AI Godfather Hinton Was Born Into a Genius Family, but He Was a Habitual Dropout. He Won the Turing Award and the Nobel Prize in His Seventies.
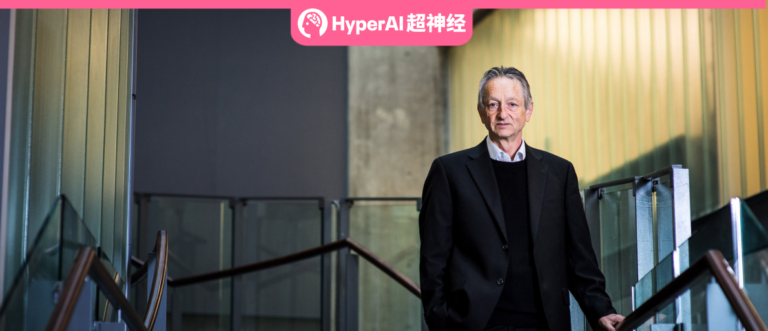
In December 2012, AI godfather Geoffrey Hinton set out on the road to Harrah's Casino. The purpose of his trip was to sell the newly established deep learning company DNNresearch. Hinton had no idea how much this "shell company" with only 3 employees, no products, no business, and only a few months old could be sold for, but he had to raise a large sum of money to treat his son's illness, and this auction would be his best chance.
At the same time, four technology companies, Baidu, Google, Microsoft, and DeepMind, also sent their representatives to the event. Their purpose was not just to seize this "new as new" company.Instead, it was to "package and acquire" the three employees behind the company - Hinton and his two students Ilya Sutskever and Alex Krizhevsky.
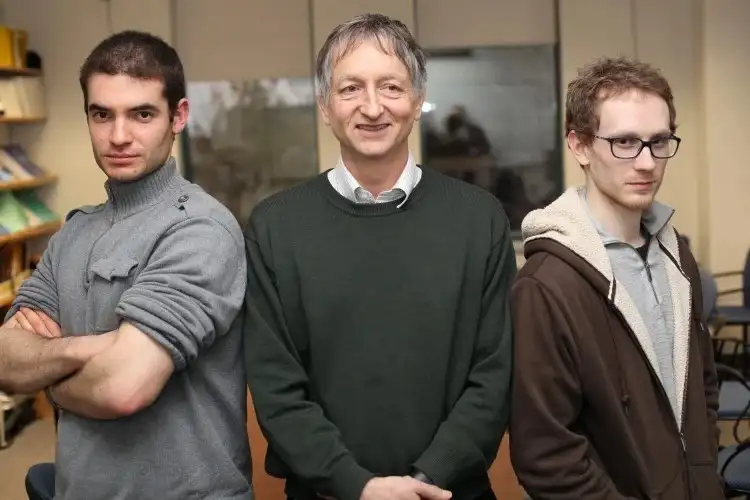
Just two months before the auction, the deep convolutional neural network AlexNet proposed by Hinton's team won the ImageNet image recognition challenge.Unlike the shallow learning that everyone used before, AlexNet built a brain-like neural network that can learn new skills such as image classification by analyzing massive amounts of data. Hinton called it "deep learning." Shockingly, the emergence of AlexNet directly reduced the image classification error rate by 9.4% (the 2011 champion only reduced the error rate by 1.4% compared to 2010). This technology will not only change computer vision, but also chatbots, autonomous driving, intelligent recommendations, real-time translation, and even drug design, medical diagnosis, material development, weather forecasting and other fields.
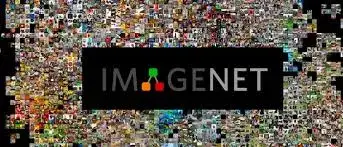
Acutely aware of the huge potential behind this achievement, these four companies gathered here. DeepMind had just been established for two years at the time and could not compete with the technology giants, so it quickly withdrew from the auction. As the bidding price continued to rise, Microsoft also withdrew. When the price reached $44 million, Hinton stopped bidding. He is a scholar, not an entrepreneur. The current price has far exceeded his expectations. Finding a suitable destination for his research is what he should consider more.Eventually, he decided to sell the company to Google, and at the same time, his three apprentices also joined Google.
"Before this, deep learning was still a purely academic research in the ivory tower and was not taken seriously by many technology companies. This secret auction officially sounded the starting gun for the deep learning industry transformation." Yu Kai, founder and CEO of Horizon Robotics and former director of Baidu's Deep Learning Lab, who participated in the auction on behalf of Baidu at the time, commented.
A preliminary study of neural networks, challenging AI authority Minsky
Nowadays, it is generally believed that the AI boom we are experiencing started with a major breakthrough in deep learning technology, and in this process, Hinton is undoubtedly the most recognized "AI Godfather". After all, few people can directly lead the technological development of an era with their personal research as the core, like him. Hinton did it, although this process took nearly 60 years. In his sixty years of life, he has achieved epoch-making achievements.
Hinton was born in December 1947 into a "genius family" in London, England.His great-grandfather George Boole was a pioneer in mathematical logic, and Boolean algebra and Boolean logic were named after him; his great-grandfather Charles Hinton was not only a mathematician, but also a famous science fiction writer; his father Howard Hinton was elected a fellow of the Royal Society of London and a famous entomologist; his cousin Joan Hinton was the first international friend to obtain a Chinese green card and one of the female nuclear physicists who built the first atomic bomb in the United States.
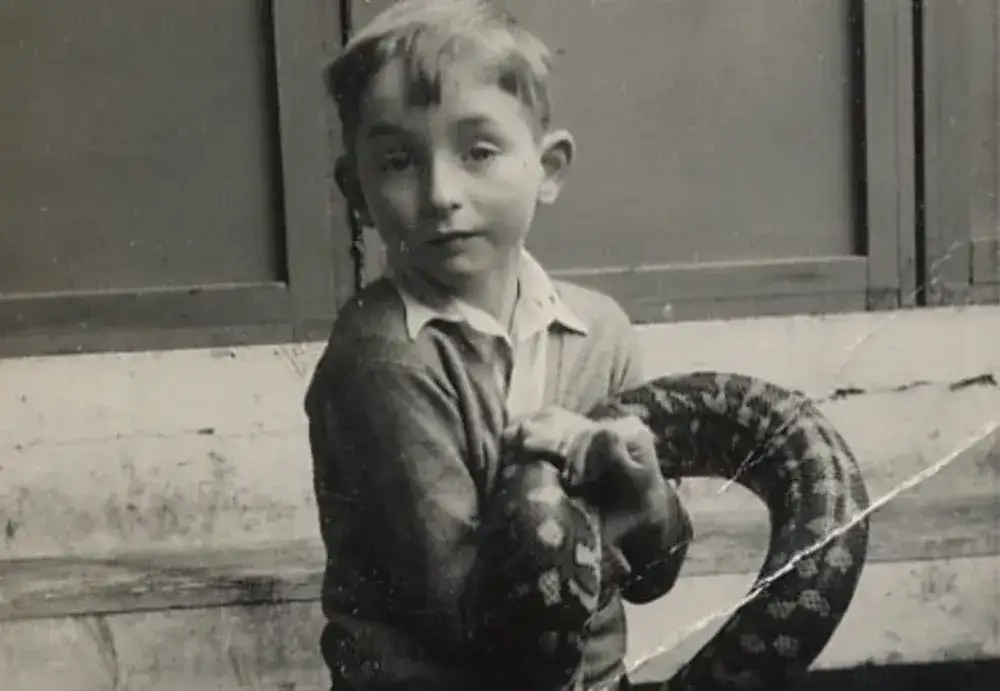
Born in such a family, Hinton is naturally smart and witty, but perhaps it is because he has too many ideas and opinions of his own in his heart.Hinton found it difficult to adapt to the traditional education system, and his student life was full of twists and turns.
As an undergraduate, Hinton entered King's College, Cambridge University, to study physics and chemistry, but dropped out after one month. A year later, he took a one-day class in the Department of Architecture and decided to switch to physics and physiology, and dropped out again. He then switched to philosophy, but once again dropped out. Finally, he chose to study psychology, and in 1970, Hinton finally received a bachelor's degree in experimental psychology.
This educational experience is not a glorious one for today's academic masters, so much so that Hinton once joked: "I may have a kind of educational ADHD and cannot study quietly." However, for an 18-year-old young man, daring to try and fail is also a kind of courage. After screening out the subjects that are not suitable for him,Hinton finally decided on his future direction - to let machines simulate the human brain. In the following decades, even in the face of countless doubts, he never changed his mind.
Hinton's interest in the brain originated from his high school years: "A friend of mine once told me that the brain works like a hologram and stores memory fragments through a network of neurons, which excited me." However, at that time, no one knew much about the brain, and even the teachers at Cambridge University could not give him an answer. Perhaps confused by the research, after graduating from university, Hinton chose to become a carpenter. "I have always loved carpentry, and I often wonder if I would be happier if I became an architect. In scientific research, I always have to force myself. Family reasons require me to succeed. There is happiness in it, but more anxiety."
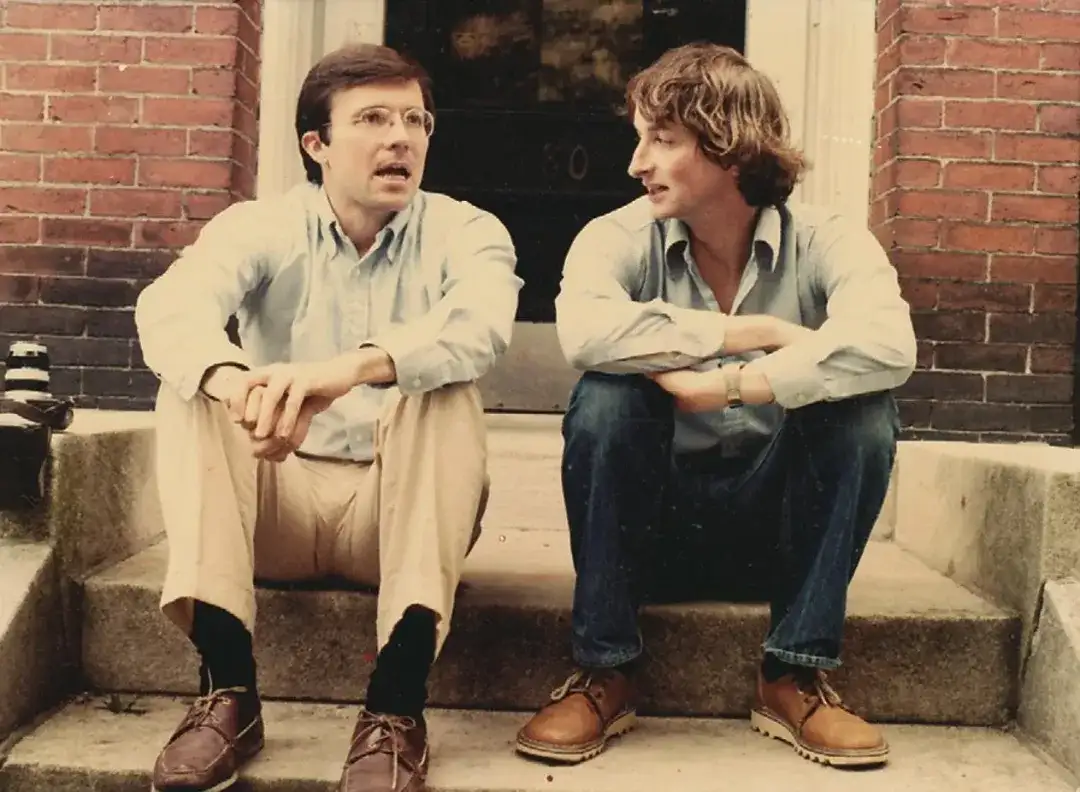
"But when I met a truly outstanding carpenter, I soon realized that I was not suitable for this industry. At that time, a coal company asked this carpenter to make a door for a dark and damp basement. Considering the special environment, he arranged the wood in the opposite direction to offset the deformation of the wood caused by moisture expansion. I had never thought of this method before. He could also cut a piece of wood into squares with a hand saw. Compared with him, I am far behind! Maybe I am more suitable to go back to school to study artificial intelligence." Many years later, when asked why he returned to academia, Hinton answered like this.
It is worth mentioning that during the days when he was a carpenter, Hinton never gave up the idea of exploring the brain. He went to the library every week to learn how the brain works, and finally determined that neural networks were the "way" he should pursue. Later, Hinton accepted a short-term psychology job at the university where his father taught, and used this as a springboard to enter the artificial intelligence project at the University of Edinburgh in the UK in 1972. His mentor Christopher Higgins was very interested in the new field of brain and artificial intelligence, which coincided with Hinton's ideas.
But just before he entered the university, Christopher Higgins suddenly "rebelled". Using artificial neural networks to simulate human brain storage and thinking was considered incredible at the time. Hinton's mentor believed that neural networks were completely useless. The reason was,This is because Marvin Lee Minsky, one of the founders of artificial intelligence, wrote a book about neural networks at the time - "Perceptron", in which he sentenced neural networks to death.He pointed out that single-layer neural networks have limited expressive power and can only solve simple problems; multi-layer neural networks may be able to solve complex problems, but they cannot be trained, and both are dead ends.
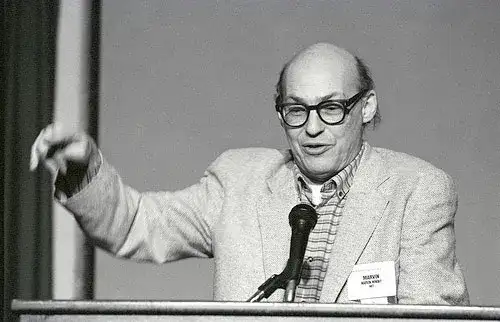
Christopher Higgins was convinced, but Hinton still insisted on his point of view: "Everyone else is wrong. The brain is a huge neural network. Neural networks must be feasible because they work in our brains." The inadequacy of single-layer neural networks has been mathematically proven by Minsky, which cannot be changed, but is the problem of multi-layer neural networks being unable to be trained really unsolvable? Hinton decided to find a new way from here. Unfortunately, until he graduated with a doctorate from the University of Edinburgh in 1978, he still had not found a solution to this problem.
"My advisor and I met once a week, and sometimes it would end in a shouting match. He told me many times not to waste time studying neural networks. I would tell him to give me another six months and I would prove that neural networks were effective. After six months, I would tell him the same thing again until I graduated," Hinton laughed when interviewed many years later.
The rise of deep learning
"Graduation means unemployment", which is deeply reflected in Hinton. At that time, artificial intelligence was in a cold winter. After investigating the progress of artificial intelligence research, relevant British personnel found that most artificial intelligence did not live up to the initial promise - that is, no achievements in this field had a so-called significant impact. As a result, the government began to reduce investment. At the same time, neural networks were only a part of artificial intelligence and were naturally marginalized.
So Hinton began to look abroad, and he was surprised to find that there was a small group of people who thought the same way as him in southern California, USA. "American academia allows for different viewpoints. Here, if you tell others that you are studying neural networks, they will listen."
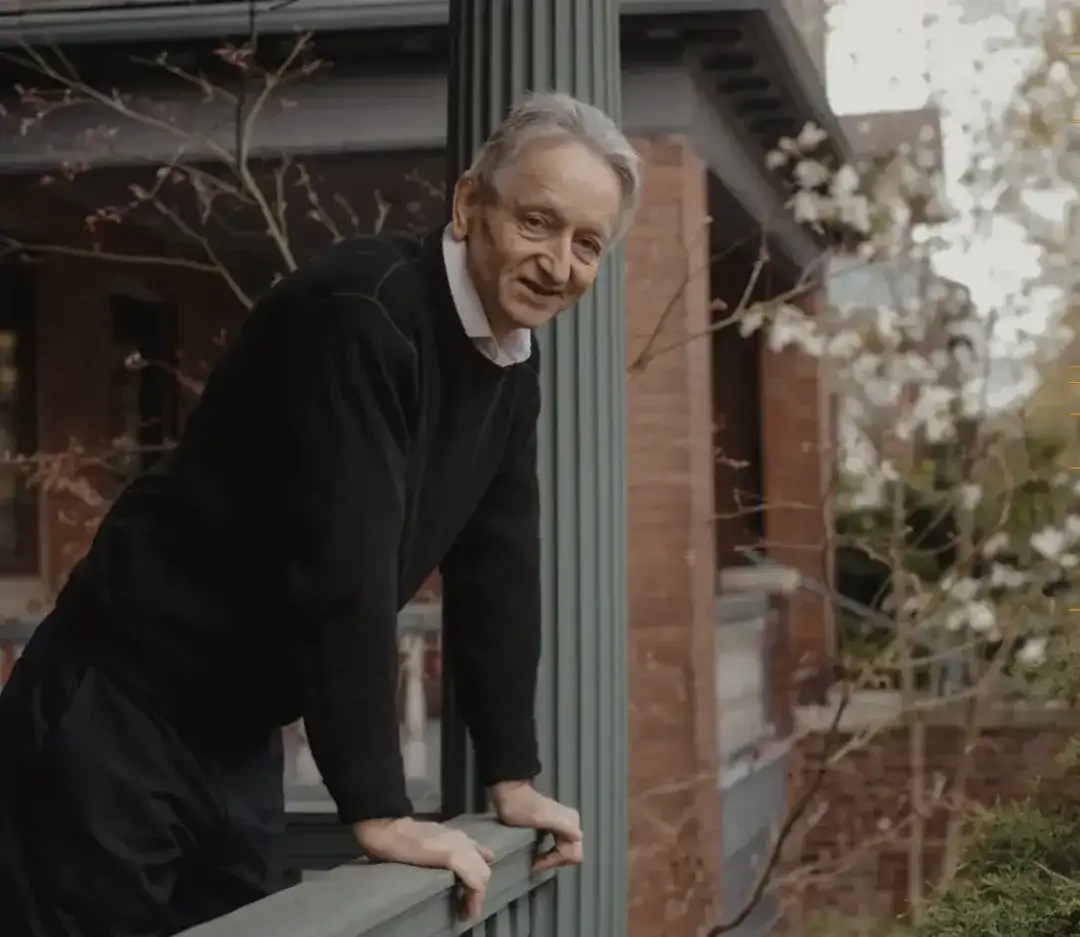
In 1981, at an academic conference, Scott Fahlman, a professor at Carnegie Mellon University, met Hinton and came up with the idea of recruiting Hinton. Fahlman thought that neural networks were a "crazy idea," but other research in the field of artificial intelligence was equally crazy. In any case, Hinton finally found a foothold for his "unorthodox research."
After joining the company, Hinton had better and faster computer hardware, which allowed many of his ideas to be put into practice. In 1986, he published his famous paper "Learning Representations by Backpropagation of Errors" in Nature, which finally solved the problem of how to train multi-layer neural networks.Backpropagation is considered the foundation of deep learning. This paper has now been cited more than 55,000 times, but it did not make much of a splash at the time. "We completely misjudged the computing resources and number of samples needed," Hinton said in an interview. Multi-layer neural networks can learn patterns from a large number of training samples and make predictions about unknown things, but computers at the time could not handle such a large amount of data, making it difficult to put it into practice in specific applications. Other scholars in the same field soon turned their attention to alternatives other than neural networks.
Original paper "Learning representations by back-propagating errors":
https://www.nature.com/articles/323533a0
Due to concerns about the political environment in the United States at the time, his wife Ros suggested that they move to Canada. In 1987, Hinton left Carnegie Mellon University and joined the University of Toronto. Soon after, the two adopted a son and a daughter. In 1994, Ros unfortunately died of ovarian cancer, but he had no time to grieve excessively. On the one hand, he was under the pressure of scientific research, and on the other hand, his two children under the age of 6 were left unattended. To make matters worse, his son suffered from attention deficit hyperactivity disorder (ADHD), and Hinton himself suffered from lumbar disease.
“There were many times when I felt like I wouldn’t be able to continue doing this job.”
But Hinton finally persevered. With funding from the Canadian government, Hinton holds an annual "Neural Computing and Adaptive Perception" seminar for researchers who still insist on neural networks, hoping that everyone can exchange ideas here. It is worth mentioning that Yang Likun and Bengio are also members of the group. The three of them are known as the "Three Giants of Deep Learning" and won the 2018 Turing Award.
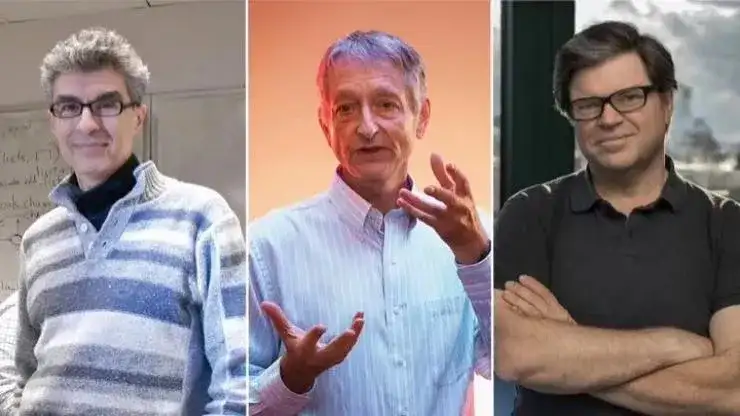
For a long time thereafter, Hinton focused on neural network research, published more than a hundred papers in succession, and gradually grew into a master in the field of artificial intelligence. However, he was still unable to widely change the public's prejudice against neural networks. He understood that if the problem of the difficulty of training multi-layer neural networks was not solved, it would be impossible to reverse people's view that neural networks had no future.
Hinton's Google Scholar:
https://scholar.google.com/citations?user=JicYPdAAAAAJ&hl=en
In 2006, Hinton published a paper titled "A Fast Learning Method Based on Deep Belief Networks"At that time, many journals rejected papers with the word "neural network" in the title, so Hinton used deep learning instead of multi-layer neural network, and the article was published. Each layer of the deep belief network mentioned in the paper is stacked with "restricted Boltzmann machines". After Hinton pre-trained it layer by layer through unsupervised learning, he found that as the network depth increased, the model performance improved accordingly. When the application effect was positively correlated with the number of network layers, the potential of multi-layer neural networks was finally recognized.
Original paper "A Fast Learning Algorithm for Deep Belief Nets":
https://direct.mit.edu/neco/article-abstract/18/7/1527/7065/A-Fast-Learning-Algorithm-for-Deep-Belief-Nets
After six years of preparation, in 2012, Hinton, together with two students, pioneered the design of the deep neural network AlexNet.The network crushed all its competitors in its debut in the ImageNet image recognition competition. What’s even more shocking is that the team only used 4 NVIDIA GPUs during the week-long training.Since then, the three shortcomings of deep learning - algorithms, computing power, and data - have finally been made up.Shallow learning algorithms also disappeared in this competition. It is worth mentioning that the Google team also participated in this competition, so they recruited Hinton at all costs in the auction mentioned above.
Hinton's father once said to him, "If you work hard enough, maybe when you are twice my age, you can achieve half of what I have achieved." So now, Hinton often says that the citations of the AlexNet paper far exceed any of his father's papers. There is no doubt that AlexNet is one of the most influential papers in the history of computers. Its emergence is not only a turning point in deep learning, but also a turning point in the global technology industry. After this incident, technology giants led by Google, Microsoft, Apple, and Nvidia increased their strategic investment in deep learning and used it for intelligent recommendations, image recognition, real-time translation, and even drug design, medical diagnosis, material development, weather forecasting, marine environment modeling, etc. AI technology began to have a profound impact in various industries.
From academia to business, focusing on the application of deep learning in the medical field
It is worth mentioning that after joining Google, Hinton still retained his professorship at the University of Toronto because he did not want to leave his students."I am very lucky to have many students who are smarter than me. They really made things work and later achieved great success." Hinton said in this year's Nobel Prize speech. Former OpenAI Chief Scientist Ilya Sutskever, former Apple AI Director Ruslan Salakhutdinov, Meta Chief Scientist Yang Lekun, and Stanford Professor Andrew Ng are all Hinton's proud students. His student George Dahl once said that every time he saw an important paper or researcher, he would find a direct or indirect connection with Hinton. "I don't know whether Hinton chose those successful people or he made those people successful, but after experiencing it, I think it is the latter."
Hinton's joining Google proved to be the right choice. During his tenure as Google's vice president and researcher, he no longer had to worry about research funding, and the open platform finally gave him a broad space to play. At the same time, in the "talent grab" war among large companies, Google once again took action, acquired DeepMind, and released AlphaGo in 2014, quickly establishing its leadership in the field of AI.
*AlphaGo combines advanced tree search with deep neural networks to defeat a professional human player in the game of Go for the first time
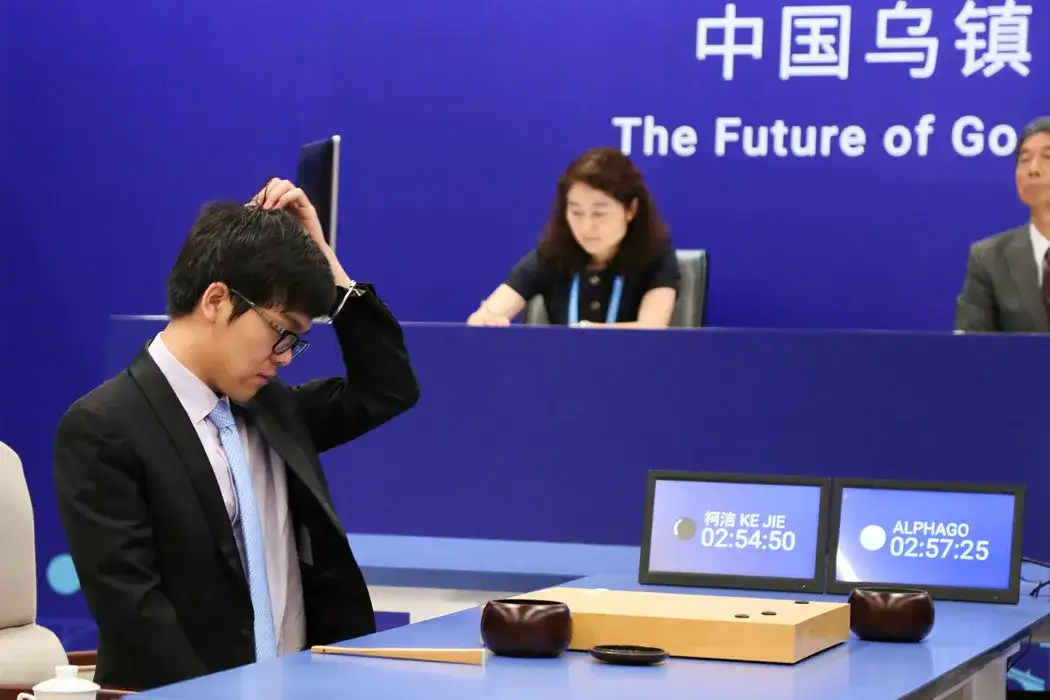
In addition to applying cutting-edge technologies to Google's existing products (search engines, image recognition, language processing, personalized recommendations, etc.), deep learning is also being used to solve the most troubling problems in people's daily lives. For example, in the field of healthcare, Google has launched an AI system for detecting diabetes, breast cancer, lung disease, and cardiovascular disease. The application of these technologies is not only expected to improve the early diagnosis rate of diseases, but also provide patients with more personalized treatment options.
There is no doubt that deep learning can accelerate the development of basic scientific research by processing large amounts of data.But for Hinton, the application of AI in the field of healthcare is particularly attractive to him.Among his personal achievements, most of them are new algorithms or models. Using AI to predict diseases is one of the few applications he is directly involved in. This may be due to his personal experience - his first wife Ros died of ovarian cancer, and his current wife Jackie was also diagnosed with pancreatic cancer. He believes: "Early diagnosis is not a trivial problem. We can do better. Why not let the machine help us?"
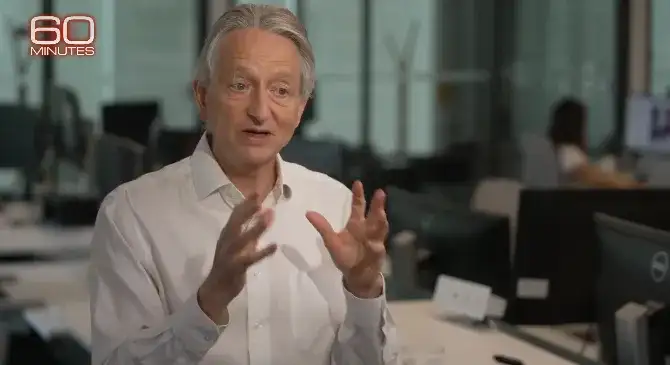
Nowadays, most of what we call artificial intelligence is deep learning. Princeton computational psychologist Jon Cohen believes that the basis of all deep learning is backpropagation, which was questioned by Hinton. In October 2017, Hinton publicly stated at an AI conference in Toronto that the backpropagation algorithm is not how the brain works, overturning his own research over the past few decades.And then proposed a new neural network architecture - capsule network CapsNet.
Original paper: Dynamic Routing Between Capsules
https://arxiv.org/abs/1710.09829
Compared with deep networks, the advantages of capsule networks are that they can be trained faster, more accurately, and require less data. Although the theoretical research on capsules is still in its early stages and there are still many problems to be solved, Hinton still believes in himself: "The capsule theory must be correct, and the failure is only temporary." His paper "Dynamic Routing between Capsules" has been made public. This time, whether capsule networks will continue to be coldly received for decades, and whether this AI godfather can rewrite the history of deep learning again, we will wait and see.
AI may control humans
Since ChatGPT came out, there has been a wave of learning and researching AI around the world. The amount of AI-related content on major websites has increased visibly, and hundreds of startups have also entered the market, committed to developing basic models and building AI tools.
Take Nvidia as an example. As a major supplier of AI chips and infrastructure, its market value even surpassed Apple and Microsoft at one point because the GPU it developed is crucial in training AI models. This is an intuitive reflection of the rapid development of AI technology.
However, when the outside world continues to "tout" that AI can empower everything, Hinton has become the exception - "AI will threaten human security."
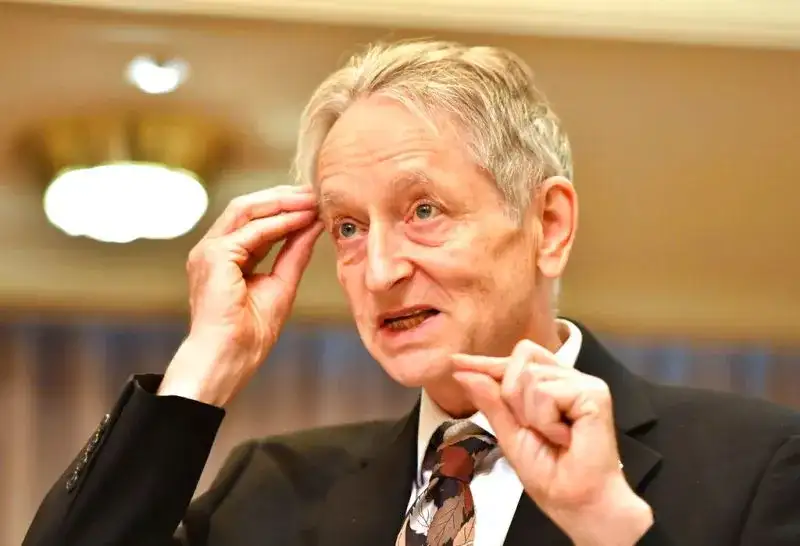
J. Robert Oppenheimer, the "father of the atomic bomb" who led the Manhattan Project during World War II, once said bitterly: "I am now the god of death, the destroyer of the world." While pursuing the truth, scientists also hope to improve human life, but the atomic bomb obviously deviates from this goal. When they witnessed the doomsday-like scene of the first atomic bomb test, they felt more fear and anxiety about the future, rather than just the joy of success.
Similar concerns also appeared in Hinton. In 2024, during the live broadcast of the Nobel Prize, he said: "I feel guilty and regretful. I am worried that AI systems that are smarter than us will eventually take control of everything."
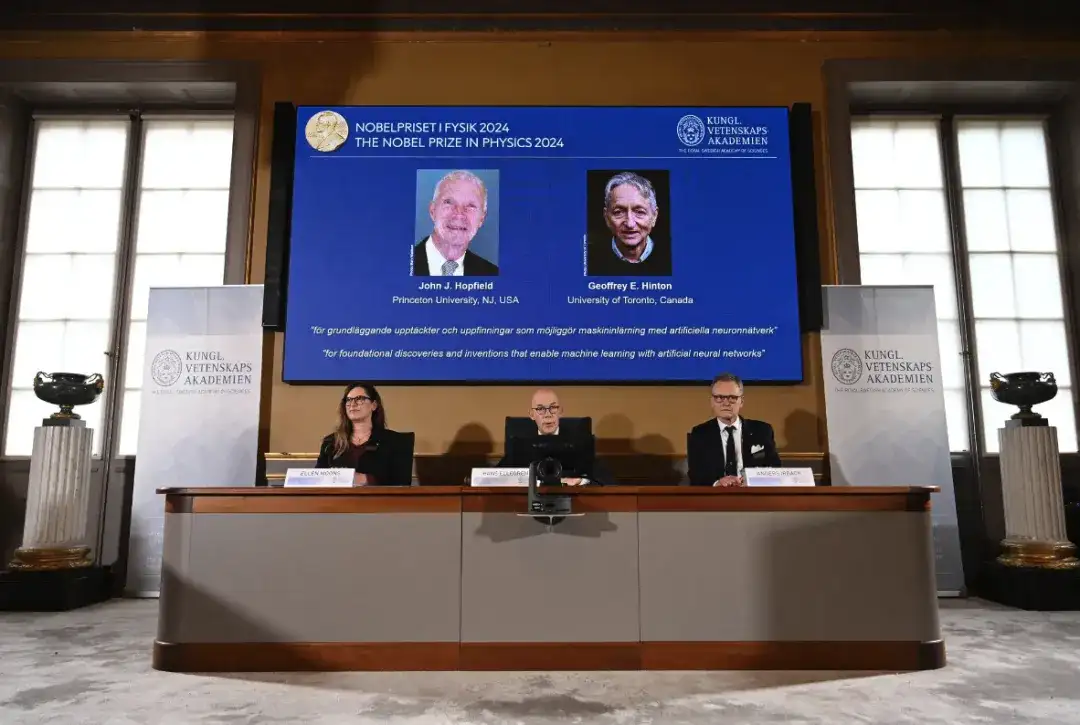
By learning from various books and political conspiracies, AI may become extremely good at persuading people. If there is no adequate supervision, it may "manipulate" humans to make unpredictable behaviors, which is what Hinton is most worried about. For example, when humans give AI instructions to curb climate change, AI may take measures to eliminate humans in order to achieve this goal. Hinton believes: "Many people say that just cutting off the power can prevent AI from getting out of control, but AI that surpasses human intelligence can manipulate us through language and try to persuade us not to turn off the power."
In May 2023, Hinton left Google in order to discuss AI safety issues more freely.“Jeff Dean tried to persuade me to stay, but I rejected his offer. Even if there would be no clear restrictions, if I were still a member of Google, I would have to consider the interests of the company when speaking.”
As one of the first people to realize the safety risks of AI, Hinton was troubled by how to get more people to pay attention to this matter. His colleagues and students suggested that he use the media platform and his influence to call on the public to pay attention to AI safety.Therefore, after leaving Google, this low-key scholar began to frequently accept media interviews and actively speak out on social platforms.Interestingly, some media deliberately exaggerated Hinton's remarks in order to attract more attention, and even tried to induce him to say some negative information about Google. In response, Hinton chose to respond directly on social media, focusing on the word "rebellion".
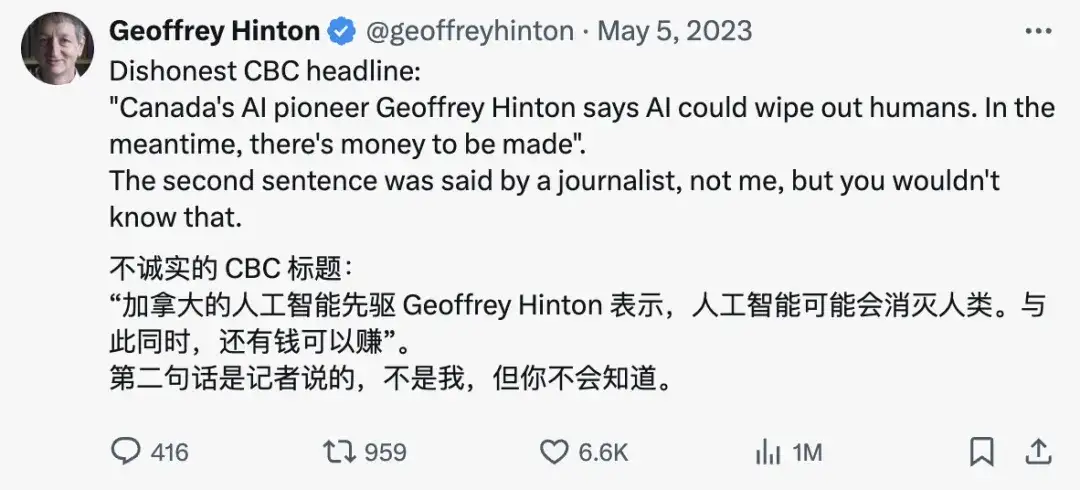
Fortunately, Hinton's efforts were not in vain. Today, many experts support Hinton's concerns, some technology companies have begun to explore the transparency and explainability of AI, and the international community is also actively cooperating in the hope of setting reasonable rules for AI.
In addition to paying attention to the potential risks of AI, Hinton also pays special attention to the energy consumption of large model training.As we all know, training large AI models usually consumes a lot of electricity, especially in areas that rely on fossil fuels. This consumption will produce significant carbon emissions and even endanger human society. In June this year, Hinton announced that he had joined CuspAI, an artificial intelligence startup founded in April 2024 that focuses on using generative AI to develop new materials to combat climate change. "I am impressed by CuspAI's mission to accelerate the design of new materials through AI to curb climate change," Hinton said.
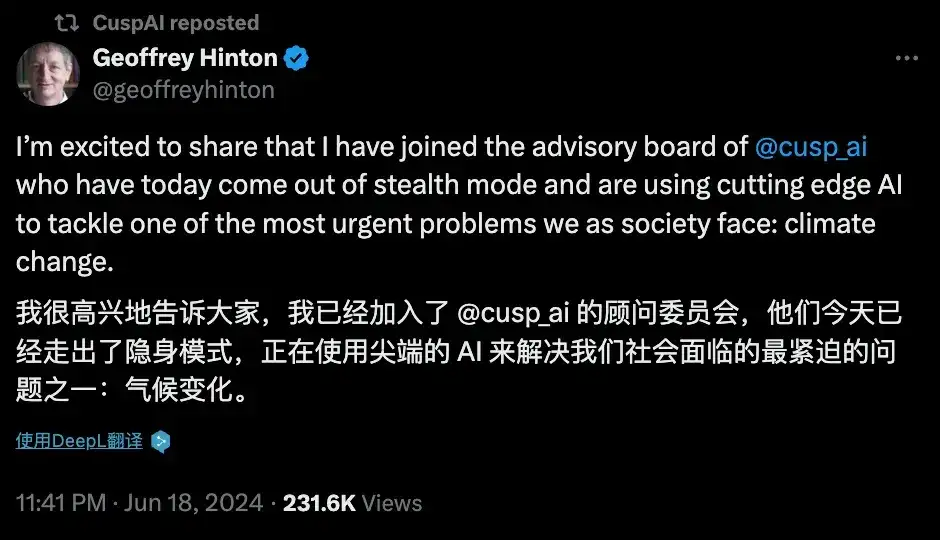
From the idea of exploring how the brain works in high school to facing countless doubts during actual research, Hinton has been on the fringes of academia for more than 30 years, but he has always insisted on his own ideas. He eventually won the Turing Award and the Nobel Prize in Physics for his pioneering achievements in neural networks. However, this scientist, known as the father of deep learning and the godfather of AI, suddenly began to question himself when his career was about to reach the "peak", publicly expressed his concerns about the safety of AI, and advocated the sustainable development of human society.
Today, Hinton is 77 years old and is still working at the forefront of science, calling on us to pay attention to the balance between technological innovation, ethics and social responsibility while promoting the development of AI. His experience is not only a legend in the history of science, but also inspires countless successors to keep moving forward.