David Baker's Latest Achievement! De Novo Design of Macrocyclic Peptide Binder Framework RFpeptides, Providing New Possibilities for Undruggable Proteins
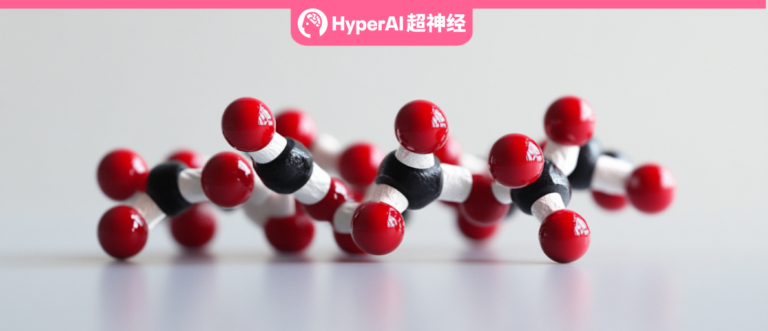
In the long struggle between humans and diseases, drug research and development has always been the forefront of scientific exploration. Among them, small molecule drugs have become the main force in drug research and development due to their advantages such as easy preparation, strong cell permeability, convenient oral administration, and low manufacturing cost. However, for proteins that lack deep hydrophobic pockets, small molecule drugs are often helpless. With the advancement of science, macrocycles, with their unique three-dimensional structure and high affinity, can regulate molecular targets that are difficult to reach with traditional small molecule drugs, providing new therapeutic possibilities for those "undruggable" proteins.
However, traditional drug development relies on the discovery of natural products or high-throughput screening technology, which is time-consuming and costly. The development of AI has brought new breakthroughs in drug design.The team of David Baker, an outstanding computational biologist and director of the Washington Institute for Protein Research, who won the 2024 Nobel Prize in Chemistry, has developed an innovative diffusion model-based technology, RFpeptides, to design high-affinity macrocyclic peptide binders for a variety of protein targets.
Specifically, the technology uses modified RoseTTAFold and RFdiffusion with loop relative position encoding to generate accurate macrocycle skeletons, integrates ProteinMPNN and Rosetta Relax for sequence optimization, and can achieve targeted and efficient macrocycle design, opening up new possibilities for drug development and diagnostic technology. The study is titled "Accurate de novo design of high-affinity protein binding macrocycles using deep learning" and has been published as a preprint on bioRxiv.
Research highlights:
* RFpeptides excels in designing macrocycles with different secondary structures, including α-helix, β-sheet, and loop conformations, which can be tailored to specific protein interfaces, advancing therapeutic and diagnostic applications
* RFpeptides designed macrocycles targeting MCL1, MDM2, GABARAP and RbtA all showed high binding affinity
* RFpeptides can design de novo binders for proteins with unsolved structures, changing the rules for designing targets for underexplored or uncharacterized proteins

Paper address:
https://doi.org/10.1101/2024.11.18.622547
Follow the official account and reply "大环设计" to get the complete PDF
The open source project "awesome-ai4s" brings together more than 100 AI4S paper interpretations and provides massive data sets and tools:
https://github.com/hyperai/awesome-ai4s
Dataset: Targeting MCL1, MDM2, GABARAP and RbtA, selecting target proteins for macrocycle design
In the de novo design study of macrocyclic binders, the research team chose MCL1, which plays a key role in anti-cancer treatment, as the first target protein.Using RFpeptides technology, the researcher generated 9,965 diverse cyclic peptide backbones and designed four amino acid sequences for each backbone using the ProteinMPNN and Rosetta Relax design processes. After deep learning and physical-based index screening, 27 designs were finally selected for experimental characterization.
The study also targeted MDM2, which interacts with the tumor suppressor protein p53.10,000 macrocyclic backbones were generated, and 4 amino acid sequences were designed for each backbone. Of the 40,000 designs predicted by AfCycDesign, 7,495 were considered to bind effectively to MDM2.
In designing macrocyclic compounds of GABARAP,The research team defined six hotspot residues, generated 20,000 macrocyclic backbones, and designed amino acid sequences. Of the 80,000 designs, 335 macrocyclic designs were selected for study.
For RbtA,The research team used AF2 and RF2 to predict its structure, defined 7 hotspot residues, generated 20,000 main chains, and designed 4 amino acid sequences for each main chain. During this process, iterative ProteinMPNN and Rosetta Relax were used in the design process.
RFpeptides: A generative deep learning pipeline for de novo design of macrocycles targeting target proteins
RFpeptides enables targeted and efficient macrocycle design, specifically, utilizing modified RoseTTAFold and RFdiffusion with loop relative position encoding to generate accurate macrocycle backbones, integrating ProteinMPNN and Rosetta Relax for sequence optimization.

RFpeptides: Further extension based on RoseTTAFold2 and RFdiffusion
The study first evaluated the modeling ability of the RoseTTAFold2 (RF2) structure prediction network for known cyclic peptide structures. As shown in Figure A below, the researchers made key improvements to RF2 by introducing a cyclic relative position encoding mechanism and observed that it achieved robust predictions of natural cyclic peptide structures.

Given this success, the researchers reasoned that cyclic relative position coding might also enable RFdiffusion to generate macrocyclic peptide structures due to its similar network architecture. Therefore, as shown in Figure BC above, this study added cyclic relative position coding to RFdiffusion and successfully observed the robust generation of diverse macrocyclic peptides.
Inspired by the transferability of cyclic relative position coding, the research team began to use RFdiffusion to design protein-binding macrocycles from scratch. As shown in Figure D below, the study used cyclic relative position coding in the RFdiffusion protein design process to provide coding for the generated chain. Then, as shown in Figure E below, ProteinMPNN was used to design an amino acid sequence that matches the macrocycle main chain, thereby completing the construction of RFpeptides. As shown in Figure F below, RFpeptides can rapidly generate macrocyclic compounds with diverse secondary structures against target proteins.

Macrocyclic compounds: refined screening based on RFpeptides design products
After using RFpeptides to generate diverse macrocyclic compound backbones for different targets, the study continued to use ProteinMPNN and Rosetta Relax to make local changes to the generated main chains to obtain diversity in amino acid sequences.
First, based on iPAE, model similarity assessment, and RF2-assisted screening, the researchers used AfCycDesign to re-predict protein-macrocycle complexes designed using macrocycle sequences and target structures as templates, and selected designs with high confidence.
Secondly, the study used Rosetta to calculate quality indicators such as binding affinity (ddG), spatial aggregation tendency of designed macrocycles (SAP), and molecular surface area of interface contact (CMS) to refine the screening of candidates.
Ultimately, the researchers selected a handful of screened designs, determined their binding affinities in experiments through chemical synthesis and biochemical characterization, and verified them through comparative testing to ensure the accuracy and effectiveness of the designs.
Near-perfect accuracy validates RFpeptides’ binding prediction power
Design and characterization of macrocyclic compounds targeting MCL1 and MDM2
In order to verify the effectiveness of RFpeptides, the researchers first selected myeloid cell leukemia protein 1 (MCL1) as the first target protein and conducted experimental characterization. As shown in Figure AB below,The researchers found that MCB_D2 (purple) bound most tightly to MCL1 (grey surface), exhibiting a binding affinity of 2μM.
To confirm whether the macrocycle binds in the designed manner, the researchers determined the X-ray crystal structure of MCB_D2 bound to MCL1.The crystal structure is almost identical to the designed model.The Cα RMSD is 0.7 Å. As shown in Figure D below, when the macrocycle is overlapped with the crystal structure, the Cα RMSD is 0.4 Å, and the side chain conformations of the interacting residues in the crystal structure are also very close to the designed model. In further crystal structure analysis, Figures E and F also reveal that the loop region of MCB_D2 has hydrophobic contacts and cation-π interactions with MCL1.

Inspired by the experimental verification of MCL1 binding to cyclic molecules, the research team then set out to design MDM2 binders. As shown in Figure GI below,The researchers found that MDB_D8 was the best macrocyclic compound for MDM2, showing a high affinity of 1.9μM.Furthermore, the key contact points at the interface predicted by computational modeling share similarities with the interactions observed in the native MDM2-p53 complex structure.

Design and characterization of macrocyclic compounds targeting GABARAP
To further analyze RFpeptides, the researchers next designed a compound with a completely different binding site to the structure of MCL1 and MDM2 - gamma-aminobutyric acid type A receptor-associated protein (GABARAP).
The experimental results show that, as shown in Figures AB and DE,Both GAB_D8 and GAB_D23 are effective compounds for GABARAP, showing affinities of 6nM and 36nM, respectively, among which GAB_D8 is the most effective macrocyclic compound for GABARAP found so far.As shown in Figures C and F below, X-ray crystal structure analysis found that the structure of the GAB_D8-GABARAPL1 complex is highly consistent with the design model, and the structure of the complex of GAB_D23 and GABARAP is also very consistent with the design model, which verifies the accuracy of the key interactions in the design model.

Although there are slight differences between the designed model and the crystal structure in some areas, the prediction based on multiple sequence alignment (MSA) shows a higher consistency with the X-ray crystal structure. As shown in Figure GI below, despite these slight differences, the results predicted by MSA are still more consistent with the experimentally obtained crystal structure.

Design and characterization of macrocyclic compounds targeting RbtA of unknown structure
The study also set out to design macrocyclic compounds for target proteins with unknown experimental structures to speculate whether RFpeptides can effectively reduce design risks. Taking RbtA as an example, the researchers first used AF2 and RF2 to predict its protein structure, and the two methods predicted similar overall structures. On this basis, the researchers chose to use RFpeptides to design almost identical regions predicted by AF2 and RF2. The results show that, as shown in Figure AB below,RBB_D10 is a potent macrocyclic compound of RbtA, displaying a binding affinity of 9.4 nM.

To confirm the binding mode between RbtA and RBB_D10, the researchers further analyzed the high-resolution X-ray crystal structure of apo and RbtA binding. As shown in Figure C above,X-ray crystallography confirmed that the apo structure is highly consistent with the predicted structure (RMSD 1.2Å and 1.1Å), and the complex structure shown in Figure D above is an almost complete match with the designed model (RMSD 1.4Å).As shown in Figure 4E-H above, the X-ray structure of the macrocyclic compound is almost consistent with the designed model (RMSD 0.4Å), which verifies the accuracy of the designed model.
David Baker: From the Hand of God to Nobel Prize Winner
As a heavyweight winner of the Nobel Prize in Chemistry, David Baker's name is undoubtedly a resounding symbol in the field of protein research. As one of the key figures in artificial intelligence prediction of protein structure, David Baker has not only greatly promoted the progress of protein structure prediction, but also achieved fruitful results in the field of protein design.
As early as 2003,David Baker's team has designed the first new protein that is not derived from nature, Top7.This achievement not only amazed the scientific community, but also represented an important breakthrough for humanity in the field of de novo protein design.
Original paper:10.1126/science.1089427
Although the design of Top7 is impressive, it is only based on a specific structure and has no actual function. David Baker did not stop there. He and his team continued to explore and tried various computational methods, including functions for calculating protein energy, multiple backbone and side chain sampling methods, and global optimization algorithms such as Monte Carlo simulation and continuous optimization methods.
With the development of generative AI and machine learning technologies, it is becoming possible to design new proteins with specific biological functions. In June 2024, David Baker's team made another breakthrough. They designed a new ring-shaped protein.It can regulate the fibroblast growth factor (FGF) signaling pathway and promote vascular differentiation. This work not only broadens the application scope of protein de novo design, but may also have a profound impact on the development of this field.
Original paper:
https://www.cell.com/cell/fulltext/S0092-8674(24)00534-8
David Baker's research has greatly advanced the field of protein design. His breakthrough in de novo protein design indicates that we are on the threshold of a new era, an era in which humans can precisely manipulate the basic building blocks of life. The development and application of these technologies are expected to solve a series of global challenges.
