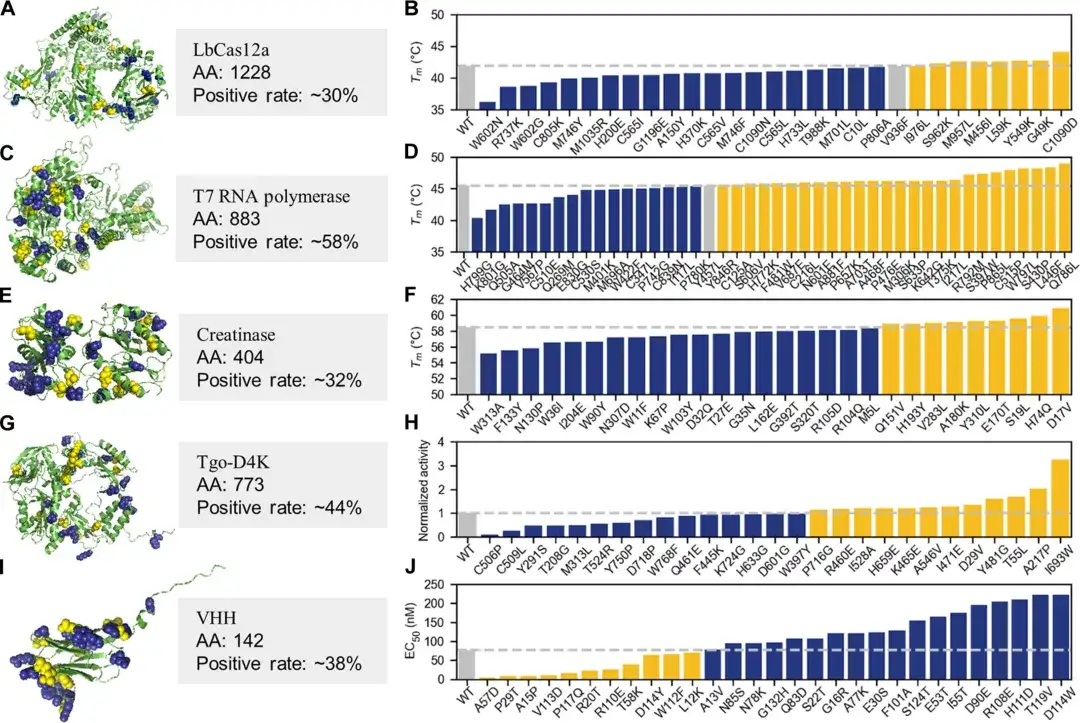
It is worth mentioning that the team also demonstrated an efficient method based on PRIME.Multi-site mutants with enhanced activity and stability can be rapidly obtained.Through this small sample fine-tuning method, very excellent protein mutants can be produced in 2-4 rounds of evolution with less than 100 wet experimental samples.
For example, after four rounds of dry-wet iterations, T7 RNA polymerase successfully obtained multi-point mutants with high activity and high stability. The highest multi-point mutant Tm was 12.8℃ higher than the wild type, and the activity was nearly 4 times that of the wild type. Some products outperformed similar products from the world's leading biotechnology company (New England Biolabs) that have dominated the market for 10 years. In addition, in experiments with LbCas12a and T7 RNA polymerase, Pro-PRIME can superimpose negative single-point mutations to obtain positive multi-point mutations.
This suggests that PRIME can learn the epistatic effects of protein mutations from sequence data, which is of great significance to traditional protein engineering.
Deepening protein engineering to overcome the problem of small samples
In the field of protein engineering, protein expression, purification and functional testing usually require expensive reagents and instruments, and the experiments are time-consuming, which greatly limits the number of samples that can be generated. In protein function research, testing the effects of protein mutations on functions (such as catalytic activity, thermal stability, binding affinity, etc.) requires more precise and complex experiments, and it is difficult to measure the performance of all possible mutations through one-time high-throughput.
This makes it difficult for machine learning models to obtain sufficient training on limited samples, resulting in poor performance of the model in predicting new mutations. In addition, experimental errors or noise in small sample data may cause greater interference to model training.The challenge of small sample data has, to some extent, limited the research efficiency and accuracy in the field of protein engineering.This has greatly encouraged researchers to explore innovative technologies, combining machine learning, experimental techniques, and multimodal data analysis to break through the limitations of small samples.
The research team described in this article has performed outstandingly in this regard. In addition to PRIME mentioned above, Professor Hong Liang's team and Dr. Tan Pan have also published a number of results on small sample learning.
Previously, the team used a combination of meta-transfer learning (MTL), learning to rank (LTR), and parameter-efficient fine-tuning (PEFT).We developed a training strategy, FSFP, that can effectively optimize protein language models when data is extremely scarce.It can be used for small-sample learning of protein adaptability. It greatly improves the effect of traditional protein pre-training large models in mutation-property prediction when using very little wet experimental data, and also shows great potential in practical applications.
The related research was titled "Enhancing efficiency of protein language models with minimal wet-lab data through few-shot learning" and published in Nature Communications, a subsidiary of Nature.
In addition, Professor Hong Liang has also shared relevant views. He believes that "in the next three years, in the fields of protein design, drug development, disease diagnosis, new target discovery, chemical synthesis pathway design, and material design, general artificial intelligence in professional fields will bring about a clear paradigm shift, transforming the scientific discovery model that relied on sporadic trial and error of the human brain in the past into an AI large-model automated standard design model."
Specific changes include building zero-sample or small-sample learning methods, and building pre-training technology models.In the absence of data, a large amount of fake data with slightly lower accuracy is generated through a physical simulator for pre-training, and then fine-tuned with real and valuable data to complete reinforcement learning.
Professor Hong emphasized that "fake data refers to data that is not from the real world, but has a certain degree of reliability. It can be generated by AI or obtained through physical computational simulation for data enhancement. Finally, real wet experimental data is the most valuable and is used for the final fine-tuning of the model."
Indeed, the challenge of data scarcity exists not only in the field of protein engineering. Small sample and even zero sample learning methods are crucial. We look forward to Professor Hong Liang’s team and Dr. Tan Pan bringing more high-quality results around this pain point.