Dialogue With Hong Liang From Shanghai Jiao Tong University: It Is More Fulfilling to Implement AI for Science
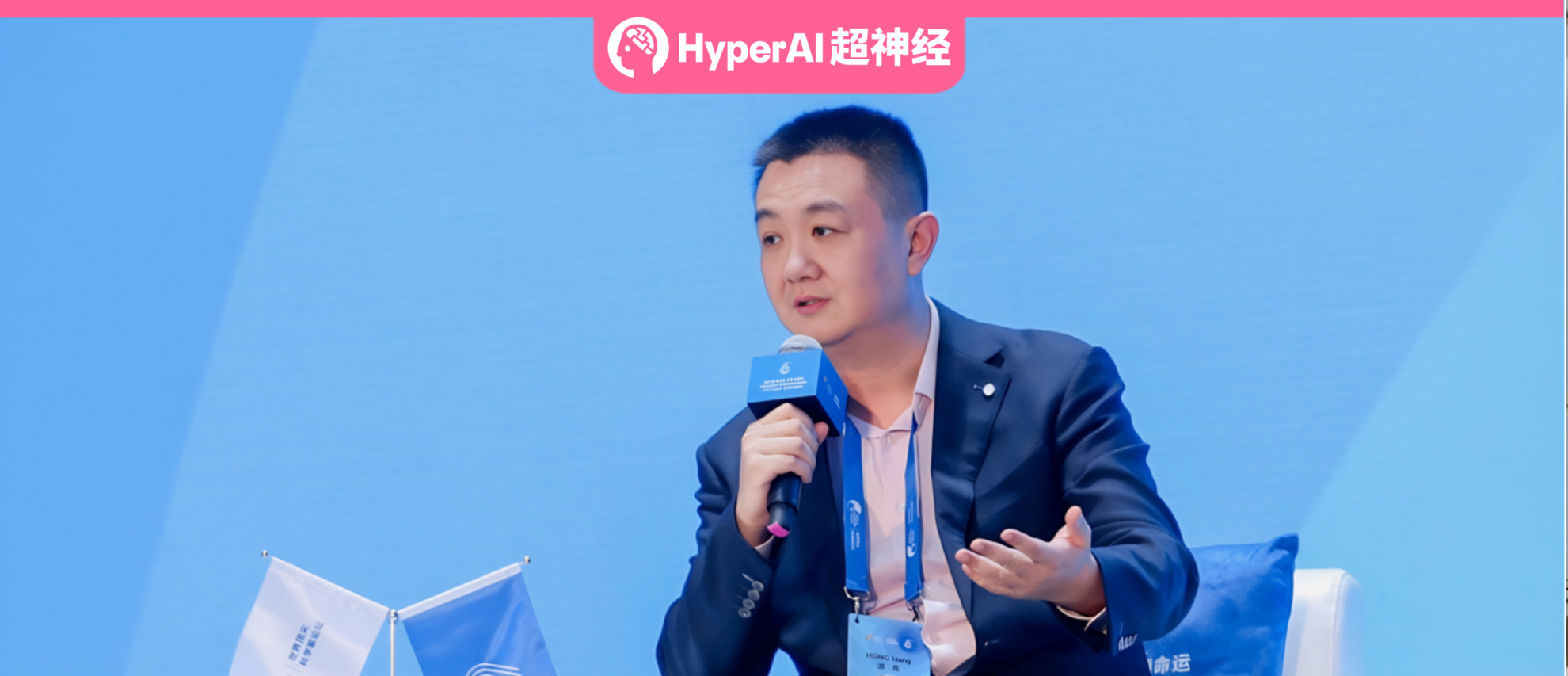
In recent years, with the new generation of information technology led by the new infrastructure being explored in thousands of industries, "industry-university-research-application collaboration" has frequently appeared in policy documents and industry development plans. This year's government work report clearly proposed to "deepen the integration of industry-university-research-application". The author believes that"Industry-university-research-application" can actually be divided into two areas: academia-research and industry-application.Academic research refers to education and scientific research, namely talent cultivation and scientific research; production and application refers to the implementation of innovative technologies in production links and actual application scenarios.
For a long time in the past, research and production were separated by a sea of differences. The academic community focused on high-tech breakthroughs, while the industry was limited by the actual pain points of "no cure". Subsequently, the importance of the transformation of scientific and technological achievements became increasingly prominent, and a bridge of communication and exchange was gradually built between industry and research. Today, a new wave of AI is surging, which is not only a powerful assistant for the industry to improve quality and efficiency, but also gradually reconstructing the scientific research paradigm. Against this background,The "two-way movement" between academia and industry has become a general trend.
on the one hand,Industrial AI applications focus more on the intelligent transformation of business processes and production methods, and fully autonomous and controllable AI tools or solutions are relatively scarce;on the other hand,The development of AI for Science has accelerated the progress of scientific research and has also spawned a large number of groundbreaking and innovative results, which urgently need more real data or application scenarios to verify their feasibility.
As Hong Liang, distinguished professor of Natural Science Research, School of Physics and Astronomy, and School of Pharmacy at Shanghai Jiao Tong University said:"In today's development environment, researchers are increasingly aware that publishing papers is no longer the only goal, and it is more important to focus on solving practical engineering problems."

Professor Hong Liang's open attitude towards the integration of industry and research is also reflected in the summer school activities he organized. Unlike most schools whose curriculum is completely centered around the display and sharing of academic achievements, Shanghai Jiao Tong University's AI for Bioengineering Summer School focuses on the field of protein engineering. It not only invited experts from Shanghai Jiao Tong University, Xiamen University, Fudan University, Sun Yat-sen University and Shanghai Artificial Intelligence Laboratory to share the latest research progress, but also jointly with Jinsai Pharmaceutical, Weilan Biotechnology and Zhongyuan Huiji, introduced the development progress of the AI bioengineering field in the industry from an application perspective.
It is worth mentioning that the participants of this summer school include not only experts and scholars from many domestic universities, but also R&D personnel from nearly 30 companies.Among them were team leaders and even chairmen of large companies. They listened to the entire three-day course together with the students present.This phenomenon, which is rarely seen in cutting-edge technology seminars held in schools/research institutes, appeared in university summer school activities, which fully demonstrates that the industry is full of enthusiasm for AI, especially "practical AI."
During the summer school, HyperAI had the honor of having an in-depth conversation with Professor Hong Liang. Starting from the development of AI in the field of protein engineering, he comprehensively sorted out the challenges and approaches of implementing AI for Science (AI4S), as well as how AI and Science can be organically combined.
Decisive transformation, earning an AI degree at Station B
Indeed, AI for Science has achieved rapid development in the past two years. Initially, some research groups explored AI tools on a small scale, trying to improve data processing efficiency. Today, in many scientific research fields, AI has become the key to breaking through research obstacles, and even its performance in many engineering tasks has surpassed the capabilities of human experts. As a result, AI practitioners have flocked to the scientific research field, and researchers have begun to teach themselves AI. There is no doubt that the removal of the barriers between AI and Science has spawned a large number of eye-catching scientific research results.But only a few actually step out of the laboratory.
In this regard, Professor Hong Liang said, "When doing AI4S, you should avoid weaving the emperor's new clothes, because sooner or later they will be exposed, so you should think about implementation." He said frankly,"It will be more fulfilling to successfully implement AI4S."
Professor Hong Liang used his own experience as an example to further analyze the charm of AI4S implementation. He was also engaged in basic scientific research at first, "I could only imagine how scientific research could be applied in practice in published articles." However, when his team ran the protein engineering general artificial intelligence and actually entered more than 20 companies to help them with the research and development of protein products, when he saw the molecules designed on his computer being produced in a 5,000L fermenter and used in real life,"At that moment, for the scientists who had done basic scientific research, the feeling of happiness was overwhelming."
It is worth mentioning that, at present,Based on the self-developed protein engineering Pro series large model, the team directly designs protein sequences based on functional requirements.We have already achieved the world's first and second large-scale model design and industrialization of high-difficulty protein products.
When talking about being the first to realize industrialization in the field of protein engineering research, Professor Hong Liang believes that the platform, personal academic background, research direction, etc. are all crucial.
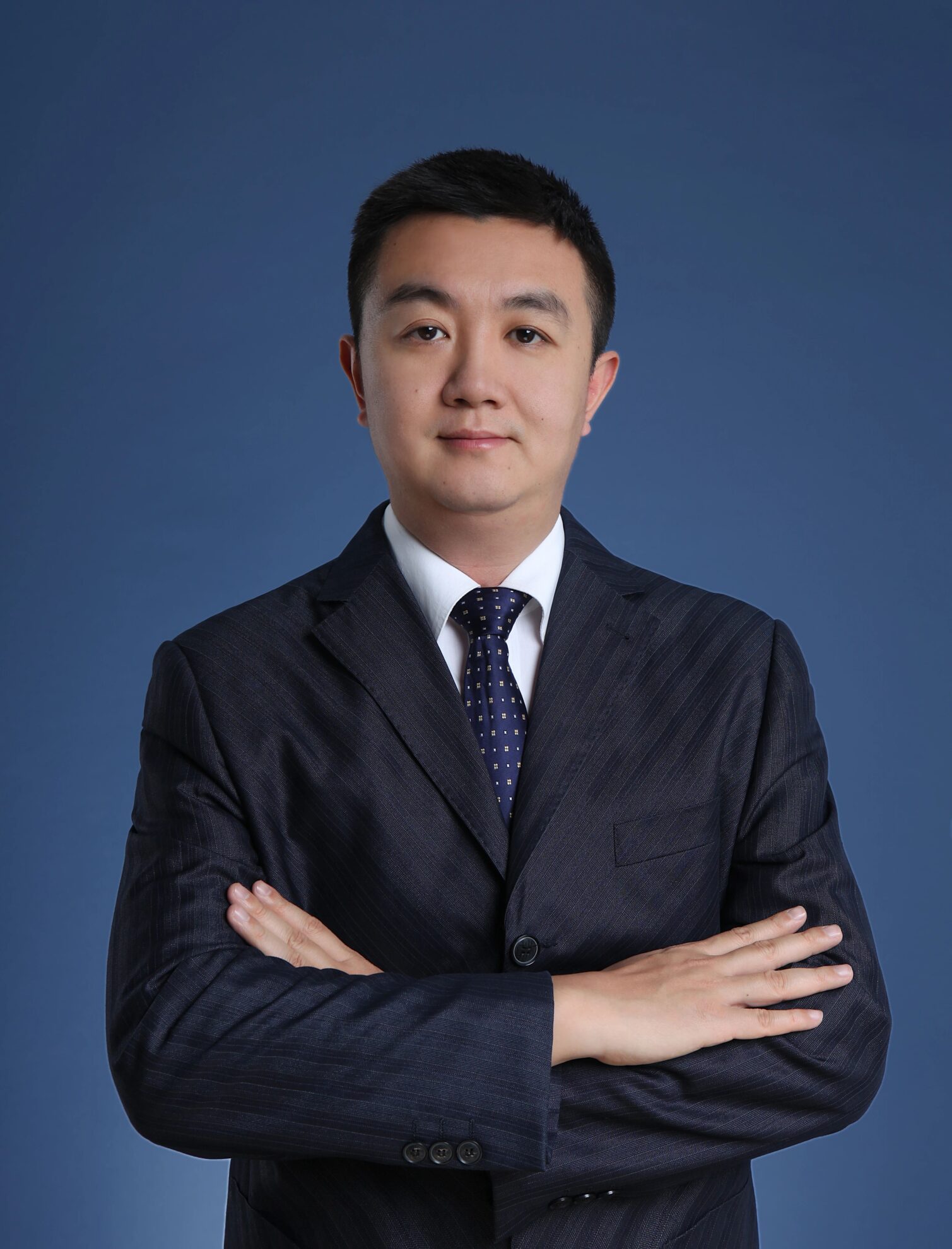
In terms of platform, Shanghai Jiao Tong University where he works is an institution known for its engineering majors. "I am very grateful to the school for supporting the research team in integrating industry, academia and research."The overall academic atmosphere of Shanghai Jiao Tong University is open and inclusive to industry, academia and research.The school will provide great material and spiritual encouragement to the research team in the process of promoting the implementation of results.
In addition, Professor Hong Liang's academic background and his deep insight into the development trends of AI are the cornerstones that prompted him to move towards industrial research and development.
He studied physics at the University of Science and Technology of China for his undergraduate degree, the Chinese University of Hong Kong for his graduate degree, and the University of Akron for his doctorate, focusing on the physicochemical properties, dynamics, and phase transitions of polymers and proteins.
In 2010, he went to the United States to continue his studies and joined the Oak Ridge National Laboratory as a postdoctoral student, focusing on protein structure, dynamics and function in the field of computational biology.Perhaps it was this experience in the United States that laid the foundation for his future main research direction - protein function. In 2015, he joined Shanghai Jiao Tong University as an independent PI to conduct molecular biophysics research.
In 2016, AlphaGo defeated South Korean Go champion Lee Jae-seok, becoming famous and showing the world the power of AI. In 2018, AlphaFold came out, bringing a huge shock to the biological field and making Professor Hong Liang start to think about how to combine his research direction with AI.
The real turning point came at the end of 2019, when the COVID-19 pandemic was raging. While at home, he took the AI course posted on Bilibili by Professor Li Hongyi of National Taiwan University and truly entered this seemingly mysterious emerging technology.Although he joked that he "got an AI degree from Bilibili", these 80 classes gave him a clearer understanding of AI.Then I decisively chose AI for Science.
"When industry technology changes, it is not subject to personal will."Faced with the unstoppable AI revolution, Professor Hong Liang began to combine AI, computing, and wet experiments to conduct protein design research in 2020. He switched from physics to chemistry, then from chemistry to biology, and finally from wet experiments to computing and artificial intelligence. It can be said that he followed the rhythm of technological leaps and took every step down to earth, so that he could finally lead the team out of the laboratory with experimental results.
The author believes that this is a true portrayal of "preparation is the key to success", but Professor Hong Liang said with a smile,"I'm pretty lucky."
As a researcher in the field of Science, he actively embraced AI and started with general knowledge to understand its mechanism, which laid the foundation for his exploration of AI4S. Luck was more reflected in the choice of research direction. "After deciding to do AI for protein engineering, I chose function among the three directions of structure prediction, dynamics and function."He admitted that "there is no product without function".
But this choice is actually very bold and full of uncertainty. "Even if a product is polished, it may not be able to be industrialized." But Professor Hong Liang was exactly 39 years old at the time, at the intersection of his thirties and forties, and he still maintained the passion to fight and the courage to start over.
"I was 39 years old at the time, and I could still try. If I failed, I would have wasted a few years and chosen a new direction to try again. Fortunately, we were lucky enough to finally get it working." From a technical perspective,"It is also a kind of luck that the data structure and data volume in the field of Science can match the technological progress of AI."
Although he attributed the success of industrialization to luck, there is no lack of analysis of the development trend of AI4S. For example, when he saw that AlphaFold had achieved such success in the field of protein structure prediction, and there was a business giant like Google behind it to support it, he was very excited about the epoch-making scientific achievement. However, after calm thinking and comprehensively considering the foundation and strength of his own team,After determining that he could not survive in the field of protein structure prediction, he resolutely gave up this research direction.
final,Professor Hong Liang chose the field of general artificial intelligence for protein engineering based on his own strengths.A notable feature of this field is that protein function data cannot be standardized, which is a challenge that is difficult to overcome in one go for researchers who only focus on computer science. Only experts with a deep background in related fields can make progress through continuous iterations of laboratory "wet experiments".
Fortunately, Professor Hong Liang's research team successfully "broke the barrier" in this field by relying on their years of accumulation in the interdisciplinary fields of computer science and protein, and became the first to taste the crab meat.
AI4S: AI should respect science, and Science should learn AI on its own
There is no doubt that the success of AlphaFold has greatly promoted and inspired the development of AI4S, and has broken the ice between AI and Science. However, the current development of AI4S is still in its infancy, and the two sides have not explored a universal cooperation model.The industry has also been thinking about this: AI for Science, is it led by AI or Science?
Although the industry has been calling for cross-disciplinary research talents in AI + traditional scientific research, its growth cycle is predictable and can be said to be something that can only be encountered by chance but not by force. The efficiency and design innovation of researchers in the field of AI in model construction, framework optimization, etc. are often difficult for scientific researchers to catch up quickly, while the precise positioning and analysis of scientific problems in vertical fields by researchers is difficult for AI to achieve quickly.
In comparison, Professor Hong Liang believes thatThe core of AI for Science lies in Science. Scientific or engineering problems must be defined first before AI solutions can be proposed.
Professor Hong Liang took DeepMind's team model as an example. Its expert team covers traditional scientific research fields as well as data science and computer science, completing a closed loop from scientific problem identification to AI method construction. The team building of his research group is similar to this. "In 2020, we called several CS students to work with us on AI for protein engineering. After the final implementation, they also had a new understanding of AI4S and felt a great sense of accomplishment."Hong Liang's research group has also recruited talents in the fields of CS and protein engineering to carry out collaborative research.
In practice, Dr. Zhou Bingxin, a core member of the team, introduced that students in the CS field would also come up with ideas and want to try to use AI to optimize a traditional scientific research method. At this time, CS would take the lead in feasibility discussions. Similarly, students in the protein engineering discipline would also clearly describe the scientific problems they found and seek solutions from AI. At this time, Science would take the lead in jointly finding innovative methods.
Professor Hong Liang and his team have well demonstrated the inclusiveness of the field of science. At the same time, he also suggested that researchers in traditional fields learn AI on their own, especially the heads of research groups."The team leader is like a business owner. The transformation from traditional scientific research to AI4S is like a strategic transformation within the company. It requires project establishment. It is very dangerous if the person in charge has no idea about new products and technologies."
However, he also said frankly that not all research directions can achieve breakthroughs with the help of AI at this stage, but the current national policy encourages AI to "impact" various scientific research fields. "I hope that teachers in the field of science, especially young teachers, will be bold in trying. If they are lucky enough to find a research direction that can be broken through, once the methodology is broken through, it will be a huge contribution to the field."
But it is worth noting that a successful impact does not mean that the industry can be implemented.
For example, AI is currently being used in the field of new drug development. From scientific research institutions to enterprises, from research to clinical trials, huge human and financial resources have been invested. Many start-ups have even failed to see the dawn of hope."The closed-loop time for AI drug design is too long."Professor Hong Liang explained, “Computational biology (physical computing + AI) often mainly helps researchers determine in vitro (molecular or cellular) experimental indicators, but the correlation between these in vitro experiments and the results of animal experiments may be very low. Even if the results of animal experiments are good, it does not mean that clinical trials can provide good feedback.”

This is also why,His team is more focused on the research direction of enzyme function."Molecular experiments are the final results and can be quickly verified in a closed loop. The research and development of excellent enzyme products can bring significant benefits to many fields such as food and beverage, beauty and skin care, washing and textiles, biomedicine, etc., and directly contribute to the main battlefield of the national economy."
In fact, in addition to scientific research teams like Professor Hong Liang who have actively transformed into AI4S, research teams in the field of AI also hope to make great strides in the field of AI4S, but the technical barriers they encounter are imaginable.Professor Hong Liang’s advice is to “find the right research direction, and don’t just show off your skills or mechanically apply large models.”
Specifically, he believes that "people in the AI field who are doing AI4S should first find research fields where input and output data can be standardized and structured, rather than imagining scientific problems." This is also the advantage of scientists, that is, "in research involving functions, the data is difficult to standardize and requires experimental iteration. If AI can reduce experimental costs, coupled with a deep understanding of scientific problems, it will make a huge contribution to the development of the industry."
Conclusion
During the interview with Professor Hong Liang, he mentioned "luck" more than once. In my opinion, "it's time, luck, and fate, and it's not something I can control." However, those who can truly seize opportunities must have forward-looking insights, efficient execution, and the courage to try and fail, which are also reflected in every choice made by Professor Hong Liang, and he ultimately settled on AI for protein engineering. I look forward to him and his team bringing more tangible results.
Finally, I would like to recommend an academic event! Click on the picture to learn more about the event↓
