Successfully Screened 4 Highly Active Hydrogen Evolution Catalysts! Chinese and American Research Groups Jointly Used Active Learning to Identify 14,000 High-entropy Oxides
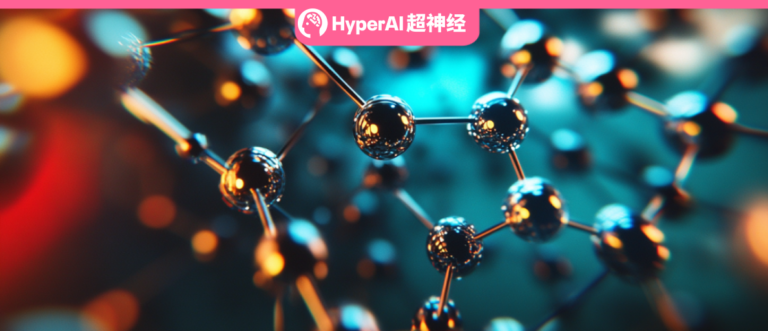
In recent years, high entropy materials (HEMs) have shown great potential in the fields of material design and functional control.High entropy oxides (HEOs) show broad application prospects in the field of chemical catalysis due to their abundant active sites, adjustable specific surface area, stable crystal structure, unique geometric compatibility and electronic structure.
Specifically, HEOs are composed of 5 or more principal elements in equal or nearly equal molar ratios. Traditional HEOs research and development requires the exploration of a vast composition space on the one hand, and relies on time-consuming and laborious laboratory trial and error on the other. Machine learning can efficiently explore the vast catalyst space and identify the best performing catalyst by capturing complex structure-performance relationships.
However, direct prediction of structure-property relationships remains challenging due to the limitations of databases and the subjectivity of sample selection by researchers.Active learning (AL), as an important tool for efficiently exploring chemical space, has been widely used in the fields of functional materials and drug development.
In this context,Wang Xun's team from the Department of Chemistry at Tsinghua University, Wu Liang from the Department of Chemistry at Shanghai Jiao Tong University, Chu Shengqi from the Institute of High Energy Physics at the Chinese Academy of Sciences, Lin Guang from the Department of Mathematics at Purdue University, and Xiang Yan from the Department of Bioengineering at Duke University,An active learning framework for discovering high-entropy spinel oxides (HESOs) is proposed and its efficiency in exploring the vast compositional space of HEOs with limited experimental data is demonstrated.
After multiple iterations, the researchers successfully identified the most promising HESOs in a wide range of chemical space.It exhibited excellent hydrogen production performance (251 μmol gcat⁻¹ min⁻¹) in the 300°C water-gas shift reaction and showed excellent stability in a test lasting 120 hours. The related research was published in the Journal of the American Chemical Society under the title "Active Learning Guided Discovery of High Entropy Oxides Featuring High H2‑production".
Research highlights:
* This study proposes an active learning (AL) strategy that operates as a closed-loop system, iterating through the “training, prediction, and experimentation” phases to efficiently explore the vast composition space of HEOs
* Through multiple active learning iterations, the researchers successfully screened out 4 new HEOs from a large number of potential combinations. These new materials showed excellent stability and demonstrated excellent hydrogen production performance in the water-gas shift reaction
* The CrMnCoNiCu catalyst was successfully prepared, indicating that the proposed method has excellent reproducibility and scalability potential
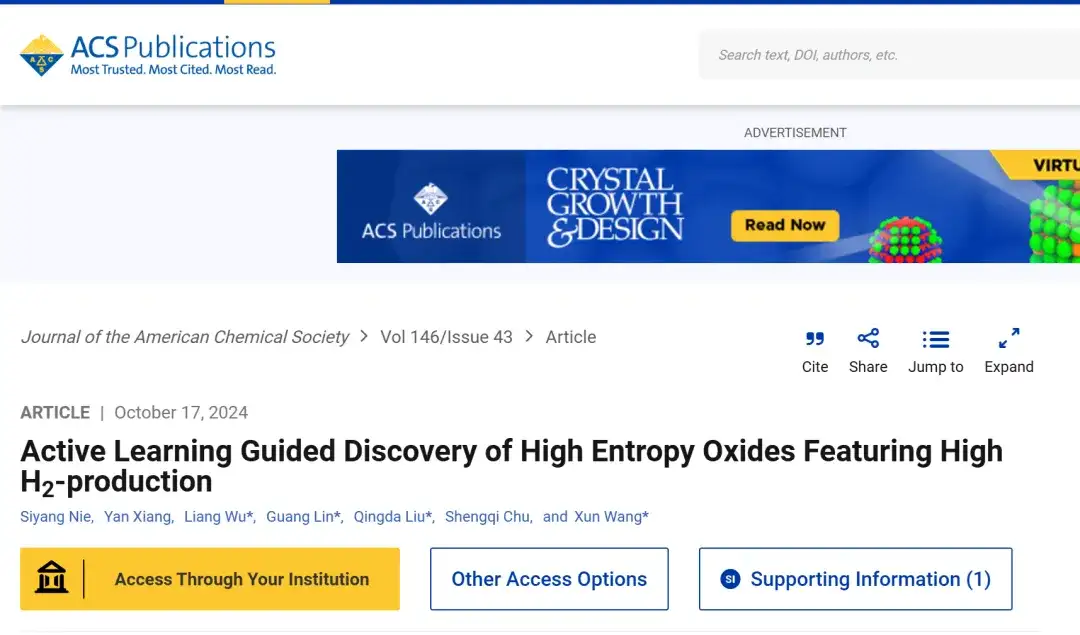
Paper address:
https://pubs.acs.org/doi/10.1021/jacs.4c06272
The open source project "awesome-ai4s" brings together more than 100 AI4S paper interpretations and provides massive data sets and tools:
https://github.com/hyperai/awesome-ai4s
Dataset: Diverse initial dataset
AL requires a certain initial training dataset to start the process, and when the dataset is limited, machine learning models can gain advantages from a diverse dataset.
In the initial stage of the study, the researchers constructed an additional library containing 14 transition metals. In theory, the tetrahedral coordination center and the octahedral coordination center can accommodate 5 to 10 metal elements, and are distributed in an equimolar ratio. Therefore, the total number of potential HESOs candidates is 14,443, which constitutes the catalyst space of the research target. The researchers used the Kennard-Stone algorithm to sample 305 data points from them. These samples were subsequently synthesized and their crystal phases were characterized, as shown in the following table:
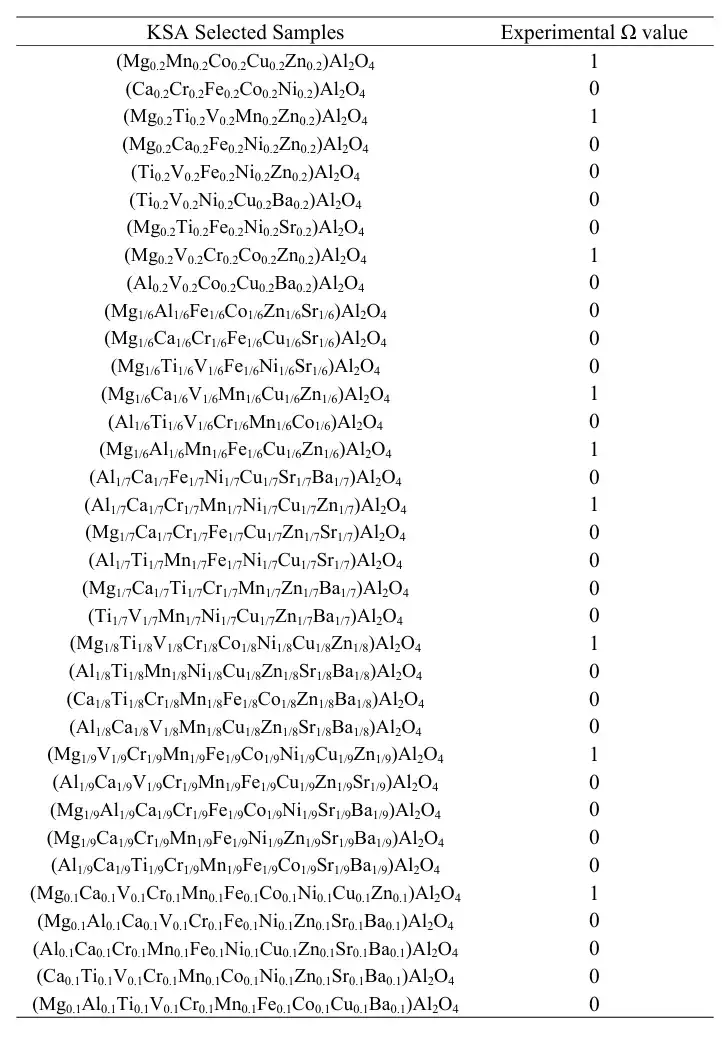
In addition, the researchers combined an additional 209 samples collected during preliminary work, resulting in a diverse initial dataset of 514 data points.Only 105 samples in this initial dataset are single-phase, of which 55 samples are from the KS set and 50 samples are from the Others set, and their T90 (the temperature required to achieve 90% conversion of carbon monoxide in the water gas shift reaction) ranges from 334 to 800 °C.
Model architecture: Active learning to implement the training-prediction-experimentation cycle
This study uses an exploration-based active learning method to explore the catalyst space of high-entropy spinel oxides and screen out high-entropy oxides with high hydrogen production characteristics.The active learning framework includes multiple "Training-Prediction-Experiment" cycles.The workflow is shown in the following figure:
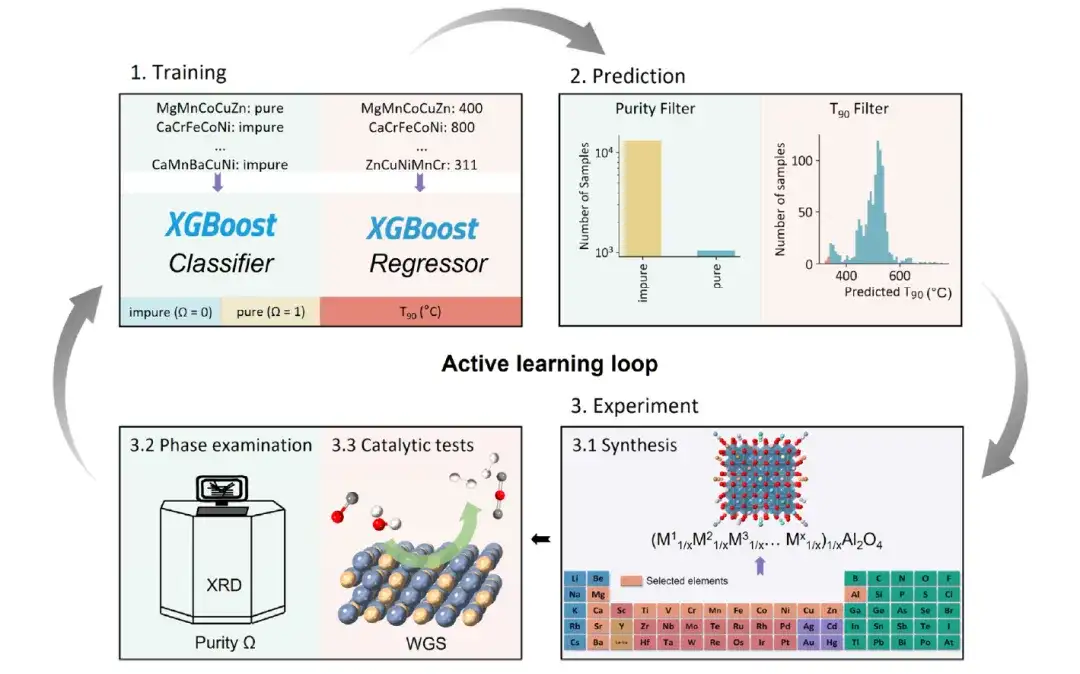
Initially, the researchers used the Kennard-Stone sampling method to select a representative subset for training - this sampling method ensures that the representative subset is evenly distributed across the entire dataset. This process is crucial because it ensures the predictive accuracy of the machine learning model across the entire catalyst space, otherwise AL may be biased and limited to exploring a narrow area.
Subsequently, the purity and catalytic activity of the selected samples were determined experimentally. X-ray diffraction (XRD) was used to evaluate whether the samples had a single-phase structure, while the catalytic activity was evaluated by measuring T90.
In each iteration, the phase purity of HESOs was predicted using XGBoost classifier, and only samples with a probability of more than 50% predicted by the purity classifier were considered, and their catalytic activity was predicted using XGBoost regressor. Then, the samples predicted to be phase pure and with the highest catalytic activity (the top 5 samples with the lowest T90 values) were selected for experiments, and the experimental data were integrated into the training set of the next iteration.This iterative cycle continues until no catalyst with high catalytic activity can be found.
In this way, the researchers were able to efficiently screen for high-performing HEOs that produce hydrogen at rates significantly higher than conventional catalysts in the water-gas shift reaction.
Research results: 4 HESOs catalysts with excellent performance were screened out
In the experiment, the researchers used X-ray diffraction (XRD) patterns as a descriptor, called the Ω value, to assess the purity of the spinel phase; in the water-gas shift reaction, T90 was used as a descriptor to characterize the catalytic activity of the selected HESOs. The lower the T90 value, the higher the activity level of the selected HESOs.
① Verify the ability to discover HESOs through active learning
The researchers conducted 4 rounds of AL iterations, selecting 5 samples in each round. The results are shown in the figure below:
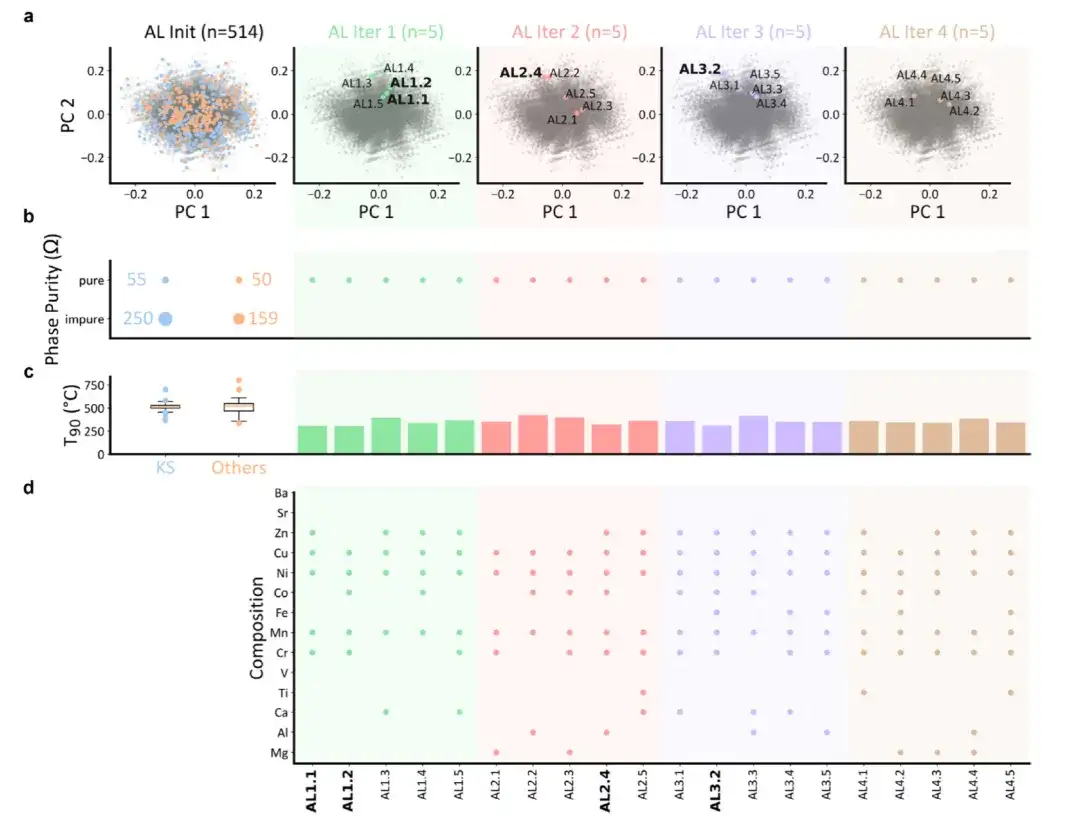
(a) Principal component analysis of the catalyst space, projecting the data onto a two-dimensional plane. Each point represents a sample, the gray background represents the entire data space, and the colored points represent the initial dataset or samples selected by AL.
(b) The spinel phase purity of the samples
(c) T90 value of the sample
(d) Composition of the sample selected by AL
None of the 20 samples selected by AL were found to be non-single-phase, showing an impurity rate of 0.%. This is in stark contrast to the initial dataset, which had an impurity rate as high as 8.4%, as shown in Figure (b) above. The reduction in impurities demonstrates the effectiveness of the proposed AL method in identifying pure samples. In addition, the T90 value of the samples selected by AL was 357 ± 32 °C, which is significantly lower than the T90 value of the initial dataset (513 ± 66 °C). This result indicates thatThe proposed AL algorithm can efficiently find HESOs with higher catalytic activity.
As shown in Figure (c) above, AL also found 4 samples (A1.1, A1.2, A2.4 and A3.2) with T90 values of 311 °C, 307 °C, 323 °C and 312 °C, respectively, which are all lower than the lowest T90 value (334 °C) in the initial data set. This proves the effectiveness of the AL workflow proposed in this study, enabling researchers to efficiently identify new HESOs catalysts with ideal properties.
② Verify the catalytic activity of AL selected samples
The water gas shift reaction (WGS) is of great industrial significance and is mainly used to produce high-purity hydrogen to meet the application requirements of ammonia, methanol synthesis and fuel cells. Before testing the catalytic activity of the AL selected samples, the researchers analyzed the crystal structures of the four samples:
(Cr0.2Mn0.2Co0.2Ni0.2 Cu0.2)Al2O4, denoted as CrMnCoNiCu
(Cr0.2Mn0.2Ni0.2Cu0.2Zn0.2)Al2O4, denoted as CrMnNiCuZn
(Al1/7Cr1/7Mn1/7Co1/7Ni1/7Cu1/7Zn1/7)Al2O4, denoted as AlCrMnCoNiCuZn
(Cr1/7Mn1/7Fe1/7Co1/7Ni1/7Cu1/7Zn1/7)Al2O4, denoted as CrMnFeCoNiCuZn
To evaluate the scalability potential of existing synthetic methods,The researchers successfully synthesized 20 g of CrMnCoNiCu catalyst in a single batch using a 500 mL scale-up crucible and the same muffle furnace. As shown in the figure below, the large-scale synthesized catalyst still maintains a good single-phase spinel structure, which shows that the method used in this study has excellent repeatability and scalability potential.
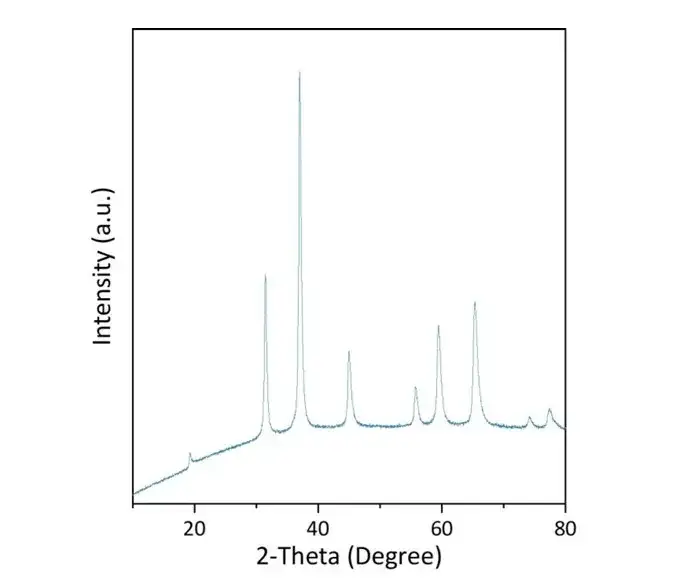
In the XRD test, the scanning rate of CrMnCoNiCu large-scale catalyst was 0.02° and the counting time per step was 2 s.
The researchers then tested the catalytic activity of the four AL-selected HESO catalysts, as shown below:
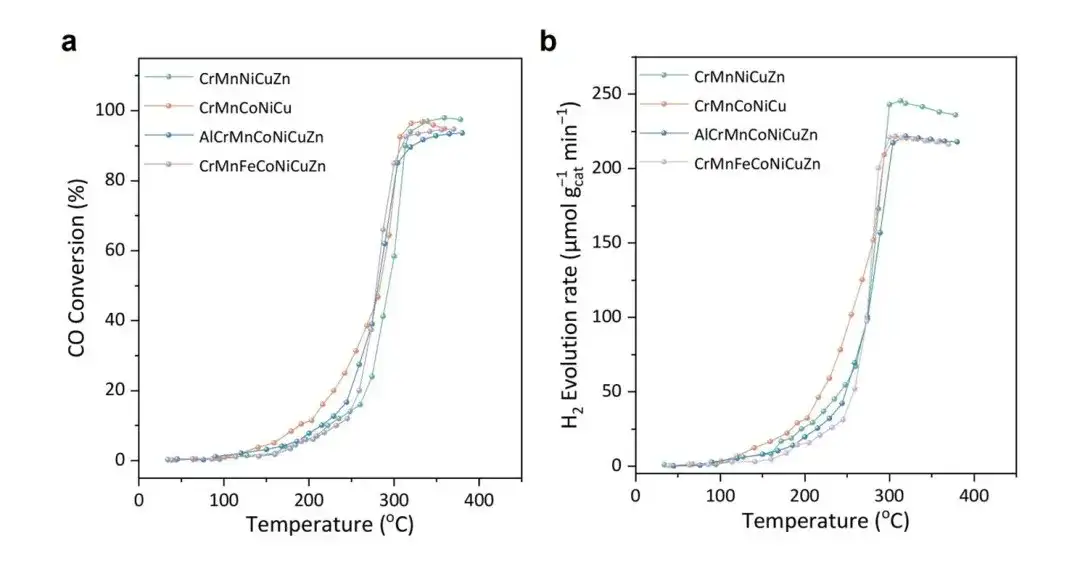
(a) CO conversion rate;
(b) H2 generation rate
Reaction conditions: space velocity (WHSV) = 200,000 mL/(h·gcat); feed gas: 1.8% CO and 2.5% H2O, inert gas is Ar
As shown in Figure (a), the catalytic activities of all samples not only match the predicted T90 values, but also show the best screening activity. For example, ML predicts that the T90 of the CrMnCoNiCu sample is 311°C, while the actual measured T90 is 310°C.
More importantly,Compared with the traditionally designed catalysts CZA and Fe/Cr, the samples screened by ML showed higher catalytic activity.For example, under the same reaction conditions, the hydrogen yield of the CrMnCoNiCu sample is 135 μmol gcat⁻¹ min⁻¹, which is significantly higher than 15 μmol gcat⁻¹ min⁻¹ of Fe/Cr and 81 μmol gcat⁻¹ min⁻¹ of CZA, as shown in the figure below.Furthermore, further stability tests showed that under T90 conditions, the CrMnCoNiCu and CrMnFeCoNiCuZn samples had good stability and maintained high activity in hydrogen production.
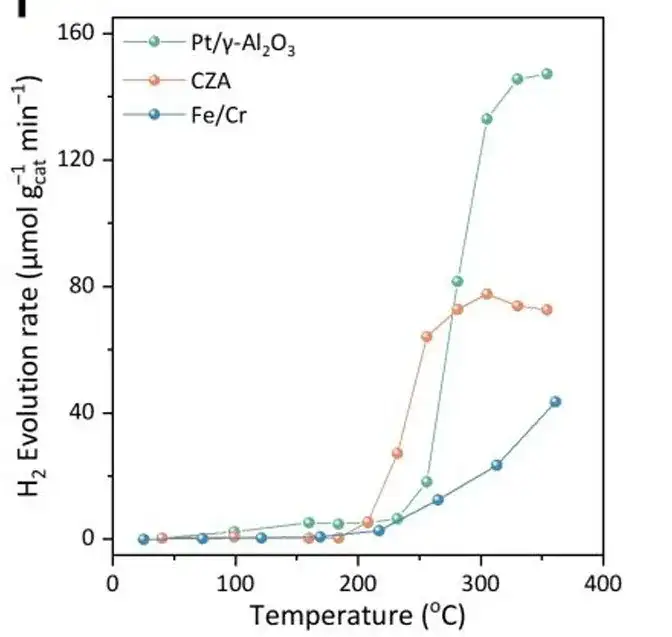
After multiple iterations, the researchers successfully identified the most promising HESOs in a wide range of chemical space, which exhibited excellent hydrogen production performance (251 μmol gcat⁻¹ min⁻¹) in the water-gas shift reaction at 300 °C, while showing excellent stability in tests lasting up to 120 hours.
In summary, the researchers screened out 4 highly efficient high-entropy MgAl2O4 from 14,443 candidate samples. Type spinel catalyst.
AI injects strong innovative power into the research and development of high entropy materials
If you ask what is the hottest material in the 21st century, high entropy materials must occupy a place, mainly represented by the two categories of HEA (high entropy alloy) and HEO (high entropy oxide).
Among them, high entropy alloys have the advantages of high strength, high hardness, corrosion resistance, wear resistance, high temperature resistance, radiation resistance and soft magnetism, etc., and have broad application prospects. For example, their good refractoriness makes them suitable for use as turbine blade materials, welding materials, heat exchanger materials, refractory materials for high-temperature furnaces, and aerospace materials, etc.
Although high-entropy alloys have excellent characteristics, discovering new high-entropy alloy components is still a difficult research task. The element composition in high-entropy alloys needs to be controlled within a certain range. Excessive or insufficient elements may cause the alloy performance to deteriorate. At the same time, the various element compositions of high-entropy alloys may produce nonlinear effects, and it is difficult to accurately predict their performance through traditional experimental methods. In this context, the use of AI technologies such as machine learning can complete the research and development of high-entropy alloys more quickly and conveniently.
Not long ago, Su Yanjing's team at the University of Science and Technology Beijing designed a multi-objective optimization (MOO) framework that combines ML, genetic search, cluster analysis, and experimental feedback.And for the composition space of refractory high entropy alloys (RHEAs), we searched for alloys with optimal high temperature strength and room temperature ductility. Specifically, the research team synthesized 24 RHEAs and determined that the ZrNbMoHfTa alloy has potential for high temperature applications. Among them, the Zr0.13Nb0.27Mo0.26Hf0.13Ta0.21 alloy exhibited excellent mechanical strength, with a yield strength of nearly 940 MPa at 1200°C and a room temperature fracture strain of 17.2%. The alloy's significant heat resistance and good structural stability indicate its potential for structural applications at high temperatures, while room temperature ductility improves the alloy's processing performance.
Let’s look at high entropy oxides. Due to the countless elemental compositions, it is extremely difficult to discover HEOs catalysts through trial and error., Zhang Pengfei's research group at the School of Chemistry and Chemical Engineering of Ningxia University has made progress in the research of machine learning screening of high-entropy oxide catalysts.The team established a high-quality regression model by searching for negative data and selecting appropriate training data to search for catalysts with better performance. Ultimately, the unconventional catalyst Ni0.04Co0.48Zn0.36V0.12Cr2Ox was screened out, which has excellent sulfur and water resistance and long-term stability (>7000 hours, T(90)=345°C).
The related research was published in JACS under the title "Machine Learning Accelerated Discovery of Entropy-Stabilized Oxide Catalysts for Catalytic Oxidation".
Obviously, artificial intelligence technology has injected powerful innovative impetus into the development of high-entropy alloys, and it is expected to be more widely used in fields such as energy, environmental protection and new materials in the future.
References:
1.https://pubs.acs.org/doi/10.1021/jacs.4c06272
2.https://www.mip1953.com/newsinfo/6451538.html