Be the First to Experience the Demo! The Genome-based Model Evo Is on the Cover of Science, Achieving Prediction and Generation From Molecular to Genomic Scales
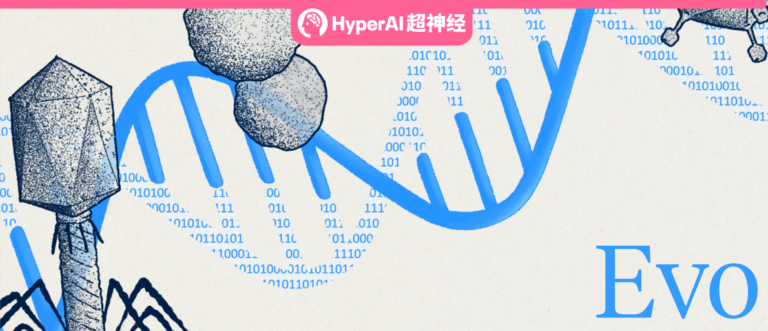
Recently, AI has made great progress in the field of biology.A research team from Stanford University and the Arc Institute in the United States proposed a genome-based model Evo, which can achieve zero-sample prediction and high-precision generation in multimodal tasks of DNA, RNA and protein.
The related research was published in Science as a cover article with the title "Sequence modeling and design from molecular to genome scale with Evo".

Paper address:
https://www.science.org/doi/10.1126/science.ado9336
Follow the official account and reply "Evo" to get the complete PDF
As the first author of the paper, Eric Nguyen posted several updates to introduce Evo's achievements, thanked the team members many times, and said frankly, "It is an honor to work with such an amazing team!"

According to the paper, Evo uses the StripedHyena architecture and is trained on a large genomic dataset containing more than 80,000 bacterial and archaeal genomes.and millions of predicted phage and plasmid sequences, covering 300 billion nucleotide tokens, capable of generating DNA sequences with a reasonable genome architecture of more than 1 megabase in length.
also,With a parameter scale of 7 billion and a maximum context length of 131,072 tokens, Evo can reveal the intricate co-evolution between coding and non-coding sequences and design complex biological systems.Such as CRISPR-Cas complexes and IS200 and IS605 transposons.
In summary, Evo's ability to predict, generate and design entire genome sequences not only provides new theoretical support for life sciences, but is also expected to be applied to gene editing, drug discovery, disease diagnosis, agriculture and other fields, helping to develop breakthrough results in multiple fields.
Many netizens were shocked by the release of Evo and were looking forward to the specific application of this model.


In order to let everyone experience the powerful functions of the Evo model for the first time,HyperAI Hyperneuron Tutorial is now online "Evo: Prediction and Generation from Molecular to Genomic Scale".No need to enter any commands, just one-click cloning for a quick experience!
Tutorial Link:
Demo Run
1. Log in to hyper.ai, on the Tutorials page, select Evo: Prediction and Generation from Molecular to Genomic Scale, and click Run this tutorial online.


2. After the page jumps, click "Clone" in the upper right corner to clone the tutorial into your own container.

3. Click "Next: Select Hashrate" in the lower right corner.

4. After the page jumps, select "NVIDIA RTX A6000", and select "Pay as you go" or "Daily/Weekly/Monthly Package" according to your needs, select the "PyTorch" image, and click "Next: Review". New users can register using the invitation link below to get 4 hours of RTX 4090 + 5 hours of CPU free time!
HyperAI exclusive invitation link (copy and open in browser):
https://openbayes.com/console/signup?r=Ada0322_QZy7

5. After confirmation, click "Continue" and wait for resources to be allocated. The first cloning process will take about 2 minutes.


6. When the status changes to "Running", click the "Open Workspace" option.

7. After entering the Jupyter workspace, double-click the "README" file to officially enter the Evo model running page.

Effect Demonstration
1. After entering the Evo model running page, all parameters are in default mode. Scroll down to "2. Start the model and enter relevant parameters" to adjust the prompt parameter values according to your needs.It should be noted that the default value of prompt is "ACGT", which represents an initial sequence consisting of DNA base pairs (A, C, G, T).You can modify this value to generate different DNA sequences as needed.

2. For example, change the default value of prompt to AGCT. After adjusting the default parameter values, click the "Restart and run all cells" option and select "Restart" to run.


3. Wait for a while, when the [*] turns into a number, it means the operation is complete. At the bottom of "2. Start the model and enter relevant parameters", you can see the generated sequence.



4. In addition, the Evo model can also analyze the generated DNA sequences and learn the co-evolutionary relationship between coding sequences and non-coding sequences. It can also predict protein-coding genes from DNA sequences, encode and design RNA systems, and thus predict the generated protein folding structure, which is finally presented in the form of an image.

We have established a "Stable Diffusion Tutorial Exchange Group". Welcome friends to join the group to discuss various technical issues and share application results~
Scan the QR code below to add HyperaiXingXing on WeChat (WeChat ID: Hyperai01), and note "SD Tutorial Exchange Group" to join the group chat.
